Evaluation of water-user performance in smallholder irrigation schemes in KwaZulu-Natal Province, South Africa: A stochastic meta-frontier analysis
- 1Department of Agricultural Economics, Rural Development and Extension, University of Pretoria, Pretoria, South Africa
- 2Discipline of Agricultural Economics, University of KwaZulu-Natal, Pietermaritzburg, South Africa
- 3Gordon Institute of Business Science, University of Pretoria, Pretoria, South Africa
Smallholder Irrigation Schemes (SIS) are pivotal in sustaining livelihoods and creating employment in rural communities of South Africa. However, despite the revitalization and rehabilitation of SIS by the government, the performance of farmers is still below par. This study evaluates the performance of water-users across four SIS in KwaZulu-Natal Province (KZN). Technical Efficiency was used as a proxy for performance and the Stochastic Meta-Frontier Analysis method was employed to measure the overall efficiency across 306 households. The efficiency model results showed that governance index, psychological capital, land tenure security, credit access and gender affect water-users' technical efficiency. The study concludes that interventions should be geared toward strengthening institutions and the empowerment of farmers through relevant training and access to credit. Furthermore, adequacy of water and its availability in the schemes should be improved to ensure profitability and productivity of water.
Introduction
Over the years, climate change has impacted South African agriculture as evident in rapid changes in temperatures and rainfall, which subsequently puts pressure on the demand for water in agricultural production (Bernstein, 2013). As such, irrigation is increasingly becoming a critical factor in enhancing agricultural productivity and consequently alleviating poverty in rural areas (Sinyolo et al., 2014). It is estimated that about 1.3 million hectares of land in South Africa are under irrigation; of which about 0.1 million hectares are under smallholder irrigation schemes (SIS) (Van Averbeke, 2008). SIS are defined as “agricultural projects that involve multiple holdings, which depend on a shared distribution system for access to irrigation water” (Van Averbeke, 2012; 413). In South Africa, SIS were mainly put in place with the primary focus of food security of the irrigators (Van Averbeke, 2012), however, an increasing number of smallholder farmers are gradually shifting from subsistence to commercial production.
In general, SISs and their water users in the country are underperforming due to a combination of infrastructural and economic factors (Van Averbeke et al., 2011; Muchara et al., 2014; Sinyolo et al., 2014). However, often overlooked, is the potential effect of institutional arrangements on farmers' performance in SISs. Institutions are defined in several ways in different schools of thought. In water resource management, institutions are ground rules for resource use, and can either be formal or informal. The former includes policies, rules, regulations, organizations, plans, procedures, while the latter represents norms, traditions, practices, and customs (Bandaragoda, 2001; Muller, 2012; Muchara et al., 2014). Institutions have the potential to affect the costs of exchange and production, as well as the behavior of decision-making entities, thus affecting their performance. Given the lack of studies that link performance to institutions, this study aims to assess the technical efficiency of water users in SIS and answer the following question: what are the institutional, socio-economic, economic and technical factors that affect water user performance in SIS in KwaZulu-Natal? Several factors have been found to influence farmer performance as shown in literature.
Manjunatha et al. (2013) analyzed the impact of land fragmentation, land ownership and farm size on farm technical efficiency. The study found that smaller farms have lower inefficiencies, this was consistent with Debebe et al. (2015) who found that smallholding size of land yield better crop efficiencies due to lower transaction costs and easier management compared to larger farms. In contrast, Rios and Shively (2016) found that small farms were less efficient than larger farms due to scale investments in irrigation infrastructure. Land ownership was also found to positively affect farm performance (Manjunatha et al., 2013; Ebarle et al., 2014). A study conducted in Southern Philippines on the profitability of vegetable farmers suggested that farmers that owned land had a higher probability of running profitable farm businesses (Ebarle et al., 2014), which was also found to be the case in India (Manjunatha et al., 2013).
Literature highlights several economic factors that affect farm performance, and these include low production costs, high agricultural yields and prices, as well low marketing costs (Dlamini and Masuku, 2013; Ebarle et al., 2014; Mushi and Ngaruko, 2015). Dlamini and Masuku (2013) found that farming experience has a positive influence on farm profitability amongst sugarcane farmers in Swaziland Social factors such as farmer groups and cooperatives have been found to improve farm performance (Debebe et al., 2015; Herbert et al., 2015). A study conducted in the Democratic Republic of Congo, Burundi and Rwanda showed that farmers who were members of farmer groups performed better than those that were not and had a higher probability of being economically or financially efficient in their agricultural production (Herbert et al., 2015). This was due to the sharing of valuable information.
Debebe et al. (2015) found that access to extension services is an important factor that affects farm performance because extension services provide farmers with new information and provide recommendations on agricultural activities and market access. Mumba et al. (2012) found that the closer the farm is to the market, the higher the probabilities of farm profitability as shorter distances to markets usually translate into lower transaction costs. Personal farmer characteristics such as age, and gender has also been found to influence farmer performance (Dlamini and Masuku, 2013; Debebe et al., 2015; Mushi and Ngaruko, 2015; Gandasari et al., 2021). Gandasari et al. (2021) found that the age of the farmer has a negative effect on performance, as older farmers are less likely to seek new information, are reluctant to adopt innovative practices, and stick to traditional. Furthermore, (Maniriho et al., 2021) suggest that the older the farmer, the less fit they may be to carry out activities, even though they have a wealth of experience. Agyeman et al. (2014) found a positive effect of female household head on household income diversification, which contrasted with the findings of Maniriho et al. (2021), who found an inverse results, as depicted by a significant differential of farm income between male and female farmers. In assessing performance, previous studies have adopted several proxies and measures of performance; this has also resulted in the use of techniques used to analyse the factors which affect performance (Ojo, 2006; Speelman et al., 2008; Verhofstadt and Maertens, 2014; Tajbakhsh and Hassini, 2015).
Different methods, including but not limited to accounting, economic, agricultural, and environmental (performance) indicators have been used to evaluate factors affecting farmer performance (Speelman et al., 2008; Verhofstadt and Maertens, 2014). The performance of a farm can be assessed by estimating its efficiency and productivity. Technical Efficiency (TE) can be estimated by comparing the observed output to the potential maximum optimum output, given the total inputs used. TE is attained when a farm can produce the maximum feasible output from a given bundle of inputs, or by using the minimum feasible amounts of inputs to produce a certain level of outputs, using a particular technology (Ojo, 2006; Speelman et al., 2008).
