Analysis of the impact of farm machinery outsourcing services on farmers’ cropping structure and grain production: an empirical evidence from Henan Province, China
- 1School of Economics and Management, Jiangxi Agricultural University, Nanchang, China
- 2College of Economics and Management, South China Agricultural University, Guangzhou, China
- 3School of Digital Economy, Jiangxi Open University, Nanchang, China
Introduction: The impact of farm machinery outsourcing services (FMOS) on grain production has been extensively studied in the literature. However, whether FMOS would adjust cropping structures and ensure food security is little known. To address this research gap, we analyzed the impact of FMOS on the cropping structure adjustment and grain production of farmers.
Methods: We estimate the impact of FMOS on grain production by using data collected from a survey of 3,863 wheat farmers in China. We employ the Extended Regression Model (ERM) to address selectivity bias.
Results and discussion: The empirical results show that FMOS can adjust the cropping structure and increase the proportion of household food cultivation. Among all segments of FMOS, fertilizer application services drive the increase in grain acreage more significantly. We also find that large-scale households prefer to self-purchase farm machinery rather than FMOS, leading to an insignificant impact of FMOS on cropping structure. Therefore, in the process of promoting the development of FMOS, we should focus on the coordinated development of various types of FMOS, strengthen the popularization and application of fertilization services, and formulate a differentiated subsidy policy for heterogeneous farmers in terms of scale to better play the role of agricultural machinery in promoting grain production.
1 Introduction
Meeting the food demand of the global population is an essential challenge for humanity (Godfray et al., 2010; Springmann et al., 2018). China has been making great efforts to ensure food security (Zhu and Jin, 2013; Huang and Zou, 2018). In 2022, the Chinese Government’s Central Document No. 1 clearly emphasizes the need to stabilize grain production and ensure food security (Ye et al., 2023). In the past two decades, the sown area and production of grain crops in China have experienced fluctuations of decreasing and then increasing. The data in Figure 1 show that China’s sown grain area declined between 2000 and 2003. The sown area of grain began to rise between 2004 and 2016. The sown area of grain stabilized after 2017. Grain production and area sown to grain follow the same trend. What motivates adjusting the planting structure from cash to grain crops? Clarifying this question is of great practical significance to China’s efforts to build a food security system.
Existing literature explains the causes of fluctuations in China’s grain cultivation structure from different perspectives, such as changes in property rights and the transfer of agricultural land. Some literature attribute the rapid rise in grain output in the early reform period to the introduction of the household contract responsibility system (Lin, 1992). The subsequent decline in grain output stems from the exhaustion of property rights incentives (Li et al., 1998). The adjustments of agricultural land make property rights unstable and and inhibit farmers’ incentives to invest in production, leading to worry about grain production. The imperfections of agricultural land property rights lead to slow land transfer, hindering the efficient allocation of agricultural land and weakening the performance of agricultural operations (Kimura et al., 2011). In recent years, agricultural land property rights’ role in planting structure adjustment has been reintroduced. The combination of property rights stability and factor allocation works in planting structure adjustment (Luo et al., 2018; Qiu and Luo, 2018; Hong and Luo, 2019). Property rights stability promotes farmers’ long-term investment, increasing the proportion of food cultivation. However, with the completion of the work of confirming and certifying the right to farmland, the right to land is further solidified, and it is necessary to explain the generating mechanism of planting structure adjustment from outside the property rights.
Other scholars have explored the influencing factors of planting structure adjustment based on the perspective of agricultural land transfer (Cai et al., 2015) and found that the degree of market development of agricultural land transfer has an opposite development trend to the area of grain cultivation (Xu et al., 2017). Excessively high farmland transfer costs will lead to planting structures biased towards cash crops (Zhang et al., 2014; Tian and Zhang, 2017), but the phenomenon of non-food planting structure generated by the transfer of land only applies to the scenario of a smaller scale of operation. As the scale of operation expands, the proportion of food planting will increase significantly, presenting the characteristics of the planting structure adjusted toward food production (Zhang and Du, 2015; Chen and Kong, 2016). Transferring land enhances the proportion of grain planting, which is the most crucial factor for the development of the agricultural land market and the development of the agricultural land market. It is worth noting that the transfer of agricultural land has not achieved the expected policy effects, and the trend of land-scale operation is not obvious (Qian et al., 2018a). However, the area and output of grain cultivation show a continuously increasing trend. Based on the agricultural land transfer perspective does not fully explain the phenomenon of planting structure adjustment to grain (Luo, 2019). Some other scholars have examined the generation mechanism of planting structure adjustment from farm household differentiation (Jiang, 2015; Zhao and Zhou, 2018) and grain subsidy policy (Liu and Liu, 2013; Lv and Hu, 2017; Zhou and Zeng, 2019). However, these studies did not achieve consistent conclusions because of the different sources of data and the use of different methodologies.
After China’s rapid urbanization, the rural labor force began to move to the towns and cities, which led to a change in the cultivation structure. Some scholars explored the intrinsic motivation of planting structure adjustment based on the perspective of non-agricultural transfer of labor (Qian et al., 2018b) and formed two different schools of thought. Some scholars advocated that the non-agricultural transfer of labor will significantly promote the planting structure adjustment, increase the proportion of grain planting (Huang and Li, 2019), and reduce the planting of cash crops (Qi and Tang, 2017). The off-farm employment of farmers can alleviate financial constraints (Chiodi et al., 2012) and promote grain production (Wei et al., 2017). Others argued that the off-farm transfer of labor tightens agricultural labor supply, increases prices, and reduces the cultivation of relatively labor-intensive grain (Yang et al., 2016). The key to the disagreement is whether or not to consider substituting agricultural machinery for labor factors and whether agricultural mechanization can offset the negative impact of the off-farm labor transfer on grain cultivation (Zhong et al., 2016). Non-farm transfer of labor promotes farmers to invest in machinery factors and thus promote the planting structure adjustment (Ji et al., 2012; Wang and Chen, 2016). Agricultural machinery factor inputs have an essential impact on planting structure adjustment.
