Optimization of watermelon waste as a bulking agent for sustainable co-composting of livestock manures using response surface methodology
- 1Department of Animal Science, Faculty of Agriculture, Universiti Putra Malaysia, Serdang, Selangor, Malaysia
- 2Department of Process and Food Engineering, Faculty of Engineering, Universiti Putra Malaysia, Serdang, Selangor, Malaysia
- 3Laboratory of Sustainable Animal Production and Biodiversity, Institute of Tropical Agriculture and Food Security, Universiti Putra Malaysia, Serdang, Selangor, Malaysia
Global human population growth has resulted in significant intensive agricultural activity, posing substantial challenges to waste management and environmental conservation. Watermelon waste (WW), chicken manure (CM) and horse manure (HM) are among the main contributors to agricultural waste due to their abundant waste production. This study aims to manage the daily production of these wastes by utilizing WW as a bulking agent in the co-composting of CM and HM. Response surface methodology (RSM) was employed to analyze the effects of four independent factors: HM:CM composition, particle size, composting period, and bulking agent amount. Thirty treatments were developed using central composite design and in-vessel composting reactors were employed to study the relationship between the factors involved and compost physicochemical quality parameters. The results demonstrated significant effects on organic matter (OM), nitrogen (N), potassium (K), dry matter, moisture content, bulk density, and pH, while the carbon-to-nitrogen ratio (C/N) and phosphorus level were not affected. The optimized co-composting conditions obtained from RSM were 75:25 for HM:CM composition (%), 0.5 cm for particle size, 40 days for composting period, and 10% of WW, resulting in a compost with 61% OM, 2.5% N, and 2.5% K. These optimal conditions agreed closely with the predicted values; root mean square prediction error (RMSPE) was less than 0.50, revealing the success of RSM in determining optimal process parameters and developing models for predicting responses. Our study demonstrated that WW as a bulking agent in the co-composting of CM and HM has significantly enhanced the organic matter and nutrient levels of the final compost product.
1 Introduction
Over recent years, fruits and vegetables exhibit the highest levels of wastage among all food categories (Sirohi et al., 2021). The Department of Agriculture Services Malaysia (2021) stated that watermelon stands as the fourth most produced fruit, followed by durian, pineapple, and banana. The production volume in the year of the report was over 210 thousand tons. Moreover, watermelon is widely cultivated in tropical and temperate regions, ranking second to banana as the most extensively produced fruit worldwide (Shahbandeh, 2022). Watermelon rind waste comprises the squishy white and tough green skin parts of the watermelon (Citrullus lanatus), excluding its red/pink flesh that is commonly consumed (Egbuonu, 2015). The rind constitutes approximately 30% of the total weight of the fruit (Kumar et al., 2012; Bhattacharjee et al., 2020). Due to the substantial weight of the rind, watermelon generates a significant amount of inedible waste per fruit, ranking second only to durian waste (Capossio et al., 2022; Chua et al., 2023). Unlike durian, this tropical fruit is consumed and produced throughout the year, growing at a faster pace without any seasonal variation. Therefore, the production of watermelon waste (WW) could be estimated to exceed that of durian waste. The rind contains not only all the nutrients found in the flesh, but also larger quantities of certain antioxidants, minerals, vitamins, and active ingredients (Bhattacharjee et al., 2020; Dubey et al., 2021).
Chicken and horse manures are valuable sources of organic matter, beneficial microorganisms, and plant nutrients commonly used to enhance agricultural crop yield (Keskinen et al., 2017; Yong-Hong et al., 2021). Chicken manure (CM) is widely known among farmers as “black gold” due to its high nitrogen-phosphorus-potassium (NPK) contents (Abumere et al., 2019). Chickens constitute 95% (approximately two billion birds) of the overall livestock production, making them the main contributors to livestock waste in Malaysia (Zayadi, 2021; Department of Veterinary Services Malaysia, 2022). Additionally, CM has also displayed the highest manure production growth in recent years among all livestock species worldwide (Food and Agriculture Organization of the United Nations, 2023). On the contrary, there were concerns about using CM as a plant fertilizer due to its low carbon-to-nitrogen ratio (C/N) (Oshins et al., 2022), which is unfavorable for nutrient mineralization and may hinder efficient microbial degradation during composting (Fuchs et al., 2018).
In comparison, horse manure (HM) relatively produces the largest volume of fresh manure per animal daily compared to other major livestock species (Teferra and Wubu, 2018). Horses require the highest amount of bedding compared to other livestock husbandry systems, discarding up to 9 kg of bedding materials per animal daily (Westendorf and Krogmann, 2013). When combined with horse excretion (23 kg/head) and residual feed, this could consequently result in more than 11,600 kg/head of waste annually (Komar et al., 2012). With over four thousand horses in Malaysia, approximately 46,000 tons of manure were produced in the year 2022 (Department of Veterinary Services Malaysia, 2022). These abundant manures are mixed with bedding materials such as sawdust, straw, and/or hay. Unfortunately, they are generally managed poorly and dumped aside, causing the spread of dust and aeroallergens. This poses potential risks of air pollution and respiratory health hazards (Tanner et al., 1998; Bambi et al., 2018). Additionally, HM has a significantly high carbon content, resulting in reduced nitrogen availability when applied to soil due to the immobilization of soluble nitrogen (Keskinen et al., 2017). As a result, HM is frequently considered less reliable for agricultural crop utilization when compared to CM.
Given the substantial production and negative issues associated with these wastes, many treatment alternatives through research have been proposed, including co-composting. Co-composting involves the simultaneous conversion of multiple feedstocks into nutrient-rich soil conditioners, producing higher-quality compost compared to mono-composting (Qian et al., 2014; Greff et al., 2022). Certain wastes may not be compostable as single raw materials due to deficiencies in their biological and/or physicochemical properties (Greff et al., 2022). In addition, co-composting enables the concurrent management and reutilization of more waste (Abu Qdais and Al-Widyan, 2016). The key to successful co-composting is achieving a balanced mix of organic matter that provides the necessary C/N (Oshins et al., 2022). Optimizing the composition and nutrient ratio through co-composting formulations can enhance the biodegradation process and result in products with innovative properties (Giagnoni et al., 2020). The co-composting of CM and HM offers complementary benefits, particularly in achieving a balanced C/N, given that CM is rich in nitrogen while HM is high in carbon. Additionally, WW as a bulking agent could further enhance the decomposition process and final compost quality, owing to its reportedly high moisture and nutrient levels (Huang et al., 2012; Rob, 2021).
This study manipulated the factors that influence the composting process, which are feedstock composition, particle size, composting period, and bulking agent amount (Bortolini et al., 2020; Wan et al., 2020). Composition manipulation is vital to determine the optimum C/N, which highly influences the final compost maturity (Oshins et al., 2022). Smaller particle sizes will increase the surface area, thus accelerating the microbial degradation process. However, particles that are too small may lead to challenges in handling and storage, while those that are overly large can result in unnecessary aeration and nutrient leaching (Sarlaki et al., 2021). Composting has traditionally been perceived as time-consuming by waste management stakeholders. But, it can be shortened by adjusting several parameters associated with the composting process (Sebaaly et al., 2018). The incorporation of bulking agents serves multiple purposes, including regulating moisture content and C/N, reducing ammonia volatilization, minimizing leachate production within the composting matrix, and ultimately enhancing the quality of the final compost (Meng et al., 2017; Lim et al., 2019).
Studies done on the co-composting of HM and CM as well as its impacts on physicochemical properties are scarce. Previously, CM and HM were co-composted using various types of green waste, sugar beet, and grape pomace as the bulking agent (Renčo et al., 2011; Weil et al., 2013; Liu Y. et al., 2018). However, studies on variations in physicochemical properties were only mentioned by Liu Y. et al. (2018). To date, no preceding studies have been conducted on the co-composting of CM and HM with WW as a bulking agent. Therefore, this study aims to address this gap, considering the substantial production and issues associated with these wastes. Concurrently, their influences on compost physicochemical quality parameters were assessed and optimized using Response Surface Methodology (RSM). This polynomial equation approach enables model development and the optimization of multiple factors affecting a single response (Homayoonfal et al., 2015). The objective is to simultaneously optimize feedstock composition, particle size, composting period, and bulking agent amount to achieve optimal compost performance (Wong et al., 2015; Zahmatkesh et al., 2022).
2 Materials and methods
2.1 Feedstock
Watermelon was purchased from the commercial market and the inedible parts (rind) were separated for this research. CM was obtained from the poultry breeding unit in Taman Pertanian, Universiti Putra Malaysia. Fresh HM (pure horse dung without bedding) was supplied by MAEPS Rubinga Equine Centre in Serdang, Malaysia. HM was dried under the sun for 2 to 3 days to remove excess moisture.
