Perennial grassland agriculture restores critical ecosystem functions in the U.S. Upper Midwest
- 1Department of Agronomy, University of Wisconsin-Madison, Madison, WI, United States
- 2Social Science Department, University of Wisconsin-Stout, Menomonie, WI, United States
- 3Department of Entomology, University of Wisconsin-Madison, Madison, WI, United States
Dominant forms of agricultural production in the U.S. Upper Midwest are undermining human health and well being. Restoring critical ecosystem functions to agriculture is key to stabilizing climate, reducing flooding, cleaning water, and enhancing biodiversity. We used simulation models to compare ecosystem functions (food-energy production, nutrient retention, and water infiltration) provided by vegetation associated with continuous corn, corn-soybean rotation, and perennial grassland producing feed for dairy livestock. Compared to continuous corn, most ecosystem functions dramatically improved in the perennial grassland system (nitrate leaching reduced ~90%, phosphorus loss reduced ~88%, drainage increased ~25%, evapotranspiration reduced ~29%), which will translate to improved ecosystem services. Our results emphasize the need to incentivize multiple ecosystem services when managing agricultural landscapes.
Introduction
Agriculture is central to the fundamental challenges facing human society (Godfray et al., 2010; Foley et al., 2011; Wheeler and von Braun, 2013; Amundson et al., 2015; Kremen and Merenlender, 2018). We must develop and grow agricultural systems that provide for our well being while building the capacity of future generations to do the same. These agricultural systems must be resilient in the face of drought, flooding, and extreme weather, as well as socio-economic shocks such as pandemics and market failures (Lesk et al., 2016; Lioutas and Charatsari, 2021; Ortiz-Bobea, 2021).
Critical ecosystem functions of agricultural systems include plant and animal productivity, soil carbon storage (Rowntree et al., 2020; Guillaume et al., 2022; Rui et al., 2022), soil stabilization (Montgomery, 2007; Palm et al., 2014; Schulte et al., 2017), nutrient retention (Schulte et al., 2017; Hussain et al., 2019; Jackson, 2020), water infiltration and storage (Basche and DeLonge, 2019; Baker et al., 2022), and wildlife habitat (Kimoto et al., 2012; Tsiafouli et al., 2015; Schulte et al., 2017). While these factors range in their scale of influence (e.g., soil carbon storage influences greenhouse gas concentrations globally while habitat for soil arthropods is quite local), each of the functions have practical relevance for those living in the U.S. Upper Midwest where surface and groundwater pollution, flooding, soil erosion, and plummeting biodiversity undermine human welfare and well being (Werling et al., 2014; Hussain et al., 2019; Antolini et al., 2020; Bendorf et al., 2021; Borchardt et al., 2021; Burch et al., 2021; Raff and Meyer, 2022; Wisconsin Groundwater Coordinating Council Report to the Legislature, 2022).
Solutions to this multifaceted dilemma require holistic approaches that consider land management effects on ecosystem functions that underpin ecosystem services provided by farms and, more broadly, the landscapes or regions in which they are nested (Strauser et al., 2022). Holistic solutions are required because of the complexity and connectedness of these landscapes, where focusing on a single dimension typically exacerbates problems in others. We must understand and develop systems that solve for multiple variables simultaneously. While the currently dominant form of agriculture is immensely productive, it is also the world's leading driver of environmental change (Foley et al., 2005, 2011). Fortunately, agricultural approaches exist that have the potential to help stabilize global change as we move further into the Anthropocene (Campbell et al., 2017; Rockström et al., 2017).
The dominant agricultural system of the U.S. Upper Midwest is based on monocultures of corn and soybeans grown to feed mostly confined livestock. This system is incentivized by rewarding farmers almost exclusively for more production (Jordan et al., 2018), which comes at the expense of other functions critical to ecological and societal well being [e.g., purification of water, stabilization of soil, regulation of infectious disease, provisioning of wildlife habitat; Alexander et al. (2008), Wepking et al. (2017), Christianson et al. (2018)]. Currently, critical ecosystem functions and the services that they underpin are not properly valued, so their costs are externalized; borne by society as a whole (Suparak Gibson, 2022). An alternative agricultural system, based on perennial grassland, is possible (Jackson, 2022) but requires society to pivot away from the status quo toward a system that rewards a range of ecosystem services. Currently, farmers' individual decisions to participate in the corn and soybean dominated agricultural system are driven by constructed narratives around productivism and maximizing food production (Burton, 2004; McGuire et al., 2013), aesthetic preferences about the 'neatness' and perceived care of the landscape (Nassauer, 1988), and definitions of place at regional scales (Strauser et al., 2022). To incentivize agricultural systems that simultaneously provide multiple ecosystem services to farmers and society, we must understand tradeoffs and synergies in ecosystem functions provided by alternative cropping systems.
To further this understanding we used Agro-IBIS [Integrated Biosphere Simulator; Kucharik et al. (2000), Kucharik (2003), Kucharik and Brye (2003)] to represent a variety of biophysical and biogeochemical processes. These processes included nitrate leaching and phosphorus loss as indicators of nutrient retention and drainage and evapotranspiration as indicators of water retention. These indicators were then simulated across vegetation types associated with three dairy cropping systems (see Methods). In addition to controlling the type of vegetation grown in the model, different simulated land-use decisions can be made regarding fertilizer and manure applications and crop rotations.
Recent work with Agro-IBIS has focused on meeting targeted policy goals with increasing grassland cover. In particular, Campbell et al. (2022) estimated the amount of perennial grassland cover needed to meet water quality goals within the Yahara River Watershed in southern Wisconsin to the year 2070. Similarly, water quality outcomes were assessed with simulations designed to achieve the goals of the Renewable Fuel Standard providing insight into the beneficial water quality effects of improved miscanthus and switchgrass cover (Ferin et al., 2021). Other models, such as DairyMod, APSIM, and DayCent have been used to simulate soil N mineralization and pasture growth (Bilotto et al., 2021), and DairyMod in particular has been instrumental in simulating ammonia volatilization in pastures (Smith et al., 2020), but with a specific focus on Australia and New Zealand where DairyMod was calibrated (Johnson et al., 2008).
Within this stream of the literature, there is no work addressing regional variation in a broad suite of ecosystem services across the U.S. Upper Midwest. In particular, we contribute to the literature by including water quantity in addition to water quality, and matching regional variation within these environmental outcomes to food-energy production outcomes. By simulating these ecosystem functions across three common land cover-land use scenarios (described below), we are better able to anticipate how a wider range of ecosystem services might vary with management.
We examined ecosystem functions under three types of land cover associated with cropping systems typical of the U.S. Upper Midwest. We gathered site-specific data (previous cropping practices, soil type, slope, aspect) from five Wisconsin farms—two in the “Ridge & Valley” region of southwest Wisconsin (Vernon County) and three in the “Cloverbelt” region of central Wisconsin (Marathon County). While both of these regions have a strong agricultural focus, they vary in their topography as well as their edaphic and environmental characteristics. We used simulation models and literature estimates to predict outcomes of ecosystem functions under three land cover-land use scenarios—continuous corn, corn-soy rotation, and grassland. Ecosystem functions included estimates of food-energy production as well as water and nutrient dynamics. We expected that increasing perennial cover would improve a range of ecosystem services with potential tradeoffs in food-energy (meat and dairy) production.