Although some scholars have noted the role of agricultural machinery inputs in planting structure adjustment, they tend to discuss it based on the perspective of property rights or labor transfer (Tan et al., 2019). However, they have yet to clarify its generating mechanism positively. In particular, in 2004, the Ministry of Finance and the Ministry of Agriculture jointly promulgated the agricultural machinery purchase subsidy policy, further expanding the strength and coverage of agricultural machinery purchase subsidies. The purchase of agricultural machinery has given rise to many FMOS supply organizations. As of December 2018, there were about 200,000 agricultural machinery cooperatives and other operation service organizations in China, with a cumulative operation service area of more than 4 billion mu per year. Compared with self-purchased farm machinery, FMOS can effectively crack the dilemma of small farmers’ capital shortage, especially the cross-area operation service of farm machinery, which breaks through the geographical limitation and the constraints of operation scale and has an essential impact on grain production (Fang et al., 2017). Yang et al. (2018) explored the impact of agricultural mechanization services on grain-sowing areas.
The improvement of agricultural machinery socialization services on grain production has also been verified (Akinbamowo, 2013; Xu et al., 2022). However, the impacts of farm machinery socialization services on food production are different in different segments. Chen et al. (2022) used data from Jiangsu Province. They found that socialization services for transplanting rice seedlings and pest control significantly positively impacted rice yield. In contrast, socialization services during harvesting did not significantly impact rice yield. Different socialized services can be classified into the following two categories to distinguish the heterogeneity of socialized services: technology-intensive and labor-intensive. Technology-intensive includes the plant protection segment (Mottaleb et al., 2017). Labor-intensive refers to the cultivation, transplanting, and harvesting segments, which require large labor inputs (Wang et al., 2016). Using socialized farm machinery services accelerates the saving of labor inputs and may have a smaller effect on rice yield. Liu et al. (2019) found that socialized services significantly positively affected rice yield. Labor-intensive services did not affect rice yield, while technology-intensive services increased rice yield. In addition, other scholars have studied the influencing factors of outsourcing forestry production (Wen et al., 2023). However, they did not analyze further the mechanism of its role and the difference in the role of distinguishing the heterogeneity of the size of farmers.
Therefore, the overall objective of this paper is to analyze whether FMOS affects farmers’ cropping structure adjustment to increase the proportion of food cultivation. In addition, we explore the heterogeneity and the mechanism of action of the impact of FMOS on the cropping structure of farm households. We will make two contributions to the literature that will help enrich the literature on FMOS in developing countries. First, we use ERM modeling to address the selectivity bias. Since farmers voluntarily choose FMOS, the issue of selection bias has to be considered when quantifying the impact of using FMOS. The ERM model addresses selection bias due to observable factors (e.g., age, education, and household size) and unobservable factors (e.g., farmers’ innate ability). Second, we contribute to the literature by investigating whether treatment effects are heterogeneous across conditions of household and farm-level characteristics. The literature pay little attention to this question.
The rest of the paper is organized as follows. Section 2 provides the analytical framework. Section 3 presents the materials and methods. Section 4 presents the results and discussion. Section 5 provides conclusions and implications.
2 Analytical framework
We referred to the study of Reddy (2018) and combined it with microeconomics theory to construct the theoretical analytical framework of this paper. We assume that farmers do not have preference differences in the choice of crop varieties and planting methods and allocate the scale of crop cultivation only with the decision-making objective of utility maximization (Luo and Qiu, 2018). FMOS can replace labor and adjust the structure of farmers’ production factor inputs while also impacting cropping structure. We can analyze it from supply and demand. First, the supply characteristics of FMOS guide the adjustment of agricultural planting structures. An imbalance of service categories mainly characterizes the current FMOS in China. The imbalance of service categories refers to the large gap between the FMOS levels of different types of crops, and the current FMOS service targets are mainly concentrated in grain crops such as wheat and rice. At the same time, there is a relative shortage of FMOS supply for economic crops such as fruit trees and vegetables, and the gap between the service levels of different types of crops is noticeable. The characteristics of the imbalance and inadequacy of the supply of FMOS have led farmers to plant crops that can deeply participate in the division of labor, and grain crops naturally become the priority. Crops and food crops naturally become the preferred option. Secondly, farmers’ demand for FMOS adjusts the agricultural planting structure (Yang et al., 2018). In China, if FMOS can save labor well, there is a higher demand for it from farmers. At this time, farmers can transfer more labor quantity and time to non-agricultural fields to get more wage income. Food production can satisfy this characteristic well, and farmers choose FMOS to be more willing to produce grain.
The restructuring of the cropping structure by FMOS can be realized through the following paths(see Figure 2). The ultimate goal of both paths is to increase agricultural productivity (Qiu and Tang, 2019). First, FMOS can ease labor constraints, reduce production costs, and improve agricultural productivity. Rising labor costs are an important factor in China’s agricultural production decisions. A large amount of labor is needed in food production, and the price of labor has risen in recent years. Farmers will produce cash crops to reduce production costs. However, the emergence of FMOS will reduce the labor shortage and lower the production cost. At this time, farmers will adjust the planting structure and choose food production again. Secondly, FMOS can reduce the yield loss in food production and improve the efficiency of agricultural production. Traditional grain harvesting relies on labor, at which time the harvesting loss is about 10%. The loss of harvesting will be reduced to less than 3% after the adoption of FMOS, which will bring more food income to farmers. Farmers are also willing to adjust their planting structure for grain production.