2.2 Experimental design
Central composite design (CCD) was employed, incorporating four independent variables: HM:CM composition (HM:CM), particle size (PS), composting period (CP), and bulking agent amount (BAA), each at five levels. This design was executed using Design Expert Software (Version 7, Stat-Ease), as shown in Table 1. The matrix consisted of 30 experiments, which comprised 24 factorial experiments and 6 central points. Multiple regression analysis was conducted on the response variable data, influenced by the independent variables. The polynomial regression equation obtained is depicted in Eq. 1;
Co-compost quality denoted as Y, serves as the response variable, encompassing organic matter (OM), nitrogen (N), C/N, phosphorus (P), potassium (K), dry matter (DM), moisture content (MC), pH, and bulk density (BD). C0 represents the constant of the model. The regression coefficients (C1, C2, C3, and C4), (C12, C13, C14, C23, C24, and C34) and (C11, C22, C33, and C44) respectively, represent the linear, interaction, and quadratic effects of the model. X1, X2, X3, and X4 are the values of the independent factors, namely HM:CM, PS, CP, and BAA, respectively. The term “e” denotes the random error of the model.
2.3 Co-composting procedure
Thirty compost reactors were prepared using a passive aerated system, following the in-vessel composting reactor design developed by Vincent Ling (2009). Each compost reactor was designed using a 5-liter rectangular plastic container with 14 perforated holes (0.5 cm diameter) on each wall and 8 perforated holes at the bottom to simulate natural ventilation. Apart from that, a one-foot of two-inch diameter polyvinyl chloride (PVC) pipe with 20 perforated holes (4 holes vertically) was inserted into the reactor, serving as a duct for central aeration. The feedstocks were prepared according to the pattern designated by RSM, as presented in Table 2. Manures were mixed based on the HM:CM composition creating 1 kg of total manure, while the amount of WW was determined as a percentage of the total manure in each reactor. After thorough mixing, all feedstocks were loaded into the compost reactors. These reactors were then positioned in the laboratory with minimal exposure to sunlight, spaced 5 cm apart from each other. The arrangement of rows was adjusted daily downward, with the reactors at the lowest row moved to the top to minimize the influence of ambient temperature. In addition, the composts were turned every 5 days to promote aeration. Moisture was controlled at 60–70% by spraying water into the reactors and monitored using a standard moisture meter. Temperature was also monitored by measuring with a bi-metal thermometer near the core of the pile.
2.4 Sampling and physicochemical analysis
Sampling was carried out on day 25, 30, 35, 40, and 45 (n = 30), according to the composting period (X3). Compost physicochemical quality parameters (OM, N, C/N, P, K, DM, MC, pH, and BD) were determined on the final day of the composting process. The gravimetric method was used to determine DM and MC by drying the compost sample (at 105°C for 24 h) and weighing moisture loss. pH was measured by using a digital pH meter, calibrated using pH4 and pH7. Organic carbon and organic matter (OM) contents were calculated as follows: Organic carbon (%) = OM (%)/1.8, OM (%) =100-Ash (%) (Adhikari et al., 2009; Sharma et al., 2018). The content of ash was determined as the difference between the dry matter content and the gravimetric loss-on-ignition produced by ashing the previously dried samples, at 550°C for 8 h (Hazarika and Khwairakpam, 2018; Jain et al., 2018). Total N (%) was determined by the Kjeldahl method using the FOSS Protein Analyzer for the distillation process. Total P and K contents were determined via extraction by single dry ashing method which is commonly used for organic materials. Blue color development (Blue Method) using a spectrophotometer was applied for P determination while K was determined by using Atomic Absorption Spectroscopy (AAS). BD was determined by dividing the mass of the sample by its volume.
2.5 Statistical analysis and RSM optimization
Data obtained from the laboratory procedures conducted were analyzed using RSM employed in Design Expert Software. From RSM, Analysis of Variance (ANOVA) results were calculated based on 95% confidence intervals. The models exhibited were considered reliable based on the following conditions: (1) the p-value of the model was lower than 0.05, (2) insignificant lack of fit test (p-value >0.05), (3) R2 adjusted was within 0.6–1.0 (Mohamad Mazlan et al., 2019). These conditions are necessary for designing spatial modeling and numerical optimization. The models selected were linear, two-factor interaction (2FI), and quadratic as suggested by the software. The fitted polynomial equation was expressed in the form of perturbation graphs for linear models and three-dimensional (3D) surface plots for 2FI and quadratic models. Coefficient estimates (CE) have positive and negative values that provide valuable information about the direction and magnitude of the relationships between the independent factors and the response variables (Pashaei et al., 2020). Response variables with significant models were optimized through numerical optimization by using the desirability function. Listed solutions with desirability at almost 1.000 or exactly 1.000 were selected as the optimized condition.
2.6 Validation of RSM models
The comparison between the predicted optimal values and the actual experimental values was essential for the validation of RSM models. The validation analysis was conducted using root mean square prediction error (RMSPE).
3 Results and discussion
3.1 Physicochemical properties analysis of feedstocks
The physicochemical properties of feedstock can influence the composting process and final compost quality. Table 3 presents the physicochemical properties of each feedstock utilized in this study. The physicochemical properties analysis revealed that HM had the highest OM content at 82.01% ± 0.09%, followed by WW (62.12% ± 1.93%), and CM (59.03% ± 1.1%). CM recorded the highest N content at 2.81% ± 0.15%, followed by WW (2.20% ± 0.07%), and HM (1.79% ± 0.02%). Additionally, CM displayed the highest DM content at 95.0% ± 1.41%, whereas WW had the lowest at 2.9% ± 0.15%. Contrastingly, WW exhibited the highest MC at 97.09% ± 0.15%, while CM had the lowest at 5.0% ± 1.41%.
Based on the results, HM exhibited an exceptionally high level of organic matter (>80%). This is likely attributed to horses’ heavy consumption of green roughage, which is the primary source of carbon. Carbon is the key component of organic matter (Adhikari et al., 2009; Sharma et al., 2018). Additionally, it was also proven that the animal’s diet significantly influenced the nutrient quality of manure (Hadin et al., 2016). CM demonstrated the lowest carbon content and the highest nitrogen content, resulting in the lowest C/N among the feedstocks tested. Conversely, HM had the highest C/N due to its high carbon and low nitrogen content. This study reveals that HM and CM offer complementary benefits by providing an optimal C/N in the compost mixture. The N content of WW was only marginally lower (0.6%) than that of CM, consistent with previous studies by Mo et al. (2016) and Romelle et al. (2016). CM reported a MC of only 5%, whereas earlier studies reported values ranging from 72.6 to 78.1% (Jifeng et al., 2017; Kong et al., 2018; Liu et al., 2019). This distinctive difference could be attributed to the fact that CM utilized in this study was dried litter stored in an empty barn for three to 4 months before collection.
3.2 Response surface analysis on physicochemical quality properties
The derived models for each response variable offer valuable insights into navigating the design space. Table 2 presents the experimental design and the corresponding nine response variables of physicochemical quality determined. The regression equations parameterized with independent factors for each significant response variable are shown in Eqs. 2–8:
Table 4 presents the predictability of the linear model that was applied to explain the effects of the independent factors on OM (Eq. 2), N (Eq. 3), and K (Eq. 4). Table 5 presents the predictability of the 2FI model to explain the interaction effects of DM (Eq. 5), MC (Eq. 6), and pH (Eq. 7). A quadratic model was employed for BD (Eq. 8), and the model’s predictability is shown in Table 6. Statistical analysis from ANOVA results indicated that the p-value for the “Lack of Fit test” was insignificant across all response variables, implying that the models were well-fitted to the data. All response variables, except for C/N and P, had p-values less than 0.05, suggesting their statistical significance. Thus, only significant variables will be discussed further. The coefficients of the polynomial equations were calculated based on experimental data to estimate the response variable values relative to the independent factors (Table 7).
3.2.1 Organic matter, nitrogen and potassium
Linear models were applied to describe the relationship of the independent factors on OM, N, and K. This model represents a first-order function and lacks curvature (Homayoonfal et al., 2015). OM is a crucial parameter for nutrient content and water retention, ultimately enhancing conditions for plant growth. In this study, OM was positively influenced at p < 0.05 by two linear effects, HM:CM [line A] and PS [line B] with CE = 7.53 and 1.81, respectively (Table 7). These effects are illustrated by a perturbation graph in Figure 1A, which depicts the positive influence of each independent factor on OM. From the graph, it can be observed that HM:CM displayed the steepest line, indicating a prominent influence of HM composition on OM compared to other independent variables. This observation aligns with the significantly high OM value in HM (82.01%) (Table 3).
