The carbon footprint of livestock farms under conventional management and silvopastoral systems in Jalisco, Chiapas, and Campeche (Mexico)
- 1Líder Grupo de Investigación PROECUT, Facultad de Ingeniería Agronómica, Universidad del Tolima, Ibagué, Colombia
- 2Livestock and Environmental Management Unit, The Tropical Agricultural Research and Higher Education Center (CATIE), Turrialba, Costa Rica
- 3Forest and Biodiversity in Productive Landscapes, The Tropical Agricultural Research and Higher Education Center (CATIE), Turrialba, Costa Rica
Livestock production is one of the most important agricultural sources of greenhouses gasses (GHG). Transforming the livestock sector is a critical task to mitigate the effects of climate change, and the implementation of silvopastoral systems (SPS) may be a way to do so. Carbon footprint (CF) estimation was carried out for 131 conventional livestock farms (CONF) and SPS farms (SPSF) selected in the states of Jalisco, Chiapas and Campeche (Mexico). GHG emissions were estimated based on interviews with the farmers and farm monitoring to collect information about management, milk production and the live weight gain (LWG) of calves (for meat production) using national emission factors. Emissions from animals, fossil fuels, electricity and nitrogen fertilizers were estimated using Tier I and II criteria. Land use distribution was recorded for each farm, and their carbon fixation in total biomass and soils was estimated using studies from tropical Latin America. CF was estimated for area and for production of milk and the LWG of calves. SPSF had lower GHG emissions, higher carbon fixation rates and a better CF than CONF (5.7 vs. 8.0 t CO2e/ha/year; 6.9 vs. 5.5 t C/ha/year and − 5.0 vs. −2.9 t CO2e/ha/year, respectively). The CF of milk production and calf LWG were − 68.6 to −4.6 kg CO2e/kg and − 3.2 to −0.1 t CO2e/kg, respectively. Our results highlight the importance of implementing SPS to mitigate climate change in livestock production, given its contribution to GHG emissions and the importance of capturing atmospheric carbon in biomass and soils. National and regional policies should focus on promoting implementing SPS as an active and effective strategy for improving CF in livestock farms.
1 Introduction
Livestock activity contributes between 12 and 14.5% of anthropogenic greenhouse gas (GHG) emissions, with milk and meat production responsible for 3–4% (Velarde-Guillén et al., 2022) and 11–12%, respectively (Grain, 2017). Methane (CH4) is the major contributor to these emissions, but with a wide range, from 34 to 86% (Gerber et al., 2013; Rice et al., 2017; Mazzetto et al., 2020; González-Quintero et al., 2021; Hawkins et al., 2021), with some researchers finding rates of more than 70%. Mexico is the 12th biggest emitter of CO2 in the world (International Energy Agency [IEA], 2020) and accounts for 1% of global GHG emissions (He et al., 2021). The agricultural sector in Mexico emitted 92 Tg CO2e to which livestock contributed close to 50% (Prospero-Bernal et al., 2019). Mexico has proposed a reduction of 22% by 2030, with a target of 8% for the livestock sector (Instituto Nacional de ecología y Cambio Climático [INECC], 2018). The most recent Instituto Nacional de ecología y Cambio Climático [INECC], (2018) estimated 683 Tg CO2e the emissions to which livestock contributed 10.3%; this makes livestock production the second most important contributor to GHG emissions after the energy sector (70% of the national total). In the livestock sector, enteric fermentation is the main source of emissions (76%), followed by manure management (24%) (Instituto Nacional de ecología y Cambio Climático [INECC], 2018).
Worldwide, the demand for livestock production, as well as its derivatives such as milk and cheese, has increased in recent decades as a result of increases in population and consumption preferences (Ghosh et al., 2020; Navarrete, 2020). In 1950, Mexico had a population of 25.8 million people; 70 years later, Mexico’s population has almost quintupled (126 million). It is estimated that, by 2050, Mexico’s population will reach 148.2 million (de México, 2018). Cattle ranching is the most widespread productive activity in rural areas of Mexico and is carried out in all agroecological regions on about 110 million hectares (60% of the territory), with about 28.4 million head of cattle. Production ranges from highly innovative and integrated systems, to more traditional approaches (Pagiola et al., 2004; Instituto Nacional de Estadística y Geografía [INEGI], 2016). Traditional cattle farms are based on extensive grazing with or without supplementation and practices to improve livestock management, without considering the effect on environment. More technologically oriented systems usually use silvopastoral systems (SPS), take records and apply good farming practices.
Carbon footprint (CF) estimation has become necessary for livestock farming and management strategies in light of climate change (Molina-Rivera et al., 2019; Ali et al., 2020; Elshimy and El-Aasar, 2020; Ghosh et al., 2020; Secretaría de Medio Ambiente y Recursos Naturales [SEMARNAT], 2020). Numerous strategies have been applied to mitigate the emissions caused by livestock production, such as carbon capture and conservation in tree-dominated land uses such as forests and SPS (Andrade et al., 2008; Molina-Rivera et al., 2019). Improving a farm’s CF can also occur by improving animal feeding through the use of a higher quality diet and improved genetics, having more productive animals (Molina-Rivera et al., 2019; Prospero-Bernal et al., 2019). Establishing SPS on conventional open pastures increases the capture of carbon in biomass and soils, as well as reducing deforestation and GHG emissions (Andrade et al., 2008; Aryal et al., 2022; Oldfield et al., 2022; Udawatta et al., 2022). Several studies have confirmed the positive effect of SPS in reducing the CF of livestock farms (Schettini et al., 2021; Brook et al., 2022; Parra et al., 2022).
The project “Promoting biodiversity conservation through climate-smart agrosilvopastoral practices in livestock-dominated landscapes in three regions of Mexico” (Biodiversidad y Paisajes Ganaderos Agrosilvopastoriles Sostenibles; i.e. BioPaSOS started in 2017) in the states of Jalisco, Chiapas and Campeche (Mexico); one of the main purposes of this project was to help reduce GHG emissions by promoting the implementation of SPS across livestock farms. The goal of the present work is to estimate the CF of livestock farms with conventional systems (CONF) and those using SPS (SPSF) in these three states and to identify the most important components of their CF. The results will provide a guideline for the formulation of policies and strategies for technology transfer and adoption to make livestock systems more environmentally friendly to mitigate climate change.
2 Methods
2.1 Study area
This study was carried out in the states of Jalisco, Chiapas and Campeche in Mexico. The main characteristics (i.e., biophysical conditions and management) of the three states are described in Aryal et al. (2022). The states included in the research were selected – with the support of the national, state and local Secretary of Livestock and Environment – according to the following criteria: (1) deforestation problems, (2) areas with pastures for livestock, (3) problems with forest fires and erosion and (4) priority areas for conservation.
Jalisco is located in the temperate region at 20°34′00″N and 103°40′35″W (Bautista et al., 2020), where forests, grasslands, shrublands and agriculture can be found in a transition zone between the Nearctic and Neotropical regions (Figure 1). The average annual rainfall is 862 mm, with an average of 11.19 days of frost per year. The average temperature in the dry season is 30°C and 12°C in the rainy season. These factors are key to the development of productive activities, especially cattle (de Jalisco, 2011). The total cattle population of the state is 3,409,172 heads (71 and 29% for meat and milk production, respectively) (OEIDRUS-Jalisco, 2017). Livestock is the main source of income for most families, and there is a mean animal stocking rate of 0.76 AU/ha foraging grasses, shrubs and trees (Sánchez-Romero et al., 2022). In 2015, Jalisco produced 2.2 million L of milk a day and 216,535 t of meat each year (OEIDRUS-Jalisco, 2017).
Chiapas is located in the intertropical zone (16°24′36″N and 92°24′31″W) with a humid tropical climate (Bautista et al., 2020) (Figure 1). The annual rainfall in coastal areas reaches 4,000 mm, while in central areas, the rainfall is less than 1,000 mm; the average altitude is 320 m. In some places, the average temperature is above 23°C and in other places the temperature falls below 15°C. Chiapas has 12,025 km2 of primary forests, 16,643 km2 of cropland and 19,518 km2 of cultivated or induced pastures dedicated to livestock, which have increased in area at an average rate of 345.7 km2 each year (Gobierno del Estado de Chiapas, 2019). Chiapas has 2.7 million head of cattle and is the third biggest producer of meat in Mexico (217,395 t/year) (Servicio de Información Agroalimentaria y Pesquera [SIAP], 2016), mainly in dual purpose systems (Martínez-García et al., 2012).
Campeche is located in the tropical region (18°50′11″N and 90°24′12″0) (Bautista et al., 2020) (Figure 1). The state covers 57,924 km2 with 11 municipalities that represent 3% of the country. It is primarily made up of sub-horizontal plains (41%) and hilly areas (35%). The average annual temperature is approximately 26°C, with a mean annual rainfall of 1,272 mm. The area is dominated by secondary vegetation (54%), forests (16%) and grasslands (13%), as well as, to a lesser extent, by agriculture, forest, water bodies, areas without vegetation and urban areas (Biodiversidad y Paisajes Ganaderos Agrosilvopastoriles Sostenibles [BioPaSOS]. (s.f.), 2017). The livestock sector covers 58% of agricultural production in Campeche (Gobierno del Estado de Campeche, 2015), with a total of 650,000 head of cattle (Servicio de Información Agroalimentaria y Pesquera [SIAP], 2016).
2.2 Farm selection
The sample included 131 livestock farms. In each state, a meeting was held with producers interested in participating in the BioPaSOS project, from which a list was drawn up and approved by the local authorities of each Ejido.
Farms were classified either as conventional (CONF) or SPS farms (SPSF). CONF practice extensive grazing, with or without supplementation, and implement practices or technologies to improve livestock productivity only, without considering the effect on the environment. SPSF implement SPS production systems, including dispersed trees in native or improved pastures, fodder banks and multi-stratum live fences. These farms also engage in good livestock practices, such as pasture rotation, management of feed records, vaccination, deworming and production, as well as rational use of veterinary products and supplemental feeding with silage and multi-nutritional blocks. Farmers with SPSF were selected to participate in the BioPaSOS project based on the following criteria: (1) be an initiated sustainable livestock producer, (2) innovative (creative), (3) recognized in the community, (4) participatory, (5) interested in learning, (6) availability of time and (7) willingness to experiment (participatory research). Farmers with CONF were randomly selected from among those that were not participating in the BioPaSOS project.
