Indicators of Complexity and Over-Complexification in Global Food Systems
- 1Department of Geography, Environment, and Geomatics, Arrell Food Institute, University of Guelph, Guelph, ON, Canada
- 2Global Institute for Water Security, University of Saskatchewan, Saskatoon, SK, Canada
Global food systems have increased in complexity significantly since the mid-twentieth century, through such innovations as mechanization, irrigation, genetic modification, and the globalization of supply chains. While complexification can be an effective problem-solving strategy, over-complexification can cause environmental degradation and lead systems to become increasingly dependent on external subsidies and vulnerable to collapse. Here, we explore a wide array of evidence of complexification and over-complexification in contemporary global food systems, drawing on data from the Food and Agriculture Organization and elsewhere. We find that food systems in developed, emerging, and least developed countries have all followed a trajectory of complexification, but that return on investments for energy and other food system inputs have significantly declined—a key indicator of over-complexification. Food systems in developed countries are further along in the process of over-complexification than least developed and emerging countries. Recent agricultural developments, specifically the introduction of genetically modified crops, have not altered this trend or improved return on investments for inputs into food systems. Similarly, emerging innovations belonging to the “digital agricultural revolution” are likewise accompanied by energy demands that may further exacerbate over-complexification. To reverse over-complexification, we discuss strategies including innovation by subtraction, agroecology, and disruptive technology.
Introduction
Since the advent and rapid proliferation of industrialized agricultural practices in the mid-twentieth century, global food systems have consistently succeeded in producing more food, overall and per-capita, every year (Figure 1). While this is a tremendous technological achievement, these gains have required immense investments of social and ecological capital, and as such, have been accompanied by great environmental and societal costs (Campbell et al., 2017). The agri-food industry is among the largest contributing sectors to numerous global challenges, including climate change, biodiversity loss, and freshwater contamination (Hajer et al., 2016; Evans et al., 2019). Global malnutrition and hunger are likewise high and on the rise, and the ability of our existing food systems to continue to feed current and future populations is questionable, given the expected environmental impacts of climate change on crop yields and the various systemic supply chain vulnerabilities highlighted by COVID-19 (Dawson et al., 2016; Laborde et al., 2020). As such, many scholars argue that nothing less than a rapid and radical transformation of global food systems is necessary (Rotz and Fraser, 2015; Searchinger et al., 2019; Rockström et al., 2020).
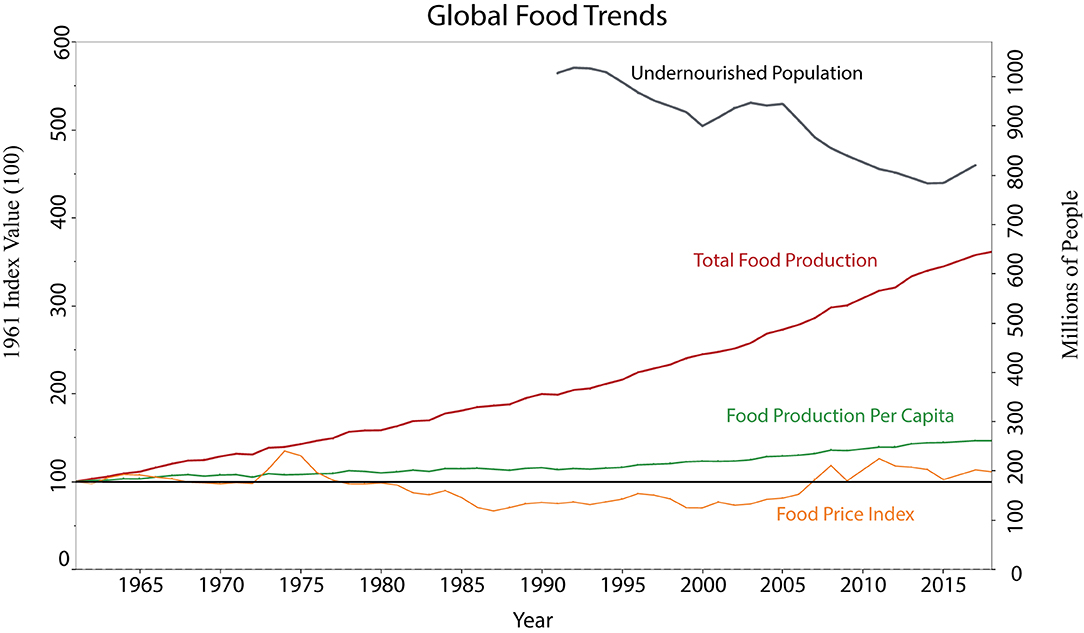
Figure 1. Trends in global food systems illustrate global successes in producing more food every year, both in total and per-capita, while keeping food prices relatively stable (left axis). Undernourishment has dropped modestly during this period but remains a significant problem (right axis). Food production data are standardized to a 1961 index value of 100. See the Supplementary Material for source data.
In this paper, we offer an innovative analysis of the successes and challenges of global food systems framed by historian Harold Tainter's theory of societal complexification (Tainter, 1995, 2006). Tainter defined complexification somewhat differently than is currently used in such areas as ecology and complex systems theory; he defines it as a strategy for solving problems, specifically solutions and technologies that require increased specialization of social roles and institutional hierarchies, greater technical competencies, larger scales of integration, increased use of energy, and increased production and flow of information (Flannery, 1972; Tainter, 1995, 2006; Strumsky et al., 2010). In this sense, complexification is an historically ubiquitous human strategy for adaptation and problem solving, but it is also costly, because increases in complexity generally require attendant increases in energy investments (Boserup, 1975; Tainter, 1995; Pelletier et al., 2011).
Some studies of past societies suggest that sustained complexification can become maladaptive and lead to diminishing returns (Tainter, 2006; Strumsky et al., 2010) (Figure 2). Tainter calls this over-complexification: a stage of development in which investments in complexity produce few benefits, increase dependence on externalities, and make societies more vulnerable to collapse (Tainter, 2006; Fraser, 2011; Angeler et al., 2020). As the costs and pitfalls of over-complexification become evident, Tainter argues, people begin to reject complex solutions and turn instead toward simple or traditional ones.