TE is generally measured using Data Envelopment Analysis (DEA) or the Stochastic Frontier Analysis (SFA). The DEA is a non-parametric approach which enables the evaluation of relative efficiencies of a set of comparable outcomes and does not require that the explanatory variables be estimated (Tajbakhsh and Hassini, 2015). SFA is a parametric approach used when several independent variables can be estimated (Buckley and Carney, 2013). The method considers that individual observations may be affected by random noise and enables the modeling of productivity and efficiency (Bogetoft and Otto, 2010). Additionally, it can disentangle the inefficiency from the standard statistical error related and allows the assessment of determinants of inefficiency (Amsler et al., 2017). Using SFA, Wang et al. (2021) found that land fragmentation negatively affected farmer performance in the farming provinces in China. Cillero and Reaños (2022) used SFA in modeling farmer performance and found that a positive relationship existed between technical efficiency and the Green, Low-Carbon, Agri-Environment Scheme for dairy farms in Ireland. Kostlivý et al. (2020) found that lower altitude-based farms, innovative farms, and those that use more sustainable ways of farming are more productive, they used SFA to model performance in Czech crop farms. This study estimates technical efficiencies of irrigators in four irrigation schemes using the SFA through modeling Cobb-Douglas and Translog production.
Due to the noticeable underperformance of SIS, several studies have attempted to identify key issues, particularly relating to institutions, governance, and socioeconomic patterns (Gomo et al., 2014; Sinyolo et al., 2014; Dlangalala and Mudhara, 2020; Mwadzingeni et al., 2020; Mugejo et al., 2022). The studies considered various aspects such as the water security of water-users (Sinyolo et al., 2014), the assessment of local institutional actors on scheme performance (Mwadzingeni et al., 2020), farmer satisfaction of scheme performance (Gomo et al., 2014), assessment of performance of irrigation infrastructure and governance system (Mugejo et al., 2022), and the awareness of water governance across gender and intergenerational dimensions (Dlangalala and Mudhara, 2020). Furthermore, there are few studies that assessed performance of SIS using SFA such as Makombe et al. (2017), Oulmane and Benmehala (2019), and Morais et al. (2021). The studies assessed farm performance across different irrigation typologies (Makombe et al., 2017), effects of irrigation technology adoption (Oulmane and Benmehala, 2019), and assessment of performance between irrigators and non-irrigators (Morais et al., 2021). The SIS included in this study use different methods of irrigation, which constitutes technology. The method of irrigation affects water access, availability, which consequently affects the productivity of the farmers. As such, the contribution of this study is that it considers the performance of water-users in four SISs with varying irrigation systems and institutional arrangements in KwaZulu-Natal. The SIS differences are captured using a stochastic meta-frontier analysis, instead of pooling or estimating various individual SFA derived production functions. Furthermore, the governance aspect is quantitatively derived and included amongst other institutional variables to determine efficiency across the SIS. To our knowledge this is the first study to use a stochastic meta-frontier analysis to analyze water-user performance in SIS that have varying institutional arrangements and technologies, while considering inefficiency effects of socioeconomic, economic, technical, and institutional variables. The estimation of measures of technical efficiency, including exogenous variables enables policy recommendations to be made, not only to improve technical efficiency but also to provide an opportunity to enhance performance through improved institutional arrangements. This study aims to provide empirical evidence of the technical efficiency of water-users as well as the inefficiency effects in SIS in KwaZulu-Natal, South Africa. The following section outlines the conceptual framework adopted and is followed by the methodology, presentation and discussion of results, conclusions, and recommendations.
Methodology
Study sites and data collection
The study is focused on four irrigation schemes in KwaZulu-Natal Province, namely Tugela Ferry, Ndumo, Mooi River, and Makhathini Flats. The schemes were chosen due to their contrasting characteristics, including governance regimes, farmer compositions, irrigation type and region. Makhathini Flats (MFIS) and Ndumo (NIS) Irrigation Schemes are situated in the Umkhanyakude District, under the Jozini Local Municipality, north-eastern KZN. The Makhathini Flats Irrigation Scheme comprises of individual (mostly males) and cooperative irrigators (mostly women). The individual farmers produce sugarcane, while the cooperatives mostly produce vegetables. The individual irrigators operate on land provided by the land trusts (under tribal authorities), and the cooperative irrigators operate on state-owned land. The Ndumo B Irrigation scheme is a 500 ha scheme operated by 50 farmers. The farmers produce a wide variety of commodities and production is more commercially oriented. The irrigators belong to two cooperatives, one representing the older and the other, newer block.
The Tugela Ferry (TFIS) and Mooi River Irrigation Schemes (MRIS) are situated in central KZN, under the uMzinyathi District, in the Msinga Local Municipality. The MRIS comprises of about 840 irrigators, drawing water from a 25 km scheme. It is undergoing rehabilitation, and water access is extremely variable. TFIS comprises about 1,500 farmers on 800 ha of land. The TFIS irrigators use various water transmission mechanisms (canal via gravity, diesel and electric pumps) and farmer production is more for subsistence. The farmers operate on an average of 0.4 ha of land allocated through the local tribal authority. Figure 1 shows the location of the irrigation schemes across the province.
The systematic random sampling was employed, 306 farmers were selected. Due to the different sizes of the scheme, 100 farmers were selected in the MRIS and TFIS, located in the Msinga Local Municipality. The same sampling procedure was used in the Jozini local municipality, where 60 farmers from the MFIS and 41 from the NIS were selected. Focus group discussions (FGD) were also held in each of the schemes.
Irrigation technology and key contrasting characteristics of the schemes:
• Mooi River Irrigation Scheme.
MRIS is situated in the Msinga Local Municipality. Irrigation water flows through a gravity flow canal from the Mooi River. The scheme is divided into three parts, the upper, middle, and lower tail of the canal, comprising fifteen blocks in total. Farmers get water through distribution canals on specifically scheduled days. The schedule is set by farmers and block committees. The land is allocated by the local tribal authority, and farmers are part of agricultural cooperatives.
• Tugela Ferry Irrigation Scheme.
TFIS is also situated in the Msinga Local Municipality, and farmers irrigate through a short-furrow system. It is an older irrigation scheme that spans across 540 hectares with about 1,500 water-users. Plots in the scheme are allocated by the local tribal authority. Most of the farmers are not part of cooperatives, and water access is subject to fee payment.
• Makhathini Flats Irrigation Scheme.