Out of the total of 131 farms, 70 were visited only once, 40 in Chiapas and 30 in Campeche. The remaining farms were visited once a month for 12 consecutive months (11, 40 and 10 in Jalisco, Chiapas and Campeche, respectively). A semi-structured interview was applied to those farms that were visited once. Records of information, such as milk production and live weight per cow, by weighing milk and animals with scales, were carried out for two farms per state for two days during each monitoring period.
2.3 Variables measured
Data were collected on the most relevant variables for estimating GHG emissions, such as geographic area (applied geographic scope, climate and soil), land use distribution, milk production and quality, cattle characteristics (breed and number and weight of animals), stocking rate, pastures and supplements used, as well as their nutritional characteristics (digestibility, crude protein, neutral detergent fiber and metabolizable energy of supplements) and dry matter consumption per animal. We also inquired about production costs, electrical energy used on the farm, use of nitrogen fertilizers, use of fossil fuels and firewood and manure applied in the different land uses, fuel used on and off the farm and percentage of digestibility intake. Ym, which quantifies enteric CH4 emissions based on gross energy intake, and country-specific emission factors were used for these estimations.
2.4 GHG emissions analysis
The data collected were organized and analyzed to estimate the total emissions generated by identified production systems and farms (t CO2e/ha/year and t CO2e/farm/year) and on a unit basis per animal unit (t CO2e/UA/year) or per product unit (kg CO2e/kg milk and t CO2e/kg meat of calves sold). GHG emissions were estimated with a local calculation tool (GEI-CATIE Tool) (Vega, 2016) at an animal scale; this is a tool generated by CATIE researchers in a spreadsheet for making GHG estimates in livestock systems. It contemplates Tiers I and II, according to the availability of activity data for the region studied and following the Intergovernmental Panel on Climate Change [IPCC] (2006) guidelines. Tier I is a basic method for estimating GHG emissions, using default emission factors and simple national activity data. It is generally less precise and is used when detailed country-specific data are unavailable. Tier II involves more detailed approaches for estimating emissions, utilizing country-or region-specific emissions factors and more comprehensive activity data. It provides more accurate estimates than Tier I and is applied when more detailed data are available.
Emissions were considered in two phases. Phase 1, on-farm emissions, includes data from enteric fermentation, manure management, use of nitrogen fertilizers, use of fossil fuels and electricity consumption. Phase 2, off-farm emissions, includes data from use of fossil fuels in the purchase of inputs, transportation of labor and marketing of products and emissions from production processes in the manufacture of inputs (gas).
The following emissions factors were used: 0.01 kg N₂O/kg N (Intergovernmental Panel on Climate Change [IPCC], 2006) for nitrogen fertilization; 2.596 and 2.322 kg CO₂e/l for diesel and gasoline, respectively (Instituto Nacional de ecología y Cambio Climático [INECC], 2014; Secretaría de Medio Ambiente y Recursos Naturales [SEMARNAT], 2021a); and 0.494 t CO₂e/MWh for electricity (Secretaría de Medio Ambiente y Recursos Naturales [SEMARNAT], 2021b). These calculations were developed following Eq. (1).
Where:
GHG emissions: t CO2e/year/farm.
Activity data: Number of animals/ha or quantity of inputs (e.g., kWh/month/farm).
Emissions factor: GHG emissions by unit of animal or input (e.g. g CO2e/kWh of electricity).
2.5 Estimation of carbon sequestration in total biomass and soils
Information about land use distribution in each livestock farm was collected via surveys and monitoring visits. Specifically, data about area and characteristics of land uses were acquired from farmers. A literature review was also conducted to determine the carbon fixation rates of the biomass and soils of land uses present across selected farms (Table 1). Based on this information, the total carbon fixation rate for each farm was estimated (Eq. 2).
Where:
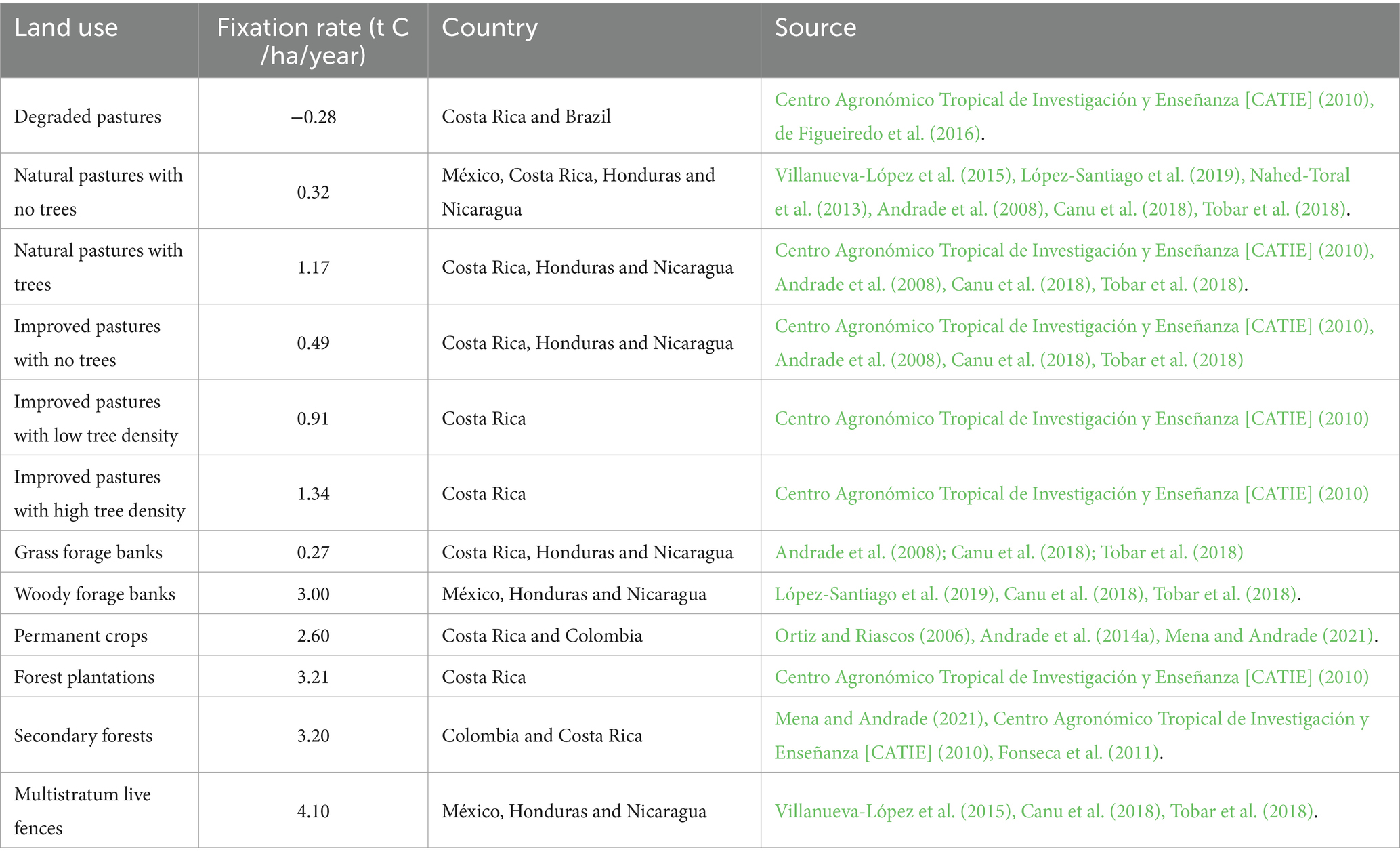
Table 1. Carbon fixation rates in total biomass and soils of land use systems estimated in some Latin-American countries in the tropics.
CC: Carbon capture per farm (t CO2e/farm/year).
CCRi: Carbon capture rate by land use (t CO2e/ha/year).
Ai: Area of each land use (ha).
2.6 Carbon footprint of farms
The CF was estimated as the difference between the carbon fixation rate in biomass and soils and the GHG emissions of each farm (Eq. 3). Likewise, the CF per unit of animal, milk production and calf LWG was estimated dividing CF by the animal stocking rate, milk production and calf LWG (Eq. 4). Information about milk production and meat sales was gathered from farmers. Total CF in dual purpose farms was divided by milk production and calf LWG employing the allocation factor recommended by Thoma et al. (2013) (Eq. 5). The GHG equivalency factors, recommended by Intergovernmental Panel on Climate Change [IPCC] (2013), were used: 1 kg CH4 = 28 kg CO2e and 1 kg N2O = 265 kg CO2e.
Where:
CF: Carbon footprint per farm (t CO2e/farm/year).
GHGe: Greenhouse gas emissions per farm (t CO2e/farm/year).
CC: Carbon capture per farm (t CO2e/farm/year)
Where:
CFu: Unitary carbon footprint (e.g., t CO2e/UA/year or kg CO2e/kg of milk).
CF: Carbon footprint per farm (t CO2e/farm/year).
Quantity: Quantity of animals, milk production or calf LWG (e.g., UA/farm or kg milk/year/farm)
Where:
AFmilk: Allocation factor for milk production.
BMR: Ratio of production of milk corrected/live weight of animals sold.
2.7 Statistical analysis
The data were analyzed with descriptive statistics and scatter graphs to determine the relationships between GHG emissions, pasture area and total number of animals. Variance analyses were carried out to determine the behavior of GHG emissions and the CF for CONF and SPSF. The analyses were done using the software program Infostat, version 2019 (Di Rienzo et al., 2019).