Methods
Study region
The Cloverbelt and Ridge & Valley regions of Wisconsin are both known for dairy, beef, and crop production and each region has a strong identity and ethos associated with agriculture and the environment (Supplementary Figure S1, Supplementary Tables S2–S4). In each region annual grain crops (mainly corn and soybeans) are grown on most agricultural land to feed confined livestock whose genetic improvements and concentration in space continue to increase production and efficiency when the latter is assessed as calories produced per input.
While similar in many ways, these regions are quite different. The Cloverbelt is relatively flat with moderate- to poorly-drained soils where local climate and edaphic conditions are favorable to clover production in pastures, giving the agriculture of the region a distinctive Dairyland signature. The Ridge & Valley region is characterized by silty, erodible soils on highly dissected topography that make the region flood prone. Annual average precipitation and temperature for the last 10 years (2010 through 2020) were 107.2 cm and 7.1°C for Vernon County (Ridge & Valley) and 93.7 cm and 6.4°C for Marathon County [Cloverbelt; PRISM Climate Group, Oregon State University (2022)]. This variation between farms and regions provides a representation of a significant part of farming in the U.S. Upper Midwest (see Tables S2–S4 for additional details).
Data collection
We gathered crop histories and soil tests from reports submitted by the operators of each of the five farms (Table 1). To protect the privacy of these farm operators, we retain the confidentiality of each farm and report only overall summaries of each. Data included farm size, individual field delineation, soil types, soil phosphorus, slope, slope length, and previous land use.
The physical characteristics of these farms are highly variable (Table 1) and they currently use a mix of row-crop rotations, tillage and no till, and managed grazing. Importantly, especially for water quality, soil P levels varied considerably among farms. Also, the slope and slope length of each farm likely affect water quality in different ways. Consistent with regional descriptions above, Cloverbelt farms were larger, flatter, and participated primarily in more row-crop agriculture for dairy production while Ridge & Valley farms were smaller, on steeper slopes, and used more pasture. We reported land in agricultural production only, not including some forested land, which for one farm was steep. Therefore, while the slope was relatively shallow for Farm 2 the adjacent forested land was steep and listed as highly flood prone.
Simulation models
Agro-IBIS is a spatially explicit agroecosystem and land surface model that simulates the movement of water, energy, momentum, carbon, nitrogen, and phosphorus, in both natural and managed ecosystems. The structure of Agro-IBIS has been described in detail (Kucharik et al., 2000; Kucharik, 2003; Kucharik and Brye, 2003; Motew et al., 2017) and many components and output variables of the model (e.g., crop yield, net primary productivity (NPP), net ecosystem exchange (NEE), evapotranspiration and drainage, nitrate leaching, soil temperature and moisture) have been validated across a range of ecosystems at various spatial and temporal scales (Kucharik et al., 2000, 2006; Kucharik, 2003; Kucharik and Brye, 2003; Kucharik and Twine, 2007; Motew and Kucharik, 2013; Soylu et al., 2014; Zipper et al., 2015; Motew et al., 2017). Agro-IBIS was integrated with the variably saturated soil water flow model HYDRUS-1D to enable simulation of groundwater-vegetation interactions (Soylu et al., 2014), and P cycling and dynamics were recently added based on SurPhos, a state-of-the-art dissolved P loss model for agricultural systems receiving manure (Vadas et al., 2004, 2005, 2007; Motew et al., 2017). The P module features P application, transformation, and loss of dissolved P to runoff; in-soil cycling of organic and inorganic forms of P; and loss of particulate-bound P with erosion (Motew et al., 2017).
Before running the scenarios of land cover-land use, a long-term model spin-up run was executed from 1650 to 1961 to achieve a steady-state equilibrium in soil biogeochemical cycling that reflects changes in land use and build-up of soil organic C and N pools (Donner and Kucharik, 2003). Agro-IBIS model simulations were executed using a 60-min time-step on a 1 x 1-km regularly spaced grid; the model uses SSURGO soil textural data to delineate dominant soil texture and soil physical properties for each grid cell and soil layer, and daily weather data (air temperature, precipitation, relative humidity, solar radiation, and wind speed) from the gridMET (gridMET, 2013) that was interpolated from 4- to 1-km spatial resolution. Agro-IBIS uses statistical models to interpolate daily weather variables to the hourly time-step (Kucharik et al., 2000). During the model simulation period from 1650 through 1978, a random draw of weather years was taken from the actual data time-series of 1979 through 2016; simulation years from 1979 through 2016 represent the actual weather time-series from gridMET. Nutrient inputs (inorganic fertilizer and manure) originate from a spatiotemporal database of linked agricultural, environmental, and economic data (Lark et al., 2022).
We simulated three different agricultural scenarios or vegetation types: continuous corn, corn-soybean rotation, and generalized C4-dominant perennial grassland for five locations described in Table 1. Continuous corn and corn-years in the corn-soybean rotation received between 91.8 and 180 kg N ha−1 yr−1 and 9 and 22 kg P ha−1 yr−1 based on historical fertilization for that location, which varied by year; soybean and grass did not receive any N and P fertilizer because neither receive N and P fertilization as part of typical grass or soy production. Soil was tilled in continuous corn and corn-soybean rotation was tilled before planting while the grass was never tilled.
For the corn-soy rotation, we ran two scenarios starting with both corn and soybean and then aggregated the output. Annual estimates of nitrate leaching, phosphorus loss (including both sediment and dissolved phosphorus), evapotranspiration, and groundwater recharge (drainage) were gathered after running those different vegetation scenarios from 1961 through 2016. We then filtered out the first 18 years of data (keeping the 38 years from 1979 through 2016), as the model output took approximately 10 years after a restart simulation (the restart year was 1961 for each scenario) to reach equilibrium, and because the time-series of actual weather begins in 1979.
After gathering the output data, we used linear mixed effects models (one for each of the above dependent variables) using the nlme package in R (Pinheiro et al., 2022) to limit the effects of vegetation type and year (fixed effects) and farm nested within region (random effects) to account for site/geographical variation.