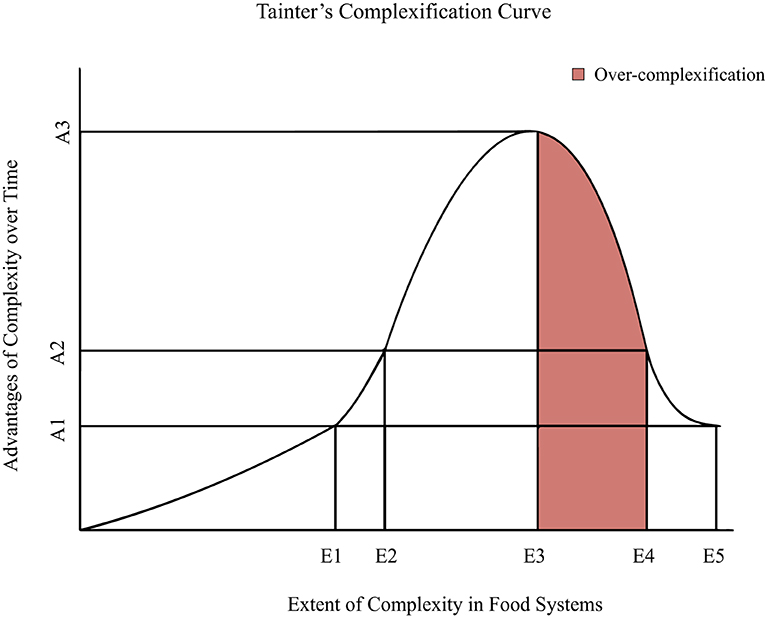
Figure 2. Tainter's theoretical relationship between complexification and efficiency. Some complexification in society is adaptive, e.g., technological innovations (A1,E1) which favorably impact the relationship between inputs and outputs (ROI). However, there is a threshold (A2,E2) at which the introduction of new technologies, while still beneficial to overall productive output, begin to have less benefit. Eventually, a threshold of over-complexification is reached (A3,E3), beyond which (shaded area) new investments in complexity are more costly than they are worth, and as such, can only be sustained by external subsidies. Historically, this is the point at which past societies have started on the pathway to collapse. If corrective action is taken (A2,E4), the relationship between complexity and energy can be stabilized to balance costs and advantages (A1,E5). Adapted from Tainter (1995).
Below, we draw on a wide array of quantitative data to explore the extent of complexification in global food systems and evaluate whether there is evidence of over-complexification. First, we present evidence that the recent history of global food systems has been one of ever-increasing complexification, beginning with mechanization in the developed world in the early twentieth century, and most recently taking such forms as genetic modification and globalization of supply chains. Then, we explore evidence of over-complexification; following Tainter, we focus on return on investments (ROI) for energy (E-ROI) as well as for mechanization, irrigation technology, chemical-based fertilizer and pesticides, and most recently, adoption of genetically modified (GM) crops (Woods et al., 2010; Pelletier et al., 2011). We also discuss evidence of societal rejection of complexity, specifically in the forms of local and alternative food movements and GM skepticism.
Note that we recognize that “global food systems” comprise a heterogeneous assemblage of systems for food production, distribution, processing, and marketing, some of which exhibit tighter local and regional couplings than others. As such, we explore evidence of complexification globally, broken down by developed countries, least developed countries, and emerging countries, and also for the four top GM-adopting countries (which we take as an indicator of advanced complexification): Argentina, Brazil, Canada, and the United States. Given the presumptively transformative nature of GM technology, our assumption is that any evidence of benefits of GM crops to E-ROI will be particularly evocative regarding the state of complexification in these nations.
We couch our findings in a discussion of emerging trends in agribusiness, such as digital agriculture, vertical farming, and the use of big data. Given these unfolding innovations, and given too the urgency to rapidly transform global food systems, our discussion is both timely and critical (Weersink et al., 2018; Rotz et al., 2019). As such, we conclude by exploring existing and emerging strategies for reversing over-complexification in food systems, including innovation by subtraction, disruptive innovation, and agroecology.
Methods
For the purposes of this analysis, we define complexification as any strategy for problem-solving that requires greater specialization of social roles and institutional hierarchies, greater technical competencies, larger scales of integration, increased use of energy, and increased production and flow of information (Flannery, 1972; Tainter, 1995, 2006; Strumsky et al., 2010). By this definition, GM technology, for example, is more complex than traditional breeding because it involves laboratories, advanced technologies, specialized academic knowledge, and introduces more complicated corporate hierarchies and intellectual property considerations into the supply chain (Mueller and Flachs, 2021). It is important to note for the purposes of disambiguation that the definitions of complexity and complexification used here are different from those found in other disciplines, such as algebra or complex systems theory. The latter, for example, defines complex systems as having few similar parts and rules that interact to create emergent phenomena, feedbacks, and self-organization (Ashby, 1947; Von Bertalanffy, 1972). Complexity, in this sense, can be rather elegant and is a central feature of social and ecological systems. Complexity as used by Tainter, and therefore as used here, relates more closely to how the word is used in the vernacular: as a synonym for complicatedness.
Complexification in agricultural production systems and supply chains takes a variety of forms, and as such, is difficult to measure with a single indicator (Serdarasan, 2013; Lin et al., 2019). While we know of no single established indicator of food systems complexification, scholars have identified multiple technological and social trends that contribute complexity to food systems. Below, we examine trends in total energy inputs (Pelletier et al., 2011), proliferation of mechanization and irrigation (Sassenrath et al., 2008), adoption of genetically modified crops (Rótolo et al., 2015), trophic level of protein in the food system (Bonhommeau et al., 2013), aquaculture orientation of fisheries production (Troell et al., 2014), export-orientation of national agricultural systems (Porkka et al., 2013), and transnationality of agribusiness (Senauer and Venturini, 2005) as potential evidence of complexification.
Unless otherwise noted below, we examine these trends globally and clustered into three categories: least developed countries, developed countries, and emerging countries, using the definition provided by the United Nations for the first two and the definition for the latter provided by the OECD. There is no overlap among the countries in each category. In addition, we also explore complexification at the national level for United States, Canada, Argentina, and Brazil. We selected these four countries for targeted analysis because they are the four top GM-adopting nations in the world, which as noted in the Introduction we interpret as an indicator of advanced complexification.
Irrigation adoption is calculated as ratio of cropland under irrigation to total cropland. Mechanization adoption is the number of tractors per 1,000 Ha. GM adoption rates are calculated as cropland under GM production (ha) divided by total cropland under production (ha). Trophic level of protein is explored via livestock density, which we calculate as number of animals per 1,000 ha arable land, and as the ratio of reared animal protein to total protein produced. Aquaculture orientation of fisheries is calculated as the proportion of total aquaculture production (tonnage) to the sum of total aquaculture production (tonnage) and wild capture fisheries (tonnage). Export orientation is the ratio of total export value to agricultural production value, both in USD. Transnationality is an index calculated by UNCTAD World Investment Reports as the average of three ratios: foreign assets to total assets, foreign sales to total sales, and foreign employment to total employment (United Nations Conference on Trade and Development, 1998). Here, we explore the trend in average transnationality index for corporations involved in agri-food (following Senauer and Venturini, 2005).
All data noted above, save transnationality and GM crop adoption were retrieved from the “Data” section of Food and Agriculture Organization (FAO)'s FAOSTAT database in 2020 (Food Agriculture Organization, 2020). Data for the adoption of GM Crops were retrieved from the International Service for the Acquisition of Agri-biotech Applications (ISAAA)'s Global Status of Commercialized Biotech/GM Crops briefs (Aldemita et al., 2015; ISAAA, 2019). Additional details regarding the variables accessed and any conversions applied can be found in the accompanying Supplementary Material.
