Prediction of Marshall Test Results for Dense Glasphalt Mixtures Using Artificial Neural Networks
- 1Civil and Environmental Engineering Department, Faculty of Engineering, Mutah University, Karak, Jordan
- 2Civil Engineering Department, Faculty of Engineering, Applied Science Private University, Amman, Jordan
- 3Civil Engineering Department, Faculty of Engineering, University of Petra, Amman, Jordan
- 4Civil Engineering Department, Faculty of Engineering, The Hashemite University, Zarqa, Jordan
Asphalt mixture comprising waste glass as an aggregate is referred to as “glasphalt”. Limited studies have been oriented to investigate the Marshall test results of dense-graded glasphalt mixes considering a wide range of variables. This study aims to utilize the artificial neural networks (ANNs) to develop predictive models for Marshall stability and Marshall flow of dense glasphalt mixes based on a large experimental database collected from the literature. Eight independent variables covering the material and mix properties were utilized as inputs in the models. The proposed models resulted in an experimental-to-predicted ratio of 1.00 and 1.00, coefficient of variation of 8.6% and 8.7%, RMSE of 1.63 kN and 0.54 mm, and R-squared of 93.6% and 85.7% for the glasphalt stability and flow models, respectively. Comprehensive parametric analyses have been conducted to further validate the models by investigating the sensitivity of their parameters to the predicted stability and flow values. The analyses revealed some desirable design values that could be considered for a better performance of dense glasphalt mixes. The results indicate that 4% is the desired design air void content of glasphalt mixes. High stability value can be achieved for glasphalt mixes containing a crushed aggregate of 12.5 mm maximum size and 50% glass cullet of 4.75 mm maximum size. Lower viscosity asphalt binder would provide uniformly compacted mixes. Furthermore, glasphalt flow increases as the maximum size of ingredient particles, the penetration grade of asphalt cement, asphalt cement content, and VMA% increase.
Introduction
Environmental waste materials increase every day as a result of domestic and industrial waste production, and landfill areas for those wastes are limited continuously (Ektas and Karacasu, 2012). Incorporating waste materials into asphalt mixtures has been investigated worldwide (Rahman et al., 2020). It has significant benefits in reducing the adverse impacts of waste materials on the environment, saving raw construction materials, using sustainable and cost-effective materials in asphalt mixes (Jony et al., 2011; Anochie-Boateng and George, 2016; Almuhmdi et al., 2021), and mitigating the high cost of landfill disposal (Disfani et al., 2011).
Many researchers have investigated the feasibility of utilizing waste crushed glass for road materials. Viswanathan (Viswanathan, 1996) investigated the applicability of utilizing glass cullet as a highway material and found that the glass waste has properties similar to natural aggregates and could be used as a highway construction material. As the awareness on environmental and natural resource issues increases, waste materials such as glass powder can be beneficially used in road construction (Finkle and Ksaibati, 2007; Arabani et al., 2017), and the use of glass cullet, with a maximum size of 12.5 mm (1/2 inch) and up to 20% replacement rate, was recommended as a supplement to the road-base materials. The high angularity of the glass cullet can enhance asphalt mix stability. Other characteristics of the waste glass such as low absorption, low specific gravity, and low thermal conductivity could offer better heat retention for asphalt mixes (Salem et al., 2017). Furthermore, constructing asphalt pavements with recycled glass offers an appropriate light reflection that enhances night visibility (Su and Chen, 2002).
Asphalt mixture comprising waste crush glass as an aggregate is referred to as “glasphalt” (Kandahl, 1992; Flynn, 1993). Alhassan et al. (Alhassan et al., 2018) investigated the feasibility of incorporating fine cullet from waste glass bottles with a maximum size of 4.75 mm into asphalt mixes at different percentages of 5%–10%. The study proved the applicability of using waste cullet as a partial replacement of fine aggregate in asphalt mixes. The results of Marshall stability, flow, bulk density, and air void content achieved their best values at 8.0% glass content. Dalloul (Dalloul, 2013) has also studied the effect of adding a crushed glass with a maximum fraction size of 4.75 mm into asphalt mixes at different percentages ranging from 2.5% to 15% by aggregate weight. His study indicated that 7.5% is the optimum content for waste glass fractions in asphalt mixes, where the stability and bulk density of HMA satisfied the standard limits of acceptance.