The subjective traits, behavioral capabilities, and cost constraints of small-scale and large-scale households are heterogeneous, leading to the tendency of small-scale farmers to choose FMOS. In contrast, large-scale households tend to purchase farm machinery (Hu et al., 2019). After comparing their opportunity costs, the market price of operation services, and the average cost of using farm machinery of different powers, farmers choose FMOS or purchasing their farm machinery within the scale interval (Zhang and Du, 2018). The capital threshold of agricultural machinery is generally higher. For land preparation machines, seeders, harvesters, dryers, and other such high acquisition costs of agricultural equipment, financial constraints of small farmers usually can not afford to buy, at the same time, the small scale of operation, land fragmentation will make the surplus of small agricultural machinery, large-scale agricultural machinery idle, so the small farmers to purchase their farm machinery is not consistent with maximizing returns, minimizing costs. They are more inclined to choose the FMOS. In contrast, large-scale households, driven by comparative advantage, purchase their farm machinery. Heterogeneity in the scale of farmers’ operation leads to different ways of investing in agricultural machinery, which has a differentiated impact on the adjustment of planting structures. For small-scale farmers, FMOS can significantly contribute to the adjustment of cropping structure towards grain cultivation. For large-scale households, the impact of FMOS on planting structure adjustment has yet to be apparent. Based on the above theoretical analyses, this paper proposes the following three research hypotheses:
H1: FMOS significantly positively affects the planting structure adjustment towards grain cultivation.
H2: FMOS promotes planting structure adjustment by enhancing grain production efficiency.
H3: The effect of FMOS on the planting structure adjustment has scale heterogeneity.
3 Materials and methods
3.1 Data
The data originated from a 2017 survey of wheat production in six counties of Henan Province of China. The mechanization level of wheat in Henan Province is rising. In 2017, the comprehensive mechanization rate of wheat production has reached about 80%, with most farmers adopting machinery, but focusing on land preparation and harvesting. We utilized a combination of stratified sampling and random sampling to facilitate sample selection. We selected Zhengyang County, Shangcai County, Qixian County, Maoyang County, Anyang County, and Xinan County for the questionnaire survey based on their geographic location, wheat planting area, per capita disposable income of farmers, and other relevant indicators. The survey was conducted in two batches. Firstly, 10 townships were randomly selected in Zhengyang County.
From each township, 5 villages were selected, and 40 sample households were randomly selected from each village, resulting in 2,000 samples. 1,914 valid questionnaires were recovered. The second time survey is founded on the principle of stratified sampling. We finally sampled five counties: Shangcai County, Qiyi County, Maoyang County, Anyang County, and Xin’an County. We divided all the townships in each county into quintiles according to their level of economic development. One township is chosen randomly from each quintile, obtaining five sample townships.
Similarly, all villages in the towns surveyed were split into two groups based on their economic status, and one village from each group was randomly chosen to form two sample villages. A total of 2,000 sample households were selected, with 40 households selected at random from each village. We conducted one-on-one household interviews by scheduling appointments in advance with sample households and village cadres in sample villages. Eventually, 2,000 valid questionnaires were obtained. The group distributed 4,000 questionnaires cumulatively to farmers, obtaining 3,914 valid samples, resulting in a questionnaire validity rate of 97.8%. In accordance with the research purpose of this paper, we ultimately selected 3,863 samples of farm households for the study.
3.2 Models
3.2.1 ERM model
This paper aims to investigate the effect of FMOS on cropping structure. However, the decision of farmers to adopt FMOS is influenced by unobservable factors, such as production preferences and skills (Silva et al., 2010; Mponela et al., 2023). To address the endogeneity problem caused by self-selection, ERM can provide more precise parameter estimates. The ERM model can handle both continuous and discrete endogenous variables. Therefore, the ERM model was chosen for regression, and the model was set as Equations 1,2:
In the equation, represents an individual farmer, represents whether farmer utilizes land preparation services, fertilizer application services, and harvesting services; Plastr, Instru, Farmer, Land, Reigon, and Price represent the variables of cropping structure, the average utilization rate of outsourced farm machinery services (instrumental variables), farmer characteristics, cropland characteristics, village characteristics, and market price, respectively. and represent the estimated coefficients of the corresponding variables. and are the stochastic perturbation terms. ERM is divided into two stages. The first stage involves the determination model of FMOS, while the second stage entails analyzing the impact of the FMOS model on cropping structure.
3.2.2 Mediation model
The mediation model provides a detailed explanation of the mechanism between two variables (Zhang and Du, 2018). To investigate the role of FMOS in planting structures, the following mediation model is constructed to examine the mechanism of action Equations 3–5.
Where Y denotes the cropping structure, Service and Media denote FMOS variables and grain production efficiency (mediating variable), respectively. X is the control variable that may affect both the cropping structure and the mediating variable, including farm household characteristics, cropland characteristics, village characteristics, and market price. We determine whether there is a mediating effect by comparing the size and significance of the estimated parameters of the three equations.
3.3 Variable definitions and descriptive statistics
3.3.1 Explained variables
This paper examines the intrinsic motivation for the change in planting structure. We selected the proportion of grain cultivation and the area of grain cultivation as the explained variables (Luo and Qiu, 2018). As one of the three significant grains, wheat’s planting scale is strategically essential to national food security. Thus, this paper takes wheat production as an example and selects wheat planting proportion and planting area as explained variables.
3.3.2 Core explanatory variables
Land preparation service, fertilizer application service, and harvesting service are FMOS commonly adopted by farmers (Qiu and Tang, 2019). In this paper, land preparation service, fertilizer application service and harvesting service are also selected as proxy variables of FMOS to ensure the reliability of the research findings.
3.3.3 Instrumental variables
Referring to the existing literature (Ma et al., 2013; Zhang et al., 2020), the average utilization rate of land preparation service, the average utilization rate of fertilizer application service, and the average utilization rate of harvesting service of other farmers in the village except this farmer are used as instrumental variables for whether this farmer uses land preparation service, fertilizer application service, and harvesting service. Due to the herd effect, the FMOS decisions of other farmers in the village will affect whether the farmer uses FMOS or not. However, they will not directly affect the farmer’s planting structure decision, so the average FMOS utilization rate of other farmers in the village satisfies the correlation and exclusion constraints required by the instrumental variables.