2.8 Limits of the work
Emissions estimates were calculated following Tier I and Tier II criteria (Intergovernmental Panel on Climate Change [IPCC], 2006) and using emissions factors specifically developed for Mexico (Instituto Nacional de ecología y Cambio Climático [INECC], 2014; Secretaría de Medio Ambiente y Recursos Naturales [SEMARNAT], 2021a). Emissions from the use of nitrogen fertilizers, fossil fuels, electric energy and N2O emissions from solid and liquid waste management were estimated with Tier I. Emissions of CH4 from the use of fossil fuels were estimated with Tier II. CH4 emissions from enteric fermentation by livestock category and waste management from solid and liquid waste management were estimated with Tier II. The CF was estimated for the livestock production of farms, including fossil fuels for inputs, as well as labor and product transportation.
3 Results
3.1 GHG emissions
Increasing the forage area reduced GHG emissions (Figure 2A), while increasing the number of animals resulted in a quadratic trend in emissions – that is, at a medium number of animals, emissions were maximized (Figure 2B). These trends did not seem to be affected by the type of production (CONF or SPSF) or by state (Jalisco, Chiapas or Campeche). Some atypical cases of GHG emissions were, however, observed for some farms that have management strategies differing from the traditional ones.
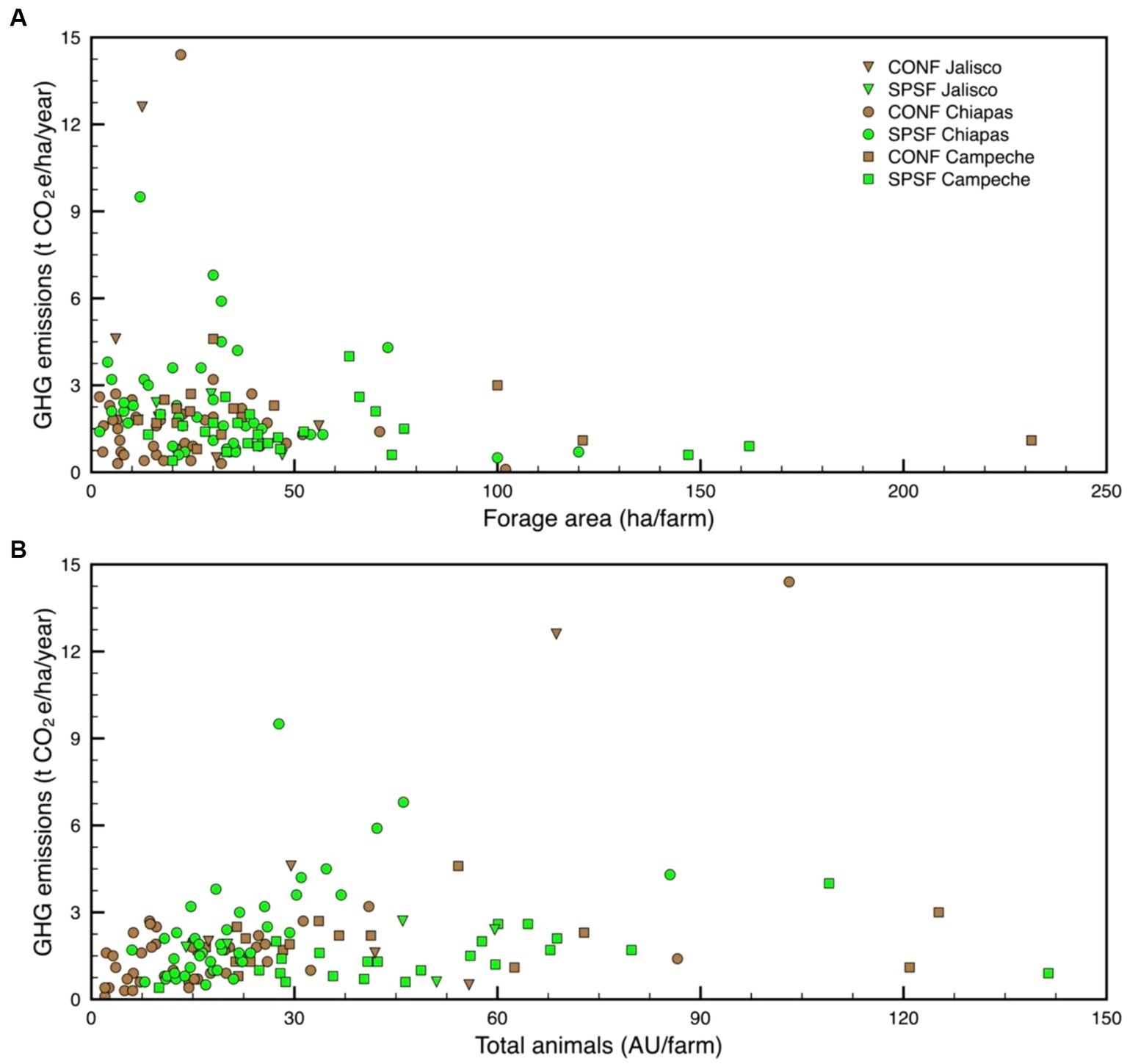
Figure 2. Relationship between greenhouse gas (GHG) emissions and forage area (A) and total number of animals (B) in livestock farms with conventional (CONF) and silvopastoral systems (SPSF) in Jalisco, Chiapas and Campeche (Mexico).
CONF emitted a mean of 8.0 t CO2e/ha/year, with higher values than those found for SPSF (5.7 t CO2e/ha/year); use of SPS thus corresponded to a 16.5% reduction. However, the results differed by state. In Campeche and Jalisco, emissions were higher for CONF than for SPSF (2.0 ± 0.2 vs. 1.5 ± 0.2 and 4.3 ± 2.2 vs. 1.9 ± 0.4 t CO2e/ha/year, respectively), but SPSF in Chiapas emitted more GHG than did CONF in that state (2.4 ± 0.3 vs. 1.7 ± 0.3 t CO2e/ha/year, respectively) (Figure 3A). However, the differences in GHG emissions between states and between management types were not significant (p > 0.05). In Chiapas, emissions from animals, fertilizers and fuels were up to 70% higher in SPSF than in CONF.
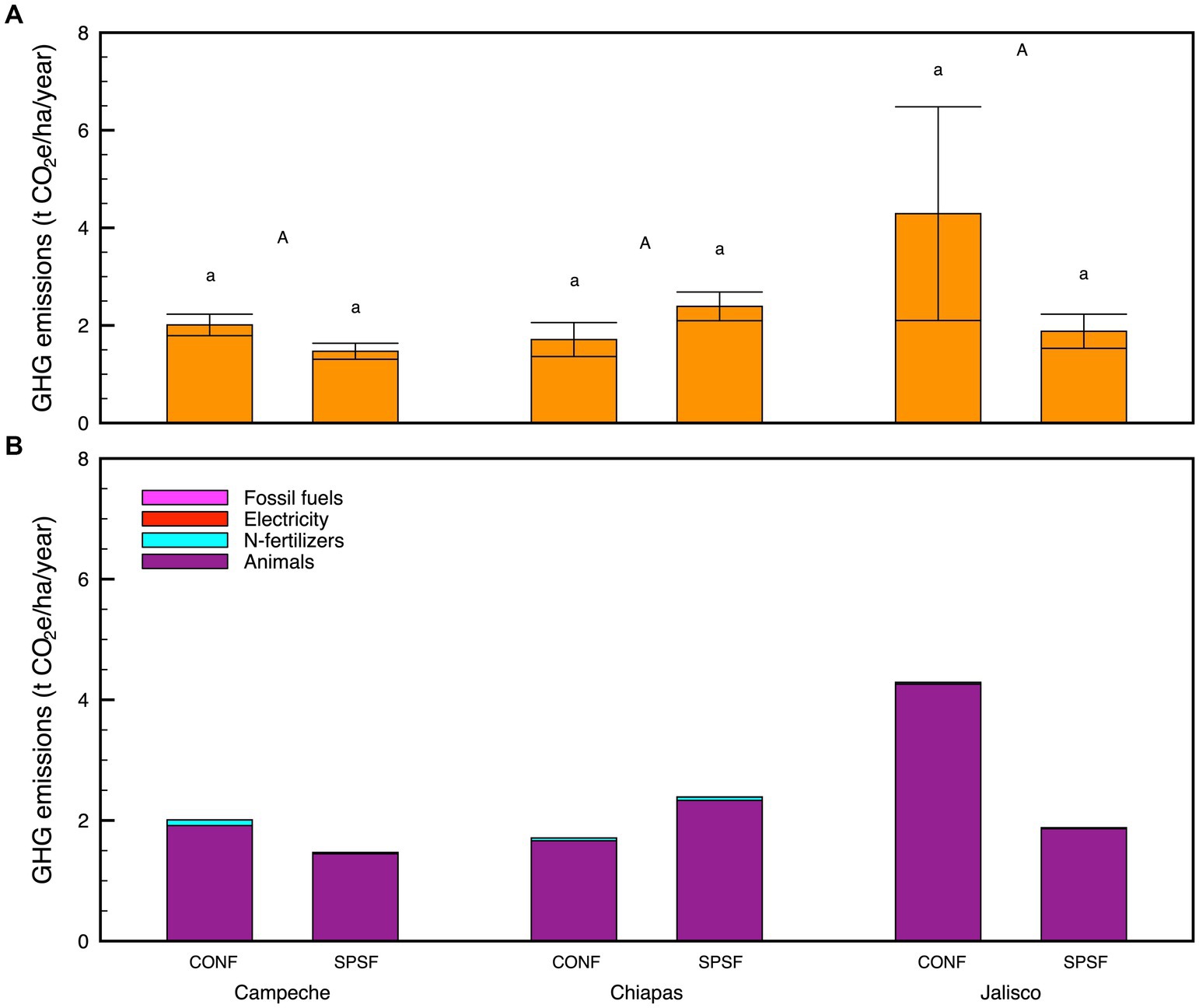
Figure 3. Greenhouse gas (GHG) emissions (A) and their distribution by components (B) in livestock conventional farms (CONF) and silvopastoral production farms (SPSF) in Campeche, Chiapas and Jalisco (Mexico). Error bars indicate standard error of the mean. Different lowercase letters indicate statistical differences (p < 0.05) between system types by each state. Different capital letters indicate statistical differences (p < 0.05) between states.