Lacking an existing, established measure of food systems complexification, it is not possible to replicate the theoretical complexification curve in Figure 2 with real data. As such, and following Tainter (1995, 2006), our analysis focuses primarily on return on investment (ROI) and return on energy investment (E-ROI) in tandem with technology trends, as the indicator of over-complexification. In other words, we interpret the various individual indicators of complexification discussed above via qualitative triangulation (Jick, 1979), rather than one by one or in a comparative way. Based on Tainter's theory, if ROIs are declining while complexity is increasing, the system in question likely falls somewhere between points E2 and E5 on the curve shown in Figure 2. Triangulating across multiple indicators and trends also helps address the fact that the data for the individual indicators have different time depths based on available data.
We calculate all ROIs by evaluating the ratio between total output and total input, including for total energy inputs (E-ROI), as well as for return on N fertilizer inputs, and pesticide inputs. We do so for all years for which data are available (see the Supplementary Material for more details). The following formula template is used for all ROI calculations:
Total calories produced annually is calculated for each organizational level (e.g., global, national) as follows:
where x is the organizational level of interest, y is the year, C is per-capita daily calories produced, P is population, and D is the number of days in the year.
Resource utilized is in Terajoules for E-ROI, tons for fertilizer ROI, and tons for pesticides ROI. Appropriate conversation techniques were used to convert Terajoules to Joules and Tons to Grams to present the numbers in the simplest form.
A final way that we explore the global trend of food systems complexification, in part to compensate for the lack of data prior to 1970, is by creating a chronosequence from the collective 147 years of calculated data for E-ROI in least developed, emerging, and developed countries. A chronosequence is a single data series compiled from multiple sites that represent different ages or stages of development, using place-for-time substitution. For example, if you have 10 years of data regarding plant regrowth after a fire in two locales, one that experienced fire 10 years prior and another that experienced fire 20 years prior, a chronosequence could be created by combining the two datasets to back-cast the early recovery in the older regrowth area or to forecast the next decade of recovery in the younger site.
Here, we use the actual data for E-ROI in emerging and least developed countries to reconstruct historical values for E-ROI in developed countries. This is relatively easy to achieve, because all three E-ROI curves are downward trending, and the highest value on each group's curve is 1970. To create the single curve for developed countries we simply shift backward in time the curve for emerging countries, so that the lowest E-ROI value on the emerging countries' curve aligns with its closest match on the developed countries' E-ROI curve. Next, we do the same to shift the E-ROI curve for least developed countries to before the curve for emerging countries, again matching the curves at their closest values. The result is a single E-ROI curve for developed countries starting at 1901, using the values for least developed countries for 1901–1920, the values from emerging countries for 1921–1969, and the actual developed countries data from 1970 onward. See the Supplementary Material for more details.
Results
While data availability varies depending on the indicator in question, numerous technological indicators offer collective evidence of increasing complexity in our food systems over the past century (Figures 3A–D), including mechanization, reliance on reared animal-based protein, the proliferation of aquaculture, the extensification of trade and globalization of supply chains, and the adoption of GM crops.
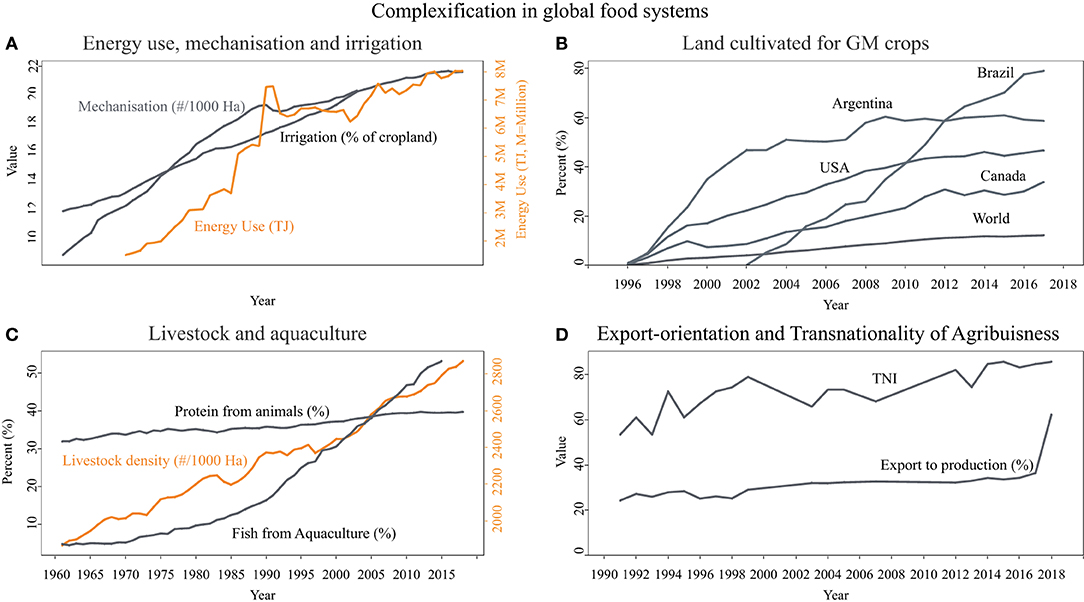
Figure 3. Evidence of complexification in global food production, including: (A) increased mechanization and irrigation (left axis) and energy use (right axis); (B) adoption rates for GM crops globally and for the top four adopting countries; (C) increased reliance on animal-based protein and aquaculture (left axis) and livestock density (right axis); and (D) growth in the export-orientation of food systems, shown as the percentage of exported calories to total calories produced (update of Ercsey-Ravasz et al., 2012) and trans-nationality index (TNI) for the largest corporations in agriculture (update of Senauer and Venturini, 2005).
Per Tainter (2006), whether these investments in complexity have been adaptive, or have led to over-complexification, depends on the ROIs associated with the progressive adoption of these increasingly complex technologies and processes. If these investments in complexity have been adaptive (that is, occupying the space between E1,A1 and E3,A3 in Figure 2), we would expect to see ROIs that are stable or increasing. However, our analysis finds consistent patterns of declining ROIs at both global and national levels (Figure 4) for the entire period for which data are available. The various ROIs evaluated here all appear to be relatively stable for developed countries, but this is likely for two reasons: first, because the available data do not extend back far enough in time to reflect the steep increases in energy use and concomitant declines in E-ROIs associated with mechanization and adoption of other technologies in developed countries before 1970; second, declines in ROIs over time will necessarily be smaller from year to year as overall efficiency approaches a theoretical limit of zero.