3.3.4 Mediating variables
FMOS combines technological improvement and labor substitution, leading to enhanced grain production efficiency (Yang and Li, 2022). This process aids in the adjustment of planting structure. This paper uses grain production efficiency as the mediating variable for FMOS to analyze how planting structure adjustment is achieved.
3.3.5 Control variables
Combined with the existing literature, other variables were further controlled for their effects on planting structure adjustment, such as farm household characteristics, cropland characteristics, village characteristics and market prices (Luo et al., 2018). The definitions of variables and descriptive statistics are shown in Table 1. In the survey sample, the average proportion of wheat planted by farmers was 13.4%, and the planted area was 7.838 mu. The average use rate of land preparation service was 74.9%; the average use rate of fertilizer application service was the lowest, 22.9%; and the average use rate of harvesting service was the highest, 84.4%, which indicated that the degree of mechanization of planting wheat was higher, and the application of mechanized ploughing and harvesting services was more widespread. The average yield of wheat per mu was 331.771 kg, and the average yield fluctuated wildly due to the influence of the scale of operation. The proportion of high school and above education reaches 17.1%; the proportion of farmers taking loans from formal financial institutions is deficient, only 3.1%; the sample farmers are generally of the big surnames in the village, indicating that the village residents mainly live centrally based on the family name as a link. The proportion of party members is relatively low, at 15.6%. The quality of arable land is average, while the average transportation conditions in the villages are average, and the percentage of those with farmers’ cooperative organizations is low at 8.2%. The market price of wheat is relatively stable, averaging 2.352 yuan/kg.
4 Results and discussion
4.1 ERM results
Table 2 shows that FMOS has a significant positive effect on adjusting planting structures. This suggests that farmers tend to cultivate grain after adopting FMOS. The findings of the study are consistent with Hua et al. (2022). Adoption of FMOS by farmers increases enthusiasm for grain cultivation. Chen et al. (2022) also showed that FMOS can increase the scale of grain production and thus grain productivity.Thus, hypothesis H1 is supported. Additionally, we examined the impact of FMOS adoption on different stages of planting structure. Regarding the proportion of grain planting, the harvesting service plays a more significant role in adjusting the planting structure than the land preparation service, with the fertilization service having the most negligible impact. The findings of this study are in basic consistency with those of Chen et al. (2012). Regarding the grain planting area, the fertilization service has a more significant impact on the planting structure, followed by the harvesting service, with minimal influence from the land preparation service (Zhang et al., 2022). The advancement of labor-intensive services, including land preparation and harvesting, and technology-intensive. A high labor substitution rate and high service quality characterize farmers’ demand for FMOS. A high labor substitution rate means that FMOS can cover as much of the whole process of crop growth as possible. The higher the labor substitution rate is, the more amount and time of labor farmers can transfer to the non-farming field, thus gaining more wage income. Relative to cash crops, food crops have fewer production stages and higher labor substitution rates. High service quality means that the quality of agricultural machinery socialization services can reach the satisfaction of farmers; at present, the agricultural machinery operation technology for staple food crops has been relatively mature, and there is a high degree of satisfaction in the process of its use by farmers (Qiu and Tang, 2019; Jiang et al., 2023). As a result, farmers will adjust their cropping structure to favor grain production after adopting FMOS. Such an adjustment holds high importance in securing the country’s grain supply.
4.2 Mediation effect results
As shown in Table 3, FMOS promotes the adjustment of cropping structure towards grain production by increasing production efficiency. Therefore, hypothesis H2 is confirmed. Specifically, the mediating effect of harvesting services on the share of grain crops and the area under grain crops was the strongest, with 0.102 and 0.812, followed by land preparation services, with 0.065 and 0.549, respectively. The mediating effect of fertilizer application services was the weakest, with 0.028 and 0.235, respectively. This was followed by fertilization services with 48.48 percent. Harvesting services had the lowest share, with 43.44%. Concerning the area under cereals, land preparation services had the largest share of mediated effects, with 69.10%. This was followed by harvesting services with 28.59%. Fertilizer application is the lowest, with 24.74%. In summary, labor-intensive FMOS has a more pronounced effect on the cropping structure adjustment by improving grain production efficiency.
4.3 Heterogeneity results of planting scale
This paper examines the impact of FMOS on adjusting planting structures while considering the dissimilar financial limitations, behavioral competencies, and subjective traits of small farming households and large-scale ones. This paper uses scale cut-offs of 5, 8, 10, and 20 mu, and farmers with crop areas equal to or greater than the cut-offs are classified as large households, while those with smaller areas are classified as small. Group regression analysis has been performed between FMOS and the proportion and area of cereal cultivation. Table 4 shows that FMOS has a scale heterogeneity effect on crop structure adjustment. Regarding the proportion of grain cultivation, the impact of FMOS on the planting structure adjustment of large-scale households is considerably less than that of small farmers. Regarding the area under food cultivation, we have not found FMOS to be statistically significant for the planting structure adjustment of large-scale households. Conversely, FMOS has a significant influence on the planting structure adjustment of smallholders. Therefore, Hypothesis H3 is tested. Land fragmentation is worse for small-scale producers in China. Small-scale farmers often give up growing food because of low income and insufficient labor. FMOS can pool dispersed land and operate it together, linking labor constraints and boosting food production. The lack of significant effect of FMOS on planting structure adjustment of large-scale households may be attributed to their relatively higher financial resources and behavioral capabilities, leading them to prefer self-purchasing farm machinery over using FMOS. It is suggested that the lack of significant effect of FMOS on planting structure adjustment of large-scale households may be attributed to their relatively higher financial resources and behavioral capabilities, leading them to prefer self-purchasing of farm machinery over using FMOS.
4.4 Robustness tests
This paper replaces the core explanatory variables to check for robustness. We use the number of FMOS to replace FMOS, subdivided into the level of service of land preparation, the level of service of fertilizer application, and the level of service of harvesting. We take the number of its adoption as a measure. As shown in Table 5, the number of FMOS significantly positively affects planting structure adjustment, which indicates the robustness of the previous regression results.