Animals contributed 98.0% of total GHG emissions, compared to nitrogen fertilizers, electricity and fossil fuels (1.5, 0.3 and 0.1%, respectively). Nitrogen fertilizers emitted twice as much GHG in CONF than in SPSF; that is, the use of tree production systems contributes to reducing emissions and costs by about 50%. Likewise, animals, fuel use and electrical energy emitted 1.4, 1.3 and 1.2 times more in CONF than in SPSF (Figure 3B). The average emissions from animals in SPSF in Campeche were 1.4 t CO2e/ha/year, followed by 1.9 and 2.3 t CO2e/ha/year in Jalisco and Chiapas, respectively. In contrast, animals in CONF in Chiapas had the lowest emissions (1.7 t CO2e/ha/year) compared to Campeche and Jalisco, which emitted 2.0 and 4.3 t CO2e/ha/year, respectively (Figure 3B).
3.2 Carbon fixation rates
Degraded pastures in conventionally managed farms have a negative fixation rate of 0.7 t C/farm/year – that is, they have GHG emissions of about 2.6 t CO2e/farm/year (Figure 4). This is the only land use system emitting GHGs on these farms – that is, it is contributing to increasing atmospheric CO2 concentrations. The average carbon fixation in CONF was 115.5 t C/farm/year, which contrasts with the 87.5 t C/farm/year for SPSF (5.5 vs. 6.9 t C/ha/year, respectively); SPS are thus contributing to the reduction of emissions by 32% at the farm level and 24% per ha.
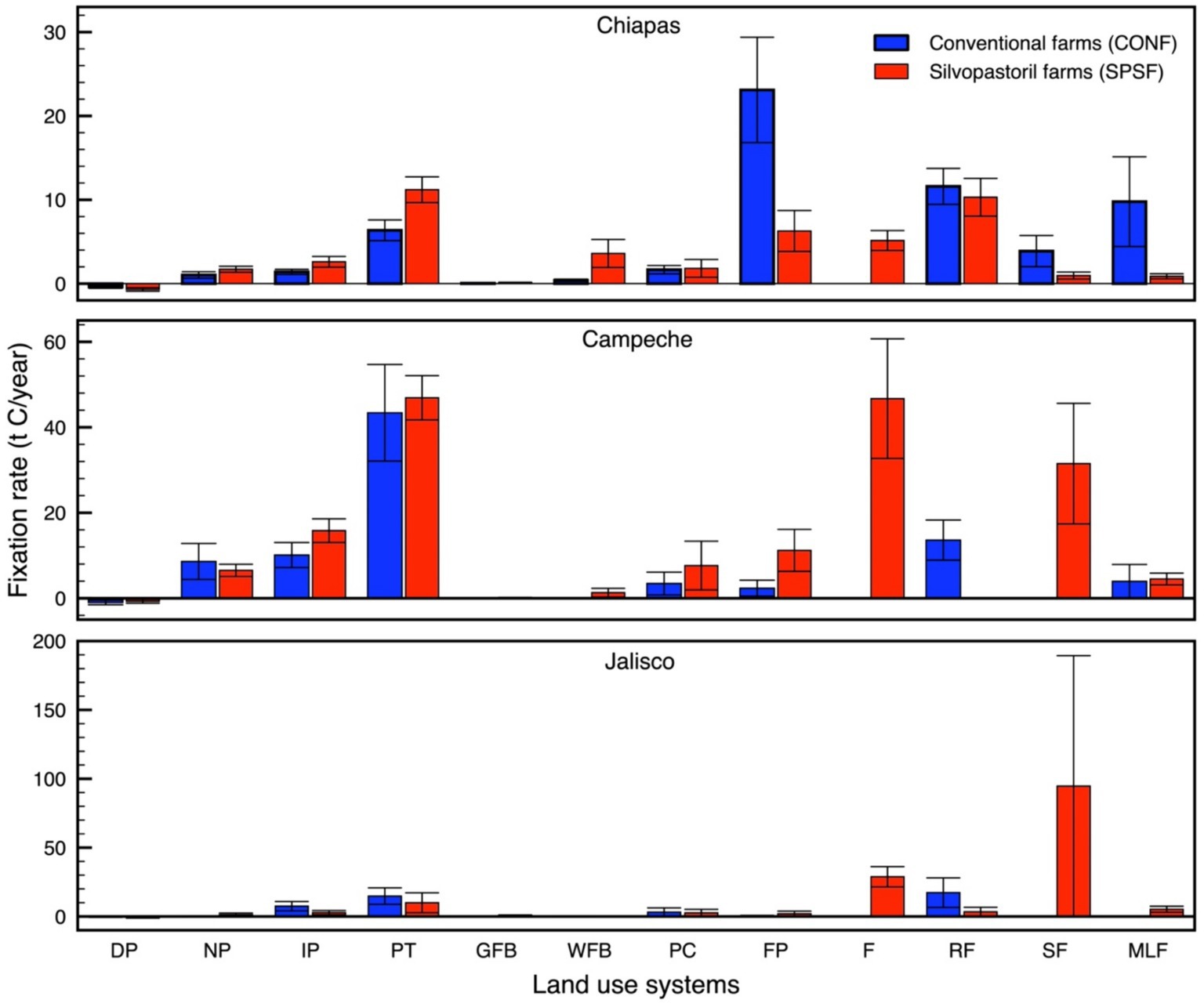
Figure 4. Carbon sequestration rate in total biomass and soils by type of land use system in livestock farms with conventional (CONF) and silvopastoral systems (SPSF) in Campeche, Chiapas and Jalisco (Mexico). Error bars indicate standard error of the mean. DP: degraded pastures; NP: native pastures; IP: improved pastures; PT: pastures with trees; GFB: grass fodder banks; WFB: woody fodder banks; PC: perennial crops; FP: forest plantations; F: fallows (natural regeneration); RF: riparian forests; SF: secondary forests; MLF: multistrata live fences.
The highest carbon fixation rates in CONF were found in forest plantations (FP), fallows (F) and pastures with trees (PT), with an average of 8.6, 14.2 and 21.5 t C/farm/year, respectively. In contrast, grass fodder banks (GFB), woody fodder banks (WFB) and riparian forests (RF) fix just 0.1, 0.2 and 1.3 t C/farm/year, respectively. For SPSF, the highest carbon fixation rates were achieved in PT, F and secondary forests (SF), with 22.7, 26.9 and 42.4 t C/farm/year, respectively (Figure 4). In Campeche, the average fixation rate was 87.5 vs. 171.5 t C/farm/year in CONF and SPSF, respectively. The same trends appeared in Jalisco (45.3 vs. 150.5 t C/farm/year for these systems), in contrast to the state of Chiapas, which reported just 60.0 and 43.9 t C/farm/year for CONF and SPSF, respectively (Figure 4). These values depended greatly on the land uses and the area of each.
3.3 Carbon footprint
Emissions in CONF were higher in Jalisco, with 4.3 ± 2.2 t CO2e/ha/year, which contrasted with the higher emissions recorded in SPSF in Chiapas (2.4 ± 0.3 t CO2e/ha/year). The highest carbon fixation in CONF was in the state of Chiapas, with 7.0 ± 0.4 t CO2e/ha/year; for SPSF, the highest carbon fixation rate appeared in Jalisco, with 7.4 ± 1.6 t CO2e/ha/year (Figure 5). The CF of the livestock sector was negative in the systems and states evaluated, with average values between −3.8 and − 5.6 t CO2e/ha/year, with no statistical differences (p > 0.05) between production types or between states. The exception to this general trend was the CONF in Jalisco, where the CF was 0.4 and 5.5 t CO2e/ha/year (Figure 5), with the CONF being just a net emitter of GHG (0.4 t CO2e/ha/year).