MFIS is situated in the Jozini Local Municipality, and irrigation water is extracted through a main canal, conveying water to the plots. The scheme has about 538 hectares in use by 600 plot holders. The land is allocated through trusts, and a monthly fee must be paid to the local managing agency for water access. Some farmers in the scheme occupy about 10 hectares of land, while the rest, who are part of cooperatives produce on smaller plots.
• Ndumo Irrigation Scheme.
NIS is situated in the Jozini Local Municipality, is about 500 hectares occupied by about 50 farmers. An underground water conveyance system is used for the extraction of water and diverted into the plots through pipes. Farmers do not pay for water but pay for electricity pro-rata. There are two main cooperatives that farmers belong to the scheme. Land is allocated through the local tribal authority.
Theoretical FrameworkA farmer uses various inputs to yield a quantity of produce that is sold to generate a certain amount of revenue. Assuming that an ith farmer belonging to jth irrigation scheme combines a number of Xi inputs, to attain a Yi in sales, technical efficiency can be associated with a set of group and farm-specific exogenous variables. Technical efficiency is the maximization of output given a certain number of inputs and can be outlined on a production frontier. A meta-frontier envelopes the group-specific efficiencies and helps with the derivation of the technology gap ratio (TGR), which indicates the distance between farms in a certain group to the most efficient in the sample. A meta-frontier is represented in Figure 2, enveloping the group-specific Frontiers 1, 2, 3 and 4. At any given input xi, a water-user's given output relative to the meta-frontier, FM (xji) includes the technology gap-ratio, the water-users' technical efficiency (Fi(xji)), as well as the random noise term (e-ujt <) (Huang et al., 2014).
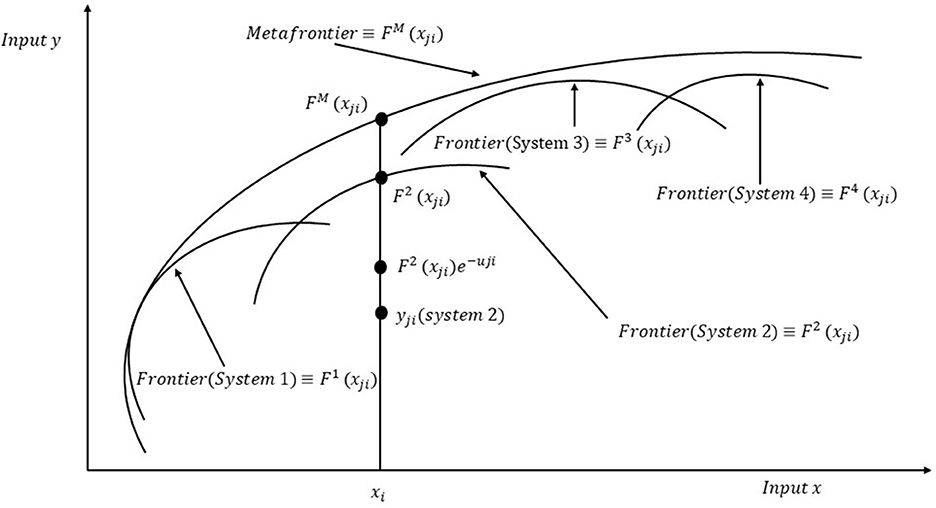
Figure 2. Concept of a stochastic meta-frontier. Source: Melo-Becerra and Orozco-Gallo (2017).
Empirical method of evaluating technical efficiency of water-users in SIS
Water-users maximize output using a combination of inputs. Given the differences in institutional arrangements and production systems, the technical efficiency of water-users is not comparable using a comprehensive production frontier (Huang et al., 2014; Melo-Becerra and Orozco 2017). In maximizing output, the choice of production and input combinations may be affected by factors such as climatic conditions, institutional arrangements, land tenure and water availability, that are beyond the individual's control. Battese and Rao (2002) introduced the application of a meta-frontier to estimate technical efficiencies across different groups. Battese and Rao (2002) and O'Donnell et al. (2008) later introduced a two-step method of estimating technical efficiencies of decision-making units. The first step involves the calculation of efficiencies using stochastic frontiers, while the second stage is composed of linear programming methods to estimate the meta-frontiers. Huang et al. (2014) proposed a new technique that considers a stochastic meta-frontier instead of a mathematical linear programming approach. The advantage of a stochastic meta-frontier method is that statistical properties of the frontier are ensured in the second stage, thus controlling for white noise, as opposed to the mathematical model. As such, this study adopts a Stochastic Meta-frontier Analysis to estimate the technical efficiencies of water-users in the four irrigation schemes.
Suppose for the jth irrigation scheme, the ith water-user's stochastic frontier at a certain period is modeled as:
Where Yji denotes the scalar output at time t, and xjit denotes the scalar inputs of the ith water user in the jth irrigation scheme. The random errors vjit represent statistical noise; a normally distributed error term that captures noise with the notion that deviations from the frontier are due to variables beyond the control of the water-user. ujit are non-zero error terms which represent technical inefficiency. The assumption is that the vji's are distributed independently and are independent of the Uji's (Melo-Becerra and Orozco-Gallo, 2017). The technical efficiency in production is then given by Equation 2:
The technical efficiency is associated with a set of farm-specific exogenous variables within the irrigation schemes. The meta-frontier production function for all water-users in the schemes is defined as fM(xji), where the function is the same for all irrigation schemes. The meta-frontier fM(xji), envelopes scheme production frontiers f (xji), in the following relationship.
where – is ≥0, therefore at any given period, fM(.)>f (.) and the ratio between the jth irrigation scheme production frontier and the meta-production frontier gives the Technology Gap Ratio (TGR);
Thus, at any given input level, a water-user's observed output Yji, relative to the meta-frontier (fM(xji) consists of three components, the TGR = e−uMji; the water-user's TE = e−uji; and the random noise term .