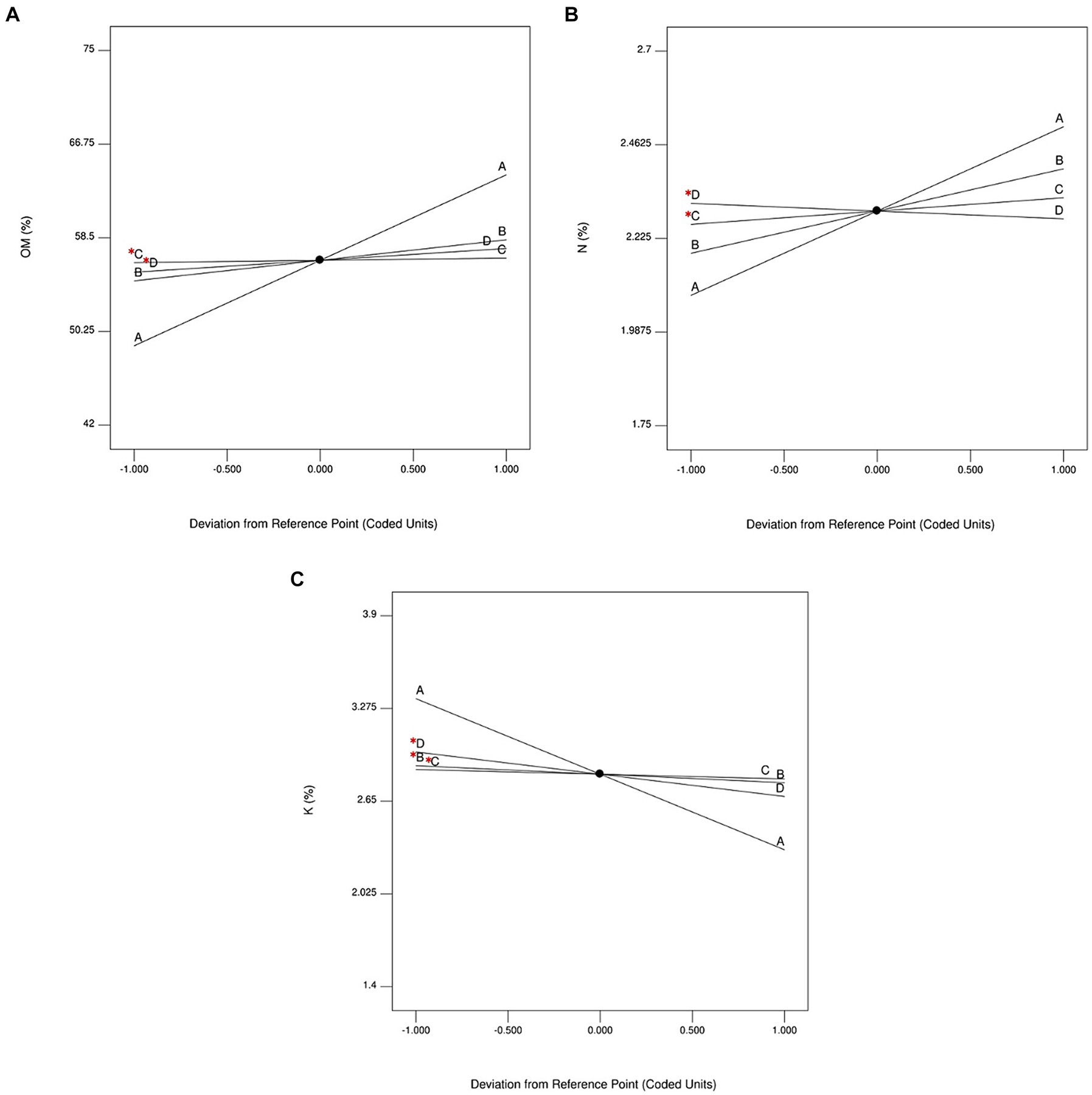
Figure 1. Perturbation graph displaying the linear effect of independent factors on response variables for HM and CM co-composting with WW as bulking agent. (A) OM (%); (B) N (%); (C) K (%). (A = HM:CM composition, B = particle size, C = composting period, D = bulking agent amount, Coded units = level of independent factors, * = non-significant variable).
The effect of PS on OM can be understood through a study by Lata Verma and Marschner (2013), who found that compost with smaller PS (<0.3 cm) boosted microbial biomass and P availability more than compost with larger PS (0.3–0.5 cm and > 0.5 cm). A similar study by Liu L. et al. (2018) stated that compost piles with PS <0.3 cm tend to have higher microbial propagation, enhancing OM mineralization. This is due to the high surface area-to-volume ratio and decomposability of compost with small particle sizes. In parallel, larger PS may have lower N and P contents than smaller PS due to the predominance of carbon-rich compounds (Tognetti et al., 2008). As proven by Duong et al. (2012), composts with smaller PS were found to release more N and P than composts with larger PS. However, findings from the current study indicated that larger particle sizes (0.75–1.25 cm) contain more OM than smaller particle sizes, contrary to the previous studies (Duong et al., 2012; Lata Verma and Marschner, 2013; Liu L. et al., 2018). Nevertheless, Lata Verma and Marschner (2013) noted that the differences among compost size fractions relative to their effect on soil nutrients were minimal. Correspondingly, the positive effect of PS on OM in this study was also small with CE = 1.81.
Nitrogen is essential for improving both the yield and quality of plants (Leghari et al., 2016). In this co-composting study, N was positively influenced at p < 0.05 by two linear effects, HM:CM [line A] and PS [line B] (Table 4). The effect of linear variables was illustrated in Figure 1B, indicating that N was slightly more sensitive to HM:CM than PS with a 0.1 difference in the CE values. The positive effect of HM:CM on N implies that increasing HM composition in the compost mixture would remarkably enhance N content of the final compost. Despite the lower N content of HM compared to CM (Table 3), the more balanced C/N attributed to higher HM composition in the co-compost mix ensured an adequate supply of degradable organic carbon and nitrogen for optimal mineralization. Thus, a substantial increase in N content was observed at the end of the composting process. Mishra and Yadav (2022) stated that N concentration generally increases at the end of the composting process due to the activity of nitrogen-fixing bacteria. The linear effect shows that an increase in PS would significantly enhance N release in the final compost. This finding was contradictory to the findings of Duong et al. (2012), who reported that N immobilization was often related to large compost particle sizes due to the smaller surface area for microbial activity.
Potassium is a crucial macronutrient that plays an essential role in the development of plants. The results showed that HM:CM [line A] was the sole independent factor that exhibited a significant effect on K at p < 0.0001 (Table 4), with CE = −0.51 (Table 7). The effect of HM:CM on K suggests that increasing HM composition in the compost would substantially decrease the K content. This relationship is illustrated by the evident negative gradient line observed in Figure 1C. This phenomenon could be explained by the low K content in HM, which was only 0.62% as compared to 1.94% in CM (Table 3). Therefore, as the proportion of HM increased, resulting in a decrease in CM composition in the co-compost mix, there was a corresponding reduction in K content. Other factors did not have any remarkable effect on K.
3.2.2 Dry matter and moisture content
Optimal dry matter and moisture levels are essential to facilitate sufficient microbial activity within and between the compost particles. These two parameters were explained using 2FI models, providing deeper insights into the interactions between the independent variables (Wong et al., 2015). DM and MC were significantly influenced at p < 0.05 (Table 5) by the linear effect of HM:CM, with CE = −3.05 (Table 7). This indicates that increasing HM composition in the co-compost mix would significantly reduce DM content while simultaneously increasing MC. The DM value of HM was significantly lower than CM (Table 3), resulting in a notable decrease in DM value in the co-compost mixture as the proportion of HM increases. Additionally, DM and MC were significantly influenced at p < 0.05 by two interaction effects, PS vs. CP and CP vs. BAA. Figure 2A presents the response surface plot illustrating the relationship between PS and CP at constant HM:CM (50:50) and BAA (15%). The plot indicates that higher DM content and lower MC were obtained under two conditions; smaller PS (0.25–0.35 cm) with longer CP and larger PS (1.1–1.25 cm) with shorter CP. This implies that composts with smaller particle sizes were better at providing moisture retention over longer CP than composts with larger particle sizes. This aligns with the findings of Głąb et al. (2020), who discovered that variations in water retention qualities could depend on the PS of compost. Larger compost particles led to decreased available water content due to their smaller surface areas, potentially hindering water absorption and disrupting the activity of microorganisms involved in organic matter decomposition.