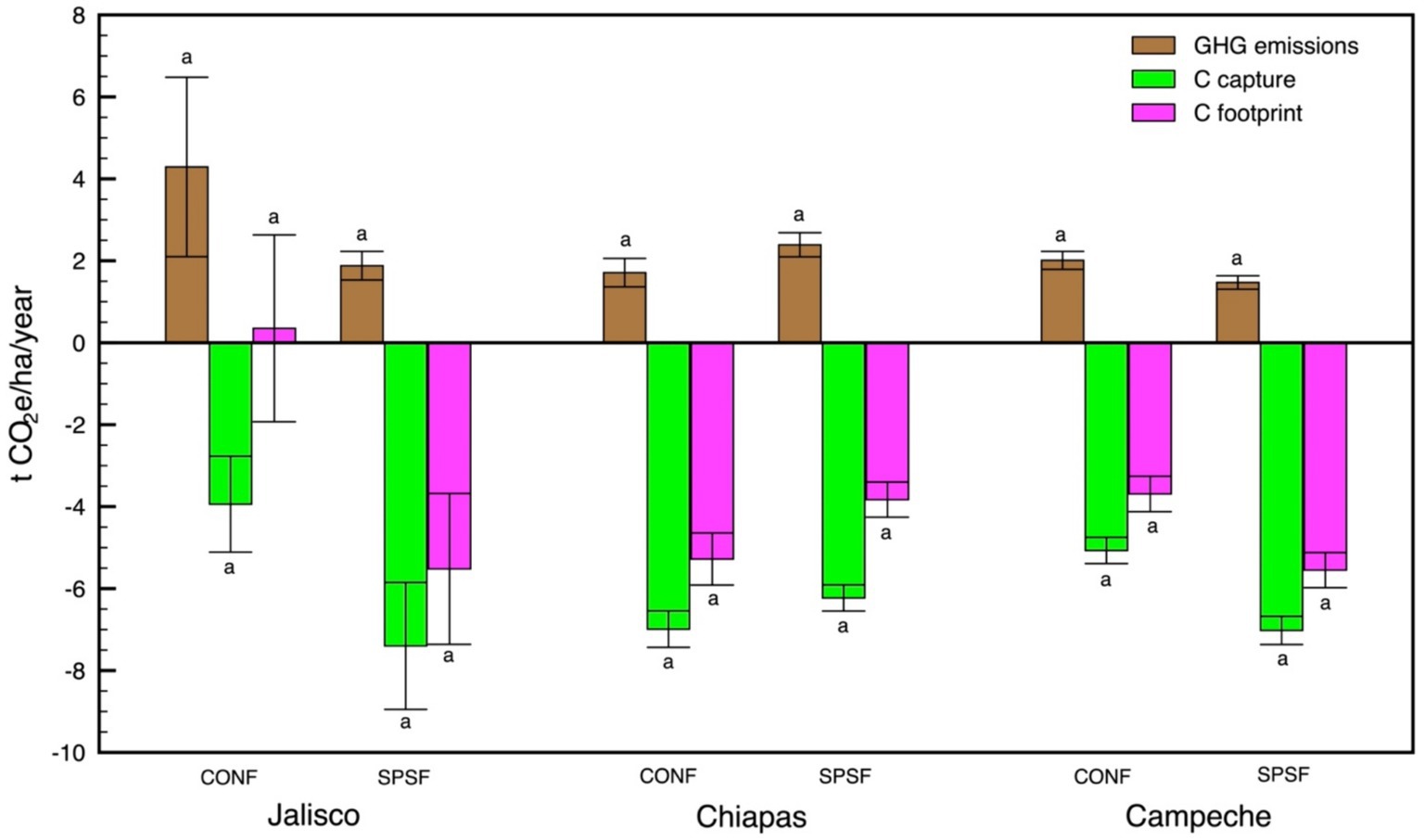
Figure 5. Estimated greenhouse gas (GHG) emissions, carbon fixation and carbon footprint according to conventional and silvopastoral systems in the livestock sector in Jalisco, Chiapas and Campeche (Mexico). Error bars indicate standard error of the mean. Different letters indicate statistical differences (p < 0.05) between system types and between states. CONF: conventional farms; SPSF: silvopastoral systems farms.
The CF of the SPSF was 73% lower than for CONF (−5.0 vs. −2.9 t CO2e/ha/year, respectively). The SPSF clearly fix more carbon than the CONF and thus appear to be more environmentally friendly. SPSF presented similar CF for the three states: −3.8 vs. −5.5 vs. −5.6 t CO2e/ha/year for Chiapas, Jalisco and Campeche, respectively. In contrast, the lowest CF for CONF was achieved in Chiapas (−5.3 t CO2e/ha/year), which had a 43% greater degree of carbon sequestration than in Campeche and much larger than in Jalisco, which had a positive CF – that is, they captured less carbon than the GHG they emitted (Figure 6A).
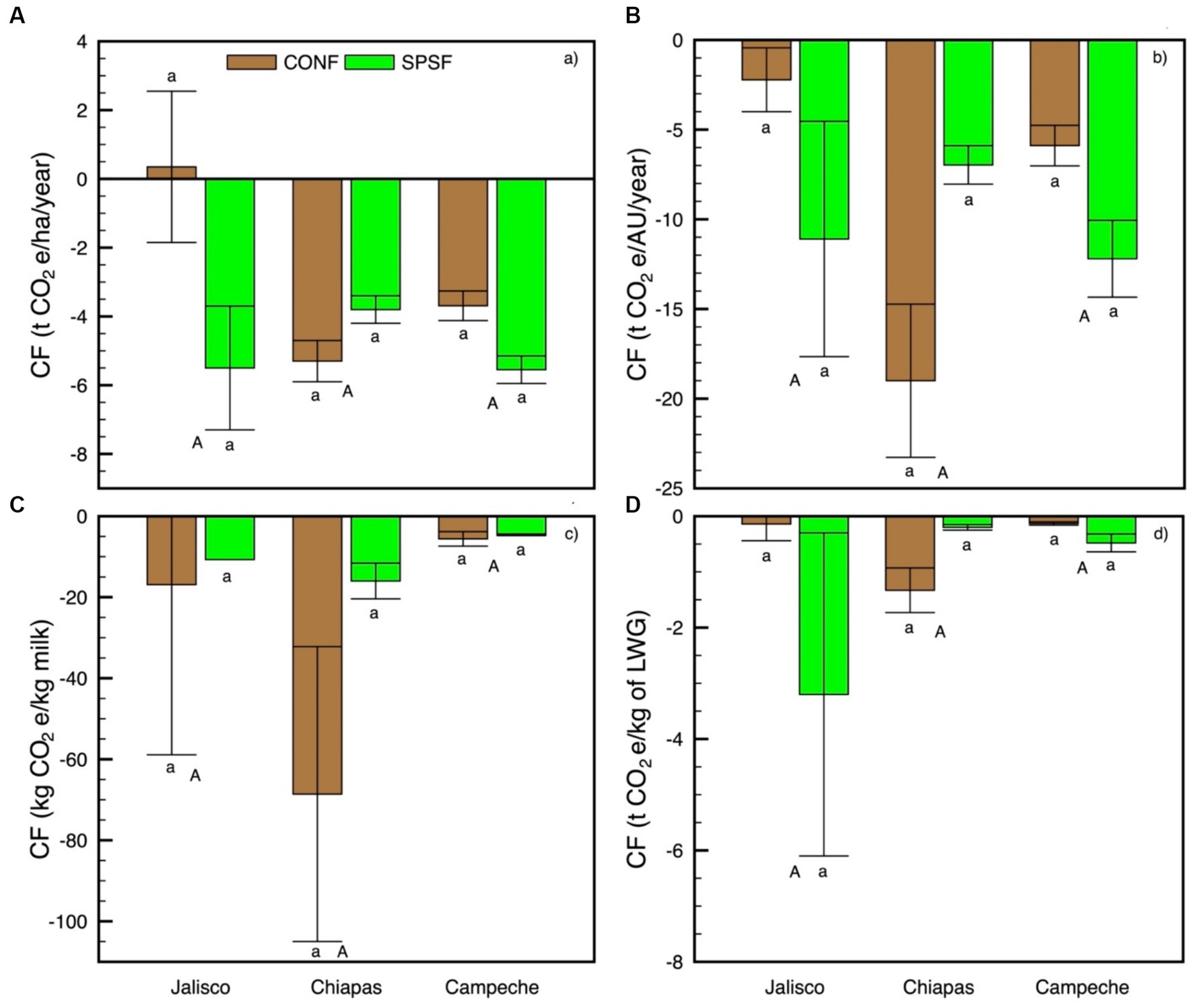
Figure 6. Carbon footprint in livestock conventional farms (CONF) and silvopastoral systems farms (SPSF) in Campeche, Chiapas and Jalisco (Mexico). (A) Total carbon footprint (CF), (B) unitary CF per animal unit (b), (C) unitary CF per milk production and (D) unitary CF per meat sold (d). Error bars indicate standard error of the mean. Different lowercase letters indicate statistical differences (p < 0.05) between system type by state. Different capital letters indicate statistical differences (p < 0.05) between states.
3.4 Unitary carbon footprint
SPSF had a unitary CF 11.7% lower than CONF (−10.1 vs. −9.0 t CO2e/UA/year, respectively). However, no statistical differences (p > 0.05) were detected between states and management types. In particular, SPSF in Chiapas presented a unit footprint 23% higher than in Campeche and Jalisco (−7.0 vs. −11.1 vs. −12.2 t CO2e/AU/yr., respectively) (Figure 6B).
The CF of cattle farms with milk production did not show significant differences (p > 0.05) between management systems or between states. The average CF of the CONF amounted to −50.3 kg CO2e/kg of milk, 3.3 times lower than for SPSF (−15.1 kg CO2e/kg of milk). In the state of Chiapas, CONF had the lowest CF (−68.6 kg CO2e/kg of milk), in contrast to the states of Jalisco and Campeche where this value reached −16.9 and − 5.6 kg CO2e/kg of milk, respectively (Figure 6C).
The CF from beef farms did not show significant differences between management types (p > 0.05); however, the average of the SPSF was higher than for the CONF (−0.61 vs. −0.84 t CO2e/kg of beef) (Figure 6D). In SPSF in Chiapas and Campeche, a higher footprint was estimated than that achieved for the same types of farm in Jalisco (−0.22 vs. −0.48 vs. −3.2 t CO2e/kg of meat). In the state of Campeche, CONF had a higher CF than those with the same management, but in Jalisco and Chiapas (−0.13 vs. −0.14 vs. −1.3 t CO2e/kg of meat, respectively) (Figure 6D).
4 Discussion
GHG emissions in livestock production systems depend greatly on the characteristics of the herd and how it is fed (Yunga, 2020). This was confirmed in the present research, because animals have more options to select higher quality feed when they have more forage area, which would reduce methane emissions (Gutiérrez Bermúdez and Mendieta Araica, 2022). Numerous studies (López-Santiago et al., 2019; Carvajal and Andrade, 2020; Yunga, 2020), have confirmed that the use of SPS contributes to reducing GHG emissions from livestock farms. Here, we found that this reduction was on the order of 17%, which is a considerable step toward achieving the goals of returning to livestock as a mitigator of climate change (Schettini et al., 2021; Gutiérrez Bermúdez and Mendieta Araica, 2022). Despite this, some slight differences can be noted between states, which can basically be explained by the broad characteristics of SPS, which can vary in the abundance, type and size of perennial woody plants (Villanueva-López et al., 2015; López et al., 2019; Schettini et al., 2021).