Food-energy calculations
We examined another important ecosystem function, agricultural production, for each of the three scenarios by calculating their food-energy output from harvested biomass. We used the historical cropping data for each farm to generate average farm-level output (bushels ha−1). We then used this past output and created a “target” output to use in the counterfactual simulations. As a specific example, the average farm-level production at Farm 3 during the pre-simulation period was 354.1 bushels ha−1 yr−1 (9.6 Mg ha−1 yr−1) of corn grain and 49.8 bushels ha−1 yr−1 (3.3 Mg ha−1 yr−1) of soybeans. We therefore simulated food-energy production for Farm 3 using these values as output, while averaging the output over two years for the corn-soy rotation. We did not have farm-specific yield data for farms that did not practice a cropping system during the years for which we obtained observational data, so for these farms we used USDA Census of Agriculture average yield maps for that county and set the target yield at the value given in the map (USDA—National Agricultural Statistics Service, 2022). For pastures without yield data, we assumed an average height of the grass of 63.5 cm and multiplied that by an estimated harvest of 326 kg DM ha−1 (Barnhart, 1998), which is equivalent to 8.4 Mg ha−1 yr−1 of harvested dry matter. We then converted these target yields for each farm to food-energy using the process described in Sanford et al. (2021). Like Sanford et al. (2021), our representative farms and cropping systems represent agricultural production in the U.S. Upper Midwest (WI), which is a major producer of dairy products. We therefore examined food-energy in the form of milk and dairy beef (Gcal ha−1 yr−1). Briefly, Sanford et al. (2021) make their conversion from harvested yield to food-energy using the following steps:
1. Convert volume yield (bushels ac−1) to mass yield (Mg ha−1), while assuming that 79.2% of soybean grain results in soybean meal and 18.7% results in soybean oil. We used national data from 1980 to 2016 to estimate these percentages (USDA-ERS, 2018).
2. Convert dry matter yield to total digestible nutrients (TDN) using mean nutrient content values from Dairy One Cooperative (Feed Composition Library | Dairy One, 2022): 88% for corn, 80% for soybeans, and 60% for grass.
3. Convert TDN yield to milk and dairy beef food-energy using conversions from Peters et al. (2014) and USDA (USDA—FoodData Central, 2022). We used the same process as Sanford et al. (2021) to calculate a conversion factor of 1.04 Gcal Mg−1 for milk (1,042 kcal kg−1 TDN) and 0.37 Gcal Mg−1 for dairy beef (366 kcal kg−1 TDN).
Finally, we compared observable farm factors and ecosystem functions by assessing correlations among these variates.
Results
Across the five farms and two regions, vegetation type significantly affected nitrate leaching (Figure 1A, Supplementary Table S1, Supplementary Figure S2). This effect was driven by the low average level of nitrate leaching over the 38 years analyzed in the grass system (4.1 ± 0.2 kg ha−1) compared to continuous corn (39.9 ± 1.0 kg ha−1) and the corn-soy rotation (33.5 ± 0.8 kg ha−1). Both continuous corn and the corn-soy rotation leached significantly more than the grass system in a pairwise comparison (P < 0.001 and P < 0.001, respectively), but continuous corn also leached significantly more than corn-soy (P < 0.001). Across the three vegetation types nitrate leaching generally increased over time, on average increasing 0.4 kg ha−1 yr−1 [P < 0.001 (Supplementary Figure S2)]. Annual variation in nitrate leaching was driven by precipitation and management differences (Supplementary Figure S3). When considered across the five farms and two regions separately within the grass vegetation, nitrate leaching appeared to vary by region with Marathon County (6.0 ± 0.1 kg ha−1) exhibiting greater leaching than Vernon County [1.3 ± 0.04 kg ha−1 (Supplementary Figure S4)].
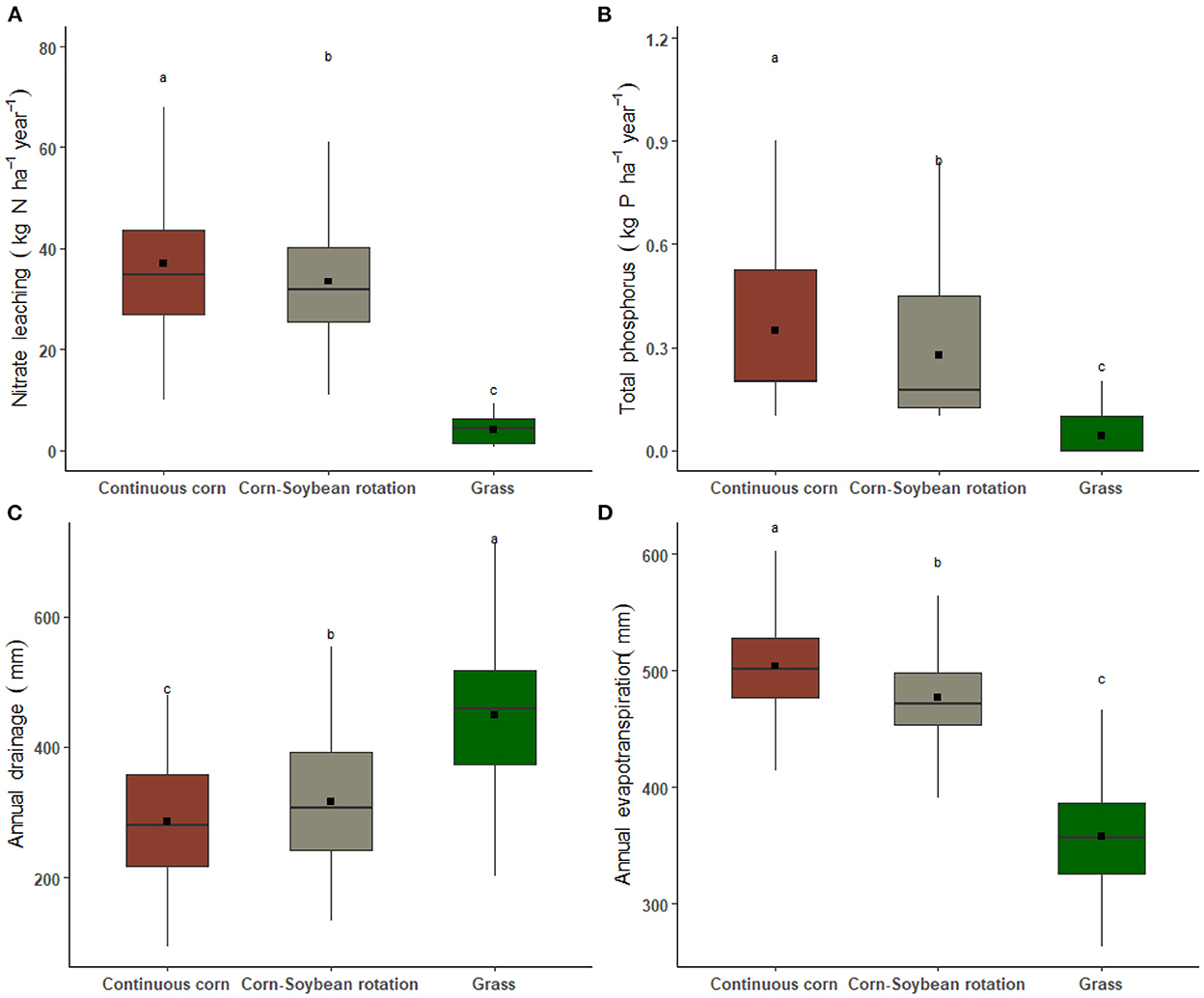
Figure 1. (A–D) Annual averages across the modeled 38 years for (A) nitrate leaching, (B) phosphorus loss, (C) drainage, and (D) evapotranspiration across the three vegetation types on five combined farms in Marathon County, WI, USA (Cloverbelt), and Vernon County, WI, USA (Ridge & Valley). Box plots denote range of values (vertical lines), the mean (solid point), median (horizontal line), and 25th and 75th percentile range. Groups that do not share the same letters are significantly different at the 0.05 level.