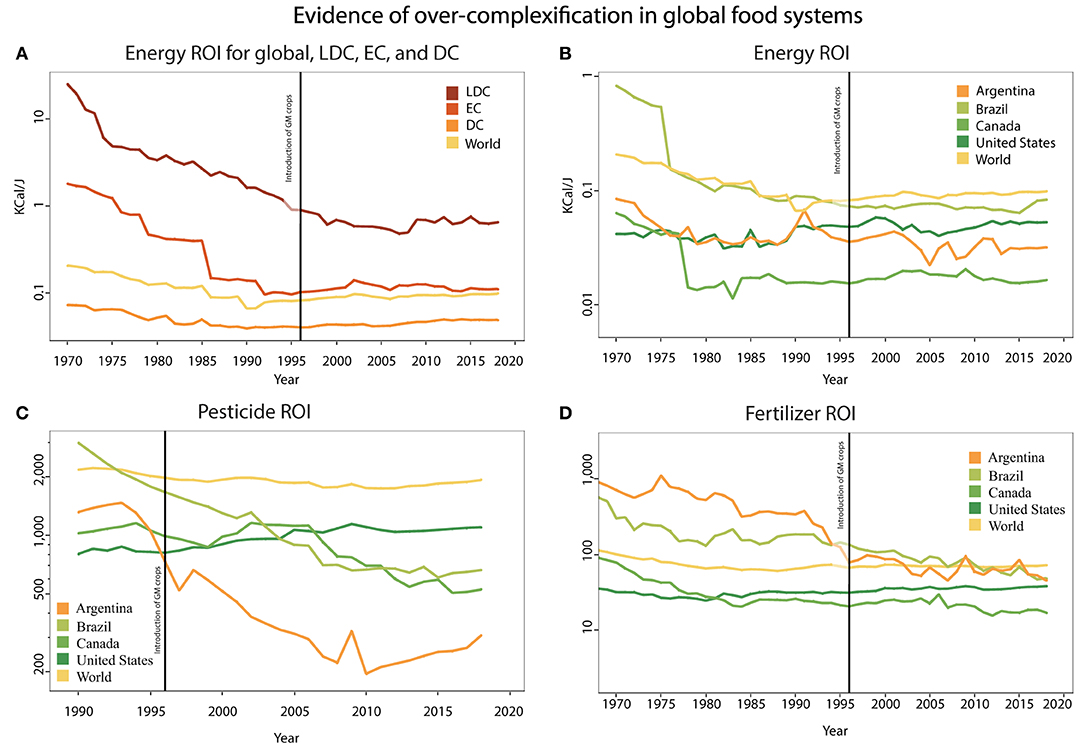
Figure 4. Decreased return on investments in global agriculture. E-ROI for: (A) global and grouped by OECD categorization of developed countries (DC), emerging countries (EC), and least developed countries (LDC); (B) E-ROI for Argentina, Brazil, Canada, and United States; and, ROI for pesticide use (C) and fertilizer use (D) for Argentina, Brazil, Canada, and United States and globally. Data are plotted on a logarithmic scale to ensure comparability and account for the necessarily asymptotic nature of the data, so that the more dramatic patterns seen for LDCs and ECs do not dampen the trends found for DCs. Note there is no evidence of a change in ROI trends following the introduction of GM crops (vertical line at 1996).
A second indication of over-complexification of global food systems is that there is no clear evidence that the introduction and proliferation of new GM technologies have improved E-ROI. Proponents of GM technology often describe it as a game changer for global agriculture, often specifically in terms of increased yields and efficiency and reduced costs and chemical inputs (Paul et al., 2018). While some of these benefits may accrue to producers, their widespread adoption appears to have had no noteworthy, system-level effect on the relationship between energy inputs and caloric outputs, whether globally or for the top four GM-adopting countries. E-ROI for the top four GM-adopting countries show no marked improvement following 1996, the year that GM adoption began (Figures 4B–D). Neither do you see a noteworthy trend change past 2005 for Argentina, the year that adoption rates exceeded 50% in that country. Some improvements are seen on the ROI for pesticides in the US, but this trend starts prior to 1996 and can be better explained by reductions in overall pesticide use that started in the US in 1992.
The single, back-casted chronosequence for developed countries (Figure 5) is particularly evocative in that it suggests a single historical process of food system complexification in developed countries that begins at the turn of the twentieth century. Based on these data, we propose that the period of widespread mechanization in the first quarter of the twentieth century marks a transition for food systems in developed countries past theoretical point A2,E2 in Figure 2—at which ROI rates on new investments begin to decline—and that the introduction of industrial farming methods and chemical inputs following World War II marks point A3,E3 in Figure 2—the threshold at which additional investments in complexification become maladaptive. This agrees with other discussions regarding agricultural transitions during this period (Berry, 1982; Kimbrell, 2002).
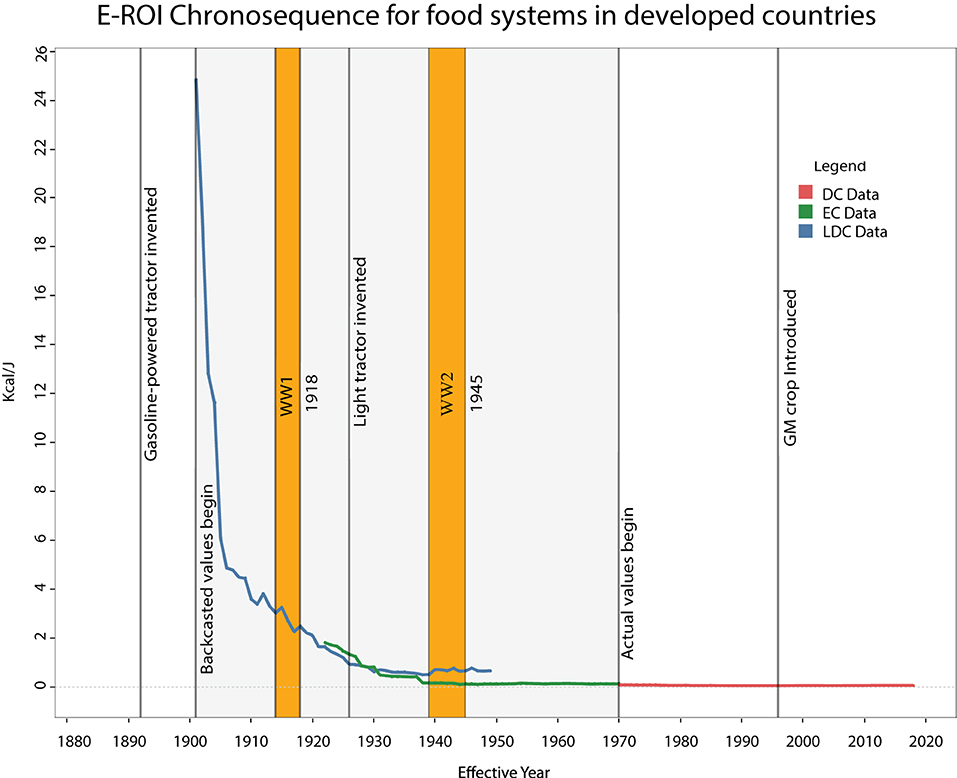
Figure 5. A combined chronosequence of E-ROI data for Least Developed (LDC), Emerging (EC), and Developed (DC) countries offers a theoretical model for the complexification of DC food systems in the twentieth century, beginning with the introduction of mechanization and widespread irrigation, and then continuing through the addition of chemical inputs and industrial methods post-WW2. Data for DC represent actual values (beginning at 1970), whereas EC and LDC are year-adjusted to effectively back-cast the trend in DC using place-for-time substitution (see methods). We propose that DC food system transitions reached the point of over-complexification, where new investments in complexity began to be maladaptive, roughly around the end of WW2.