Animal emissions contributed 98% to total GHG emissions, mainly in the form of methane from enteric fermentation, as has been widely noted (Molina-Rivera et al., 2019; González-Quintero et al., 2021; Tongwane and Moeletsi, 2021; Uddin et al., 2021; Villanueva, 2022). These findings have been confirmed in Brazil, where the largest impact from dairy production is from enteric fermentation (50–52%). In contrast, in semi-confined systems, the largest contribution to farms’ CF comes from feed (with 48%) (de Léis et al., 2015). In Spain, enteric fermentation also explains between 43 and 64% of the CF of livestock systems, which has been linked to system intensification and a grazing diet (Eldesouky et al., 2018). Here, the second most important component of the farms’ CF turned out to be nitrogen fertilization, which is commonly the most heavily weighted in the field of agriculture (Andrade et al., 2014b, 2015).
Use of SPS has also been shown to have positive carbon fixation results, which can reverse the CF. For example, Camero (2020) recorded 3.8 t C/ha/year of carbon fixation, which offset emissions from Brachiaria brizantha and Erythrina berteroana in northern Costa Rica. In contrast, in a previous study in Campeche, no differences in GHG emissions were found between extensive monoculture grazing, intensive SPS and low-intensity system or fallows, because 92% of animal consumption was grasses in all systems and the area devoted to leguminous crops and fallows was only 12 and 15%, respectively (Molina-Rivera et al., 2019).
Switching from degraded pastures to any other type of land use system always results in positive benefits for climate change mitigation, because it allows for carbon concentration (Apan et al., 2021). SPS allow such reconversion – that is, from net CO2 emitting systems, such as degraded pastures, to net carbon-fixing systems – while at the same time increasing production, quality and improving the supply of other ecosystem services (Andrade et al., 2008; López-Santiago et al., 2019; Apan et al., 2021; Schettini et al., 2021).
Although most of the livestock farms evaluated presented a negative CF (that is, they fixed more carbon than they emitted), those involving SPS contributed more to climate change mitigation by fixing more carbon per animal unit or per unit of milk or meat sold. However, there was an exception in Chiapas, where CONF appeared able to fix more carbon than SPSF. This may be due to the nature of SPS, which could be very similar to conventional systems, or because the level of livestock production on conventional cattle ranches is much higher than on those that use trees (Apan et al., 2021). The application of these results should thus consider local information, because different factors affect CF, including the uncertainty in the permanence of carbon in biomass.
The CF depends greatly on the extent to which the country has developed and their cattle production. Countries such as Norway, Sweden, Portugal, Denmark and the Netherlands have reported a CF for conventional cattle systems higher than 1 kg CO2e/kg milk (de Léis et al., 2015). In contrast, confined dairy production systems Brazil showed a lower CF than semi-confined or grazing systems (0.54 vs. 0.78 and 0.74 kg CO2e/kg milk, respectively) (de Léis et al., 2015). In temperate and tropical Mexico, these values have been estimated at 0.55 and 0.91 kg CO2e/kg milk, respectively; while values were around 0.53 kg CO2e/kg milk in India (Garg et al., 2013). In Colombia, small cattle ranches with low levels of technology had a CF of 3.75 kg CO2e/kg milk (Páez et al., 2018) and 3.3 kg CO2e/kg milk and 10.5 kg CO2e/kg meat sold in dual-purpose cattle ranching (González-Quintero et al., 2021). The differences from this study are possibly related to higher levels of milk production, consistent diet quality, lower use of external inputs and the presence of trees on farms (Villanueva, 2022).
Looking at meat production, the CF was 32.5 kg CO2e/kg of beef and 28.0 kg CO2e/kg of meat of small ruminants for 2010 in Spain (Aguilera et al., 2020). Specialized dairy production systems in Costa Rica have a CF between 2.28 and 11.06 kg CO2e/kg, and dual-purpose livestock contribute slightly more, with values between 5.45 and 23.72 kg CO2e/kg for milk and LWG, respectively (Villanueva, 2022). Meanwhile, in hot and humid areas of Mexico, Molina-Rivera et al. (2019) found that emissions of 23.1 kg CO2e/kg live weight for beef production.
Latin America is seen as an area with potential for improving food security, where cattle ranching is presented as an opportunity for sustainable economic growth in Mexico (Apan et al., 2021). However, improving livestock farming requires cooperation between actors to adopt improved pastures and implement productive and environmentally friendly practices, such as SPS, which support the initiatives of the Global Roundtable on Sustainable Livestock (Triana-Ángel and Burkart, 2021). Rigorous local studies are still needed on sustainable soil development in agricultural and forestry practices, given that the efficiency of mitigation and adaptation strategies depends on climatic, edaphic and social characteristics, as well as the historical pattern of land use and management (Aryal et al., 2020; Alexandre et al., 2021). Unification of methodologies is also needed to estimate the CF of the sector, including a thorough analysis of the type of system, number of individuals, the technological level in each link of the production chain (Páez et al., 2018), associated plant species, livestock genotype, integrated feeding strategies and best agricultural management practices (Aryal et al., 2020; González-Quintero et al., 2021).
Livestock CF monitoring can help identify opportunities to improve environmental performance and allows tracking and evaluation of environmental impacts that contribute to climate change and land use changes (de Léis et al., 2015). Sustainable cattle ranching that includes native trees and shrubs provides other benefits that contribute to biodiversity, reforestation and added value for timber and non-timber forest products, which in turn can strengthen the family and cooperative economy (Ruíz et al., 2021).
5 Conclusion
The CF of livestock farms in the three states studied was dependent on the total forage area and the number of animals. In this exercise, SPS contributed to reduce total GHG emissions – 8.0 to 5.7 t CO2e/ha/year – mainly due to improvements in the animals’ diet and reductions in the doses of nitrogen fertilizers. SPS also increased carbon fixation in total biomass and soils, clearly providing a double benefit in terms of GHG emissions reduction and carbon fixation. SPSF increase a negative CF 73% lower than CONF, which demonstrates the importance of SPS in mitigating climate change.
In all management type–state combinations, except for CONF in Jalisco, a negative CF was found – that is, carbon fixation in biomass and soils was greater than GHG emissions. This demonstrates that these farms are currently mitigating climate change by fixing carbon. However, the use of SPS on livestock farms currently contributes to an increase the net carbon sequestration. The CF per animal unit and per unit of milk produced and meat sold turned out to be negative and quite variable among the different combinations of state and management types. In any case, the best CF values were found in the CONF of Jalisco for milk and in the SPSF of Campeche for the production and sale of meat. The findings of this study provide the basis for policy and technical guidelines to promote more environmentally friendly livestock farms by fixing a greater amount of atmospheric CO2.
Data availability statement
The original contributions presented in the study are included in the article/supplementary material, further inquiries can be directed to the corresponding author.
Author contributions
HA: Conceptualization, Writing – original draft, Writing – review & editing. AV: Validation, Writing – review & editing. AM-S: Supervision, Validation, Writing – review & editing. CV: Formal analysis, Writing – review & editing. JJ-T: Methodology, Validation, Writing – review & editing. JB-S: Methodology, Validation, Writing – review & editing. EP: Methodology, Validation, Writing – review & editing. MI: Funding acquisition, Investigation, Supervision, Writing – review & editing. CS: Funding acquisition, Methodology, Supervision, Writing – review & editing.
Funding
The author(s) declare that no financial support was received for the research, authorship, and/or publication of this article.
Acknowledgments
The authors would like to thank the BioPaSOS project and especially the local technicians and producers who kindly shared their information with us.
Conflict of interest
The manuscript was prepared and reviewed by the authors, who declare that there are no conflicts of interest that would jeopardize the validity of the results presented.
Publisher’s note
All claims expressed in this article are solely those of the authors and do not necessarily represent those of their affiliated organizations, or those of the publisher, the editors and the reviewers. Any product that may be evaluated in this article, or claim that may be made by its manufacturer, is not guaranteed or endorsed by the publisher.
References
Aguilera, E., Piñero, P., Infante Amate, J., González de Molina, M., Lassaletta, L., and Sanz Cobeña, A. (2020). Emisiones de gases de efecto invernadero en el sistema agroalimentario y huella de carbono de la alimentación en España. España: Real Academia de Ingeniería.
Alexandre, G., Cheval, A., Perrette, J., Apotout, M., Diman, J. L., Larade, A., et al. (2021). Livestock activities in agroforestry systems in Guadeloupe: systems of production and functions. Agrofor. Syst. 95, 1445–1458. doi: 10.1007/s10457-021-00651-5
Ali, A., Akram, W., Ahmad, F., and Ahmad, M. (2020). Dynamic relationship among agriculture-energy-forestry and carbon dioxide (CO2) emissions: empirical evidence from China. Environ. Sci. Pollut. Res. 27, 34078–34089. doi: 10.1007/s11356-020-09560-z
Andrade, H. J., Brook, R., and Ibrahim, M. (2008). Growth, production and carbon sequestration of silvopastoral systems with native timber species in the dry lowlands of Costa Rica. Plant Soil 308, 11–22. doi: 10.1007/s11104-008-9600-x
Andrade, H. J., Campo, O., and Segura, M. A. (2014b). Huella de carbono del sistema de producción de arroz (Oryza sativa) en el municipio de Campoalegre, Huila. Colombia. Corpoica Ciencia y Tecnología Agropecuaria. 15, 25–31,
Andrade, H. J., Marín, L. M., and Pachón, D. P. (2014a). Fijación de carbono y porcentaje de sombra en sistemas de producción de café (Coffea arabica L.) en el Líbano, Tolima, Colombia. Bioagro. 26, 127–132,
Andrade, H. J., Segura, M. A., and Varona, J. P. (2015). Estimación de huella de carbono del sistema de producción de caña de azúcar (Saccharum officinarum) en Palmira, Valle del Cauca, Colombia. Revista de Investigación Agraria y Ambiental. 6, 19–27,
Apan, G. W., Jiménez, G., Nahed, J., Pérez, E., and Piñeiro, Á. T. (2021). Masificación de sistemas silvopastoriles: un largo y sinuoso camino. Tropical and Subtropical Agroecosystems. 24, 1–17,
Aryal, D. R., Morales-Ruiz, D. E., López-Cruz, S., Tondopó-Marroquín, C. N., Lara-Nucamendi, A., Jiménez-Trujillo, J. A., et al. (2022). Silvopastoral systems and remnant forests enhance carbon storage in livestock-dominated landscapes in Mexico. Sci. Rep. 12:16769. doi: 10.1038/s41598-022-21089-4
Aryal, J. P., Rahut, D. B., Sapkota, T. B., Khurana, R., and Khatri-Chhetri, A. (2020). Climate change mitigation options among farmers in South Asia. Environ. Dev. Sustain. 22, 3267–3289. doi: 10.1007/s10668-019-00345-0
Bautista, M. A., Bonales, J., and Val, D. (2020). Dinámicas de la cadena de bovinos productores de carne mexicana en el siglo XXI, in Factores Críticos y Estratégicos en la Interacción Territorial Desafíos Actuales y Escenarios Futuros, eds. S. VegaDe la, R. E. Rózga, and C. Hoyos. Mexico: Universidad Nacional Autónoma de México y Asociación Mexicana de Ciencias para el Desarrollo Regional A.C.