Therefore, at a period of time;
Following the approach by Huang et al. (2014), the meta-frontier estimation is summarized in Equations 6 and 7.
where ln (Xji) represents the estimates of the irrigation scheme-specific frontier, which should be estimated j times. The estimates ln fj (Xji) from all the schemes are then pooled to estimate the meta-frontier (equation 7). To ensure that the estimated meta-frontier is greater or equal to the scheme frontiers, the TGR is always less or equal to unity. The model was estimated using the Cobb-Douglas and Translog production functions as they are commonly used in efficiency studies in agriculture (Ghoshal and Goswami, 2017; Makombe et al., 2017; Sujan et al., 2017; Njuki and Bravo-Ureta, 2018). The estimated inefficiency models are given by equation 8, as:
where Uji represents the inefficiency of a water-user in the jth irrigation scheme, O0 represents the constant term, Wj represent the exogenous variables in the model and Oji represents unknown parameter estimates. The choice of functional form to be used in estimating a stochastic production function is important. The Translog and Cobb-Douglas production functions are commonly used in efficiency studies (Ghoshal and Goswami, 2017; Makombe et al., 2017; Sujan et al., 2017). Cobb-Douglas is usually tested against a relatively flexible function form, such as the Translog function (Bezat, 2011; Makombe et al., 2017). Additionally, a test to determine whether inefficiency effects need to be included in the model is performed. This indicates whether non-production or exogenous variables should be included in the model. As such two hypotheses are tested, i.e., that the Cobb-Douglas is more appropriate than the Translog production function, and that inefficiency effects should be included in the model.
Results and discussion
Description of explanatory variables and descriptive statistics
The study estimated the models with a mix of continuous and categorical socio-economic and production variables. Three input variables were used for the estimation of the production function as determinants of efficiency, while ten exogenous variables were used for the inefficiency model. The variables with their expected regression signs are presented in Table 1. The output variable is presented by the product of the quantity produced and price per farm. The area planted was measured in hectares and indicated the total amount of land used in production, fertilizer was computed as the total amount of fertilizer used in production, measured in kilograms. The number of irrigation hours was used as a proxy for irrigation due to the challenge of measuring the water used, as there are no water meters in the irrigation systems. All the production variables were entered in the TE model in logarithmic form. The tenure security variable presents whether an irrigator feels satisfied with the security of land or not. The Governance index is a composite household-level index, generated through Principal Components Analysis (PCA), which includes dimensions such as awareness of policies, perceptions toward stakeholder involvement as well as informal institutions in the schemes. It was computed from likert-scale formulated questions, where farmers would rank their views on the governance in the scheme. Psychological capital is an index which considers how the water user perceives themselves, in terms of their confidence, resilience and hope. Similar to the construction of the Governance Index, farmers were asked questions that would capture elements of self-confidence, business aspiration, and willingness to invest, learn and innovate to advance their farming activities.
Table 1 also presents the descriptive statistics of variables used in the model. The results show that the average household size in the schemes is four. It is also evident that water-users in NIS utilize more land than farmers in the other SISs. With an average of 8 hectares, the farmers are more commercially oriented. This is also evident from the average revenues gained in the scheme, which are about tenfold than what water users in the other schemes generate. Water users in the TFIS utilize about 50% less land than MFIS and generate on average 52% of the average farm incomes realized in MRIS. Water users in the schemes also irrigate for relatively similar hours, apart from farmers in NIS who irrigate an average of 11 h per day. Water users in the MRIS have the lowest number of water users that have access to credit (46%) as compared to the other schemes (62, 52, and 72%, for TFIS, MFIS and NIS, respectively). Most of the water-users are co-operative members; however, only 24% of the TFIS farmers are part of cooperatives. In general, most of the sampled water-users feel secure in terms of land tenure, and the schemes are dominated by women, apart from NIS, characterized by only 32.5% of female farmers.
Technical efficiency estimations of water-users in SIS
To test whether the farmers in the schemes belong to the same population, the likelihood ratio test for pooling was conducted through imposing elasticities of land cultivated, fertilizer, and irrigation on other scheme data. The results showed that the MRIS and TFIS belong to two different populations (LR = 19.3, p > 0.05) and as such, two separate production frontiers were estimated. This was further tested by including a dummy variable in the pooled model, which was significant at 5% level, indicating that indeed the two schemes are different. In contrast, the results showed that the NIS was a subset of the MFIS population (LR = 25.2, p < 0.01) and as such, the data was pooled to estimate one frontier for both groups. Henceforth results will be presented for three groups, MRIS, TFIS, and MFIS-NIS.
Table 2 presents the technical efficiency score distribution amongst water-users in KZN. The results indicate that most water-users in the schemes fall in the highest range of efficiency. Only 5% of water-users in TFIS fall in the lowest range of technical efficiency, while MRIS have the highest numbers of water-users in the lowest efficiency range. In general, results indicate a higher percentage of water-users from the TFIS in the highest range of technical efficiency distributions. This is also accompanied by relatively higher percentages of water-users in the lowest efficiency range, for farmers in the MRIS and MFIS-NIS.
Table 3 presents the Technological Gap Ratios, as well as the average and maximum technical efficiency scores in each SIS. Consistent with the technical efficiency distribution results, the average technical efficiency score in the TFIS is around 0.56, which is higher than the average scores in the MFIS-NIS and MRIS, showing that water-users in the former scheme are more technically efficient. The differences in the mean efficiency scores across the schemes indicate differences in conditions in the schemes, which possibly include, institutional arrangements, production orientation and market access, amongst other factors. The TGR indicate the distance between the scheme frontiers and the meta-frontier. The results indicate that water-users in TFIS are not too far off from the meta-frontier. The average water user in TFIS would have to increase output by 33% using the same combination of inputs if they were to achieve the technical efficiency of their most efficient counterpart. Water-users in the MRIS and MFIS-NIS must increase their output by 57 and 45% using the same combination of inputs, respectively, to attain the technical efficiency of the most efficient farmer.
Considering the presence of differences across the schemes, when estimating efficiency across the schemes, it cannot be assumed that the same production functional form is appropriate for all the groups (Makombe et al., 2017). Therefore, the Translog production function is estimated for each of the three groups schemes. The F-statistic is then used to determine the suitability of the Translog, which if not statistically significant, is reduced to Cobb-Douglas form specifications. The results indicate that the Translog model estimated for the TFIS is not statistically significant (p-value = 0.64), showing that the six coefficients on the squared and interaction terms are zero. Therefore the frontier was estimated using the Cobb-Douglas specifications. The Translog function for both the MRIS and MFIS-NIS yielded F-stats that are statistically significant at the 1% level, showing that the interaction and square terms should be included in the models, and as such, were specified in Translog form.