Discussion
Global food systems all exhibit strong evidence of progressive complexification since 1970, taking such forms as technological innovation, an increase in trophic level of consumed protein, and the global extensification of supply chains. This is not, on its own, surprising, as others have also commented on the challenge of increased complexity in contemporary global food systems (Sundkvist et al., 2005; Sassenrath et al., 2008; Ercsey-Ravasz et al., 2012; Rotz and Fraser, 2015). As to whether global food systems have entered the stage of over-complexification, however, the lack of any apparent benefits to ROIs from GM crops is one compelling piece of evidence that we identify here that suggest that this is the case. It is reasonable to expect that the effects of such a potentially transformative technology as GM would be seen in ROI at this scale, considering that as of 2018, adoption rates are high for all four major GM-adopting countries (Figure 3C). Whether this is a failing of GM technology to live up to its promise, or a system-wide phenomenon, wherein over-complexification of the system is dampening the realization of the full benefits of GM, requires further research.
The novel chronosequence also supports a hypothesis of over-complexification in developed countries, evident in the progressive decline of E-ROI over the last century. This finding could at first seem paradoxical, given the productive growth of these systems noted in the Introduction and Figure 1. We believe that our focus on energy allows us to reveal the hidden externalities behind this growth. In a thermodynamic sense, energy must come from somewhere. Arguably, the lack of attention in conventional agricultural production to the regenerative capacity of agroecosystems, and the economically inexpensive and politically expedient availability of energy subsidies and transfers from other locales, has allowed complexification to progress more or less decoupled from ecological feedbacks (Hagens, 2020).
The case for over-complexification is further compelling when considering recent trends of resistance against such new technologies as genetic modification, as well as in the proliferation of alternative food movements (AFM) (Trivette, 2012; Rótolo et al., 2015; Witter and Stoll, 2017). These movements, which include a diverse tapestry of social actions, align with the pattern described by Tainter where people respond to diminishing returns and inefficiencies by rejecting complex solutions in favor of simpler ones. Though diverse, AFMs all generally share in their rejection of the various inefficiencies and externalities of the global agri-food regime, favoring shorter and less complicated supply chains, less reliance on chemical and technological inputs, and greater equity in participation, power, and wealth (Kloppenburg and Lezberg, 1996; Schnell, 2013). Indeed, AFMs have become so robust in recent years that they provided critical resilience to food systems around the world during the early months of the COVID-19 pandemic, when global food supply chains were deadlocked (Stoll et al., 2021; Thilmany et al., 2021).
Continuing Trends in Complexification
Despite these emerging alternatives, the global trend of food system complexification is arguably poised to continue, given that GM crops still only account for roughly 10% of the global total, and given that agribusiness continues to aggressively promote GM technology as a solution to climate change, food insecurity, and poverty in least developed and emerging countries. While GM technology may indeed have the potential to be transformative, the evidence presented here suggests, at a minimum, that our current food systems are so overcomplicated that this potential is undermined.
Likewise, in developed nations, start-ups and industry leaders alike are pursuing various new technological innovations for agriculture, including drones, big data, lab-grown proteins, and vertical farming. While these solutions appear compelling in that they leverage the latest in technological innovation, there is a risk that they will do little more than continue the ongoing pattern of over-complexification and push global food systems into an even more tenuous position.
One such innovation is distributed ledger technology (DLT), commonly known as blockchain, a secure data provenance technology which has been identified as a potential solution to many of the informational challenges created by increasingly complex global supply chains (Kamilaris et al., 2019; Pearson et al., 2019). As supply chains have become increasingly complex, informational feedbacks within them have weakened (Sundkvist et al., 2005; Brunori et al., 2016). DLT offers a way to achieve increased transparency and information flow through supply chains without requiring centralization and high operational costs. However, DLT can be exceptionally energy consumptive because of the computing resources it requires (Pearson et al., 2019; Sedlmeir et al., 2020). Any potential gains in supply chain efficiency from DLT must be weighed against the additional costs of adoption across supply chains.
A related challenge may exist for the use of smart devices and other digital technologies such as microsensors and cloud-connected farming equipment with access to large datasets (Weersink et al., 2018). The energy investments required for producing and operating these devices may be small at the level of the individual device but could be significant when the technology is scaled up. That is, there is a risk that widespread adoption of such devices, particularly in developed countries where it is reasonable to assume adoption rates will be highest, could create an escalating pattern of energy use known as the Jevons Paradox (Alcott, 2005). Jevons Paradox describes a scenario where a new technology designed to increase resource efficiency ultimately increases overall use because adoption rates exceed efficiency gains. Jevons Paradox has been predicted as a consequence of the proliferation of smart devices (Corcoran, 2012), and has already been observed in agriculture for irrigation technology and agricultural land productivity (Sears et al., 2018; Ceddia, 2019).
Thus, an unanswered but critical question regarding the nascent digital agricultural revolution is whether these new innovations will serve to disrupt existing food systems in a way that can reverse over-complexification and improve return on energy and other natural resource investments, or if they will further contribute to the over-complexified, coerced, and vulnerable nature of global food systems. Note that we are not arguing that each food system innovation developed in the last century is inherently problematic, but that they have collectively become problematic in a cumulative pattern of over-complexification.
Conclusion: Getting Complexity Under Control
Given the tight couplings among multiple agricultural and agri-food sectors, failure of a single coerced regime could easily prompt a devastating cascade effect through multiple adjacent sectors (Rist et al., 2014; Mehrabi and Ramankutty, 2019; Davis et al., 2021). Given, too, that the majority of scenarios for global environmental change include increased frequency and severity of stochastic disruptions—such as storms, wildfires, and pandemics (IPCC, 2018)—we believe that rapid corrective actions to reduce complexity in global food systems are necessary.
One strategy for reducing complexity is “innovation by subtraction:” solving problems not by adding complexity but by removing it (Goldenberg et al., 2003). In the world of consumer electronics, reducing the number of buttons on devices (or even eliminating them outright) is a well-known example of innovation by subtraction. In food systems, one example of innovation by subtraction involves moving away from long-distance transport and year-round availability of fresh foods. Long and overly complex supply chains have high operational costs, and damaged and unsold fresh foods are a major component of food wastage. Instead, supply chains could eliminate fresh and out of season options in favor of locally sourced and processed alternatives, which can be as high in quality, if not higher, than fresh options that have traveled long distances (Miller and Knudson, 2014; Kristin et al., 2017).
Simplifying supply chains is a second example of innovation by subtraction, as shorter supply chains are generally more resilient and resource efficient (Sundkvist et al., 2005; Brunori et al., 2016). Direct marketing and community-supported agriculture and fisheries are excellent examples: strategies wherein producers take on more of their own marketing and distribution practices, eliminating wholesalers and focusing more on a regional customer base (Witter and Stoll, 2017). Implemented together, the transition to shorter supply chains and reduced long-distance transport of fresh foods could address multiple inefficiencies in global food systems, including food wastage, energy costs, wage and other social inequities, and the overuse of plastics in the supply chain. Shorter supply chains in both agriculture and fisheries proved to be resilient during the COVID-19 pandemic while global systems faltered (Stoll et al., 2021; Thilmany et al., 2021).