Similarly, we substitute the number of FMOS for FMOS and rerun the regression model for mediated effects. Table 6 shows a significant correlation between grain production efficiency and FMOS at the 1% statistical confidence level. FMOS facilitates structural adjustments in planting by improving grain production efficiency, which aligns with the results of the previous section’s mediated effect regression, thus demonstrating the robustness of the benchmark regression findings.
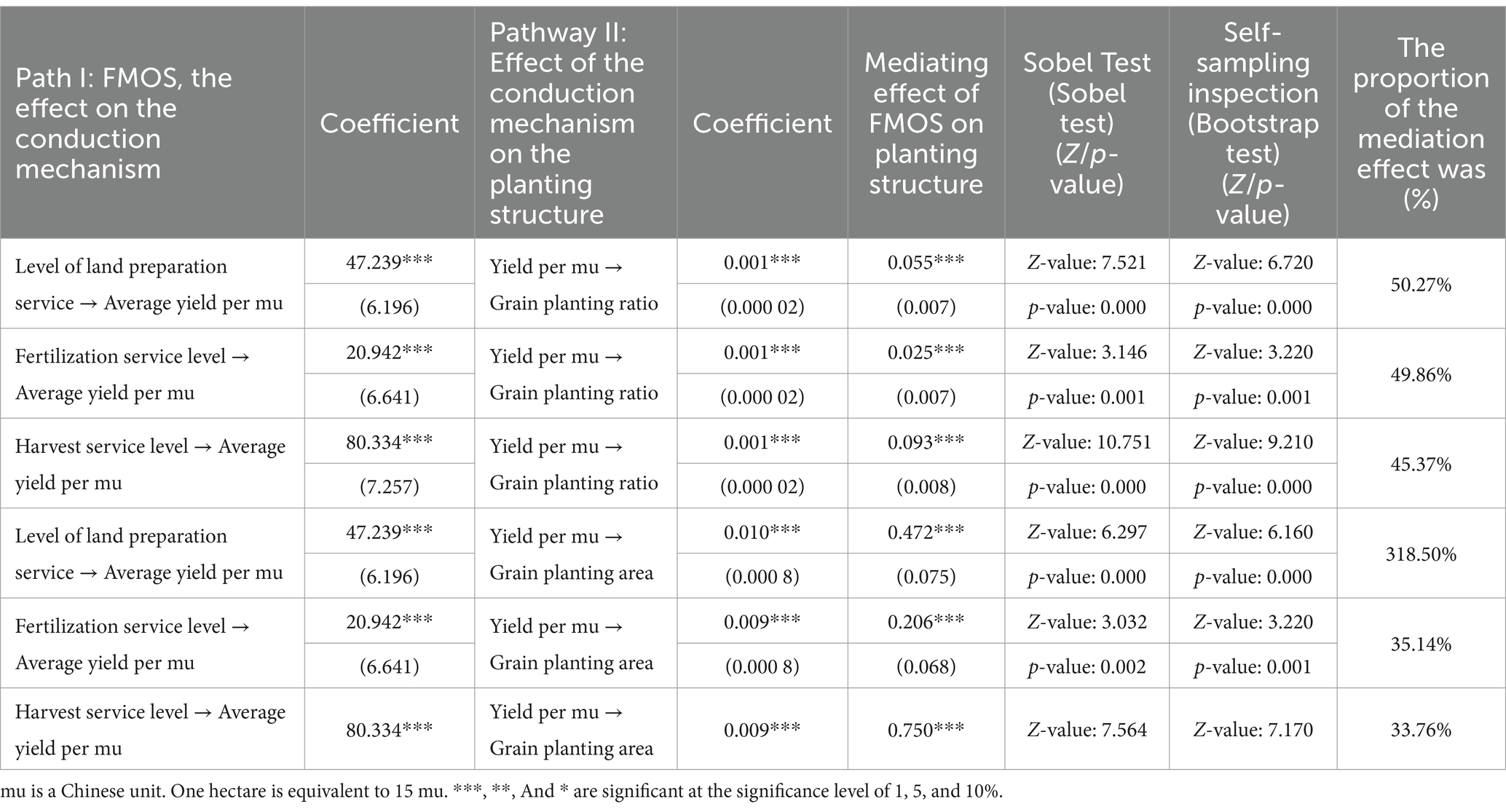
Table 6. Regression results of mediation effect of agricultural machinery outsourcing service level on planting structure.
We conducted a robustness test for the regression results of scale heterogeneity. Table 7 reveals that the impact of FMOS quantity on the proportion and area of grain cultivation displays scale heterogeneity. This outcome supports the previous benchmark regression results, indicating that the paper’s conclusions are robust. Regarding the percentage of grain cultivation, the impact of FMOS quantity on the planting structure adjustment for large-scale households is less significant than for small farmers. Additionally, the significance of FMOS quantity on the planting structure adjustment for large-scale households remains the same with the scale of operation expansion. Concerning the surface area used for food cultivation, an increase in the number of FMOS hinders the adjustment of cultivation structures in large-scale households. However, it still has a notable positive effect on the adjustment of cultivation structures in small-scale households.
4.5 Further discussion of scale heterogeneity
The regression results presented earlier indicate that FMOS does not significantly contribute to the planting restructuring of large-scale households. However, it does have a significant impact on small-scale farmers. This may be because larger farms prefer to purchase their farm machinery rather than rely on FMOS. To confirm this hypothesis, this paper examines the trend of self-purchase of farm machinery among large households. Taking the cultivation area for grain as an example, farmers’ purchased farm machinery can be categorized into land preparation, fertilizer application, and harvesting machinery. The level of farm machinery purchased by farmers is then used in comparative regression to ensure the accuracy of the regression results. The degree of farm machinery ownership signifies the proportion of work volume utilizing personal equipment in this production sector. The sector is categorized based on the degree of land preparation, fertilizer application, and harvesting equipment purchased by the producer. As shown in Table 8, the acquisition of farm machinery by large-scale households, whether self-purchased or not, significantly influences the adjustment of their planting structure. This finding contrasts the previous section’s insignificant effect of FMOS on the planting structure of large-scale households. Therefore, we have confirmed the presence of scale heterogeneity in the effect of FMOS on planting structures.