The second test performed was to determine whether the inefficiency effects need to be included in the model. Also presented in Table 4 are gamma (ɤ) values for each of the schemes. The ɤvalues are derived from the SigmaU and SigmaV in the model. If ɤ= 0, the technical inefficiency is not present and therefore, the stochastic frontier model does not need to be estimated. The closer ɤis to unity, the more likely it is that the frontier model is appropriate, and the higher the contribution of the explanatory variables to the inefficiency in the model. A lower gamma value indicates that inefficiencies are mainly caused by factors beyond the control of the water user. The TFIS and MRIS have gamma values close to one, 0.8 and 0.9, respectively. The rejection of the null-hypothesis of the absence of gamma, shows that external shocks affect water-users' inefficiency, and possible errors of measurement.
The stochastic frontier estimated for irrigation schemes yielded maximum likelihood estimates of sigmaU2 that are statistically significant, indicating that the technical inefficiency effects are significant in explaining the variability of total revenue generated in the schemes. Table 4 presents the maximum likelihood results of the frontier models estimated. The elasticities in the meta-frontier model are statistically significant, indicating that an increase in area, labor and fertilizer utilized can significantly increase mean agricultural revenue generated from irrigated crop production. Although the production input estimates are all significant in the meta-model, they differ in the level of significance across the schemes.
In MRIS, only area, and fertilizer utilized are statistically significant. The elasticities suggest that ceteris paribus, a 1% increase in land and fertilizer used increases mean farm revenue by 0.532 and 0.123%, respectively. Although irrigation and fertilizer utilized increase mean farm incomes in TFIS, only the land elasticity of 0.021 is statistically significant, as such it is encouraged that farmer increase their land usage through rental, using communal land or purchasing of land The irrigation hours elasticities are statistically significant in the MFIS-NIS model, indicating irrigation's contribution to the mean incomes in the schemes. The fertilizer elasticity in the NIS model is statistically significant, showing that a 1% increase in fertilizer increases mean revenue by 0.33%. This is as expected, due to the production orientation of farmers in the schemes who produce on a larger scale and use more fertilizer. The interaction and the squared variables in the MRIS are all statistically significant.
Increasing returns to scale are evident in the MRIS for area utilized, irrigation hours, as well as the interaction variable of area and irrigation, and fertilizer and irrigation hours. This is a strong indicator of the importance of irrigation water on farm revenue. It shows that water-users should have water available for irrigation when needed this is consistent with the findings of Sinyolo et al. (2014) who found that water access and security have a positive effect on economic well-being. This is particularly key in MRIS, where, during focus group discussions, farmers mentioned that water access for crop production is challenging, mostly due to users on the upper end of the canal extracting water outside their scheduled irrigation days. Increasing returns to scale are also evident in the MFIS-NIS group for irrigation. In MFIS, farmers must pay a monthly fee for water, which they at times cannot afford. Infrastructure problems such as lack of maintenance of irrigation equipment also prevent water-users from accessing adequate water in the NIS. Therefore, efforts to increase irrigation access and reliability in both schemes should be prioritized.
Technical inefficiency effects
Table 4 also presents technical inefficiency effects. The dependent variable in the model, represented by Lnsig2u, is technical inefficiency; therefore, a negative sign on the coefficient estimate in the model indicates that the variable improves technical efficiency. Overall, most of the resulting parameter estimates make economic sense. The results show that female water-users are more likely to be efficient as compared to their counterparts in the MFIS-NIS group. Apart from most of the farmers being female, most rural women lack resources and therefore would be more judicious with the use of their resources. This is in contrast with the findings of Gwebu and Matthews (2018), who found male farmers more efficient than female ones in smallholder tomato production in South Africa.
Most of the male farmers in the schemes are more business-oriented and usually have better access to markets, compared to the female farmers, who generally produce for subsistence. However, the results suggest that female farmers are more efficient in their production, this is because when resources are scarce, they are most likely to be used efficiently. The finding is consistent with Agyeman et al. (2014), who found a positive effect of female household head on household income diversification. This could also be a result of female farmers being solely dependent on agriculture for their livelihoods while their male counterparts also use their time on other income-generating activities. Therefore, to enhance their efficiency further and to improve the efficiency of the male farmers, empowerment through better access to markets, institutional support, as well as credit access, is needed to improve their efficiency. The results indicate that age has a positive effect on technical inefficiency in the MFIS-NIS model, showing that younger farmers perform better. This finding is consistent with Duhan and Singh (2017) and Gandasari et al. (2021), who found that younger farmers accumulate information from different sources. Younger farmers are more willing to adopt new technologies and find new markets and are therefore more likely to perform better than older farmers. Water users that have access to credit are more efficient in the MRIS, as shown by the statistically significant coefficient estimate. During FDGs, farmers indicated their lack of access to credit, which inhibits them from purchasing production inputs, whose increase would improve mean outputs and consequently, revenue. Farmers also noted that the lack of collateral and ownership of land plays a role in their failure to secure credit. As such, micro-finance institutions should consider terms in which producers who do not own land or have less surety can be provided with necessary financial assistance.
The coefficient estimate of water adequacy shows that water-users across the schemes who perceive that irrigation water is adequate for their cropping requirements tend to be more efficient. The supports the findings of Adetoro et al. (2022) who suggested that adequate water availability has positive effect on the consumption expenditure per capita of farmers. Availability of irrigation water plays a significant role in determining the production approach a farmer adopts. The model results show that farmers that are satisfied with their water supply are most likely to be efficient in their combination of inputs. Cooperative membership improves efficiency in all the schemes; however, the variable is statistically significant in the MRIS. This could be because most of the water users in the TFIS included in the study (76%) are not cooperative members, and although farmers in MFIS-NIS are officially part of co-operatives, they do not operate as such (most farmers are individualistic in their production. The coefficient estimate is particularly statistically significant in the MFIS where most farmers are part of cooperatives. This is consistent with Debebe et al. (2015) and Herbert et al. (2015), who noted that membership of social groups has a positive impact on resource management and performance. This could be attributed to the information sharing that takes place in most agricultural cooperatives. Cooperatives can be efficient institutions to foster development and productivity of farmers (Chagwiza et al., 2016) and as such water-users should be encouraged to be part of social groups, provided the groups are well managed and operated.
Water-users that have secure land tenure tend to be more efficient than those that do not, as shown by the negative parameter estimates. The coefficient estimate is statistically significant at the 5% level in the MRIS. The finding is consistent with Manjunatha et al. (2013), Ebarle et al. (2014), and Lawin and Tamini (2018), who found that land ownership is also positively related to farm performance. Although the farmers do not own the land, the mere feeling of security encourages investment in the land and the confidence to produce with minimal risk of land being confiscated. Therefore, arrangements such as long-term lease agreements and purchasing of the land should be encouraged, as it might have a positive impact on technical efficiency.