Transitioning to agroecology—the reduction or removal of the need for chemical inputs, in favor of reliance on existing ecosystem processes for soil nutrient cycling and pest management—is a third, systemic form of innovation by subtraction (Altieri, 1995; Tittonell et al., 2020). While critics have gone to great lengths to deem AFM and agroecological production systems “dead on arrival” by comparing their overall productive capacity to existing regimes, these assessments are often inaccurate or misleading because their singular focus on increasing production ignores the portfolio of goals espoused by these alternative approaches (Chappell and LaValle, 2011; Reganold and Wachter, 2016). While agroecology has largely been explored at a small scale, its potential for scaling up and out is extensive, especially in developing countries (IPES-Food, 2020; Tittonell et al., 2020). Expanding our assessments of food systems innovations to include a variety of social and environmental indicators, from quality of employment to greenhouse gasses and water quality, is essential if we are to effectively identify sustainable solutions that decomplexify our food systems while also addressing current and future food needs (Reganold and Wachter, 2016; Bennett et al., 2021).
Globally, the agricultural landscape is a highly heterogeneous and bi-polar mix of under- and overdeveloped systems. Some systems, primarily those in DC, have grown too complex; others, especially those in LDC of the global south, are less complex but are being coerced along the same trajectory by global agri-food interests. As such, there are still opportunities to adopt agroecological approaches in LDC to avoid the costs and vulnerabilities of over-complexification (IPES-Food, 2020).
Writing on the challenge of reversing over-complexification, Tainter (2006) argues that a key, but seemingly counterintuitive strategy is to stop trying to solve problems. Ostensibly, the “problem” that agri-food interests have been continuously trying to solve is to feed people; but, in practice this goal has become conflated with the goal of growth in food production (Tamburino et al., 2020). Social scientists have long pointed out that the drivers of hunger and malnutrition are principally political and economic in nature, and do not generally involve insufficient production (Sen, 1983); when the amount of food currently wasted and devoted to animal feed is taken into account, global food production is already sufficient to feed 10 billion people (Holt-Giménez et al., 2012). Not solving the problem, in this case, would not mean abandoning the goals of feeding people or solving hunger, but moving away from the assumption that the most important action for meeting these goals now or in the future is always to grow more food. How might the cost-benefit analyses of complex innovations like vertical farming change if we remove the mandate to increase food production at all costs?
Importantly, our argument here is not against the use of new technology, but that new innovations in food systems should be carefully evaluated as to whether they continue the trend of over-complexification or disrupt and reduce it. For example, social media and other internet platforms have been critical to the disruptive success of AFMs (Stevens et al., 2016). It is likewise possible that other new technologies currently being pursued—e.g., drones and automated devices—could similarly be leveraged by actors seeking to disrupt maladaptive complexity in existing systems.
Our goal with this examination of global food systems is not to measure their precise state of vulnerability or nearness to collapse; neither are we proposing that societies simply walk away from complex solutions. Rather, as Tainter (1995) argues, the first step in pursuing sustainability is recognizing where we are in our own historical trajectory. The data presented here provide a compelling indication that global food systems, and specifically those in developed countries, are at or have passed the point of over-complexification. These increasingly coerced regimes will continue to increase in vulnerability and cause ecological degradation until this trajectory is reversed. Too, our work offers support for maintaining informed skepticism regarding the benefits of new agricultural technologies, a skepticism that is sometimes disparage as being irresponsible and representing a form of anti-science (e.g., Specter, 2009). As such, future research that digs deeper into food system complexification—including developing ways to measure the phenomenon and identifying conditions of success for disruptive de-complexifications that continue to support global food security—is essential.
Data Availability Statement
The original contributions presented in the study are included in the article/Supplementary Materials, further inquiries can be directed to the corresponding author.
Author Contributions
PL conceived of the study. PS performed data analysis. PS and PL designed the figures and wrote the manuscript. Both authors contributed to the article and approved the submitted version.
Funding
This work was funded in part by the Arrell Food Institute at the University of Guelph.
Conflict of Interest
The authors declare that the research was conducted in the absence of any commercial or financial relationships that could be construed as a potential conflict of interest.
Publisher's Note
All claims expressed in this article are solely those of the authors and do not necessarily represent those of their affiliated organizations, or those of the publisher, the editors and the reviewers. Any product that may be evaluated in this article, or claim that may be made by its manufacturer, is not guaranteed or endorsed by the publisher.
Acknowledgments
Thanks to Alysa JK Loring and Evan Fraser for your suggestions.
Supplementary Material
The Supplementary Material for this article can be found online at: https://www.frontiersin.org/articles/10.3389/fsufs.2021.683100/full#supplementary-material
References
Aldemita, R. R., Reaño, I. M. E., Solis, R. O., and Hautea, R. A. (2015). Trends in global approvals of biotech crops (1992–2014). GM Crops Food 6, 150–166. doi: 10.1080/21645698.2015.1056972
Altieri, M. A. (1995). Agroecology: The Science of Sustainable Agriculture. Boulder, CO: Westview Press.
Angeler, D., Chaffin, B. C., Sundstrom, S. M., Garmestani, A., Pope, K. L., Uden, D. R., et al. (2020). Coerced Regimes: Management Challenges in the Anthropocene. 25. Available online at: https://pub.epsilon.slu.se/16709/
Ashby, W. R. (1947). Principles of the self-organizing dynamic system. J. Gen. Psychol. 37, 125–128. doi: 10.1080/00221309.1947.9918144
Bennett, E. M., Baird, J., Baulch, H., Chaplin-Kramer, R., Fraser, E. D. G., Loring, P. A., et al. (2021). “The Future of Agricultural Landscapes, Part II,” in Advances in Ecological Research, eds D. A. Bohan and A. J. Van Bergen (London: Academic Press), 1–43.