5 Conclusions and implications
5.1 Conclusion
In this paper, we use data from the 2017 questionnaire survey of wheat-producing farmers in China to empirically analyze the role mechanism of the impact of FMOS on planting structure adjustment using the ERM method and the mediated effects method and further examine the differences in the results under heterogeneity in the scale of operation of farmers. Our findings indicate that FMOS significantly positively affects the adjustment of cropping structure towards the grain. Among the various service segments, fertilizer application service significantly increased the area planted to grain.FMOS contributed to the cropping structure’s adjustment by enhancing grain production’s efficiency. The effect of FMOS on the adjustment of cropping structure was heterogeneous in terms of scale. For large-scale households, the effect of FMOS on planting structure adjustment is not apparent, but it has a significant positive effect on small-scale farmers. The main reason for this is that the financial rigidity constraints of large-scale households are weak, favoring the choice of self-purchasing farm machinery over FMOS.
5.2 Policy implications
The development of FMOS is of great importance for improving food security in developing countries. With the acceleration of the non-farm transfer process of rural labor, labor-intensive services such as land preparation and harvesting services are widely used in agricultural production. However, the popularity of technology-intensive services such as fertilizer application services must catch up. Fertilizer application services drive grain production efficiency, profoundly affecting planting structure adjustment. Therefore, when promoting FMOS, the government should focus on the coordinated development of various types of services, appropriately guide farmers to increase the use of fertilization services, set up particular subsidy policies, strengthen the subsidy, and build a two-way guarantee mechanism between the main body of the service supply and farmers.
FMOS only affects small farmers for planting structure adjustment and does not apply to large-scale households. For large-scale households, purchasing their farm machinery is more likely to prompt planting structure adjustment. In guiding farmers towards restructuring cultivation for grain production using FMOS, the government should differentiate between small-scale and large-scale farmers and adopt distinct subsidy policies. The government should prioritize providing subsidies for buying farm machinery for larger households. They should also direct them to purchase machinery instead of opting for FMOS. For smaller farmers, the government should provide subsidies for FMOS. This will enable them to participate in social services more and improve their production efficiency. It will also promote the adjustment of the cropping structure to grain production.
Data availability statement
The original contributions presented in the study are included in the article/supplementary material, further inquiries can be directed to the corresponding authors.
Author contributions
HQ: Formal analysis, Software, Validation, Conceptualization, Data curation, Funding acquisition, Investigation, Methodology, Project administration, Resources, Supervision, Visualization, Writing – original draft, Writing – review & editing. MF: Formal analysis, Validation, Writing – original draft. WT: Formal analysis, Writing – original draft. ML: Data curation, Methodology, Supervision, Writing – original draft, Writing – review & editing. JW: Project administration, Validation, Writing – review & editing. FY: Validation, Formal analysis, Software, Writing – review & editing.
Funding
The author(s) declare financial support was received for the research, authorship, and/or publication of this article. This research was funded by the National Social Science Foundation of China, grant number 22CGL027.
Acknowledgments
We would like to thank our colleagues for their support in data collection, field visits, and valuable thoughts in preparing this manuscript. And finally, the authors would like to thank the reviewers for their insightful comments. Any remaining errors are the responsibility of the authors.
Conflict of interest
The authors declare that the research was conducted in the absence of any commercial or financial relationships that could be construed as a potential conflict of interest.
Publisher’s note
All claims expressed in this article are solely those of the authors and do not necessarily represent those of their affiliated organizations, or those of the publisher, the editors and the reviewers. Any product that may be evaluated in this article, or claim that may be made by its manufacturer, is not guaranteed or endorsed by the publisher.
References
Akinbamowo, R. O. (2013). A review of government policy on agricultural mechanization in Nigeria. J. Agric. Ext. Rural Dev. 5, 146–153. doi: 10.5897/JAERD2013.0450
Cai, R., Chen, W., and Zhu, X. (2015). Cost benefit: internal factors and key solutions to the non grainification of farmland transfer. Rural Econ. 7, 44–49.
Chen, J., and Kong, X. (2016). The impact of land management scale on grain production: an analysis based on survey data of farmers in thirteen major grain producing areas in China. Hebei Acad. J. 36, 122–128.
Chen, C., Li, Y. Q., and Liao, X. Y. (2012). The productivity effect analysis of rice production outsourcing: based on the panel data of three counties in Jiangsu Province. China Rural Econ. 2, 86–96.