The results indicate that farmers that have undergone agricultural training are more efficient than those who have not, consistent with the findings of Urdiales et al. (2016), who found that the higher the hours of agricultural instruction hours, the more efficient the farm is. The variable is statistically significant in all but the MFIS-NIS. Farmers who have received agricultural training have a better understanding of input combination and therefore, are most likely to maximize their revenue. The coefficient is not statistically significant in the MFIS-NIS because even though farmers in the scheme have received training, they emphasized that they require training on how to penetrate the value chain, rather than being trained for agricultural production only. Although farm valued the training on how and when to plant, they indicated that they would also like to get training on marketing, financial management and pricing, all which influence revenue. Picazo-Tadeo et al. (2011) also found no effect of training on farmer's eco-efficiency and suggests that training could be improved through providing a holistic approach. Therefore, agricultural training should be offered to water-users and needs to be broadened to include other aspects such as marketing and pricing.
Psychological capital, which involves an individual's resilience, hope and confidence in themselves, makes economic sense in the MRIS, TFIS and MFIS-NIS models. The results show that water-users with higher psychological capital are likely to be more efficient. The results suggest that farmers that are confident in themselves as business owners, who do not lose hope, are resilient and willing to take risks are more likely to be more efficient (Chipfupa and Edilegnaw, 2018). As such, policy should be focused on developing the entrepreneurial spirit and independence of farmers through business training and extension services. Incentivized programs and sustainable access to markets could also encourage farmers to be more business-oriented and confident in their enterprises.
The results also show that water-users with higher governance index are likely to be more efficient. A higher governance index indicates a better understanding and perception of policies and strategies. They have a more enlightened perception of the governance and management of the scheme and are also satisfied with the involvement of governmental stakeholder involvement in the scheme. Therefore, farmers should be made aware of policies and even the roles of various stakeholders in the scheme, for them to seek the necessary support when needed. It also shows that if farmers understand and are satisfied with how the scheme is governed, they are more likely to perform better.
Conclusions and policy recommendations
Evaluating the factors that influence the performance of water-users is crucial for the development of smallholder irrigation policies and interventions. This is particularly important given the increased reliability of production on irrigation water usage and the contribution of smallholder production to rural food security. Using production economic methods to evaluate performance of water-users, the study concludes that the increase in production outputs, land, fertilizer, and labor, positively impacts agricultural revenue, and thus the performance of an agricultural producer. A combination of socio-economic, institutional, and social factors influences water-users' efficiency in SISs. Therefore, there is a need for a balanced approach when devising ways of improving the performance of SIS. Interventions should consider both technical factors in performance analysis and institutional arrangements that impact the schemes.
Considering that land tenure, credit access, psychological capital, governance index, cooperative membership positively affects water-user efficiency, interventions should be directed to these factors. Better land leasing arrangements, such as long-term lease agreements should be encouraged. This could encourage on-farm investments by farmers, thus improving performance. Improved management and operation of cooperatives would encourage information sharing and make participating farmers improve efficiency. Better terms for low-collateral farmers should be considered in financial institutions, as credit access enhances farmer efficiency. An improvement on better access to markets and water adequacy should be emphasized in policy interventions, as these are key to profitability and productivity in smallholder irrigation schemes. Land tenure security, governance index, water adequacy and cooperative membership are variables which are directly or indirectly affected by institutional arrangements in the schemes.
Data availability statement
The raw data supporting the conclusions of this article will be made available by the authors, without undue reservation.
Ethics statement
The studies involving human participants were reviewed and approved by University of KwaZulu-Natal, Ethics Committee. The patients/participants provided their written informed consent to participate in this study.
Author contributions
LP: conceptualization, writing, data collection, and analysis and write-up. MM: supervision, guidance, revision, conceptualization, guidance on methodology, and revision. SF: supervision and guidance. GM: supervision and revision. All authors contributed to the article and approved the submitted version.
Funding
The study was undertaken as part of a project (K5/2556/4) initiated, managed and funded by the Water Research Commission (WRC) entitled Assessment of Policies and Strategies for the Governance of Smallholder Irrigation Farming in KwaZulu-Natal Province, South Africa.
Conflict of interest
The authors declare that the research was conducted in the absence of any commercial or financial relationships that could be construed as a potential conflict of interest.
Publisher's note
All claims expressed in this article are solely those of the authors and do not necessarily represent those of their affiliated organizations, or those of the publisher, the editors and the reviewers. Any product that may be evaluated in this article, or claim that may be made by its manufacturer, is not guaranteed or endorsed by the publisher.
References
Adetoro, A. A., Ngidi, M. S. C., Danso-Abbeam, G., Ojo, T. O., and Ogundeji, A. A. (2022). Impact of irrigation on welfare and vulnerability to poverty in South African farming households. Sci. Afr. 16, e01177. doi: 10.1016/j.sciaf.2022.e01177
Agyeman, B., Asuming-Brempong, S., and Onumah, E. (2014). Determinants of income diversification of farm households in the Western Region of Ghana. Q. J. Int. Agric. 53, 55–72. doi: 10.22004/ag.econ.195729
Amsler, C., O'Donnell, C. J., and Schmidt, P. (2017). Stochastic metafrontiers. Econom. Rev. 36, 1007–1020. doi: 10.1080/07474938.2017.1308345
Bandaragoda, D. J. (2001). “A framework for institutional analysis for water-resources management in a river-basin context,” in Integrated Water-Resources Management in a River-Basin Context, Vol 5 (Colombo: IWMI).
Battese, G. E., and Rao, D. S. (2002). Technology gap, efficiency, and a stochastic meta-frontier function. Int. J. Bus. Econ. 1, 87–93.