Arabani (Arabani, 2011) assesses replacing partial aggregate of both wearing and binder course mixes with 5%–20% waste glass fractions with a maximum size of 4.75 mm. The Marshall test results revealed that 15% is the most suitable glass content for the tested mixes. Su and Chen (Su and Chen, 2002) studied the applicability of recycling waste glass into asphalt pavement. They concluded that the stability values of asphalt mixes utilizing 5%–15% recycled glass (4.75 mm maximum size) are lower than those of control mixes. However, the obtained values by glasphalt mixes satisfied the acceptance limit for Marshall stability.
Waste crushed glass can be utilized in asphalt mixtures with a maximum size of 2.36 mm and an optimal replacement ratio of 10% (Salem et al., 2017). However, the Marshall stability of glasphalt mixes increased when waste glass fractions with a maximum size of 2.36 mm were added to the mixes with a 20% replacement rate (Almuhmdi et al., 2021). An opposite trend was also noticed for glasphalt density, VMA, and air void content with a 10%–25% replacement rate (Almuhmdi et al., 2021). A study conducted by Aashish et al. (Aashish and Tamrakar, 2019) showed that 10% was the desired percentage to achieve high stability and low flow for HMA containing 1.18–0.075 mm glass fractions. Issa (Issa, 2016) concluded that a significant improvement in the Marshall properties was achieved in glasphalt mixes when compared with control asphalt mixes.
Moreover, the feasibility of incorporating glass powder as a mineral filler (passing 0.075 mm, sieve (No. 200)) into asphalt mixtures has been investigated in several studies. Jony et al. (Jony et al., 2011), Arabani et al. (Arabani et al., 2017), Kifile et al. (Kifile et al., 2020), and Saltan et al. (Saltan et al., 2015a) have added the glass powder into asphalt mixes at different percentages to assess the mixes’ performance in terms of the Marshall test properties. Jony et al. (Jony et al., 2011) found that using 7% of glass powder as a mineral filler in asphalt mixes is sufficient to provide a high average value of Marshall stability and lower average values of flow and density when compared to asphalt mixes containing Portland cement or limestone powder as fillers. A study conducted by Arabani et al. (Arabani et al., 2017) also showed that utilizing 6% of glass powder as a mineral filler in asphalt mixes provides the best stability and lowest flow values among other mixes containing waste brick powder, rice-husk ash, and stone dust powder. Kifile et al. (Kifile et al., 2020) investigated the effect of replacing crushed stone-dust filler with waste glass powder in asphalt mixes at different replacement rates. They concluded that as the glass powder content increases in asphalt mixes, the Marshall stability, bulk density, and voids filled with asphalt values increased, whereas the values of Marshall flow, air voids, and void in mineral aggregates decreased. The results proved that the 75% replacement rate can provide optimum performance for heavy traffic conditions (Kifile et al., 2020).
Proper mix design of asphalt mixes with suitable ingredients and proportions enhances pavement performance (Brown et al., 2009; Asphalt Institute, 2014). Marshall’s design method has been widely adopted for asphalt mix design. It is essentially used for designing dense-graded asphalt mixes that have well-graded aggregate particles distributed from course to fine throughout the entire range of sieves (Garcia and Hansen, 2001; Brown et al., 2009; Asphalt Institute, 2014). In general, the method involves several steps including aggregate and bitumen evaluation, test specimen preparation, density and void analysis, Marshall stability and flow tests, and data interpretation (Brown et al., 2009). The Marshall stability is defined as the maximum load carried by a test specimen of asphalt concrete before failure when tested at 60 °C and 51 mm/min loading rate. The flow is the amount of the specimen’s deformation before failure. Both properties are determined physically from the Marshall test. Other test parameters such as bulk specific gravity of asphalt mixture
Recently, the machine learning approaches have been utilized in several studies to predict models for different aspects of construction materials and civil engineering applications (Murad et al., 2021; Al Bodour et al., 2022; Iftikhar et al., 2022; Momani et al., 2022). The artificial neural networks (ANNs), which is a recent learning machine tool, was also utilized by researchers in asphalt mix design applications (Tapkın et al., 2010; Ozgan, 2011; Singh et al., 2013; Ozturk and Kutay, 2014; Shafabakhsh et al., 2015; Ozturk et al., 2016; Zavrtanik et al., 2016; Pasetto et al., 2019; Fadhil et al., 2022; Othman, 2022). An ANN–based model to predict the Marshall mix volumetric properties has been proposed by Ozturk et al. (Ozturk et al., 2016). Ozgan (Ozgan, 