Biodiversidad y Paisajes Ganaderos Agrosilvopastoriles Sostenibles [BioPaSOS]. (s.f.) (2017). Caracterización de la ganadería bovina en el estado de Campeche, México. México: CATIE, SAGARPA, CONABIO-IICA, Ministerio Federal de Medio Ambiente, Protección de la Naturaleza y Seguridad Nuclear.
Brook, R., Forster, E., Styles, D., Mazzetto, A. M., Arndt, C., Esquivel, M. J., et al. (2022). Silvopastoral systems for offsetting livestock emissions in the tropics: a case study of a dairy farm in Costa Rica. Agron. Sustain. Dev. 42:101. doi: 10.1007/s13593-022-00834-z
Camero, L. A. (2020). Fijación de carbono en un sistema silvopastoril (Erythrina berteroana Urban y Brachiaria brizantha CV Toledo) de una explotación lechera en la Región Huetar Norte de Costa Rica. Revista AgroInnovación en el Trópico Húmedo. 2, 19–26. doi: 10.18860/rath.v2i2.5194
Canu, F., Wretlind, P. H., Audia, I., Tobar, D., and Andrade, H. J. (2018). NAMA para un sector ganadero bajo en carbono y resiliente al clima en Honduras. Dinamarca: Technical University of Denmark, Gobierno de la Republica de Honduras, CATIE, UNEP DTU Partnership, NDF, NEFCO.
Carvajal, B. N., and Andrade, H. J. (2020). Captura de carbono en biomasa de sistemas de uso del suelo, municipio de Yopal, Casanare Colombia. Orinoquia 24, 13–22. doi: 10.22579/20112629.587
Centro Agronómico Tropical de Investigación y Enseñanza [CATIE] . (2010). Determinación del balance de gases efecto invernadero en fincas ganaderas de la región Chorotega, como elemento de referencia para mejorar la competitividad: Programa de fomento de la producción agropecuaria sostenible (PFPAS)-Programa de Ganadería y Manejo del medio ambiente (GAMMA). Available at: http://www.mag.go.cr/bibliotecavirtual/P40-9906.pdf (Accessed August, 2023).
de Figueiredo, E. D., Panosso, A. R., de Oliveira, R., Teixeira, D., Berchielli, T. T., and La Scala, N. (2016). Soil CO2-C emissions and correlations with soil properties in degrade and managed pastures in southern Brazil. Land Degrad. Dev. 28, 1263–1273. doi: 10.1002/ldr.2524
de Léis, C. M., Cherubini, E., Ruviaro, C. F., da Silva, V. P., Nascimento, V., Spies, A., et al. (2015). Carbon footprint of milk production in Brazil: a comparative case study. Int. J. Life Cycle Assess. 20, 46–60. doi: 10.1007/s11367-014-0813-3
Di Rienzo, J., Casanoves, F., González, L., Tablada, M., Robledo, C., Balzarini, Y., et al. (2019). Software. Córdoba, Argentina: Grupo InfoStat, FCA, Universidad Nacional de Córdoba.
Eldesouky, A., Mesias, F. J., Elghannam, A., and Escribano, M. (2018). Can extensification compensate livestock greenhouse gas emissions? A study of the carbon footprint in Spanish agroforestry systems. J. Clean. Prod. 200, 28–38. doi: 10.1016/j.jclepro.2018.07.279
Elshimy, M., and El-Aasar, K. (2020). Carbon footprint, renewable energy, non-renewable energy, and livestock: testing the environmental Kuznets curve hypothesis for the Arab world. Environ. Dev. Sustain. 22, 6985–7012. doi: 10.1007/s10668-019-00523-0
Fonseca, W., Rey Benayas, J. M., and Alice, F. E. (2011). Carbon accumulation in the biomass and soil of different ages in the humid tropics of Costa Rica. For. Ecol. Manag. 262, 1400–1408. doi: 10.1016/j.foreco.2011.06.036
Garg, M. R., Sherasiaa, P. L., Bhanderia, B. M., Phondbaa, B. T., Shelkea, S. K., and Makkar, H. P. (2013). Effects of feeding nutritionally balanced rations on animal productivity, feed conversion efficiency, feed nitrogen use efficiency, rumen microbial protein supply, parasitic load, immunity and enteric methane emissions of milking animals under field conditions. Anim. Feed Sci. Technol. 179, 24–35. doi: 10.1016/j.anifeedsci.2012.11.005
Gerber, P. J., Steinfeld, H., Henderson, B., Mottet, A., Opio, C., Dijkman, J., et al. (2013). Tackling climate change through livestock – A global assessment of emissions and mitigation opportunities. Rome: Food and Agriculture Organization of the United Nations (FAO).
Ghosh, A., Misra, S., Bhattacharyya, R., Sarkar, A., Kumar, A., Chandra, V., et al. (2020). Agriculture, dairy and fishery farming practices and greenhouse gas emission footprint: a strategic appraisal for mitigation. Environ. Sci. Pollut. Res. 27, 10160–10184. doi: 10.1007/s11356-020-07949-4
de Jalisco, G. (2011). Plan Regional de Desarrollo 2030. Región 01 Norte. 2nd Edn. Jalisco, México: Espíritu de la tierra.
de México, G. . (2018). Proyecciones de la población de México y de las entidades federativas 2016–2050. México. Available at: https://www.gob.mx/cms/uploads/attachment/file/390824/InfografaProyeccionesdelapoblacindeMxico.pdf.
Gobierno del Estado de Campeche . (2015). Plan estatal de desarrollo Campeche 2015–2021. Available at: https://n9.cl/gltwe (Accessed August, 2023).
Gobierno del Estado de Chiapas . (2019). Plan estatal de desarrollo Chiapas 2019–2024. Available at: http://www.haciendachiapas.gob.mx/planeacion/Informacion/PED/PED-2019.pdf (Accessed October 18, 2023).
González-Quintero, R., Kristensen, T., Sánchez-Pinzón, M. S., Bolívar-Vergara, D. M., Chirinda, N., Arango, J., et al. (2021). Carbon footprint, non-renewable energy and land use of dual-purpose cattle systems in Colombia using a life cycle assessment approach. Livest. Sci. 244:104330. doi: 10.1016/j.livsci.2020.104330
Grain . (2017). Big meat and dairy’s supersized climate footprint. Available at: https://www.grain.org/article/entries/5825-big-meat-and-dairy-s-supersized-climate-footprint (Accessed October 18, 2023).
Gutiérrez Bermúdez, C. C., and Mendieta Araica, B. G. (2022). Sistemas silvopastoriles: una alternativa para la ganadería bovina sostenible. La Calera. 22, 1–10. doi: 10.5377/calera.v22i38.14193
Hawkins, J., Yesuf, G., Zijlstra, M., Schoneveld, G. C., and Rufino, M. C. (2021). Feeding efficiency gains can increase the greenhouse gas mitigation potential of the Tanzanian dairy sector. Sci. Rep. 11:4190. doi: 10.1038/s41598-021-83475-8
Instituto Nacional de ecología y Cambio Climático [INECC] (2014). Factores de emisión para los diferentes tipos de combustibles fósiles y alternativos que se consumen en México. Coyoacán, México: Secretaría de Medio Ambiente y Recursos Naturales.
Instituto Nacional de ecología y Cambio Climático [INECC] (2018). Inventario Nacional de Emisiones de Gases y Compuestos de Efecto Invernadero – 2015. México: INECC.
Instituto Nacional de Estadística y Geografía [INEGI] (2016). Anuario estadístico y geográfico de Chiapas 2016. México: Gobierno del Estado de Chiapas.
Intergovernmental Panel on Climate Change [IPCC] (2006). Guidelines for national greenhouse gas inventories. Tokyo, Japan: Institute for global environmental strategies (IGES).
Intergovernmental Panel on Climate Change [IPCC] (2013). “Climate change 2013: the physical science basis” in Contribution of working group I to the fifth assessment report of the intergovernmental panel on climate change. eds. T. F. Stocker, D. Qin, G. K. Plattner, M. Tignor, S. K. Allen, and J. Boschung (Cambridge, UK: Cambridge University Press).