Bernstein, H. (2013). Commercial agriculture in South Africa since 1994: “Natural, simply capitalism”. J. Agrar. Change 13, 23–46. doi: 10.1111/joac.12011
Bezat, A. (2011). Estimation of technical efficiency by application of the SFA method for panel data. Zeszyty Naukowe Szkoły Głównej Gospodarstwa Wiejskiego w Warszawie Problemy Rolnictwa Swiatowego 11, 12–30. doi: 10.22004/ag.econ.195654
Bogetoft, P., and Otto, L. (2010). Benchmarking with DEA, SFA, and R, Vol. 157. New York, NY: Springer. doi: 10.1007/978-1-4419-7961-2
Buckley, C., and Carney, P. (2013). The potential to reduce the risk of diffuse pollution from agriculture while improving economic performance at farm level. Environ. Sci. Policy 25, 118–126. doi: 10.1016/j.envsci.2012.10.002
Chagwiza, C., Muradian, R., and Ruben, R. (2016). Cooperative membership and dairy performance among smallholders in Ethiopia. Food Policy 59, 165–173. doi: 10.1016/j.foodpol.2016.01.008
Chipfupa, U., and Edilegnaw, W. (2018). Farmer typology formulation accounting for psychological capital: implications for on-farm entrepreneurial development. Dev. Pract. 28, 600–614. doi: 10.1080/09614524.2018.1467377
Cillero, M. M., and Reaños, M. T. (2022). Farm technical and environmental efficiency and subsidy redistribution in Ireland: a simulation approach of possible performance and equity effects. J. Agric. Econ. 1–19. doi: 10.1111/1477-9552.12509
Debebe, S., Haji, J., Goshu, D., and Edriss, A. K. (2015). Technical, allocative, and economic efficiency among smallholder maize farmers in Southwestern Ethiopia: parametric approach. J. Dev. Agric. Econ. 7, 282–291. doi: 10.5897/JDAE2015.0652
Dlamini, M. B., and Masuku, M. B. (2013). Profitability of smallholder sugarcane farming in Swaziland: the case of Komati downstream development programme (KDDP) sugar farmers' associations, 2005–2011. Sustain. Agric. Res. 2, 8–14. doi: 10.5539/sar.v2n1p8
Dlangalala, S. F., and Mudhara, M. (2020). Determinants of farmer awareness of water governance across gender dimensions in smallholder irrigation schemes in KwaZulu-Natal Province, South Africa. Water SA 46, 234–241. doi: 10.17159/wsa/2020.v46.i2.8238
Duhan, A., and Singh, S. (2017). Factors affecting awareness level of farmers about crop insurance: A case study of Haryana. Asian J. Agri. Exten. Econom. Sociol. 21, 1–7. Available online at: http://www.journalrepository.org/media/journals/AJAEES_25/2017/Dec/Duhan2142017AJAEES37966.pdf
Ebarle, E. J. N., Sarmiento, J. M. P., Aguinaldo, R. T., Concepcion, S. B., Montiflor, M. O., Real, R. R., et al. (2014). “Analysing the factors affecting the profitability of vegetable farmer clusters in Southern Philippines,” in XXIX International Horticultural Congress on Horticulture: Sustaining Lives, Livelihoods and Landscapes (IHC2014): XVII 1103, (Mindanao: Acta Horticultae), 115–120. doi: 10.17660/ActaHortic.2015.1103.17
Gandasari, D., Sugiarto, M., Dwidienawati, D., Sarwoprasodjo, S., and Tjahjana, D. (2021). The study on the performance of beef cattle farmer groups as an economic institution in Indonesia: based on the communication networks. Stud. Appl. Econ. 39, 4572. doi: 10.25115/eea.v39i4.4572
Ghoshal, P., and Goswami, B. (2017). Cobb-douglas production function for measuring efficiency in Indian agriculture: a region-wise analysis. Econ. Aff. 62, 573–579. doi: 10.5958/0976-4666.2017.00069.9
Gomo, T., Mudhara, M., and Senzanje, A. (2014). Farmerssatisfaction with the performance of the Mooi River irrigation scheme, KwaZulu-Natal, South Africa. Water SA 40, 437–444. doi: 10.4314/wsa.v40i3.6
Gwebu, J. Z., and Matthews, N. (2018). Metafrontier analysis of commercial and smallholder tomato production: a South African case. South Afr. J. Sci. 114, 55–62. doi: 10.17159/sajs.2018/20170258
Herbert, A., Asten, P., Vanlauwe, B., Ouma, E., Blomme, G., Birachi, E., et al. (2015). “Improving the adoption of agricultural technologies and farm performance through farmer groups: evidence from the Great Lakes Region of Africa,” in Proceedings of the 2015 Conference, August 9–14, 2015, Milan, Italy (No. 210939). Milan: International Association of Agricultural Economists.
Huang, C. J., Huang, T. H., and Liu, N. H. (2014). A new approach to estimating the metafrontier production function based on a stochastic frontier framework. J. Prod. Anal. 42, 241–254. doi: 10.1007/s11123-014-0402-2
Kostlivý, V., Fuksová, Z., and Rudinskaya, T. (2020). Drivers of farm performance in Czech crop farms. Agric. Econ. 66, 297–306. doi: 10.17221/231/2019-AGRICECON
Lawin, K. G., and Tamini, L. D. (2018). Tenure security and farm efficiency analysis correcting for biases from observed and unobserved variables: evidence from Benin. J. Agric. Econ. 70, 116–134. doi: 10.1111/1477-9552.12275
Makombe, G., Namara, R. E., Awulachew, S. B., Hagos, F., Ayana, M., and Kanjere, M. (2017). An analysis of the productivity and technical efficiency of smallholder irrigation in Ethiopia. Water SA 43, 48–57. doi: 10.4314/wsa.v43i1.08
Maniriho, A., Musabanganji, E., and Lebailly, P. (2021). Factors affecting farm performance among small-scale farmers in volcanic highlands in Rwanda: What is the role of Institutions? Asian J. Agri. Rural Develop. 11, 262–268. doi: 10.18488/journal.ajard.2021.114.262.268
Manjunatha, A. V., Anik, A. R., Speelman, S., and Nuppenau, E. A. (2013). Impact of land fragmentation, farm size, land ownership and crop diversity on profit and efficiency of irrigated farms in India. Land Use Policy 31, 397–405. doi: 10.1016/j.landusepol.2012.08.005
Melo-Becerra, L. A., and Orozco-Gallo, A. J. (2017). Technical efficiency for Colombian small crop and livestock farmers: a stochastic metafrontier approach for different production systems. J. Prod. Anal. 47, 1–16. doi: 10.1007/s11123-016-0487-x
Morais, G. A. S., Silva, F. F., Freitas, C. O. D., and Braga, M. J. (2021). Irrigation, technical efficiency, and farm size: the case of Brazil. Sustainability 13, 1132. doi: 10.3390/su13031132
Muchara, B., Ortmann, G., Wale, E., and Mudhara, M. (2014). Collective action and participation in irrigation water management: a case study of Mooi River irrigation scheme in KwaZulu-Natal Province, South Africa. Water SA 40, 699–708. doi: 10.4314/wsa.v40i4.15
Mugejo, K., Ncube, B., and Mutsvangwa, C. (2022). Infrastructure performance and irrigation water governance in genadendal, Western Cape, South Africa. Sustainability 14, 12174. doi: 10.3390/su141912174
Muller, M. (2012). Polycentric governance: water management in South Africa. Proc. Inst. Civil Eng. Manag. Procure. Law 165, 1–8. doi: 10.1680/mpal.11.00018
Mumba, C., Samui, K. L., Pandey, G. S., and Tembo, G. (2012). Econometric analysis of the socio-economic factors affecting the profitability of smallholder dairy farming in Zambia. Livestock Res. Rural Dev. 24, 66–84.