2011) modeled the Marshall stability of asphalt mixes under different testing temperatures and exposure time conditions using the ANN technique. Tapkın et al. (Tapkın et al., 2010) proposed a neural-network model to predict the Marshall stability, flow, and Marshall quotient for polypropylene-modified asphalt mixtures. Some physical properties of standard Marshall specimens such as asphalt content, specimen height, unit weight, VMA, VFA, Va, polypropylene types, and percentage were used as inputs in the proposed model (Tapkın et al., 2010). Fadhil et al. (Fadhil et al., 2022) have utilized the ANN approach as a tool for HMA design based on actual data of 252 mixes following the Marshall design method. They used five parameters including asphalt penetration, kinematic viscosity, aggregate surface area, abrasion, and binder content as inputs in the models to predict five parameters of HMA design including the bulk density, air voids, Marshall stability, Marshall flow, and Marshall stiffness. Othman (Othman, 2022) has predicted the optimum asphalt content (OAC) based on different aggregate gradations using ANN approaches of different activation functions, number of hidden layers, and number of neurons per layer. The volumetric properties of Superpave asphalt mixes were also modeled utilizing the ANN approach at different gyration levels (Ozturk and Kutay, 2014). In addition, the performance of asphalt pavements incorporating waste materials has been predicted using the ANN approach in several studies (Xiao et al., 2009; Ektas and Karacasu, 2012; Azarhoosh et al., 2018; Mansourian et al., 2018; Keskin and Karacasu, 2021). The fatigue behavior of glasphalt mixes has been modeled analytically by Arabani et al. (Arabani et al., 2012).
Previous studies in the literature indicate that incorporating glass waste in asphalt mixes, either as an aggregate or mineral filler substitute, provided some promising results, especially in terms of Marshall stability and Marshall flow. However, there is no mutual agreement on the effect of contributing different variables on glasphalt stability and flow. In addition, there is a lack of studies evaluating the behavior of glasphalt based on a large database with a wide range of variables including the maximum aggregate size (MAS), the maximum size of waste glass fractions (MSGF), average penetration grade of asphalt (AP), asphalt content (AC%), waste glass content (WGC%), the bulk specific gravity of the glasphalt mixture
Methodology and Experimental Database
Figure 1 outlines the research program followed in this study. The study started with studying the previous literature on dense glasphalt mixes, their properties, and prediction models. A large worldwide database of 136 reliable experimental data points was then collected from thirteen different studies (Malisch et al., 1970; Arabani, 2011; Jony et al., 2011; Arabani and Kamboozia, 2013; Dalloul, 2013; Saltan et al., 2015b; Arabani et al., 2017; Salem et al., 2017; Al- Jameel and Al- Saeedi, 2018; Alhassan et al., 2018; Aashish and Tamrakar, 2019; Kifile et al., 2020; Almuhmdi et al., 2021) available in the literature and were carried out according to ASTM D1559 and ASTM D6927 testing procedures. Natural crushed aggregate, waste crushed glass, and asphalt cement were the main ingredients for the dense-graded glasphalt mixtures of the database test specimens. The specimens were prepared using a standard Marshall mold of 4 inches (101.6 mm) in diameter and 2.5 inches (63.5 mm) in height.
The experimental studies showed that the dense glasphalt mixes are mainly influenced by a wide variety of design and material properties. Therefore, eight independent variables including MAS, MSGF, AP, AC%, WGC%,
Artificial Neural Network
ANN is a relatively new soft-computing method that has been utilized in several fields of civil engineering. The ANN is known for its ability as a powerful prediction tool that describes the relationship between input parameters and the output. The ANN is a multilayer perception-based procedure as shown in Figure 3. The first layer is called the Input Layer, which includes the inputs (independents) of the problem, a hidden layer, and an output layer that contains the output of the problem of interest. Each layer consists of processing units that are fully connected with the processing units in the subsequent layer.
Each signal or input xi that reaches the processing units is multiplied by a calibration weight wji that controls the signal’s behavior and significance. The calibrated signals are added to a calibrated bias value Bji at each processing unit, as shown in Eq. (1). The combined input Ij is then sent through a nonlinear transfer function f(Ij) to generate the processing output, which will be used as the input for the following layer’s processing units (Figure 3). This research uses a hyperbolic tangent transfer sigmoid function.