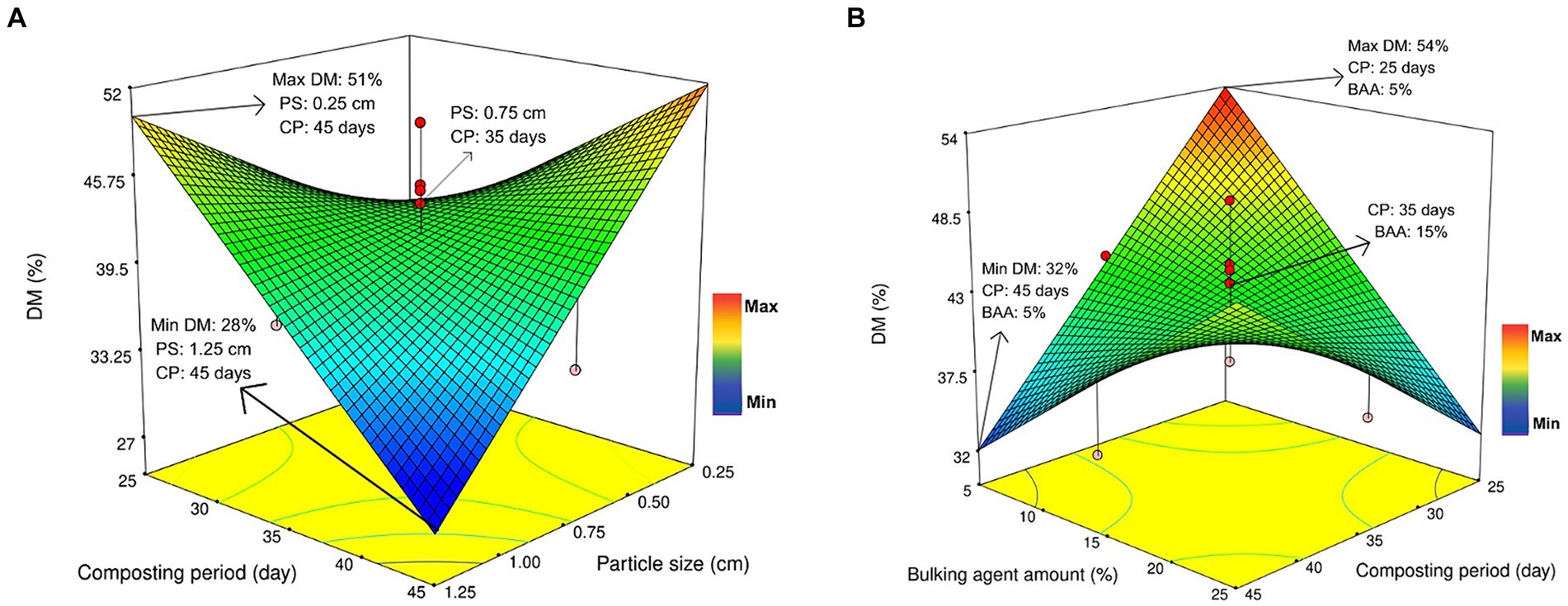
Figure 2. Response surface plots for the effect of independent variables on DM (%) and MC (%) of HM and CM co-compost with WW as bulking agent. (A) PS vs. CP; (B) CP vs. BAA.
The 3D response surface plot depicted in Figure 2B illustrates the interaction effect of CP and BAA on DM content, with HM:CM (50:50) and PS (0.75 cm) held constant. Variations in DM content were primarily influenced by the moisture retention ability of individual feedstock. Maximum DM (54%) and minimum MC (46%) were achieved at the lowest level of BAA (5–10%) of WW and the minimum level of CP (25–30 days). WW has a significantly high moisture content and retention ability, which is attributed to its rich concentration of fiber components (Hoque and Iqbal, 2015). Thus, a low amount of WW in the co-compost mix could contribute to reduced moisture-holding capacity, resulting in high DM content in the final compost. Shorter CP (25–30 days) has led to a higher proportion of undecomposed organic matter and therefore higher DM content due to limited time for microbial decomposition. Hence, the combination of lower BAA and shorter CP resulted in higher DM content in the final compost.
3.2.3 pH
Compost pH is a measure of hydrogen ion activity influenced by various chemical factors, including the mix and concentrations of cations and anions in the solution (Smith and Doran, 1997; Butterly et al., 2013). This response variable was explained by the 2FI model. The linear effect indicates that increasing HM proportion significantly reduces pH value, with CE = −0.31 (Table 7). This trend is displayed in Figure 3A, where the highest pH value is observed at the highest CM composition and smallest PS. This result is valid as CM was significantly more alkaline than HM based on previous studies. HM had a mean pH of 6.7 (Hanc et al., 2019; Stepanova et al., 2021), while CM had a mean pH of 7.9 (Li et al., 2017; Kong et al., 2018; Qasim et al., 2019). The high N content in both CM (2.81%) and WW (2.20%) have contributed to low C/N (Table 3), resulting in higher pH values due to the degradation of nitrogen-containing compounds accumulated in the composting piles (Yu et al., 2019; Wan et al., 2020).
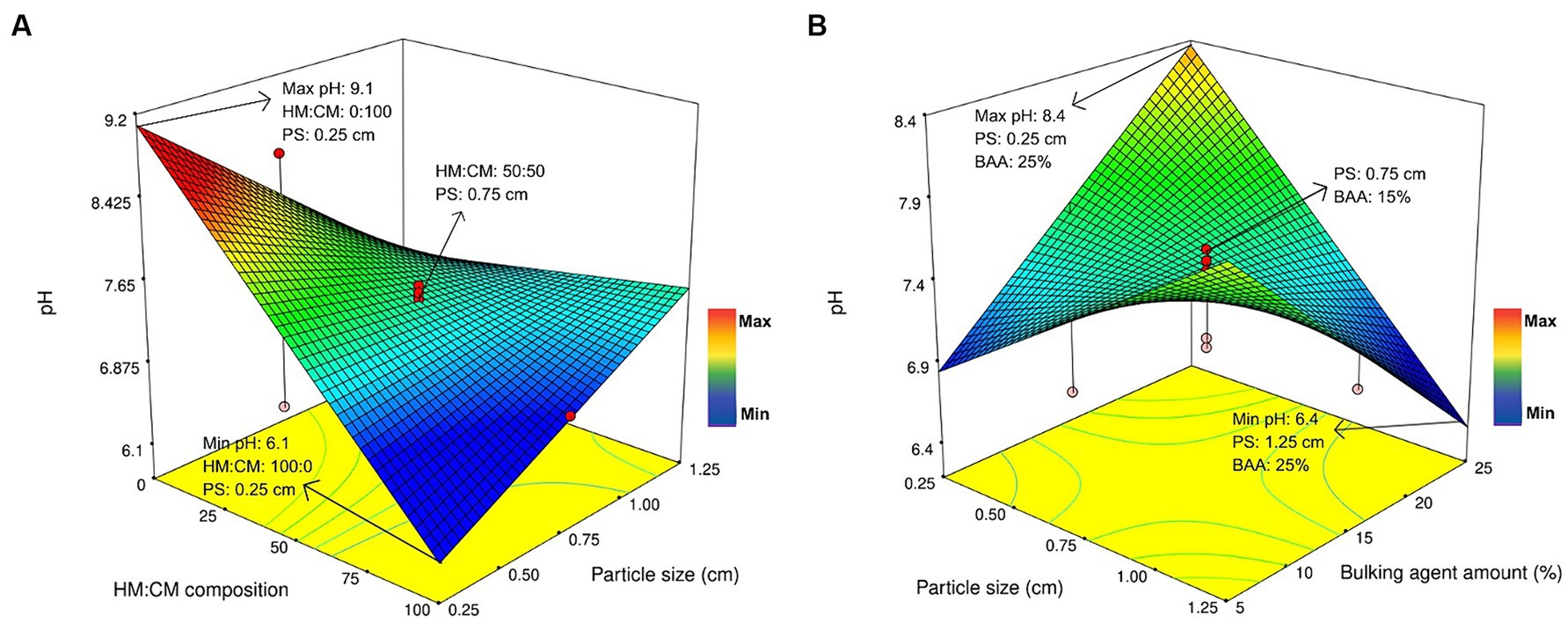
Figure 3. Response surface plots for the effect of independent variables on pH of HM and CM co-compost with WW as bulking agent. (A) HM:CM vs. PS; (B) CP vs BAA to PS vs BAA.
In addition, the impact of PS on pH observed in this study was in agreement with the findings of Zhao et al. (2012). They reported a reduction in pH value as PS decreases. This might be due to the increase in cation adsorption capacity resulting from the larger surface areas exhibited by smaller particle sizes. Consequently, more positively charged ions may bind to the particle surfaces, leading to a decline in cation concentration in the compost. Thus, the pH value of compost tends to decrease, becoming more acidic. Figure 3B illustrates the interaction of PS and BAA, showing that pH is lowest at PS of 0.25 cm and 5% of BAA. These represent the lowest levels for each independent factor. However, the pH value peaks at the same PS level and 25% of BAA, indicating that a higher amount of WW induced a higher pH value.