Chen, T., Rizwan, M., and Abbas, A. (2022). Exploring the role of agricultural services in production efficiency in Chinese agriculture: a case of the socialized agricultural service system. Land 11:347. doi: 10.3390/land11030347
Chiodi, V., Jaimovich, E., and Montes, R. G. (2012). Migration, remittances and capital accumulation: evidence from rural Mexico. J. Dev. Stud. 48, 1139–1155. doi: 10.1080/00220388.2012.688817
Fang, S., Wei, L., and Wu, J. (2017). Spatial spillover effects and distribution patterns of agricultural mechanization: a perspective on cross regional services of agricultural machinery. Manage. World 11, 65–78. doi: 10.19744/j.cnki.11-1235/f.2017.11.006
Godfray, H. C. J., Beddington, J. R., Crute, I. R., Haddad, L., Lawrence, D., Muir, J. F., et al. (2010). Food security: the challenge of feeding 9 billion people. Science 327, 812–818. doi: 10.1126/science.1185383
Hong, W., and Luo, B. (2019). The impact of security of agricultural land property rights on agricultural planting structure. J. Huazhong Agric. Univ. (Soc. Sci. Ed.) 3, 32–40. doi: 10.13300/j.cnki.hnwkxb.2019.03.005
Hu, W., Zhang, J., and Chen, Z. (2019). Small farmers and large production: land scale and agricultural capitalization - taking agricultural machinery operation services as an example. Agric. Technol. Econ. 6, 82–96. doi: 10.13246/j.cnki.jae.2019.06.006
Hua, Y., Xiaowei, L., and Mei, Z. (2022). Research on the influence of agricultural machinery socialization services on farmers’ enthusiasm for growing grain. J. Chin. Agric. Mechanization 43:199. doi: 10.13733/j.jcam.issn.2095-5553.2022.08.027
Huang, M., and Li, X. (2019). The inter provincial differential impact of rising agricultural labor prices on changes in crop planting structure. Econ. Geogr. 39, 172–182. doi: 10.15957/j.cnki.jjdl.2019.06.019
Huang, M., and Zou, Y. (2018). Integrating mechanization with agronomy and breeding to ensure food security in China. Field Crop Res. 224, 22–27. doi: 10.1016/j.fcr.2018.05.001
Ji, Y., Yu, X., and Zhong, F. (2012). Machinery investment decision and off arm employment in rural China. China Econ. Rev. 23, 71–80. doi: 10.1016/j.chieco.2011.08.001
Jiang, C. (2015). The impact of farmer differentiation on grain production and planting behavior choices and policy reflections. Theor. Explor. 1, 69–74. doi: 10.16354/j.cnki.23-1013/d.2015.01.015
Jiang, Y., Wang, X., Huo, M., Chen, F., and He, X. (2023). Changes of cropping structure lead diversity decline in China during 1985–2015. J. Environ. Manag. 346:119051. doi: 10.1016/j.jenvman.2023.119051
Kimura, S., Otsuka, K., Sonobe, T., and Rozelle, S. (2011). Efficiency of land allocation through tenancy markets: evidence from China. Econ. Dev. Cult. Chang. 59, 485–510. doi: 10.1086/649639
Li, G., Rozelle, S., and Brandt, L. (1998). Tenure, land rights, and farmer investment incentives in China. Agric. Econ. 19, 63–71. doi: 10.1111/j.1574-0862.1998.tb00515.x
Liu, Q., and Liu, P. (2013). The yield increasing effect of direct grain subsidies: an analysis based on panel data in Henan province. Econ. J. 3, 36–40.
Liu, J. C., Xu, Z. G., Zheng, Q. F., and Hua, L. (2019). Is the feminization of labor harmful to agricultural production? The decision-making and production control perspective. J. Integr. Agric. 18, 1392–1401. doi: 10.1016/S2095-3119(19)62649-3
Luo, B. (2019). From defining property rights to implementing property rights: the past and future of China's agricultural land management system reform. J. Agric. Econ. 1, 17–31. doi: 10.13246/j.cnki.iae.2019.01.002
Luo, B., and Qiu, T. (2018). Adjustment of agricultural planting structure in China: non grain oriented or grain oriented. Soc. Sci. Frontline 2, 39–51.
Luo, B., Zhang, L., and Qiu, T. (2018). The logic of small farmers' grain growing - the transformation of China's agricultural cultivation structure in the past 40 years and future strategies. South. Econ. 8, 1–28. doi: 10.19592/j.cnki.scje.361265
Lv, X., and Hu, X. (2017). Agricultural subsidies, non-agricultural employment, and grain production: based on research data from Heilongjiang, Jilin, Henan, and Shandong provinces. Agric. Econ. Issues 38, 85–91. doi: 10.13246/j.cnki.iae.2017.09.011
Ma, X., Heerink, N., Van, I. E., Van, D., and Shi, X. (2013). Land tenure security and land investments in Northwest China. China Agric. Econ. Rev. 5, 281–307. doi: 10.1108/17561371311331133
Mottaleb, K. A., Rahut, D. B., Ali, A., Gérard, B., and Erenstein, O. (2017). Enhancing smallholder access to agricultural machinery services: lessons from Bangladesh. J. Dev. Stud. 53, 1502–1517. doi: 10.1080/00220388.2016.1257116
Mponela, P., Manda, J., Kinyua, M., and Kihara, J. (2023). The impact of participatory action research and endogenous integrated soil fertility management on farm-gate dietary outputs in northern Tanzania. Heliyon 9, e21888–e21812. doi: 10.1016/j.heliyon.2023.e21888
Qi, Y., and Tang, C. (2017). The impact of rural labor force transfer on China's arable land planting structure. J. Agric. Eng. 3, 233–240. doi: 10.11975/j.issn.1002-6819.2017.03.032
Qian, L., Yuan, H., and Liu, J. (2018a). Analysis of the impact of agricultural land transfer on grain planting structure. Agric. Technol. Econ. 8, 63–74. doi: 10.13246/j.cnki.jae.2018.08.006
Qian, L., Yuan, H., and Liu, J. (2018b). Non agricultural employment, land transfer, and grain planting structure adjustment: a micro empirical study based on fixed observation points at the household level. Agric. Mod. Res. 39, 789–797. doi: 10.13872/j.1000-0275.2018.0070
Qiu, T., and Luo, B. (2018). What is the motive of "grain-oriented" planting structure? --based on the mechanism and empirical research of farmland property rights and factor allocation. China Rural Econ. 2, 65–80.
Qiu, H., and Tang, C. (2019). Can agricultural productive services promote income growth for farmers. J. Guangdong Univ. Finance Econ. 34, 100–112.
Reddy, A. A. (2018). Electronic national agricultural markets. Curr. Sci. 115, 826–837. doi: 10.18520/cs/v115/i5/826-837
Silva, G. O., Ortega, E. M., and Cancho, V. G. (2010). Log-Weibull extended regression model: estimation, sensitivity and residual analysis. Stat. Methodol. 7, 614–631. doi: 10.1016/j.stamet.2010.05.004
Springmann, M., Clark, M., Mason-D’Croz, D., Keith, W., Benjamin, L. B., Luis, L., et al. (2018). Options for keeping the food system within environmental limits. Nature 562, 519–525. doi: 10.1038/s41586-018-0594-0
Tan, Z., Hong, W., and Luo, B. (2019). Agricultural labor force transfer and "grain oriented" planting structure. Reformation 7, 111–118.