Continuous corn was found to have the highest level of phosphorus loss (0.35 ± 0.02 kg ha−1) followed by the corn-soy rotation (0.28 ± 0.02 kg ha−1); grass had the lowest level of phosphorus loss (0.042 ± 0.004 kg ha−1) across the five farms and two counties investigated (Figure 1B, Supplementary Table S1). Phosphorus runoff with continuous corn vegetation was found to be significantly greater than both the corn-soy rotation (P < 0.001) and the grass vegetation types (P < 0.001). The grass vegetation type was found to exhibit significantly lower phosphorus than the corn-soy rotation vegetation type (P < 0.001). Phosphorus tended to decrease over time as well [P < 0.001 (Supplementary Figure S2)]. However, this decline over time appears to be driven by the row-crop vegetation types; the grass vegetation type held relatively steady over the course of the 38 years analyzed (Supplementary Figure S2).
The amount of annual drainage, or recharge to groundwater, was shown to vary by vegetation type (Figure 1C, Supplementary Table S1). The grass system showed the highest annual drainage (449 ± 7.4 mm yr−1), significantly greater than both the continuous corn (286 ± 6.3 mm yr−1; P < 0.001), and corn-soy rotation (317 ± 6.4 mm yr−1; P < 0.001). The corn-soy rotation was shown to have significantly greater drainage than the continuous corn vegetation (P < 0.001). Over the course of the 38 years analyzed, annual drainage shows a general decrease over time, on average 2.23-mm lower annually (P < 0.001). In addition, grass vegetation was shown to have a stronger positive relationship than the other two vegetation types between annual drainage and annual precipitation (Figure 2).
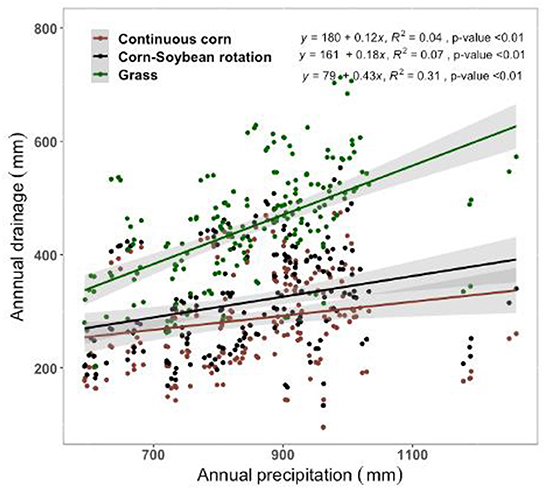
Figure 2. Multiple linear regression between annual drainage and annual precipitation across the three vegetation types investigated. The grass system showed a stronger positive relationship between annual drainage and precipitation.
Evapotranspiration trends were opposite of drainage (Figure 1D, Supplementary Table S1). Grass systems had the lowest evapotranspiration (358 ± 3.1 mm yr−1; P < 0.001), corn-soy systems had the second-highest evapotranspiration (477 ± 2.5 mm yr−1; P < 0.001), and continuous corn had the highest (504 ± 2.7 mm yr−1; P < 0.001). Generally, evapotranspiration was higher in the Ridge & Valley than the Cloverbelt (Supplementary Figure S5). In addition, across all vegetation types, the annual average ET trend was 0.91 mm yr−1 (P < 0.001).
Finally, the vegetation types differed in their levels of food-energy production. Across all cropping systems, the same level of harvested dry matter produced higher amounts of food-energy in the form of milk compared to dairy beef (Table 2). Continuous corn had the highest food-energy output, producing over 3 Gcal ha−1 yr −1 more milk energy and over 1 Gcal ha−1 yr −1 more beef energy (~60% for each) than the corn-soy rotation and grass (P < 0.001). However, no significant difference in food-energy production was observed between corn-soy rotation and grass for both milk (P = 0.70) and dairy beef (P = 0.71) output.
Discussion
Nitrate leaching was greatly reduced under grass vegetation compared to both continuous corn and corn-soy rotation because no manure or fertilizer was added to grass vegetation, which aligns with empirical field studies. Under most perennial grass bioenergy and grazed systems, nitrate leaching is much lower than corn-based cropping systems (Hussain et al., 2019; Jackson, 2020), differences that can lead to significant disparities in water quality for rural regions, where nitrate leaching contributes to impaired health and infant mortality (Knobeloch et al., 2013). Further, nitrate leaching from common corn-based systems contributes to eutrophication and consequently impaired rivers, lakes, and oceans (Orth et al., 2006; Liu et al., 2022). These waterways arguably are more impaired from phosphorus runoff, with nitrogen potentially working in concert with phosphorus to induce further eutrophication (Dodds and Smith, 2016; Schindler et al., 2016). As mentioned in the introduction, there is promise for reducing eutrophication and meeting established water quality goals by reducing nutrients in watersheds and increasing perennial cover as shown by Campbell et al. (2022).
From a water quantity perspective, grassland promoted higher water drainage (i.e., more infiltration and less runoff) and lower evapotranspiration than the other systems. Increasing evapotranspiration reduces local temperatures through increasing latent heat flux, and has been shown to mitigate increased temperatures, e.g., the urban heat island effect (Qiu et al., 2013). In an agricultural context, however, higher evapotranspiration (e.g., continuous corn, corn-soy rotation) is linked to higher water demand and consequently higher irrigation rates or water demand for crops. Systems that promote higher evapotranspiration and lower recharge have a direct impact on the volume of surface water bodies. In the case of irrigation, this has been shown to deplete groundwater levels, especially as climate change increases evapotranspiration rates over time (Condon et al., 2020). While only 1.5 and 0.4% of agricultural land in Marathon County and Vernon County are irrigated, this is an important consideration in drier areas. While we found lower evapotranspiration rates in grasses than continuous corn or the corn-soy rotation, some grasslands can be comparable to corn-based systems (Abraha et al., 2020). Agricultural impacts on groundwater depend in large part on irrigation (which was zero in our modeled grassland) and drainage (which was highest in our modeled grassland) back into groundwater systems. While beyond the scope of our modeling study, many studies have also shown the potential of perennial grassland to reduce runoff and flood risk downstream because of its ability to enhance infiltration (Jackson and Keeney, 2010; Schilling et al., 2014).
Balancing our current emphasis on agricultural production with other ecosystem services is critical. The continuous corn system produced more food-energy than the corn-soy and grass systems, which is consistent with previous work (Peters et al., 2014). However, while the grass and corn-soy systems produced similar output from a food-energy perspective, the grass system outperformed the corn-soy rotation on all other ecosystem metrics. While recent research has shown that the current amount of beef raised within the U.S. could be raised entirely on grass—and without adding acreage not already in some form of agricultural production (Jackson, 2022)—more work is needed to better understand the ramifications of transformative changes to our agricultural landscape. Spatially explicit research that can show where various forms of agriculture can either do the least damage, or conversely, can promote the most beneficial ecosystem services, is a clear need in improving our understanding and decision making around agricultural production.