3.2.4 Bulk density
Effective control of bulk density can enhance the permeability, free air space, oxygen utilization efficiency, and heat transfer in the composting mix. The quadratic model, with its highest order polynomial, showing the significance of additional terms (Wong et al., 2015), was applied to explain BD response. The surface plot in Figure 4A demonstrates the effects of HM:CM and PS on BD, while CP was held constant at 35 days and BAA at 15%. BD was significantly influenced by two linear effects (HM:CM and CP) and three quadratic effects (HM:CM2, PS2, and BAA2), with CE values equal to −36.48, 23.42, 19.27, 23.81, and 13.62, respectively (Table 7). It was observed that maximum BD was achieved at low HM composition (0–25%) across all PS levels. HM:CM exerted a significant negative influence on BD (CE = −36.48). Moisture content, porosity, particle size, and the density of the individual particles are the main factors influencing BD (Jain et al., 2019; Oshins et al., 2022). Therefore, it can be concluded that HM has lower moisture retention and density but higher porosity compared to CM, which explains the decrease in BD as HM composition increases in the co-compost mix.
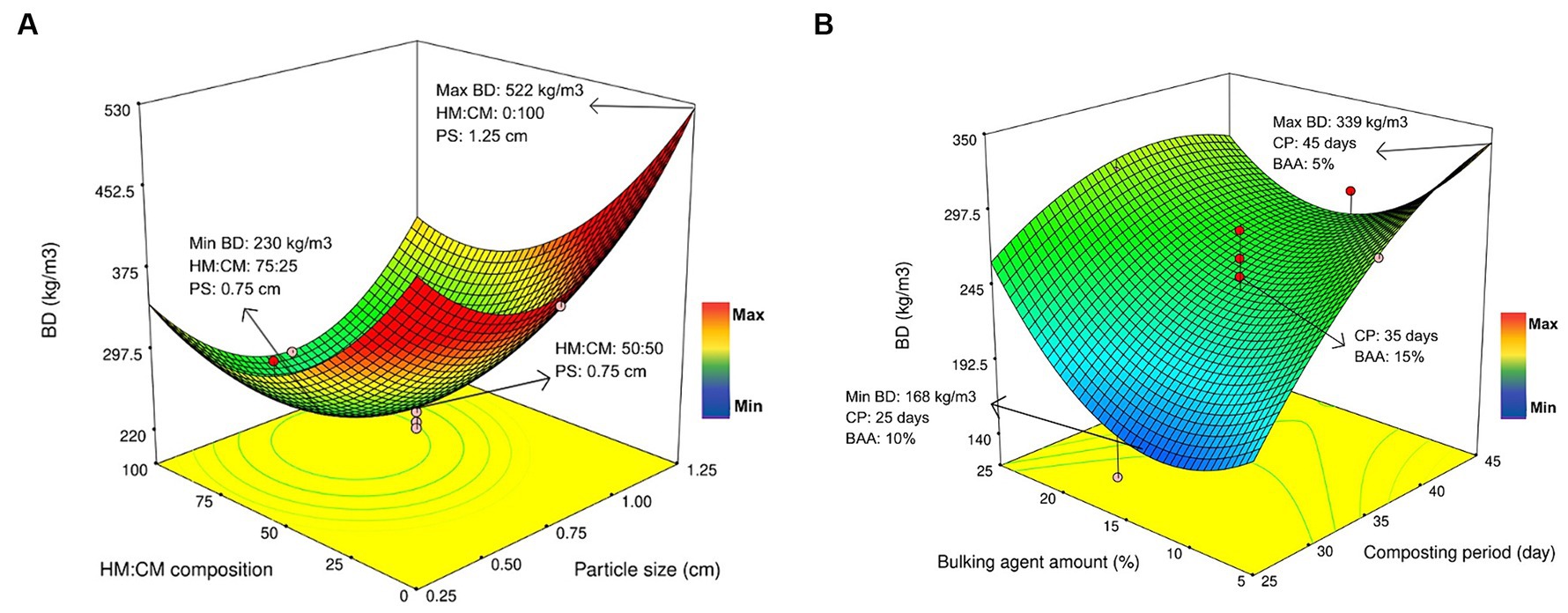
Figure 4. Response surface plots for the effect of independent variables on BD (kg/m3) of HM and CM co-compost with WW as bulking agent. (A) HM:CM vs. PS; (B) CP vs. BAA.
The quadratic effects of HM:CM, PS, and BAA on BD of the co-compost are illustrated in Figures 4A,B. BD reaches its peak at 365–486 kg/m3 with HM:CM ranging from 0:100 to 25:75, while the lowest point was at 50:50 to 75:25, resulting in a BD of 230–280 kg/m3. This highlights the preference for lower HM composition in the co-compost to achieve higher BD. This finding aligns with the statement made by Smith and Swanson (2009) regarding the low bulk density of HM, primarily attributed to the significant presence of bedding material. However, since our study utilized pure HM without bedding, the results suggest that horse dung has lower bulk density compared to CM.
The optimal range of particle sizes was presented by the quadratic relationship. PS ranging from 0.50 to 1.0 cm produced co-compost with lower BD values, whereas BD gradually increases with PS below 0.50 cm and above 1.0 cm. Excessively small particle sizes may lead to compression, potentially increasing moisture retention in the compost pile and consequently elevating BD values (Oshins et al., 2022). Conversely, excessively large particle sizes could increase the mass per particle, thereby increasing the overall mass of the compost pile per unit volume and resulting in higher BD values. This could lead to slower decomposition rates during the composting process. Furthermore, the highest level of BAA at 20–25% exhibits better BD values (>360 kg/m3), nearly reaching the suggested range of 400–600 kg/m3 by Oshins et al. (2022). This indicates the efficacy of WW as a bulking agent in the co-composting of HM and CM. These findings emphasize the significant effects of feedstock type and particle size on compost bulk density.
3.3 Numerical optimization and validation experiment
The purpose of optimization was to identify the optimal combination of independent factors that leads to the desired response or outcome. In this study, independent factors were optimized to achieve OM levels falling within the range of 40–60% (Ozores-Hampton, 2017), while maximizing N and K levels. These elements are the key parameters influencing the value of compost as a soil amendment.
The outcome of the simultaneous optimization suggested that the optimal co-composting conditions were achieved at HM:CM of 75:25, PS of 0.5 cm with 40 days of CP, and 10% of WW as the bulking agent. These conditions yielded the most favorable result, with 61% OM, 2.5% N, and 2.5% K. The optimization results reveal that co-composting HM and CM with WW as the bulking agent produced compost of desirable quality, with remarkably high levels of organic matter and nutrients. These findings align with compost quality standards recommended by Nyi et al. (2017) and Heyman et al. (2019).
For validation purposes, the co-composting process was repeated using the optimal conditions identified. Table 8 shows the comparison between the predicted and experimental values. RMSPE analysis revealed that the predicted optimal response variables closely matched the experimental values, with error values consistently below 0.50. This validation demonstrates the success of RSM and CCD in finding the best process parameters and developing models for predicting responses.
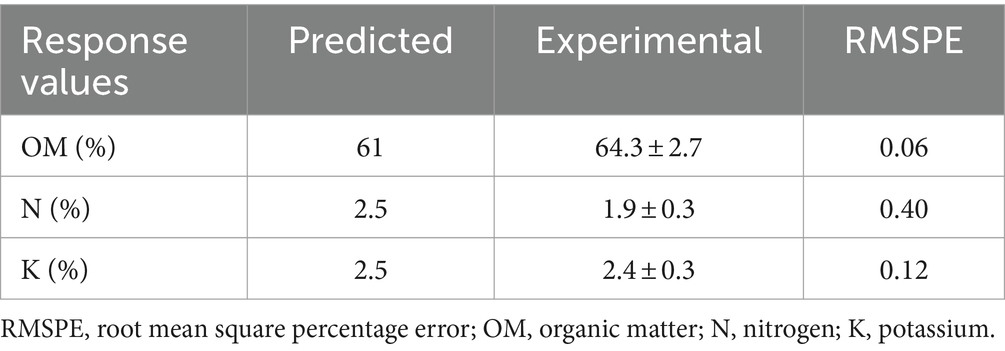
Table 8. Comparison between the predicted and experimental values for HM and CM co-composting with WW as bulking agent.
4 Overview of the co-composting process
The summary statistics of co-composting CM and HM with WW as bulking agent is presented in Table 9. The results show significant effects on OM, N, K, DM, MC, pH, and BD. Results from the thirty experimental runs show that OM exhibited a mean of 58.82%, which is within the desirable range of 40–60% for most compost applications, as suggested by Ozores-Hampton (2017). As for N and K values, there are currently no globally agreed-upon ideal range values. However, many farmers believe that higher nutrient values contribute to better soil fertility and plant productivity. The average values for N (2.29%) and K (2.83%) were notably high, suggesting that this co-composting combination was proven to significantly increase the nutrient content of the final compost. The average values for DM (41.64%) and MC (58.36%) fell within the ideal range of 40–60%, as recommended by Singh and Kalamdhad (2015). This might be owing to the moisture retention ability of WW as the bulking agent.