An algorithm adjusts the input weights and bias values using the Levenberg–Marquardt optimization approach to train the ANN model. To construct a network that generalizes well, the optimization technique minimizes a combination of squared errors and weights and then selects the optimum combination. The method is known as Bayesian regularization. The mean square error (MSE), also known as the performance function (Eq. (2)) [X], is used to express the error. The training procedure will continue until the MSE converges and no more improvement is possible. Eighty percent of the data was used for training, and the rest was used for testing and validation. MATLAB software was used to conduct the analysis.
Several iterations were used to choose the needed number of processing units in the hidden layer to represent and approximate the relationships between variables. The best number of layers was encountered by trying processing units from 1 to 10 while monitoring the MSE to minimize overfitting. Due to the use of Bayesian regularization and the amount of data in the current case, overfitting was not an issue.
In this study, two ANN models with two processing units in the hidden layers were constructed to predict the stability and flow of the dense glasphalt. The input layer for each model includes MAS, MSGF, AP, AC%, WGC%,
Parametric Analyses
The developed ANN models are used to perform parametric analyses to evaluate the effect of each independent variable on the Marshall stability and flow values of dense-graded glasphalt mixes. The parametric analyses were conducted by fixing the mean values of all variables and then varying the values of each concerned variable independently. Figures 5 and 6 show the plots of each independent variable on the stability and flow values of the dense-graded glasphalt mixes, respectively. These plots are useful as they enable better and simple visualization of the influence of each studied variable on the output parameters.
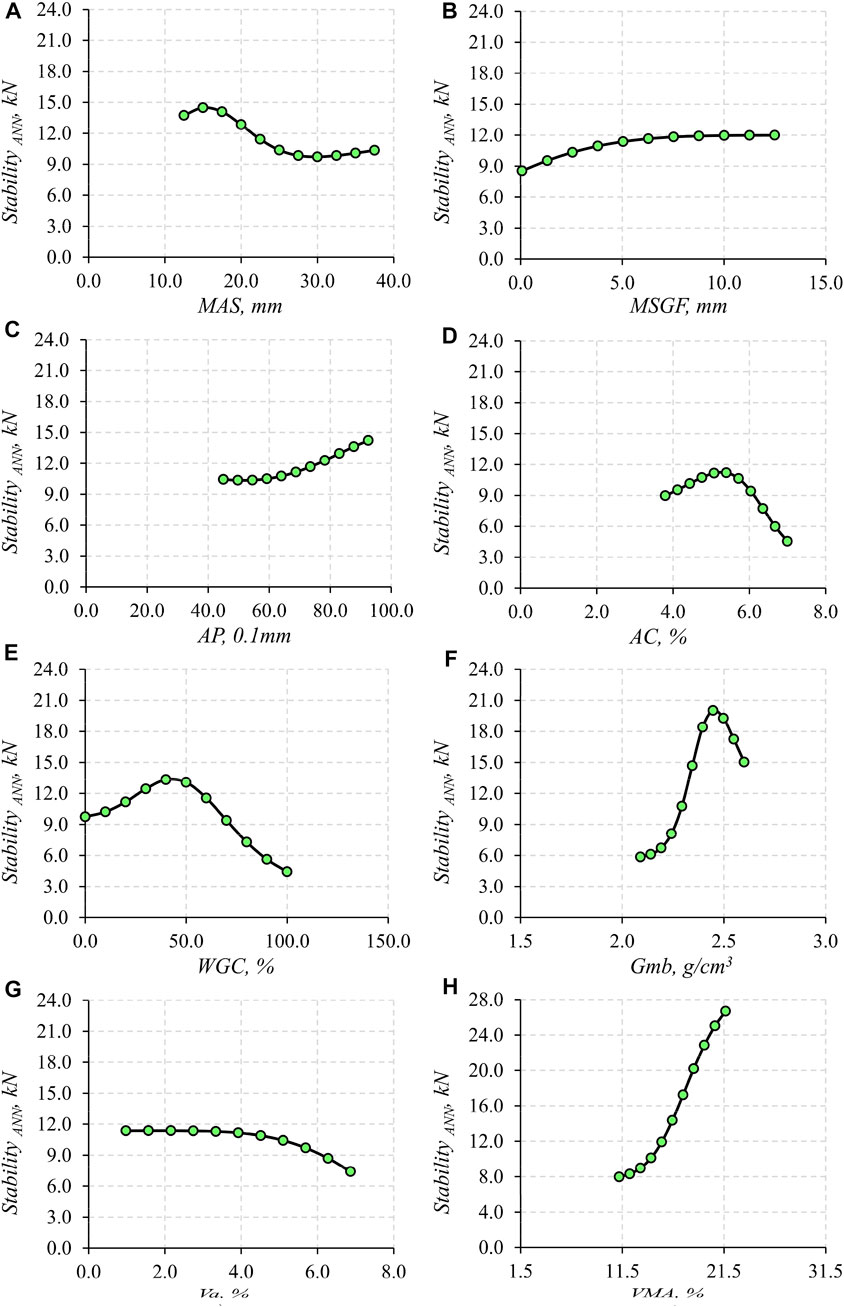
FIGURE 5. Parametric study for glasphalt stability versus (A) Maximum aggregate size (MAS), (B) Maximum size of waste glass fractions (MSGF), (C) Average penetration grade of asphalt (AP), (D) Asphalt content (AC%), (E) Waste glass content (WGC%), (F) Bulk specific gravity of glasphalt mixture, (G) Air voids content (Va%), and (H) Voids in mineral aggregate (VMA%).