Mushi, A. S., and Ngaruko, D. D. (2015). Determinants of financial sustainability of small holder sugarcane farming systems in Tanzania. Afr. J. Econ. Rev. 3, 57–73. doi: 10.22004/ag.econ.264377
Mwadzingeni, L., Mugandani, R., and Mafongoya, P. (2020). Localized institutional actors and smallholder irrigation scheme performance in Limpopo province of South Africa. Agriculture 10, 418. doi: 10.3390/agriculture10090418
Njuki, E., and Bravo-Ureta, B. E. (2018). Irrigation water use and technical efficiencies: accounting for technological and environmental heterogeneity in US agriculture using random parameters. Water Resour. Econ. 24, 1–12. doi: 10.1016/j.wre.2018.02.004
O'Donnell, C. J., Rao, D. S., and Battese, G. E. (2008). Meta-frontier frameworks for the study of firm-level efficiencies and technology ratios. Empir. Econ. 34, 231–255. doi: 10.1007/s00181-007-0119-4
Ojo, O. (2006). An examination of technical, economic, and allocative efficiency of small farms: the case study of cassava farmers in Osun State of Nigeria. J. Central Eur. Agric. 7, 423–432. doi: 10.5513/jcea.v7i3.392
Oulmane, A., and Benmehala, A. M. (2019). Effects of irrigation technology adoption on farms performance in Algeria: a stochastic frontier production function approach. Rev. Agric. Appl. Econ. 22, 81–87. doi: 10.15414/raae.2019.22.02.81-87
Picazo-Tadeo, A. J., Gómez-Limón, J. A., and Reig-Martínez, E. (2011). Assessing farming eco-efficiency: a data envelopment analysis approach. J. Environ. Manag. 92, 1154–1164. doi: 10.1016/j.jenvman.2010.11.025
Rios, A. R., and Shively, G. E. (2016). Farm size and nonparametric efficiency measurements for coffee farms in Vietnam. Sustainable agriculture and natural resource management (SANREM) knowledgebase. Selected Paper prepared for presentation at the American Agricultural Economics Association Annual Meeting, Providence, Rhode Island, July 24–27, 2005.
Sinyolo, S., Mudhara, M., and Wale, E. (2014). The impact of smallholder irrigation on household welfare: the case of Tugela Ferry irrigation scheme in KwaZulu-Natal, South Africa. Water SA 40, 145–156. doi: 10.4314/wsa.v40i1.18
Speelman, S., D'Haese, M., Buysse, J., and D'Haese, L. (2008). A measure for the efficiency of water use and its determinants, a case study of small-scale irrigation schemes in North-West Province, South Africa. Agric. Syst. 98, 31–39. doi: 10.1016/j.agsy.2008.03.006
Sujan, M. H. K., Islam, F., Azad, M. J., and Rayhan, S. J. (2017). Financial profitability and resource use efficiency of boro rice cultivation in some selected area of Bangladesh. Afr. J. Agric. Res. 12, 2404–2411. doi: 10.5897/AJAR2017.12443
Tajbakhsh, A., and Hassini, E. (2015). A data envelopment analysis approach to evaluate sustainability in supply chain networks. J. Clean. Prod. 105, 74–85. doi: 10.1016/j.jclepro.2014.07.054
Urdiales, M., Lansink, A. O., and Wall, A. (2016). Eco-efficiency among dairy farmers: the importance of socio-economic characteristics and farmer attitudes. Environ. Resour. Econ. 64, 559–574. doi: 10.1007/s10640-015-9885-1
Van Averbeke, W. (2008). Best Management Practices for Small-Scale Subsistence Farming on Selected Irrigation Schemes and Surrounding Areas Through Participatory Adaptive Research in Limpopo Province, WRC Report No TT 344/08.
Van Averbeke, W. (2012). “Performance of smallholder irrigation schemes in the Vhembe District of South Africa,” in Problems, Perspectives and Challenges of Agricultural Water Management, ed M. Kumar (Rijeka: Intech). doi: 10.5772/29182
Van Averbeke, W., Denison, J., and Mnkeni, P. N. S. (2011). Smallholder irrigation schemes in South Africa: a review of knowledge generated by the Water Research Commission. Water SA 37, 797–808. doi: 10.4314/wsa.v37i5.17
Verhofstadt, E., and Maertens, M. (2014). Smallholder cooperatives and agricultural performance in Rwanda: do organizational differences matter? Agric. Econ. 45, 39–52. doi: 10.1111/agec.12128
Keywords: smallholder irrigation scheme, stochastic meta-frontier analysis, technical efficiency, KwaZulu-Natal, governance index
Citation: Phali L, Mudhara M, Ferrer S and Makombe G (2022) Evaluation of water-user performance in smallholder irrigation schemes in KwaZulu-Natal Province, South Africa: A stochastic meta-frontier analysis. Front. Sustain. Food Syst. 6:1022410. doi: 10.3389/fsufs.2022.1022410
Received: 18 August 2022; Accepted: 10 November 2022;
Published: 01 December 2022.
Edited by:
Yanjun Shen, University of Chinese Academy of Sciences, ChinaReviewed by:
Justice Gameli Djokoto, Central University, GhanaJintao Wang, Institute of Genetics and Developmental Biology (CAS), China
Copyright © 2022 Phali, Mudhara, Ferrer and Makombe. This is an open-access article distributed under the terms of the Creative Commons Attribution License (CC BY). The use, distribution or reproduction in other forums is permitted, provided the original author(s) and the copyright owner(s) are credited and that the original publication in this journal is cited, in accordance with accepted academic practice. No use, distribution or reproduction is permitted which does not comply with these terms.
*Correspondence: Lerato Phali, lerato.phali@up.ac.za; Maxwell Mudhara, mudhara@ukzn.ac.za