The average BD value from the experimental runs was 265.65 kg/m3, remarkably lower than the suggested range of 400–600 kg/m3 (Oshins et al., 2022). This discrepancy could be attributed to the high moisture content and low porosity of WW, as bulk density is heavily influenced by these two parameters (Jain et al., 2019; Oshins et al., 2022). Most composts tend to maintain a neutral to slightly alkaline pH range, with the optimal pH range being between 6.5 and 8.0 (Ho et al., 2022; Oshins et al., 2022). The average pH value exhibited was 7.73, showing a considerably good pH level for compost application.
However, the co-composting of HM and CM with WW as bulking agent did not significantly affect C/N and P content. The average C/N observed was 13.73, significantly lower than the recommended range of 20–40 (Guo et al., 2022; Oshins et al., 2022). This could be attributed to the low C/N of CM (11.7) and WW (15.57), as shown in Table 3. This co-composting mix did not contribute to achieving a balanced C/N, which could affect the mineralization rate and cause nitrogen loss as ammonia to the environment (Oshins et al., 2022). Therefore, the choice of feedstock is crucial given its substantial influence on the physicochemical properties of compost.
5 Comparison with previous studies
There are very limited resources on previous co-composting studies involving HM and CM. Sokri et al. (2023) utilized RSM optimization to compost fresh HM with pineapple waste as the bulking agent. Their study identified the optimal composition to be 95% HM and 5% pineapple waste, resulting in compost with 90.3% OM. As a comparison, the current study uses 10% WW, demonstrating the potential to manage greater volumes of fruit waste for nutrient reutilization.
Another study by Liu Y. et al. (2018) achieved a compost with 35.9% OM and 2.3% N by co-composting mature HM and fresh CM, utilizing green waste as the bulking agent. While composting essentially involves organic matter degradation, it is recommended to maintain OM levels at 40–60% for effective agricultural application (Ozores-Hampton, 2017). The optimal OM content in this study (61%) aligns closely with this range compared to the findings of Sokri et al. (2023) and Liu Y. et al. (2018), which were 90.3 and 35.9% and, respectively. Moreover, the N content achieved under the optimized conditions surpassed that of the study by Liu Y. et al. (2018). This suggests that co-composting HM and CM with WW as a bulking agent proved more effective in achieving adequate degradation while preserving organic matter and nutrient content in the final compost.
6 Conclusion
RSM and CCD proved effective in determining optimal process parameters and creating models for predicting responses in this study. Co-composting HM and CM with WW as the bulking agent significantly influenced OM, N, K, DM, MC, pH, and BD, while showing no significant effects on C/N and P. Higher HM composition in the co-compost mix led to higher OM, N, and MC values, while decreasing K, DM, BD, and pH in the final compost. Larger particle size resulted in higher OM and N values while a longer composting period encouraged higher bulk density of compost pile. Optimal conditions derived from RSM optimization were HM:CM of 75:25, particle size of 0.5 cm, 40 days of composting, and 10% of WW as the bulking agent, yielding a compost with 64.3 ± 2.7% of OM, 1.9 ± 0.3% of N and 2.4 ± 0.3% of K. The findings showed that co-composting HM and CM with WW as a bulking agent enhanced the organic matter and nutrient levels of the final compost. However, this combination has led to an imbalanced C/N, potentially hindering successful nutrient mineralization and reducing plant nutrient absorption.
Data availability statement
The raw data supporting the conclusions of this article will be made available by the authors, without undue reservation.
Author contributions
AN: Formal analysis, Investigation, Methodology, Software, Validation, Visualization, Writing – original draft, Writing – review & editing. TT: Conceptualization, Data curation, Formal analysis, Funding acquisition, Investigation, Methodology, Project administration, Resources, Supervision, Validation, Visualization, Writing – review & editing, Writing – original draft. NC: Data curation, Resources, Supervision, Writing – review & editing. MZ: Funding acquisition, Resources, Supervision, Writing – review & editing. NN: Supervision, Writing – review & editing.
Funding
The author(s) declare financial support was received for the research, authorship, and/or publication of this article. The authors gratefully acknowledge the financial support from UPM Putra Grant – Inisiatif Putra Siswazah Grant (GP-IPS/2022/9722500) and a Graduate Research Fellowship (GRF), both under UPM for the scholarship.
Conflict of interest
The authors declare that the research was conducted in the absence of any commercial or financial relationships that could be construed as a potential conflict of interest.
Publisher’s note
All claims expressed in this article are solely those of the authors and do not necessarily represent those of their affiliated organizations, or those of the publisher, the editors and the reviewers. Any product that may be evaluated in this article, or claim that may be made by its manufacturer, is not guaranteed or endorsed by the publisher.
References
Abu Qdais, H., and Al-Widyan, M. (2016). Evaluating composting and co-composting kinetics of various agro-industrial wastes. Int. J. Recycl. Org. Waste Agric. 5, 273–280. doi: 10.1007/s40093-016-0137-3
Abumere, V. I., Dada, O. A., Adebayo, A. G., Kutu, F. R., and Togun, A. O. (2019). Different rates of chicken manure and NPK 15−15-15 enhanced performance of sunflower (Helianthus annuus L.) on ferruginous soil. Int. J. Agron. 2019, 1–10. doi: 10.1155/2019/3580562
Adhikari, B. K., Barrington, S., Martinez, J., and King, S. (2009). Effectiveness of three bulking agents for food waste composting. Waste Manag. 29, 197–203. doi: 10.1016/j.wasman.2008.04.001
Bambi, G., Rossi, G., and Barbari, M. (2018). Comparison between different types of bedding materials for horses. Agron. Res. 16, 646–655. doi: 10.15159/AR.18.124
Bhattacharjee, C., Dutta, S., and Saxena, V. K. (2020). A review on biosorptive removal of dyes and heavy metals from wastewater using watermelon rind as biosorbent. Environ. Adv. 2:100007. doi: 10.1016/j.envadv.2020.100007
Bortolini, S., Macavei, L. I., Saadoun, J. H., Foca, G., Ulrici, A., Bernini, F., et al. (2020). Hermetia illucens (L.) larvae as chicken manure management tool for circular economy. J. Clean. Prod. 262:121289. doi: 10.1016/j.jclepro.2020.121289
Butterly, C. R., Baldock, J. A., and Tang, C. (2013). The contribution of crop residues to changes in soil pH under field conditions. Plant Soil 366, 185–198. doi: 10.1007/s11104-012-1422-1
Capossio, J. P., Fabani, M. P., Román, M. C., Zhang, X., Baeyens, J., Rodriguez, R., et al. (2022). Zero-waste watermelon production through nontraditional rind flour: multi objective optimization of the fabrication process. PRO 10:1984. doi: 10.3390/pr10101984
Chua, J. Y., Pen, K. M., Poi, J. V., Ooi, K. M., and Yee, K. F. (2023). Upcycling of biomass waste from durian industry for green and sustainable applications: an analysis review in the Malaysia context. Energy Nexus 10:100203. doi: 10.1016/j.nexus.2023.100203
Department of Agriculture Services Malaysia (2021). Fruit Crops Statistics. Available at: http://www.doa.gov.my/index/resources/aktiviti_sumber/sumber_awam/maklumat_pertanian/perangkaan_tanaman/statistik_tanaman_buah_2021.pdf (Accessed December 10, 2023).
Department of Veterinary Services Malaysia (2022). 2021/2022 Livestock Statistics. Available at: https://www.dvs.gov.my/dvs/resources/user_1/2022/BPSPV/Perangkaan%202021.2022/1)_Buku_Perangkaan_Ternakan_2021_2022_Keseluruhan.pdf (Accessed November 15, 2023)
Dubey, S., Rajput, H., and Batta, K. (2021). Utilization of watermelon rind (Citrullus lanatus) in various food preparations: a review. J. Agric. Sci. Food Resour. 12, 1–3. doi: 10.37273/chesci.cs205205361
Duong, T. T., Penfold, C., and Marschner, P. (2012). Differential effects of composts on properties of soils with different textures. Biol. Fertil. Soils 48, 699–707. doi: 10.1007/s00374-012-0667-4
Egbuonu, A. C. C. (2015). Comparative investigation of the proximate and functional properties of watermelon (Citrullus lanatus) rind and seed. Res. J. Environ. Toxicol. 9, 160–167. doi: 10.3923/rjet.2015.160.167
Food and Agriculture Organization of the United Nations (2023). Statistical yearbook: world food and agriculture. Available at: https://www.fao.org/3/cc8166en/cc8166en.pdf (Accessed February 15, 2024).
Fuchs, W., Wang, X., Gabauer, W., Ortner, M., and Li, Z. (2018). Tackling ammonia inhibition for efficient biogas production from chicken manure: status and technical trends in Europe and China. Renew. Sust. Energ. Rev. 97, 186–199. doi: 10.1016/j.rser.2018.08.038
Giagnoni, L., Martellini, T., Scodellini, R., Cincinelli, A., and Renella, G. (2020). “Co-composting: an opportunity to produce compost with designated tailor-made properties” in Organic waste composting through nexus thinking. Eds. H. Hettiarachchi., S. Caucci., K. Schwärzel (Cham: Springer), 185–211.