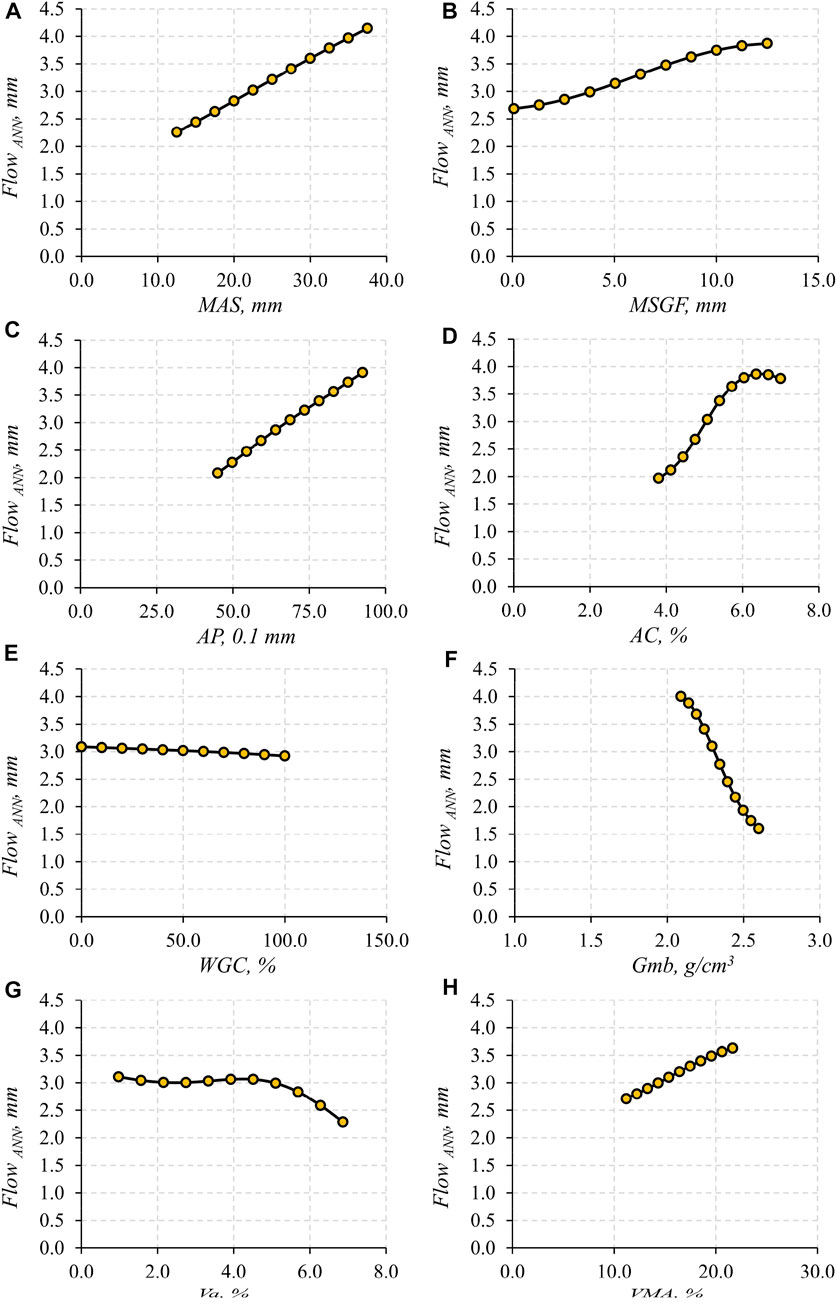
FIGURE 6. Parametric study for glasphalt flow versus (A) Maximum aggregate size (MAS), (B) Maximum size of waste glass fractions (MSGF), (C) Average penetration grade of asphalt (AP), (D) Asphalt content (AC%), (E) Waste glass content (WGC%), (F) Bulk specific gravity of glasphalt mixture, (G) Air voids content (Va%), and (H) Voids in mineral aggregate (VMA%).