López, O., Lamela, L., Sánchez, T., Olivera, Y., García, R., Herrera, M., et al. (2019). Evaluación del valor nutricional de los forrajes en un sistema silvopastoril. Pastos y Forrajes. 42, 57–67,
López-Santiago, J. G., Casanova, F., Villanueva, G., Díaz, V. F., Solorio, F. J., Martínez, P., et al. (2019). Carbon storage in a silvopastoral system compared to that in a deciduous dry forest in Michoaca’n Mexico. Agrofor. Syst. 93, 199–211. doi: 10.1007/s10457-018-0259-x
Martínez-García, C. G., Dorward, P., and Thair, R. (2012). Farm and socieconomic characteristics of small-holder milk producers and their influence on the technology adoption in Central México. Trop. Anim. Health Prod. 44, 1199–1211. doi: 10.1007/s11250-011-0058-0
Mazzetto, A. M., Bishop, G., Styles, D., Arndt, C., Brook, R., and Chadwick, D. (2020). Comparing the environmental efficiency of milk and beef production through life cycle assessment of interconnected cattle systems. J. Clean. Prod. 277:124108. doi: 10.1016/j.jclepro.2020.124108
Mena, V. E., and Andrade, H. J. (2021). Potencial de reducción de emisiones y captura de carbono en bosques y sistemas agroforestales con cacao en el Pacífico colombiano. Rev. Biol. Trop. 69, 1252–1263. doi: 10.15517/rbt.v69i4.45927
Molina-Rivera, M., Olea-Perez, R., Galindo-Maldonado, F. A., and Arriaga-Jordán, C. M. (2019). Análisis de ciclo de vida de tres sistemas ganaderos tropicales en Campeche, México: caso de estudio. Trop. Subtrop. Agroecosyst. 22, 127–141. doi: 10.56369/tsaes.2774
Nahed-Toral, J., Valdivieso-Pérez, A., Aguilar-Jiménez, R., Cámara-Cordova, J., and Grande-Cano, D. (2013). Silvopastoral systems with traditional management in southeastern Mexico: a prototype of livestock agroforestry for cleaner production. J. Clean. Prod. 57, 266–279. doi: 10.1016/j.jclepro.2013.06.020
Navarrete, C. (2020). Los sistemas de producción de rumiantes en la Comarca Lagunera, México: impacto ambiental, tendencias productivas y estrategias de mitigación. [Tesis Doctoral]. Chapingo, México: Universidad Autónoma de Chapingo.
Oldfield, E. E., Eagle, A. J., Rubin, R. L., Rudek, J., Sanderman, J., and Gordon, D. R. (2022). Crediting agricultural soil carbon sequestration. Science 375, 1222–1225. doi: 10.1126/science.abl7991
Ortiz, A. M., and Riascos, L. D. (2006). Almacenamiento y fijación de carbono del sistema agroforestal cacao Theobroma cacao L y laurel Cordia alliodora (Ruiz & Pavón) Oken en la reserva indígena de Talamanca, Costa Rica. [Tesis de pregrado]. San Juan de Pato (Colombia): Universidad de Nariño.
Páez, E. M., Corredor, E. S., and Fonseca, J. A. (2018). La huella hídrica y la huella de carbono: herramientas para estimar el impacto de la ganadería bovina. Pensamiento y Acción. 24, 81–92,
Pagiola, S., Agostini, P., Gobbi, J., De Haan, C., Ibrahim, M., Murgueitio, E., et al. (2004). Paying for biodiversity conservation services in agricultural landscapes. Washington, DC: The International Bank for Reconstructionand Development.
Parra, A. S., Ramirez, D. Y. G., and Martínez, E. A. (2022). Silvopastoral systems ecological strategy for decreases C footprint in livestock Systems of Piedmont (Meta), Colombia. Braz. Arch. Biol. Technol. 66:e23220340. doi: 10.1590/1678-4324-2023220340
Prospero-Bernal, F., Velarde-Guillén, J., and Arriaga-Jordán, C. M. (2019). La ganadería mexicana ante el cambio climático. México: Universidad Autónoma del Estado de México.
Rice, P., O'Brien, D., Shalloo, L., and Holden, N. M. (2017). Evaluation of allocation methods for calculation of carbon footprint of grass-based dairy production. J. Environ. Manag. 202, 311–319. doi: 10.1016/j.jenvman.2017.06.071
Ruíz, N., Carrera, F., Villalobos, R., and Durán, L. (2021). Sistematización de la contribución de los bosques modelo al cumplimiento de los objetivos de desarrollo sostenible. Turrialba, Costa Rica: CATIE.
Schettini, B. L. S., Jacovine, L. A. G., Oliveira Neto, S. N., Torres, C. M. M. E., Rocha, S. J. S. S., Villanova, P. H., et al. (2021). Silvopastoral systems: ¿how to use them for carbon neutral milk production? Carbon Manag. 12, 377–384. doi: 10.1080/17583004.2021.1951843
Sánchez-Romero, R., Mora-Ardila, F., Val-Arreola, D., and González-Esquivel, C. E. (2022). Estimation of the forage potential of trees in silvopastoral systems of a dry tropical forest in Jalisco Mexico. Agrofor. Syst. 96, 129–145. doi: 10.1007/s10457-021-00704-9
Secretaría de Medio Ambiente y Recursos Naturales [SEMARNAT] (2020). “Contribución determinada a nivel nacional. Actualización 2020” in México por el clima (México: Gobierno de México).
Secretaría de Medio Ambiente y Recursos Naturales [SEMARNAT] (2021a). Lista de combustibles y sus poderes caloríficos 2021 que se considerarán para identificar a los usuarios con un patrón de alto consumo, así como los factores para determinar las equivalencias en términos de barriles equivalentes de petróleo. México: Ciudad de México. Available at: https://www.gob.mx/cms/uploads/attachment/file/619062/ListaCombustibles202126feb2021.pdf.
Secretaría de Medio Ambiente y Recursos Naturales [SEMARNAT] (2021b). Factor de emisión del sistema eléctrico nacional 2020. México: Ciudad de México. Available at: https://www.gob.mx/cms/uploads/attachment/file/630693/AvisoFEE2020.pdf.
Servicio de Información Agroalimentaria y Pesquera [SIAP] . (2016). Población bovina de por Estado. Available at: www.siap.gob.mx (Accessed August 21, 2017).
Tobar, D., Vega, A., García, E., and Sepúlveda, C. (2018). Balance de carbono en fincas ganaderas de Honduras. En las tres zonas del proyecto paisajes productivos. Tegucigalpa, Honduras: CATIE, GEF, PNUD, Gobierno de la República de Honduras: Secretaría de Recursos Naturales y Ambiente.
Thoma, G., Jolliet, O., and Wang, Y. (2013). A biophysical approach to allocation of life cycle environmental burdens for fluid milk supply chain analysis. Int. Dairy J. 31, S41–S49. doi: 10.1016/j.idairyj.2012.08.012
Tongwane, M., and Moeletsi, M. (2021). Provincial cattle carbon emissions from enteric fermentation and manure management in South Africa. Environ. Res. 195:110833. doi: 10.1016/j.envres.2021.110833
Triana-Ángel, N., and Burkart, S. (2021). Gestar cambios, transformar el sector: el caso de la Mesa Colombiana de Ganadería Sostenible. Wageningen, The Netherlands: CGIAR.
Udawatta, R. P., Walter, D., and Jose, S. (2022). Carbon sequestration by forests and agroforests: A reality check for the United States. Carbon Footprints. 2023, 1–24. doi: 10.20517/cf.2022.06
Uddin, M. E., Aguirre-Villegas, H. A., Larson, R. A., and Wattiaux, M. A. (2021). Carbon footprint of milk from Holstein and Jersey cows fed low or high forage diet with alfalfa silage or corn silage as the main forage source. J. Clean. Prod. 298:126720. doi: 10.1016/j.jclepro.2021.126720
Vega, A. (2016). Análisis de herramientas para la estimación de gases de efecto invernadero (GEI) y su aplicación en sistemas de producción doble propósito en fincas ganaderas de la cuenca del río Jesús María, Costa Rica. [Tesis de maestría]. Turrialba (Costa Rica): CATIE.
Velarde-Guillén, J., Arndt, C., and Gómez, C. A. (2022). Carbon footprint in Latin American dairy systems. Trop. Anim. Health Prod. 54, 1–7. doi: 10.1007/s11250-021-03021-6
Villanueva, C. (2022). Análisis de las emisiones de gases de efecto invernadero en fincas productoras de leche de Costa Rica. [Tesis de doctorado]. [Santiago de Compostela (España)]: Universidad de Santiago de Compostela.
Villanueva-López, G., Martínez-Zurimendi, P., Casanova-Lugo, F., Ramírez-Avíles, L., and Montañez-Escalante, P. I. (2015). Carbon storage in livestock systems with and without live fences of Gliricidia sepium in the humid tropic of México. Agrofor. Syst. 89, 1083–1096. doi: 10.1007/s10457-015-9836-4
He, X., Sunday Adebayo, T., Kirikkaleli, D., and Umar, M. (2021). Consumption-based carbon emissions in Mexico: an analysis using the dual adjustment approach. Sustain. Product. Consump. 27, 947–957. doi: 10.1016/j.spc.2021.02.020
Yunga, S. A. (2020). Análisis comparativo del cálculo de huella de carbono por litro de leche producido, entre pequeños y medianos productores que emplean prácticas ganaderas convencionales y pequeños y medianos productores que emplean prácticas de ganadería climáticamente inteligente. [Tesis de Maestría]. Quito (Ecuador): Universidad Andina Simón Bolivar.
Keywords: biomass, greenhouse gasses, mitigation, land use systems, carbon capture
Citation: Andrade HJ, Vega A, Martínez-Salinas A, Villanueva C, Jiménez-Trujillo JA, Betanzos-Simon JE, Pérez E, Ibrahim M and Sepúlveda L CJ (2024) The carbon footprint of livestock farms under conventional management and silvopastoral systems in Jalisco, Chiapas, and Campeche (Mexico). Front. Sustain. Food Syst. 8:1363994. doi: 10.3389/fsufs.2024.1363994
Edited by:
Alexandra Jacquelyn Burgess, University of Nottingham, United KingdomReviewed by:
Azam Lashkari, John Innes Centre, United KingdomSimon Moakes, Aberystwyth University, United Kingdom
Copyright © 2024 Andrade, Vega, Martínez-Salinas, Villanueva, Jiménez-Trujillo, Betanzos-Simon, Pérez, Ibrahim and Sepúlveda Lopez. This is an open-access article distributed under the terms of the Creative Commons Attribution License (CC BY). The use, distribution or reproduction in other forums is permitted, provided the original author(s) and the copyright owner(s) are credited and that the original publication in this journal is cited, in accordance with accepted academic practice. No use, distribution or reproduction is permitted which does not comply with these terms.
*Correspondence: Hernán J. Andrade, hjandrade@ut.edu.co