Głąb, T., Żabiński, A., Sadowska, U., Gondek, K., Kopeć, M., Mierzwa-Hersztek, M., et al. (2020). Fertilization effects of compost produced from maize, sewage sludge and biochar on soil water retention and chemical properties. Soil Tillage Res. 197:104493. doi: 10.1016/j.still.2019.104493
Greff, B., Szigeti, J., Nagy, Á., Lakatos, E., and Varga, L. (2022). Influence of microbial inoculants on co-composting of lignocellulosic crop residues with farm animal manure: a review. J. Environ. Manag. 302:114088. doi: 10.1016/j.jenvman.2021.114088
Guo, H. N., Liu, H. T., and Wu, S. (2022). Immobilization pathways of heavy metals in composting: interactions of microbial community and functional gene under varying C/N ratios and bulking agents. J. Hazard. Mater. 426:128103. doi: 10.1016/j.jhazmat.2021.128103
Hadin, Å., Eriksson, O., and Hillman, K. (2016). A review of potential critical factors in horse keeping for anaerobic digestion of horse manure. Renew. Sust. Energ. Rev. 65, 432–442. doi: 10.1016/j.rser.2016.06.058
Hanc, A., Enev, V., Hrebeckova, T., Klucakova, M., and Pekar, M. (2019). Characterization of humic acids in a continuous-feeding vermicomposting system with horse manure. Waste Manag. 99, 1–11. doi: 10.1016/j.wasman.2019.08.032
Hazarika, J., and Khwairakpam, M. (2018). Evaluation of biodegradation feasibility through rotary drum composting recalcitrant primary paper mill sludge. Waste Manag. 76, 275–283. doi: 10.1016/j.wasman.2018.03.044
Heyman, H., Bassuk, N., Bonhotal, J., and Walter, T. (2019). Compost quality recommendations for remediating urban soils. Int. J. Environ. Res. Public Health 16:3191. doi: 10.3390/ijerph16173191
Ho, T. T. K., Le, T. H., Tran, C. S., Nguyen, P. T., Thai, V. N., and Bui, X. T. (2022). Compost to improve sustainable soil cultivation and crop productivity. Case Stud. Chem. Environ. Eng. 6:100211. doi: 10.1016/j.cscee.2022.100211
Homayoonfal, M., Khodaiyan, F., and Mousavi, M. (2015). Modelling and optimizing of physicochemical features of walnut-oil beverage emulsions by implementation of response surface methodology: effect of preparation conditions on emulsion stability. Food Chem. 174, 649–659. doi: 10.1016/j.foodchem.2014.10.117
Hoque, M. M., and Iqbal, A. (2015). Drying of watermelon rind and development of cakes from rind powder. Int. J. Novel Res. Life Sci. 2, 14–21.
Huang, K., Li, F., Li, J., Helard, D., and Hirooka, K. (2012). Rapid vermicomposting of fresh fruit and vegetable wastes using earthworm Eisenia foetida. J. Jpn. Soc. Civil Eng. Ser. G 68, III_113–III_120. doi: 10.2208/jscejer.68.III_113
Jain, M. S., Jambhulkar, R., and Kalamdhad, A. S. (2018). Biochar amendment for batch composting of nitrogen rich organic waste: effect on degradation kinetics, composting physics and nutritional properties. Bioresour. Technol. 253, 204–213. doi: 10.1016/j.biortech.2018.01.038
Jain, M. S., Paul, S., and Kalamdhad, A. S. (2019). Utilization of biochar as an amendment during lignocellulose waste composting: impact on composting physics and realization (probability) amongst physical properties. Process Saf. Environ. Prot. 121, 229–238. doi: 10.1016/j.psep.2018.10.031
Jifeng, C., Lishen, S., Hongyuan, L., Jie, Y., Ruiliang, G., Dong, C., et al. (2017). Screening of fermentation starters for organic fertilizer composted from chicken manure. Asian Agric. Res. 9, 92–98. doi: 10.22004/ag.econ.262788
Keskinen, R., Saastamoinen, M., Nikama, J., Särkijärvi, S., Myllymäki, M., Salo, T., et al. (2017). Recycling nutrients from horse manure: effects of bedding type and its compostability. Agric. Food Sci. 26, 68–79. doi: 10.23986/afsci.60443
Komar, S., Miskewitz, R., Westendorf, M., and Williams, C. A. (2012). Effects of bedding type on compost quality of equine stall waste: implications for small horse farms. J. Anim. Sci. 90, 1069–1075. doi: 10.2527/jas.2010-3805
Kong, Z., Wang, X., Liu, Q., Li, T., Chen, X., Chai, L., et al. (2018). Evolution of various fractions during the windrow composting of chicken manure with rice chaff. J. Environ. Manag. 207, 366–377. doi: 10.1016/j.jenvman.2017.11.023
Kumar, C. C., Mythily, R., and Chandraju, S. (2012). Studies on sugars extracted from water melon (Citrullus lanatus) rind, a remedy for related waste and its management. Int. J. Chem. Anal. Sci. 3, 1527–1529.
Lata Verma, S., and Marschner, P. (2013). Compost effects on microbial biomass and soil P pools as affected by particle size and soil properties. J. Soil Sci. Plant Nutr. 13, 313–328. doi: 10.4067/S0718-95162013005000026
Leghari, S. J., Wahocho, N. A., Laghari, G. M., HafeezLaghari, A., MustafaBhabhan, G., HussainTalpur, K., et al. (2016). Role of nitrogen for plant growth and development: a review. Adv. Environ. Biol. 10, 209–219.
Li, H., Duan, M., Gu, J., Zhang, Y., Qian, X., Ma, J., et al. (2017). Effects of bamboo charcoal on antibiotic resistance genes during chicken manure composting. Ecotoxicol. Environ. Saf. 140, 1–6. doi: 10.1016/j.ecoenv.2017.01.007
Lim, W. J., Chin, N. L., AnizaYusof, Y., Yahya, A., and Tee, T. P. (2019). Modelling of pilot-scale anaerobic food wastes composting process with dry leaves or cow manure. Pertanika J. Sci. Technol. 27, 421–442.
Liu, T., Awasthi, M. K., Chen, H., Duan, Y., Awasthi, S. K., and Zhang, Z. (2019). Performance of black soldier fly larvae (Diptera: Stratiomyidae) for manure composting and production of cleaner compost. J. Environ. Manag. 251:109593. doi: 10.1016/j.jenvman.2019.109593
Liu, L., Wang, S., Guo, X., Zhao, T., and Zhang, B. (2018). Succession and diversity of microorganisms and their association with physicochemical properties during green waste thermophilic composting. Waste Manag. 73, 101–112. doi: 10.1016/j.wasman.2017.12.026
Liu, Y., Wang, W., Xu, J., Xue, H., Stanford, K., McAllister, T. A., et al. (2018). Evaluation of compost, vegetable and food waste as amendments to improve the composting of NaOH/NaClO-contaminated poultry manure. PLoS One 13:e0205112. doi: 10.1371/journal.pone.0205112
Meng, L., Li, W., Zhang, S., Wu, C., and Lv, L. (2017). Feasibility of co-composting of sewage sludge, spent mushroom substrate and wheat straw. Bioresour. Technol. 226, 39–45. doi: 10.1016/j.biortech.2016.11.054
Mishra, S. K., and Yadav, K. D. (2022). Assessment of the effect of particle size and selected physico-chemical and biological parameters on the efficiency and quality of composting of garden waste. J. Environ. Chem. Eng. 10:107925. doi: 10.1016/j.jece.2022.107925
Mo, R. J., Zhao, Y., Wu, M., Xiao, H. M., Kuga, S., Huang, Y., et al. (2016). Activated carbon from nitrogen rich watermelon rind for high-performance supercapacitors. RSC Adv. 6, 59333–59342. doi: 10.1039/C6RA10719B
Mohamad Mazlan, M., Talib, R. A., Mail, N. F., Taip, F. S., Chin, N. L., Sulaiman, R., et al. (2019). Effects of extrusion variables on corn-mango peel extrudates properties, torque and moisture loss. Int. J. Food Prop. 22, 54–70. doi: 10.1080/10942912.2019.1568458
Nyi, T., Varughese, P., Bin, HJ, Bujang, M. I., Ra, K., Irianta, B., et al. (2017). ASEAN guidelines on soil and nutrient management. German Federal Ministry for Economic Cooperation and Development (BMZ). Available at: https://asean.org/wp-content/uploads/2021/08/ASEAN-Soil-and-Nutrient-Management-Guidelines.pdf
Oshins, C., Michel, F., Louis, P., Richard, T. L., and Rynk, R. (2022). “The composting process” in The composting handbook. Eds. R. Rynk, G. Black, J. Gilbert, J. Biala, J. Bonhotal, M. Schwarz, L. (Cooperband USA: Academic Press), 51–101.