Tian, X., and Zhang, S. (2017). Changes in unit area profit and adjustment of grain planting structure in China. J. South China Agric. Univ. (Soc. Sci. Ed.) 16, 59–71. doi: 10.7671/j.issn.1672-0202.2017.06.007
Wang, Y., and Chen, Y. (2016). A study on the impact of labor migration on the planting structure of farmers: based on survey data from Jiangsu and Henan provinces. Econ. Issues 2, 41–48+111.
Wang, X., Yamauchi, F., and Huang, J. (2016). Rising wages, mechanization, and the substitution between capital and labor: evidence from small scale farm system in China. Agric. Econ. 47, 309–317. doi: 10.1111/agec.12231
Wei, L., Zhang, Y., and Gao, X. (2017). The impact of agricultural labor transfer on food security in China: an empirical analysis based on panel data. Econ. Issues Explor. 2, 160–167.
Wen, Y., Feng, L., and Liu, W. (2023). Labor transfer, market development, and the outsourcing of forestry production by farmers: a case study in Fujian, China. Front. Sustain. Food Syst. 7:1282444. doi: 10.3389/fsufs.2023.1282444
Xu, Y., Lyu, J., Xue, Y., and Liu, H. (2022). Does the agricultural productive service embedded affect farmers’ family economic welfare enhancement? An empirical analysis in black soil region in China. Agriculture 12:1880. doi: 10.3390/agriculture12111880
Xu, Z., Tan, X., and Zheng, X. (2017). The impact and constraints of agricultural land transfer market development on grain production. China Rural Econ. 9, 26–43.
Yang, S., and Li, W. (2022). The impact of socialized agricultural machinery services on land productivity: evidence from China. Agriculture 12:2072. doi: 10.3390/agriculture12122072
Yang, J., Wu, B., Jin, S., and Chen, Z. (2018). The impact of agricultural mechanization development on grain sowing area in China. China Rural Econ. 3, 89–104.
Yang, J., Zhong, F., and Chen, Z. (2016). The impact of changes in rural labor prices and population structure on grain planting structure. Manage. World 1, 78–87. doi: 10.19744/j.cnki.11-1235/f.2016.01.008
Ye, F., Qin, S., Nisar, N., Zhang, Q., Tong, T., and Wang, L. (2023). Does rural industrial integration improve agricultural productivity? Implications for sustainable food production. Front. Sustain. Food Syst. 7:1191024. doi: 10.3389/fsufs.2023.1191024
Zhang, Z., and Du, Z. (2015). Will land transfer necessarily lead to "non grainification" — empirical analysis based on monitoring data from 1740 farming households nationwide. Econ. Dynamics 9, 63–69.
Zhang, Z., and Du, Z. (2018). Economic analysis of agricultural productive service decision making - taking agricultural machinery operation service as an example. Finance Trade Econ. 39, 146–160.
Zhang, S., Hou, X., Wu, C., and Zhang, C. (2020). Impacts of climate and planting structure changes on watershed runoff and nitrogen and phosphorus loss. Sci. Total Environ. 706:134489. doi: 10.1016/j.scitotenv.2019.134489
Zhang, Q., Qu, X., and Wei, C. (2014). A study on the "non grainification" of family farms in the context of food security: a case study of 21 family farms in Wugang City, Henan Province. Southeast Acad. J. 3, 94–100. doi: 10.13658/j.cnki.sar.2014.03.013
Zhang, Q., Razzaq, A., Qin, J., Feng, Z., Ye, F., and Xiao, M. (2022). Does the expansion of farmers’ operation scale improve the efficiency of agricultural production in China? Implications for environmental sustainability. Front. Environ. Sci. 10:918060. doi: 10.3389/fenvs.2022.918060
Zhao, D., and Zhou, H. (2018). Research on changes in planting structure under the background of farmer differentiation: evidence from fixed observation points in rural areas of 31 provinces in China. Resour. Sci. 40, 64–73.
Zhong, F., Lu, W., and Xu, Z. (2016). Is it not conducive to grain production for rural labor to go out for work—— analysis of the behavior and constraints of farmer factor substitution and planting structure adjustment. China Rural Econ. 7, 36–47. doi: 10.18402/resci.2018.01.07
Zhou, J., and Zeng, F. (2019). Change or invariance: a study on the adjustment of planting structure of large rice farmers by lowering the minimum purchase price of grain. Agric. Econ. Issues 3, 27–36. doi: 10.13246/j.cnki.iae.20190318.001
Keywords: cropping structure, farm machinery outsourcing services, grain production, wheat farmers, China
Citation: Qiu H, Feng M, Tang W, Luo M, Wang J and Ye F (2024) Analysis of the impact of farm machinery outsourcing services on farmers’ cropping structure and grain production: an empirical evidence from Henan Province, China. Front. Sustain. Food Syst. 8:1365576. doi: 10.3389/fsufs.2024.1365576
Edited by:
Dingde Xu, Sichuan Agricultural University, ChinaReviewed by:
A. Amarender Reddy, National Institute of Agricultural Extension Management (MANAGE), IndiaSwamikannu Nedumaran, International Crops Research Institute for the Semi-Arid Tropics (ICRISAT), India
Zhang Shaoyao, Sichuan Normal University, China
Copyright © 2024 Qiu, Feng, Tang, Luo, Wang and Ye. This is an open-access article distributed under the terms of the Creative Commons Attribution License (CC BY). The use, distribution or reproduction in other forums is permitted, provided the original author(s) and the copyright owner(s) are credited and that the original publication in this journal is cited, in accordance with accepted academic practice. No use, distribution or reproduction is permitted which does not comply with these terms.
*Correspondence: Mingzhong Luo, luomingzhong@scau.edu.cn; Jiawei Wang, wang13319407091@126.com; Feng Ye, yexiwen1995@jxau.edu.cn