From a dairy perspective, milk yields dropped when cows were fed from grassland exclusively (Jackson, 2022). However, this drop in milk production with the grass-based system can be countered by a dramatic drop in production costs (Dartt et al., 1999; Kriegl, 2005; Hanson et al., 2013) making grass-based dairies economically competitive with confinement dairies; work that shows that grass-based systems can outperform others from a multifunctionality perspective. Other work shows that from a true-cost accounting perspective, grass-based farms provide much more value to society than what are considered conventional farms, and are dramatically undervalued (Suparak Gibson, 2022). Instances such as this require a framework to reward farmers for the societal good produced, whether it be from a policy perspective or some other structure (Rissman et al., in this volume).
While we focused on agricultural production from an energetics perspective to broadly compare the vegetation types in question, there is more to food than energy. Nutritional profiles are an important consideration to include in future analysis of tradeoffs among ecosystem services. Research on this topic shows that grassfed livestock production improves both animal health and the nutritional profile of livestock products compared to conventionally raised livestock (van Vliet et al., 2021b). A key driver of this improvement in nutritional profile was the biodiversity of the plants consumed by grassfed livestock, suggesting that the promotion of biodiversity is strongly linked with human health (Provenza et al., 2021; van Vliet et al., 2021a).
Our model did not include a grazing module that mimicked disturbance-plant growth dynamics, nutrient uptake and NPP, which would likely be stimulated to an even greater degree under well-managed grazing resulting in improvement in most ecosystem functions. Current work is adding grazing and cover crop modules to further explore continuous living cover in agroecosystems. These types of modeling advances, integrated with the other capabilities of Agro-IBIS, will allow scientists to develop advanced decision support tools (DSTs) that contain model output data from many scenarios representing the potential impacts of a changing climate and land management on ecosystem services. The goal is to have crop consultants, land managers, farmers and other end users use DSTs to guide future agroecosystem management decision-making to meet sustainable development goals for humanity.
Conclusions
With the exception of food-energy yield, all the ecosystem functions we explored were improved under the grassland-based system compared to annual grain crops. If agricultural policy continues to reward yield exclusively, it will be difficult to transition to more multifunctional agricultural systems. Continuous corn yielded more food-energy than corn-soy rotation and perennial grassland, but a significant tradeoff was observed: this system had the poorest performance across all other ecosystem functions – nitrate leaching, phosphorus loss, drainage, and evapotranspiration. While the corn-soy rotation provided slightly better outcomes than continuous corn (except for yield), it was inferior to perennial grassland for most outcomes and not significantly different in food calorie yield. A more balanced delivery of ecosystem functions underpinning critical ecosystem services will require more reliance on perennial grassland for livestock production.
Data availability statement
The raw data supporting the conclusions of this article will be made available by the authors, without undue reservation.
Author contributions
CW, DS, HM, AO, ZR, EB, CK, CG, and RJ designed the research. DS, HM, AO, and ZR preformed the research and analyzed the data. CW wrote the manuscript. CW, DS, HM, AO, ZR, EB, CG, and RJ edited the manuscript. All authors contributed to the article and approved the submitted version.
Funding
This work is supported by the Sustainable Agriculture Systems Coordinated Agricultural Program Grant No. 2019-68012-29852 from the USDA National Institute of Food and Agriculture. CK was supported by the National Science Foundation's Innovations at the Nexus of Food-Energy-Water Systems (INFEWS) program, award #1855996. DS was supported by a grant from the Wisconsin Department of Natural Resources (DNR).
Conflict of interest
The authors declare that the research was conducted in the absence of any commercial or financial relationships that could be construed as a potential conflict of interest.
Publisher's note
All claims expressed in this article are solely those of the authors and do not necessarily represent those of their affiliated organizations, or those of the publisher, the editors and the reviewers. Any product that may be evaluated in this article, or claim that may be made by its manufacturer, is not guaranteed or endorsed by the publisher.
Supplementary material
The Supplementary Material for this article can be found online at: https://www.frontiersin.org/articles/10.3389/fsufs.2022.1010280/full#supplementary-material
References
Abraha, M., Chen, J., Hamilton, S. K., and Robertson, G. P. (2020). Long term evapotranspiration rates for rainfed corn versus perennial bioenergy crops in a mesic landscape. Hydrol. Process. 34, 810–822. doi: 10.1002/hyp.13630
Alexander, R. B., Smith, R. A., Schwarz, G. E., Boyer, E. W., Nolan, J. V., and Brakebill, J. W. (2008). Differences in phosphorus and nitrogen delivery to The Gulf of Mexico from the Mississippi River Basin. Environ. Sci. Technol. 42, 822–830. doi: 10.1021/es0716103
Amundson, R., Berhe, A. A., Hopmans, J. W., Olson, C., Sztein, A. E., and Sparks, D. L. (2015). Soil and human security in the twenty-firstt century. Science 348, 1261071. doi: 10.1126/science.1261071
Antolini, F., Tate, E., Dalzell, B., Young, N., Johnson, K., and Hawthorne, P. L. (2020). Flood risk reduction from agricultural best management practices. JAWRA J. Am. Water Resour. Assoc. 56, 161–179. doi: 10.1111/1752-1688.12812
Baker, J. M., Albrecht, K. A., Feyereisen, G. W., and Gamble, J. D. (2022). A perennial living mulch substantially increases infiltration in row crop systems. J. Soil Water Conserv. 77, 212–220. doi: 10.2489/jswc.2022.00080
Barnhart, S. K. (1998). Estimating Available Pasture Forage. Available online at: http://www.extension.iastate.edu/Publications/PM1758.pdf.