Bonhommeau, S., Dubroca, L., Pape, O. L., Barde, J., Kaplan, D. M., Chassot, E., et al. (2013). Eating up the world's food web and the human trophic level. Proc. Nat. Acad. Sci. U.S.A. 110, 20617–20620. doi: 10.1073/pnas.1305827110
Boserup, E. (1975). The impact of population growth on agricultural output. Q. J. Econ. 89, 257–270. doi: 10.2307/1884430
Brunori, G., Galli, F., Barjolle, D., Van Broekhuizen, R., Colombo, L., Giampietro, M., et al. (2016). Are local food chains more sustainable than global food chains? Considerations for assessment. Sustainability 8:449. doi: 10.3390/su8050449
Campbell, B. M., Beare, D. J., Bennett, E. M., Hall-Spencer, J. M., Ingram, J. S. I., Jaramillo, F., et al. (2017). Agriculture production as a major driver of the Earth system exceeding planetary boundaries. Ecol. Soc. 22, 1–11. doi: 10.5751/ES-09595-220408
Ceddia, M. G. (2019). The impact of income, land, and wealth inequality on agricultural expansion in Latin America. Proc. Nat. Acad. Sci. U.S.A. 116, 2527–2532. doi: 10.1073/pnas.1814894116
Chappell, M. J., and LaValle, L. A. (2011). Food security and biodiversity: can we have both? An agroecological analysis. Agric Human Values 28, 3–26. doi: 10.1007/s10460-009-9251-4
Corcoran, P. M. (2012). Cloud computing and consumer electronics: a perfect match or a hidden storm? [Soapbox]. IEEE Consumer Electron. Magazine 1, 14–19. doi: 10.1109/MCE.2011.2181895
Davis, K. F., Downs, S., and Gephart, J. A. (2021). Towards food supply chain resilience to environmental shocks. Nat. Food 2, 54–65. doi: 10.1038/s43016-020-00196-3
Dawson, T. P., Perryman, A. H., and Osborne, T. M. (2016). Modelling impacts of climate change on global food security. Clim. Change 134, 429–440. doi: 10.1007/s10584-014-1277-y
Ercsey-Ravasz, M., Toroczkai, Z., Lakner, Z., and Baranyi, J. (2012). Complexity of the international agro-food trade network and its impact on food safety. PLoS ONE 7:e37810. doi: 10.1371/annotation/5fe23e20-573f-48d7-b284-4fa0106b8c42
Evans, A. E., Mateo-Sagasta, J., Qadir, M., Boelee, E., and Ippolito, A. (2019). Agricultural water pollution: key knowledge gaps and research needs. Curr. Opin. Environ. Sustainabil. 36, 20–27. doi: 10.1016/j.cosust.2018.10.003
Flannery, K. V. (1972). The cultural evolution of civilizations. Annu. Rev. Ecol. Syst. 3, 399–426. doi: 10.1146/annurev.es.03.110172.002151
Food Agriculture Organization (2020). FAOStat. Food Agriculture Organization of the United Nations. Available online at: http://www.fao.org/faostat/en/
Fraser, E. D. G. (2011). Can economic, land use and climatic stresses lead to famine, disease, warfare and death? Using Europe's calamitous 14th century as a parable for the modern age. Ecol. Econ. 70, 1269–1279. doi: 10.1016/j.ecolecon.2010.02.010
Goldenberg, J., Horowitz, R., Levav, A., and Mazursky, D. (2003, March 1). Finding your innovation sweet spot. Harvard Business Review. Available online at: https://hbr.org/2003/03/finding-your-innovation-sweet-spot
Hagens, N. J. (2020). Economics for the future–beyond the superorganism. Ecol. Econ. 169:106520. doi: 10.1016/j.ecolecon.2019.106520
Hajer, M. A., Westhoek, H., Ingram, J., Van Berkum, S., and Özay, L. (2016). Food Systems and Natural Resources. Rome: United Nations Environmental Programme, 34.
Holt-Giménez, E., Shattuck, A., Altieri, M., Herren, H., and Gliessman, S. (2012). We already grow enough food for 10 billion people … and still can't end hunger. J. Sustain. Agric. 36, 595–598. doi: 10.1080/10440046.2012.695331
IPCC (2018). Global Warming of 1.5°C. Intergovernmental Panel on Climate Change. Available online at: http://www.ipcc.ch/report/sr,15/
IPES-Food (2020). The Added Value(S) of Agroecology: Unlocking the Potential for Transition in West Africa. International Panel of Experts on Sustainable Food Systems, 162. Available online at: http://www.ipes-food.org/pages/AgroecologyWestAfrica
ISAAA (2019). Global Status of Commercialized Biotech/GM Crops: 2019—ISAAA Brief 55-2019 | ISAAA.org. International Service for the Acquisition of Agri-biotech Applications. Available online at: https://www.isaaa.org/resources/publications/briefs/55/
Jick, T. D. (1979). Mixing qualitative and quantitative methods: triangulation in action. Administr. Sci. Q. 24, 602–611. doi: 10.2307/2392366
Kamilaris, A., Fonts, A., and Prenafeta-Bold?, F. X. (2019). The rise of blockchain technology in agriculture and food supply chains. Trends Food Sci. Technol. 91, 640–652. doi: 10.1016/j.tifs.2019.07.034
Kimbrell, A. (2002). The Fatal Harvest Reader: The Tragedy of Industrial Agriculture. Washington, DC: Island Press.
Kloppenburg, J., and Lezberg, S. (1996). Getting it straight before we eat ourselves to death: from food system to foodshed in the 21st century. Soc. Nat. Resour. 9, 93–96. doi: 10.1080/08941929609380954
Kristin, B., Kelly, S., Travis, H., Joseph, L., and Lori, C. (2017). Retail food environments, shopping experiences, first nations and the provincial norths. Health Promotion Chronic Dis. Prev. Canada Res. Policy Prac. 37, 333–341. doi: 10.24095/hpcdp.37.10.03
Laborde, D., Martin, W., Swinnen, J., and Vos, R. (2020). COVID-19 risks to global food security. Science 369, 500–502. doi: 10.1126/science.abc4765
Lin, X., Ruess, P. J., Marston, L., and Konar, M. (2019). Food flows between counties in the United States. Environ. Res. Lett. 14:084011. doi: 10.1088/1748-9326/ab29ae
Mehrabi, Z., and Ramankutty, N. (2019). Synchronized failure of global crop production. Nat. Ecol. Evolut. 3, 780–786. doi: 10.1038/s41559-019-0862-x
Miller, S. R., and Knudson, W. A. (2014). Nutrition and cost comparisons of select canned, frozen, and fresh fruits and vegetables. Am. J. Lifestyle Med. 8, 430–437. doi: 10.1177/1559827614522942
Mueller, N. G., and Flachs, A. (2021). Domestication, crop breeding, and genetic modification are fundamentally different processes: implications for seed sovereignty and agrobiodiversity. Agric. Human Values. doi: 10.1007/s10460-021-10265-3. [Epub ahead of print].
Paul, M. J., Nuccio, M. L., and Basu, S. S. (2018). Are GM crops for yield and resilience possible? Trends Plant Sci. 23, 10–16. doi: 10.1016/j.tplants.2017.09.007
Pearson, S., May, D., Leontidis, G., Swainson, M., Brewer, S., Bidaut, L., et al. (2019). Are distributed ledger technologies the panacea for food traceability? Global Food Security 20, 145–149. doi: 10.1016/j.gfs.2019.02.002
Pelletier, N., Audsley, E., Brodt, S., Garnett, T., Henriksson, P., Kendall, A., et al. (2011). Energy intensity of agriculture and food systems. Annu. Rev. Environ. Resources 36, 223–246. doi: 10.1146/annurev-environ-081710-161014
Porkka, M., Kummu, M., Siebert, S., and Varis, O. (2013). From food insufficiency towards trade dependency: a historical analysis of global food availability. PLoS ONE 8:e82714. doi: 10.1371/journal.pone.0082714
Reganold, J. P., and Wachter, J. M. (2016). Organic agriculture in the twenty-first century. Nat. Plants 2:15221. doi: 10.1038/nplants.2015.221
Rist, L., Felton, A., Nyström, M., Troell, M., Sponseller, R. A., Bengtsson, J., et al. (2014). Applying resilience thinking to production ecosystems. Ecosphere 5, 1–11. doi: 10.1890/ES13-00330.1
Rockström, J., Edenhofer, O., Gaertner, J., and DeClerck, F. (2020). Planet-proofing the global food system. Nat. Food 1, 3–5. doi: 10.1038/s43016-019-0010-4
Rótolo, G. C., Francis, C., Craviotto, R. M., Viglia, S., Pereyra, A., and Ulgiati, S. (2015). Time to re-think the GMO revolution in agriculture. Ecol. Informatics 26, 35–49. doi: 10.1016/j.ecoinf.2014.05.002
Rotz, S., Duncan, E., Small, M., Botschner, J., Dara, R., Mosby, I., et al. (2019). The politics of digital agricultural technologies: a preliminary review. Sociol. Ruralis 59, 203–229. doi: 10.1111/soru.12233
Rotz, S., and Fraser, E. D. G. (2015). Resilience and the industrial food system: analyzing the impacts of agricultural industrialization on food system vulnerability. J. Environ. Stud. Sci. 5, 459–473. doi: 10.1007/s13412-015-0277-1
Sassenrath, G. F., Heilman, P., Luschei, E., Bennett, G. L., Fitzgerald, G., Klesius, P., et al. (2008). Technology, complexity and change in agricultural production systems. Renew. Agric. Food Syst. 23, 285–295. doi: 10.1017/S174217050700213X
Schnell, S. M. (2013). Food miles, local eating, and community supported agriculture: putting local food in its place. Agric. Human Values 30, 615–628. doi: 10.1007/s10460-013-9436-8
Searchinger, T., Waite, R., Hanson, C., Ranganathan, J., Dumas, P., Matthews, E., et al. (2019). Creating a Sustainable Food Future: A Menu of Solutions to Feed Nearly 10 Billion People by 2050. Final Report. WRI. Washington, DC.