Ozores-Hampton, M. (2017). Guidelines for assessing compost quality for safe and effective utilization in vegetable production. HortTechnology 27, 162–165. doi: 10.21273/HORTTECH03349-16
Pashaei, H., Ghaemi, A., Nasiri, M., and Karami, B. (2020). Experimental modeling and optimization of CO2 absorption into piperazine solutions using RSM-CCD methodology. ACS Omega 5, 8432–8448. doi: 10.1021/acsomega.9b03363
Qasim, W., Moon, B. E., Okyere, F. G., Khan, F., Nafees, M., and Kim, H. T. (2019). Influence of aeration rate and reactor shape on the composting of poultry manure and sawdust. J. Air Waste Manage. Assoc. 69, 633–645. doi: 10.1080/10962247.2019.1569570
Qian, X., Shen, G., Wang, Z., Guo, C., Liu, Y., Lei, Z., et al. (2014). Co-composting of livestock manure with rice straw: characterization and establishment of maturity evaluation system. Waste Manag. 34, 530–535. doi: 10.1016/j.wasman.2013.10.007
Renčo, M., Sasanelli, N., and Kováčik, P. (2011). The effect of soil compost treatments on potato cyst nematodes Globodera rostochiensis and Globodera pallida. Helminthologia 48, 184–194. doi: 10.2478/s11687-011-0027-1
Rob, (2021). Composting watermelon: an easy and eco-friendly way. CompostHQ. Available at: https://composthq.com/composting/can-you-compost-watermelon-time-scale/#google_vignette (Accessed February 16, 2024).
Romelle, F. D., Rani, A., and Manohar, R. S. (2016). Chemical composition of some selected fruit peels. Eur. J. Food Sci. Technol. 4, 12–21.
Sarlaki, E., Kermani, A. M., Kianmehr, M. H., Vakilian, K. A., Hosseinzadeh-Bandbafha, H., Ma, N. L., et al. (2021). Improving sustainability and mitigating environmental impacts of agro-biowaste compost fertilizer by pelletizing-drying. Environ. Pollut. 285:117412. doi: 10.1016/j.envpol.2021.117412
Sebaaly, Z., Fayssal, S. A., Shaban, N., and Sassine, Y. (2018). Growing Agaricus bisporus on compost mixtures based on chicken manure and banana residues. Available at: https://www.cabdirect.org/cabdirect/abstract/20193108681
Shahbandeh, M . (2022). Global Production of Fruit by Variety Selected 2022. Available at: https://www.statista.com/statistics/264001/worldwide-production-of-fruit-by-variety/ (Accessed February 13, 2024)
Sharma, D., Yadav, K. D., and Kumar, S. (2018). Biotransformation of flower waste composting: optimization of waste combinations using response surface methodology. Bioresour. Technol. 270, 198–207. doi: 10.1016/j.biortech.2018.09.036
Singh, J., and Kalamdhad, A. S. (2015). Assessment of compost quality in agitated pile composting of water hyacinth collected from different sources. Int. J. Recycl. Org. Waste Agric. 4, 175–183. doi: 10.1007/s40093-015-0097-z
Sirohi, R., Gaur, V. K., Pandey, A. K., Sim, S. J., and Kumar, S. (2021). Harnessing fruit waste for poly-3-hydroxybutyrate production: a review. Bioresour. Technol. 326:124734. doi: 10.1016/j.biortech.2021.124734
Smith, J. L., and Doran, J. W. (1997). Measurement and use of pH and electrical conductivity for soil quality analysis. Methods Assess Soil Quality 49, 169–185. doi: 10.2136/sssaspecpub49.c10
Smith, C., and Swanson, C. A. (2009). Horse Manure Management. Virginia Cooperative Extension, Virginia Polytechnic Institute and State University. Available at: https://vtechworks.lib.vt.edu/bitstream/handle/10919/48534/406-208_pdf.pdf?sequence=1 (Accessed April 5, 2023)
Sokri, S. M., Tee, T. P., Nurin, A. M. Y., Azhar, S., Nayan, N., and Suyub, I. H. (2023). Optimization on co-composting of horse manure with pineapple waste composition using response surface methodology (RSM). IOP Conf. Ser. Earth Environ. Sci. 1160:012026. doi: 10.1088/1755-1315/1160/1/012026
Stepanova, D. I., Grigorev, M. F., and Grigoreva, A. I. (2021). Production of biological fertilizers based on worm technology in Yakutia. IOP Confe. Ser. Earth Environ. Sci. 650:012024. doi: 10.1088/1755-1315/650/1/012024
Tanner, M. K., Swinker, A. M., Beard, M. L., Cosma, G. N., Traub-Dargatz, J. L., Martinez, A. B., et al. (1998). Effect of phone book paper versus sawdust and straw bedding on the presence of airborne gram-negative bacteria, fungi and endotoxin in horse stalls. J. Equine Vet. 18, 457–461. doi: 10.1016/S0737-0806(98)80038-5
Teferra, D. M., and Wubu, W. (2018). Biogas for clean energy. Anaerobic Digestion. 1, 149–168. doi: 10.5772/intechopen.79534
Tognetti, C., Mazzarino, M. J., and Laos, F. (2008). Compost of municipal organic waste: effects of different management practices on degradability and nutrient release capacity. Soil Biol. Biochem. 40, 2290–2296. doi: 10.1016/j.soilbio.2008.05.006
Vincent Ling, H. C. (2009) Convert animal waste into bio-compost – eggshells as additive in beef cattle manure compost enhancement. Master’s thesis. Malaysia: Universiti Putra Malaysia
Wan, L., Wang, X., Cong, C., Li, J., Xu, Y., Li, X., et al. (2020). Effect of inoculating microorganisms in chicken manure composting with maize straw. Bioresour. Technol. 301:122730. doi: 10.1016/j.biortech.2019.122730
Weil, J. D., Cutter, C. N., Beelman, R. B., and LaBORDE, L. F. (2013). Inactivation of human pathogens during phase II composting of manure-based mushroom growth substrate. J. Food Prot. 76, 1393–1400. doi: 10.4315/0362-028X.JFP-12-508
Westendorf, M., and Krogmann, U. (2013). Horses and Manure. New Jersey Agricultural Experiment Station. Available at: https://njaes.rutgers.edu/fs036/
Wong, Y., Tan, Y., Taufiq-Yap, Y. H., and Ramli, I. (2015). An optimization study for transesterification of palm oil using response surface methodology (RSM). Sains Malaysiana 44, 281–290. doi: 10.17576/jsm-2015-4402-17
Yong-Hong, L., Tai-Yuan, C., Chih-Hang, C., Tzu-Che, L., You-Jen, L., Mei-Juan, L., et al. (2021). Shorten the producing process of horse manure to fermented compost and appropriate fertilization on crops. J. Agric. Biotechnol. Sustain. Dev. 13, 1–11. doi: 10.5897/JABSD2020.0381
Yu, H., Xie, B., Khan, R., and Shen, G. (2019). The changes in carbon, nitrogen components and humic substances during organic-inorganic aerobic co-composting. Bioresour. Technol. 271, 228–235. doi: 10.1016/j.biortech.2018.09.088
Zahmatkesh, S., Far, S. S., and Sillanpää, M. (2022). RSM-D-optimal modeling approach for COD removal from low strength wastewater by microalgae, sludge, and activated carbon-case study Mashhad. J. Hazardous Mater. Adv. 7:100110. doi: 10.1016/j.hazadv.2022.100110
Zayadi, R. A. (2021). Current outlook of livestock industry in Malaysia and ways towards sustainability. J. Sustain. Nat. Resour. 2, 1–11. doi: 10.30880/jsunr.2021.12.02.001
Keywords: watermelon waste, chicken manure, horse manure, co-composting, optimization, response surface methodology
Citation: Nurin AY, Tee TP, Chin NL, Zainudin MHM and Nayan N (2024) Optimization of watermelon waste as a bulking agent for sustainable co-composting of livestock manures using response surface methodology. Front. Sustain. Food Syst. 8:1368970. doi: 10.3389/fsufs.2024.1368970
Edited by:
Paula Alvarenga, University of Lisbon, PortugalReviewed by:
Mehmet Ali Küçüker, Izmir Institute of Technology, TürkiyeCarlos Rad, University of Burgos, Spain
Copyright © 2024 Nurin, Tee, Chin, Zainudin and Nayan. This is an open-access article distributed under the terms of the Creative Commons Attribution License (CC BY). The use, distribution or reproduction in other forums is permitted, provided the original author(s) and the copyright owner(s) are credited and that the original publication in this journal is cited, in accordance with accepted academic practice. No use, distribution or reproduction is permitted which does not comply with these terms.
*Correspondence: Tuan Poy Tee, ttpoy@upm.edu.my; Mohd Huzairi Mohd Zainudin, mohdhuzairi@upm.edu.my