Basche, A. D., and DeLonge, M. S. (2019). Comparing infiltration rates in soils managed with conventional and alternative farming methods: a meta-analysis. PLoS ONE 14, e0215702. doi: 10.1371/journal.pone.0215702
Bendorf, J., Hubbard, S., Kucharik, C. J., and VanLoocke, A. (2021). Rapid changes in agricultural land use and hydrology in the driftless region. Agrosyst. Geosci. Environ. 4, e20214. doi: 10.1002/agg2.20214
Bilotto, F., Harrison, M. T., Migliorati, M. D. A., Christie, K. M., Rowlings, D. W., Grace, P. R., et al. (2021). Can seasonal soil N mineralisation trends be leveraged to enhance pasture growth? Sci. Total Environ. 772, 145031. doi: 10.1016/j.scitotenv.2021.145031
Borchardt, M. A., Stokdyk, J. P., Kieke, B. A., Muldoon, M. A., Spencer, S. K., Firnstahl, A. D., et al. (2021). Sources and risk factors for nitrate and microbial contamination of private household wells in the fractured dolomite aquifer of Northeastern Wisconsin. Environ. Health Perspect. 129, 067004. doi: 10.1289/EHP7813
Burch, T. R., Stokdyk, J. P., Spencer, S. K., Kieke, B. A., Firnstahl, A. D., Muldoon, M. A., et al. (2021). Quantitative microbial risk assessment for contaminated private wells in the fractured dolomite aquifer of Kewaunee County, Wisconsin. Environ. Health Perspect. 129, 067003. doi: 10.1289/EHP7815
Burton, R. J. F. (2004). Seeing through the “good farmer's” eyes: towards developing an understanding of the social symbolic value of “productivist” behaviour. Sociol. Rural. 44, 195–215. doi: 10.1111/j.1467-9523.2004.00270.x
Campbell, B. M., Beare, D. J., Bennett, E. M., Hall-Spencer, J. M., Ingram, J. S. I., Jaramillo, F., et al. (2017). Agriculture production as a major driver of the Earth system exceeding planetary boundaries. Ecol. Soc. 22, art8. doi: 10.5751/ES-09595-220408
Campbell, T. A., Booth, E. G., Gratton, C., Jackson, R. D., and Kucharik, C. J. (2022). Agricultural landscape transformation needed to meet water quality goals in the Yahara River watershed of Southern Wisconsin. Ecosystems 25, 507–525. doi: 10.1007/s10021-021-00668-y
Christianson, R., Christianson, L., Wong, C., Helmers, M., McIsaac, G., Mulla, D., et al. (2018). Beyond the nutrient strategies: common ground to accelerate agricultural water quality improvement in the upper Midwest. J. Environ. Manag. 206, 1072–1080. doi: 10.1016/j.jenvman.2017.11.051
Condon, L. E., Atchley, A. L., and Maxwell, R. M. (2020). Evapotranspiration depletes groundwater under warming over the contiguous United States. Nat. Commun. 11, 873. doi: 10.1038/s41467-020-14688-0
Dartt, B. A., Lloyd, J. W., Radke, B. R., Black, J. R., and Kaneene, J. B. (1999). A comparison of profitability and economic efficiencies between management—intensive grazing and conventionally managed dairies in Michigan. J. Dairy Sci. 82, 2412–2420. doi: 10.3168/jds.S0022-0302(99)75492-5
Dodds, W., and Smith, V. (2016). Nitrogen, phosphorus, and eutrophication in streams. Inland Waters 6, 155–164. doi: 10.5268/IW-6.2.909
Donner, S. D., and Kucharik, C. J. (2003). Evaluating the impacts of land management and climate variability on crop production and nitrate export across the Upper Mississippi Basin. Glob. Biogeochem. Cycles 17, n/a-n/a. doi: 10.1029/2001GB001808
Feed Composition Library | Dairy One (2022). Dairy One Feed Compos. Libr. Available online at: https://dairyone.com/services/forage-laboratory-services/feed-composition-library/ (accessed June 24, 2022).
Ferin, K. M., Chen, L., Zhong, J., Acquah, S., Heaton, E. A., Khanna, M., et al. (2021). Water quality effects of economically viable land use change in the Mississippi River Basin under the renewable fuel standard. Environ. Sci. Technol. 55, 1566–1575. doi: 10.1021/acs.est.0c04358
Foley, J. A., DeFries, R., Asner, G. P., Barford, C., Bonan, G., Carpenter, S. R., et al. (2005). Global consequences of land use. Science 309, 570–574. doi: 10.1126/science.1111772
Foley, J. A., Ramankutty, N., Brauman, K. A., Cassidy, E. S., Gerber, J. S., Johnston, M., et al. (2011). Solutions for a cultivated planet. Nature 478, 337–342. doi: 10.1038/nature10452
Godfray, H. C. J., Beddington, J. R., Crute, I. R., Haddad, L., Lawrence, D., Muir, J. F., et al. (2010). Food security: the challenge of feeding 9 billion people. Science 327, 812–818. doi: 10.1126/science.1185383
gridMET (2013). Climatol. Lab. Available online at: https://www.climatologylab.org/gridmet.html (accessed June 30, 2022).
Guillaume, T., Makowski, D., Libohova, Z., Elfouki, S., Fontana, M., Leifeld, J., et al. (2022). Carbon storage in agricultural topsoils and subsoils is promoted by including temporary grasslands into the crop rotation. Geoderma 422, 115937. doi: 10.1016/j.geoderma.2022.115937
Hanson, J. C., Johnson, D. M., Lichtenberg, E., and Minegishi, K. (2013). Competitiveness of management-intensive grazing dairies in the mid-Atlantic region from 1995 to 2009. J. Dairy Sci. 96, 1894–1904. doi: 10.3168/jds.2011-5234
Hussain, M. Z., Bhardwaj, A. K., Basso, B., Robertson, G. P., and Hamilton, S. K. (2019). Nitrate leaching from continuous corn, Perennial Grasses, and poplar in the US Midwest. J. Environ. Qual. 48, 1849–1855. doi: 10.2134/jeq2019.04.0156
Jackson, L., and Keeney, D. (2010). “Perennial farming systems that resist flooding,” in A Watershed Year: Anatomy of the Iowa Floods of 2008 A Bur Oak Book. (Iowa City: University of Iowa Press).
Jackson, R. D. (2020). Soil nitrate leaching under grazed cool-season grass pastures of the North Central US. J. Sci. Food Agric. 100, 5307–5312. doi: 10.1002/jsfa.10571
Jackson, R. D. (2022). Grazed perennial grasslands can match current beef production while contributing to climate mitigation and adaptation. Agric. Environ. Lett. 7. doi: 10.1002/ael2.20059
Johnson, I. R., Chapman, D. F., Snow, V. O., Eckard, R. J., Parsons, A. J., Lambert, M. G., et al. (2008). DairyMod and EcoMod: biophysical pasture-simulation models for Australia and New Zealand. Aust. J. Exp. Agric. 48, 621. doi: 10.1071/EA07133
Jordan, N. R., Mulla, D. J., Slotterback, C., Runck, B., and Hays, C. (2018). Multifunctional agricultural watersheds for climate adaptation in Midwest USA: commentary. Renew. Agric. Food Syst. 33, 292–296. doi: 10.1017/S1742170517000655
Kimoto, C., DeBano, S. J., Thorp, R. W., Taylor, R. V., Schmalz, H., DelCurto, T., et al. (2012). Short-term responses of native bees to livestock and implications for managing ecosystem services in grasslands. Ecosphere 3, art88. doi: 10.1890/ES12-00118.1
Knobeloch, L., Gorski, P., Christenson, M., and Anderson, H. (2013). Private drinking water quality in rural Wisconsin. J. Environ. Health 75, 7.
Kremen, C., and Merenlender, A. M. (2018). Landscapes that work for biodiversity and people. Science 362, eaau6020. doi: 10.1126/science.aau6020
Kriegl, T. (2005). Pastures of Plenty: Financial Performance of Wisconsin Grazing Dairy Farms. University of Wisconsin-Madison.