Sears, L., Caparelli, J., Lee, C., Pan, D., Strandberg, G., Vuu, L., et al. (2018). Jevons' paradox and efficient irrigation technology. Sustainability 10:1590. doi: 10.3390/su10051590
Sedlmeir, J., Buhl, H. U., Fridgen, G., and Keller, R. (2020). The energy consumption of blockchain technology: beyond myth. Business Information Syst. Eng. 599–608. doi: 10.1007/s12599-020-00656-x
Sen, A. (1983). Poverty and Famines: An Essay on Entitlement and Deprivation. Oxford: Oxford University Press. doi: 10.1093/0198284632.001.0001
Senauer, B., and Venturini, L. (2005). The Globalization of Food Systems: A Conceptual Framework and Empirical Patterns. Working Paper 05-01. Working Papers. Minneapolis, MN: University of Minnesota, The Food Industry Center. doi: 10.22004/ag.econ.14304
Serdarasan, S. (2013). A review of supply chain complexity drivers. Comput. Indus. Eng. 66, 533–540. doi: 10.1016/j.cie.2012.12.008
Specter, M. (2009). Denialism: How Irrational Thinking Hinders Scientific Progress, Harms the Planet, and Threatens Our Lives. New York, NY: Penguin Press HC.
Stevens, T., Aarts, N., Termeer, C., and Dewulf, A. (2016). Social media as a new playing field for the governance of agro-food sustainability. Curr. Opin. Environ. Sustain. 18, 99–106. doi: 10.1016/j.cosust.2015.11.010
Stoll, J. S., Harrison, H. L., Sousa, E. D., Callaway, D., Collier, M., Harrell, K., et al. (2021). Alternative seafood networks during COVID-19: Implications for resilience and sustainability. Front. Sustainable Food Syst. 5:614368. doi: 10.3389/fsufs.2021.614368
Strumsky, D., Lobo, J., and Tainter, J. A. (2010). Complexity and the productivity of innovation. Syst. Res. Behav. Sci. 27, 496–509. doi: 10.1002/sres.1057
Sundkvist, A., Milestad, R., and Jansson, A. (2005). On the importance of tightening feedback loops for sustainable development of food systems. Food Policy 30, 224–239. doi: 10.1016/j.foodpol.2005.02.003
Tainter, J. A. (1995). Sustainability of complex societies. Futures 27, 397–407. doi: 10.1016/0016-3287(95)00016-P
Tainter, J. A. (2006). Social complexity and sustainability. Ecol. Complexity 3, 91–103. doi: 10.1016/j.ecocom.2005.07.004
Tamburino, L., Bravo, G., Clough, Y., and Nicholas, K. A. (2020). From population to production: 50 years of scientific literature on how to feed the world. Global Food Security 24:100346. doi: 10.1016/j.gfs.2019.100346
Thilmany, D., Canales, E., Low, S. A., and Boys, K. (2021). Local food supply chain dynamics and resilience during COVID-19. Appl. Econ. Perspect. Policy 43, 86–104. doi: 10.1002/aepp.13121
Tittonell, P., Piñeiro, G., Garibaldi, L. A., Dogliotti, S., Olff, H., and Jobbagy, E. G. (2020). Agroecology in large scale farming—a research agenda. Front. Sustain. Food Syst. 4:214. doi: 10.3389/fsufs.2020.584605
Trivette, S. A. (2012). Close to home: the drive for local food. J. Agric. Food Syst. Commun. Dev. 3, 161–180. doi: 10.5304/jafscd.2012.031.008
Troell, M., Naylor, R. L., Metian, M., Beveridge, M., Tyedmers, P. H., Folke, C., et al. (2014). Does aquaculture add resilience to the global food system? Proc. Natl. Acad. Sci. U.S.A. 13257–13263. doi: 10.1073/pnas.1404067111
United Nations Conference on Trade Development. (1998). World Investment Report 1998: Trends and Determinants. New York, NY: United Nations, 428. Available online at: https://unctad.org/system/files/official-document/wir1998_en.pdf
Weersink, A., Fraser, E., Pannell, D., Duncan, E., and Rotz, S. (2018). Opportunities and challenges for big data in agricultural and environmental analysis. Annu. Rev. Resource Econ. 10, 19–37. doi: 10.1146/annurev-resource-100516-053654
Witter, A., and Stoll, J. (2017). Participation and resistance: alternative seafood marketing in a neoliberalera. Marine Policy 80, 130–140. doi: 10.1016/j.marpol.2016.09.023
Keywords: agroecology, complexity, coerced regimes, degrowth, food system resilience, innovation, development, food system sustainability
Citation: Loring PA and Sanyal P (2021) Indicators of Complexity and Over-Complexification in Global Food Systems. Front. Sustain. Food Syst. 5:683100. doi: 10.3389/fsufs.2021.683100
Received: 19 March 2021; Accepted: 13 October 2021;
Published: 12 November 2021.
Edited by:
Mariana Benítez, National Autonomous University of Mexico, MexicoReviewed by:
Philippe V. Baret, Catholic University of Louvain, BelgiumMaria-Jose Ibarrola-Rivas, National Autonomous University of Mexico, Mexico
Copyright © 2021 Loring and Sanyal. This is an open-access article distributed under the terms of the Creative Commons Attribution License (CC BY). The use, distribution or reproduction in other forums is permitted, provided the original author(s) and the copyright owner(s) are credited and that the original publication in this journal is cited, in accordance with accepted academic practice. No use, distribution or reproduction is permitted which does not comply with these terms.
*Correspondence: Philip A. Loring, phil.loring@uoguelph.ca