Kucharik, C. J. (2003). Evaluation of a process-based agro-ecosystem model (Agro-IBIS) across the U.S. Corn belt: simulations of the interannual variability in Maize Yield. Earth Interact. 7, 1–33. doi: 10.1175/1087-3562(2003)007<0001:EOAPAM>2.0.CO;2
Kucharik, C. J., Barford, C. C., Maayar, M. E., Wofsy, S. C., Monson, R. K., and Baldocchi, D. D. (2006). A multiyear evaluation of a dynamic global vegetation model at three AmeriFlux forest sites: vegetation structure, phenology, soil temperature, and CO2 and H2O vapor exchange. Ecol. Model. 196, 1–31. doi: 10.1016/j.ecolmodel.2005.11.031
Kucharik, C. J., and Brye, K. R. (2003). Integrated biosphere simulator (IBIS) yield and nitrate loss predictions for wisconsin maize receiving varied amounts of nitrogen fertilizer. J. Environ. Qual. 32, 247–268. doi: 10.2134/jeq2003.2470
Kucharik, C. J., Foley, J. A., Delire, C., Fisher, V. A., Coe, M. T., Lenters, J. D., et al. (2000). Testing the performance of a dynamic global ecosystem model: water balance, carbon balance, and vegetation structure. Glob. Biogeochem. Cycles 14, 795–825. doi: 10.1029/1999GB001138
Kucharik, C. J., and Twine, T. E. (2007). Residue, respiration, and residuals: evaluation of a dynamic agroecosystem model using eddy flux measurements and biometric data. Agric. For. Meteorol. 146, 134–158. doi: 10.1016/j.agrformet.2007.05.011
Lark, T. J., Hendricks, N. P., Smith, A., Pates, N., Spawn-Lee, S. A., Bougie, M., et al. (2022). Environmental outcomes of the US renewable fuel standard. Proc. Natl. Acad. Sci. 119, e2101084119. doi: 10.1073/pnas.2101084119
Lesk, C., Rowhani, P., and Ramankutty, N. (2016). Influence of extreme weather disasters on global crop production. Nature 529, 84–87. doi: 10.1038/nature16467
Lioutas, E. D., and Charatsari, C. (2021). Enhancing the ability of agriculture to cope with major crises or disasters: what the experience of COVID-19 teaches us. Agric. Syst. 187, 103023. doi: 10.1016/j.agsy.2020.103023
Liu, J., Bowling, L., Kucharik, C., Jame, S., Baldos, U., Jarvis, L., et al. (2022). Multi-scale analysis of nitrogen loss mitigation in the US Corn Belt. arXiv doi: 10.48550/ARXIV.2206.07596
McGuire, J., Morton, L. W., and Cast, A. D. (2013). Reconstructing the good farmer identity: shifts in farmer identities and farm management practices to improve water quality. Agric. Hum. Values 30, 57–69. doi: 10.1007/s10460-012-9381-y
Montgomery, D. R. (2007). Soil erosion and agricultural sustainability. Proc. Natl. Acad. Sci. 104, 13268–13272. doi: 10.1073/pnas.0611508104
Motew, M., Chen, X., Booth, E. G., Carpenter, S. R., Pinkas, P., Zipper, S. C., et al. (2017). The Influence of legacy P on lake water quality in a midwestern agricultural watershed. Ecosystems 20, 1468–1482. doi: 10.1007/s10021-017-0125-0
Motew, M. M., and Kucharik, C. J. (2013). Climate-induced changes in biome distribution, NPP, and hydrology in the Upper Midwest U.S.: a case study for potential vegetation: climate impacts in the upper Midwest U.S. J. Geophys. Res. Biogeosci. 118, 248–264. doi: 10.1002/jgrg.20025
Nassauer, J. I. (1988). The aesthetics of horticulture: neatness as a form of care. HortScience 23, 973–977. doi: 10.21273/HORTSCI.23.6.973
Orth, R. J., Carruthers, T. J. B., Dennison, W. C., Duarte, C. M., Fourqurean, J. W., Heck, K. L., et al. (2006). A global crisis for seagrass ecosystems. BioScience 56, 987. doi: 10.1641/0006-3568(2006)56[987:AGCFSE]2.0.CO;2.
Ortiz-Bobea, A. (2021). Anthropogenic climate change has slowed global agricultural productivity growth. Nat. Clim. Chang. 11, 28. doi: 10.1038/s41558-021-01000-1
Palm, C., Blanco-Canqui, H., DeClerck, F., Gatere, L., and Grace, P. (2014). Conservation agriculture and ecosystem services: an overview. Agric. Ecosyst. Environ. 187, 87–105. doi: 10.1016/j.agee.2013.10.010
Peters, C. J., Picardy, J. A., Darrouzet-Nardi, A., and Griffin, T. S. (2014). Feed conversions, ration compositions, and land use efficiencies of major livestock products in U.S. agricultural systems. Agric. Syst. 130, 35–43. doi: 10.1016/j.agsy.2014.06.005
Pinheiro, J., Bates, D., DebRoy, S., Sarkar, D., HeisterKamp, S., Van Willigen, B., et al. (2022). Package “nlme.” Available online at: https://svn.r-project.org/R-packages/trunk/nlme/ (accessed June 13, 2022).
PRISM Climate Group Oregon State University. (2022). Available online at: https://prism.oregonstate.edu/ (accessed July 27, 2022).
Provenza, F. D., Anderson, C., and Gregorini, P. (2021). We are the Earth and the Earth Is Us: How palates link foodscapes, landscapes, heartscapes, and thoughtscapes. Front. Sustain. Food Syst. 5, 547822. doi: 10.3389/fsufs.2021.547822
Qiu, G., Li, H., Zhang, Q., Chen, W., Liang, X., and Li, X. (2013). Effects of evapotranspiration on mitigation of urban temperature by vegetation and urban agriculture. J. Integr. Agric. 12, 1307–1315. doi: 10.1016/S2095-3119(13)60543-2
Raff, Z., and Meyer, A. (2022). CAFOs and surface water quality: evidence from Wisconsin. Am. J. Agric. Econ. 104, 161–189. doi: 10.1111/ajae.12222
Rissman A. R. Fochesatto A. Lowe E. B. Lu Y. Hirsch R. M. Jackson R. D. (in this volume). Grasslands managed grazing policy review: trends options from the Midwestern United States. Front. Sustain. Food Syst. (in this volume).
Rockström, J., Williams, J., Daily, G., Noble, A., Matthews, N., Gordon, L., et al. (2017). Sustainable intensification of agriculture for human prosperity and global sustainability. Ambio 46, 4–17. doi: 10.1007/s13280-016-0793-6
Rowntree, J. E., Stanley, P. L., Maciel, I. C. F., Thorbecke, M., Rosenzweig, S. T., Hancock, D. W., et al. (2020). Ecosystem impacts and productive capacity of a multi-species pastured livestock system. Front. Sustain. Food Syst. 4. doi: 10.3389/fsufs.2020.544984
Rui, Y., Jackson, R. D., Cotrufo, M. F., Sanford, G. R., Spiesman, B. J., Deiss, L., et al. (2022). Persistent soil carbon enhanced in Mollisols by well-managed grasslands but not annual grain or dairy forage cropping systems. Proc. Natl. Acad. Sci. 119, e2118931119. doi: 10.1073/pnas.2118931119
Sanford, G. R., Jackson, R. D., Booth, E. G., Hedtcke, J. L., and Picasso, V. (2021). Perenniality and diversity drive output stability and resilience in a 26-year cropping systems experiment. Field Crops Res. 263, 108071. doi: 10.1016/j.fcr.2021.108071
Schilling, K. E., Gassman, P. W., Kling, C. L., Campbell, T., Jha, M. K., Wolter, C. F., et al. (2014). The potential for agricultural land use change to reduce flood risk in a large watershed: land use change and flooding. Hydrol. Process. 28, 3314–3325. doi: 10.1002/hyp.9865
Schindler, D. W., Carpenter, S. R., Chapra, S. C., Hecky, R. E., and Orihel, D. M. (2016). Reducing phosphorus to curb lake eutrophication is a success. Environ. Sci. Technol. 50, 8923–8929. doi: 10.1021/acs.est.6b02204
Schulte, L. A., Niemi, J., Helmers, M. J., Liebman, M., Arbuckle, J. G., James, D. E., et al. (2017). Prairie strips improve biodiversity and the delivery of multiple ecosystem services from corn–soybean croplands. Proc. Natl. Acad. Sci. 114, 11247–11252. doi: 10.1073/pnas.1620229114
Smith, A. P., Johnson, I. R., Schwenke, G., Lam, S. K., Suter, H. C., and Eckard, R. J. (2020). Predicting ammonia volatilization from fertilized pastures used for grazing. Agric. For. Meteorol. 287, 107952. doi: 10.1016/j.agrformet.2020.107952
Soylu, M. E., Kucharik, C. J., and Loheide, S. P. (2014). Influence of groundwater on plant water use and productivity: development of an integrated ecosystem—variably saturated soil water flow model. Agric. For. Meteorol. 189–190, 198–210. doi: 10.1016/j.agrformet.2014.01.019
Strauser, J., Stewart, W. P., and Leitschuh, B. (2022). Producing regions: connecting place-making with farming practices. Soc. Nat. Resour. 35, 1–9. doi: 10.1080/08941920.2022.2101080
Suparak Gibson, A. (2022). The Underground Economy: Regenerative Farming's Hidden Economic, Ecological, and Social Value. Master's Thesis. Harvard University Division of Continuing Education.
Tsiafouli, M. A., Thébault, E., Sgardelis, S. P., de Ruiter, P. C., van der Putten, W. H., Birkhofer, K., et al. (2015). Intensive agriculture reduces soil biodiversity across Europe. Glob. Chang. Biol. 21, 973–985. doi: 10.1111/gcb.12752
USDA—Economic Research Service. (2018). Soybean U.S. stocks. Available online at: https://www.ers.usda.gov/ (accessed October 31, 2020).
USDA—FoodData Central USDA Agricultural Research Service (2022). FoodData Cent. Available online at: https://fdc.nal.usda.gov/ (accessed June 24, 2022).
USDA-NASS—National Agricultural Statistics Service (2022). Charts and Maps - County Maps. Available online at: https://www.nass.usda.gov/Charts_and_Maps/Crops_County/index.php (accessed June 15, 2022).
Vadas, P. A., Gburek, W. J., Sharpley, A. N., Kleinman, P. J. A., Moore, P. A., Cabrera, M. L., et al. (2007). A model for phosphorus transformation and runoff loss for surface-applied manures. J. Environ. Qual. 36, 324–332. doi: 10.2134/jeq2006.0213
Vadas, P. A., Haggard, B. E., and Gburek, W. J. (2005). Predicting dissolved phosphorus in runoff from manured field plots. J. Environ. Qual. 34, 1347–1353. doi: 10.2134/jeq2004.0424
Vadas, P. A., Kleinman, P. J. A., and Sharpley, A. N. (2004). A simple method to predict dissolved phosphorus in runoff from surface-applied manures. J. Environ. Qual. 33, 749–756. doi: 10.2134/jeq2004.7490
van Vliet, S., Bain, J. R., Muehlbauer, M. J., Provenza, F. D., Kronberg, S. L., Pieper, C. F., et al. (2021a). A metabolomics comparison of plant-based meat and grass-fed meat indicates large nutritional differences despite comparable nutrition facts panels. Sci. Rep. 11, 13828. doi: 10.1038/s41598-021-93100-3
van Vliet, S., Provenza, F. D., and Kronberg, S. L. (2021b). Health-promoting phytonutrients are higher in grass-fed meat and milk. Front. Sustain. Food Syst. 4, 555426. doi: 10.3389/fsufs.2020.555426
Wepking, C., Avera, B., Badgley, B., Barrett, J. E., Franklin, J., Knowlton, K. F., et al. (2017). Exposure to dairy manure leads to greater antibiotic resistance and increased mass-specific respiration in soil microbial communities. Proc. R. Soc. B Biol. Sci. 284, 20162233. doi: 10.1098/rspb.2016.2233
Werling, B. P., Dickson, T. L., Isaacs, R., Gaines, H., Gratton, C., Gross, K. L., et al. (2014). Perennial grasslands enhance biodiversity and multiple ecosystem services in bioenergy landscapes. Proc. Natl. Acad. Sci. 111, 1652–1657. doi: 10.1073/pnas.1309492111
Wheeler, T., and von Braun, J. (2013). Climate change impacts on global food security. Science 341, 508–513. doi: 10.1126/science.1239402
Wisconsin Groundwater Coordinating Council Report to the Legislature (2022). Wisconsin Groundwater Coordinating Council.
Keywords: agroecology, ecosystem services, livestock, grazing, multifunctionality
Citation: Wepking C, Mackin HC, Raff Z, Shrestha D, Orfanou A, Booth EG, Kucharik CJ, Gratton C and Jackson RD (2022) Perennial grassland agriculture restores critical ecosystem functions in the U.S. Upper Midwest. Front. Sustain. Food Syst. 6:1010280. doi: 10.3389/fsufs.2022.1010280
Received: 02 August 2022; Accepted: 15 November 2022;
Published: 02 December 2022.
Edited by:
Jacob Jungers, University of Minnesota Twin Cities, United StatesReviewed by:
Ignacio Macedo, University of California, Davis, United StatesJoel Tallaksen, University of Minnesota Twin Cities, United States
Copyright © 2022 Wepking, Mackin, Raff, Shrestha, Orfanou, Booth, Kucharik, Gratton and Jackson. This is an open-access article distributed under the terms of the Creative Commons Attribution License (CC BY). The use, distribution or reproduction in other forums is permitted, provided the original author(s) and the copyright owner(s) are credited and that the original publication in this journal is cited, in accordance with accepted academic practice. No use, distribution or reproduction is permitted which does not comply with these terms.
*Correspondence: Carl Wepking, cwepking@wisc.edu
†ORCID: Carl Wepking orcid.org/0000-0003-1910-5999
Hunter C. Mackin orcid.org/0000-0002-4500-5046
Zach Raff orcid.org/0000-0002-3248-8835
Debendra Shrestha orcid.org/0000-0002-4594-078X
Anna Orfanou orcid.org/0000-0002-0922-3441
Eric G. Booth orcid.org/0000-0003-2191-6627
Christopher J. Kucharik orcid.org/0000-0002-0400-758X
Claudio Gratton orcid.org/0000-0001-6262-9670
Randall D. Jackson orcid.org/0000-0002-8033-1250