It can be seen from Figure 5A that the stability of dense-graded glasphalt mixes of small maximum aggregate size (MAS) would have better stability than that with large MAS. The stability of mixes reaches its peak value at about 12.5 mm (1/2 inch) and then decreases gradually as the maximum size increases to about 25 mm (1.0 inch). No considerable change in the stability was observed when the MAS reaches 37.5 mm (1 ½ inch). Figure 5B indicates that glasphalt stability improved by increasing the maximum size of waste glass fractions (MSGF) to 4.75 mm (Sieve No. 4). Then, the stability maintains at a constant level for mixes incorporating glass fractions larger than 4.75 mm MSGF.
Figure 5C shows the relation between the average penetration grade of asphalts (AP) and glasphalt stability. The plot indicates that the stability increases as the AP increases, especially when the AP is higher than 60 (0.1 mm). This may be attributed to the low viscosity of the high penetration grade of asphalt cement, which provides proper coating and uniform dispersion of the angular glass cullet and aggregate particles that result in high stability. While the assumption that “soft” asphalt cement with a high penetration number is recommended to use in cold climate regions to resist thermal cracking (Brown et al., 2009), it can be argued that constructing glasphalt pavements with appropriate asphalt cement in cold regions would provide better stability than in warm regions.
As expected, in Figures 5D and F, the stability increases as the asphalt cement content (AC %) and bulk specific gravity of the glasphalt mixture (Gmb) increase to a certain limit and then decrease. This appreciable increase in the stability is attributed to the hot asphalt cement that initially lubricates the particles, enhances the interlock, and compacts them to a high density. The gradual decrease in the stability after that refers to the additional asphalt cement that produces thicker films around particles, pushing them apart and resulting in lower stability. It should be mentioned, however, that the considerable changes in the glasphalt density can be attributed to the waste glass content in the mixes, where the density of the glasphalt mixes reduces as the glass content increases (Al- Jameel and Al- Saeedi, 2018).
Figure 5E shows a gradual increase in the stability by increasing the waste glass content (WGC) up to 50%, and then the stability decreases as the WGC% achieves 100%. This behavior refers to the high angularity of the glass cullet compared to the crushed-stone aggregate, which increases the interlock between particles. After that the stability decreases gradually by increasing the WGC% because the crushed glass has less capability to bear the load (less abrasion) than the crushed aggregate in the mixes. It can be stated that a 50% replacement rate might be the ideal rate for incorporating waste crush glass as an aggregate substitute in glasphalt mixes.
Figure 5G shows the relation between the air-void content (Va%) and glasphalt stability. It can be seen that increasing the air voids up to 4% will keep the stability at a constant level. However, a gradual decrease is observed in the stability by increasing the air voids up to 7%. This agrees with the conventional design value of 4% air void content for a dense-graded asphalt mix design. Finally, the effect of VMA on glasphalt stability is shown in Figure 5H. The stability increases as the VMA increases. While the VMA represents the amount of space available for bitumen to properly coat each aggregate particle, sufficient asphalt film thickness around the glass and aggregate particles promotes good adhesion between them and increases the mix stability. It should be mentioned that the VMA refers to the total volume of air voids and effective (non-absorbed) asphalt cement between mineral particles of a compacted mix. Thus, the design should consider the minimum VMA values needed to achieve a durable asphalt film thickness around particles, which is determined based on the nominal maximum particle size and the design air voids of the mix (Brown et al., 2009; Asphalt Institute, 2014).
According to the flow results of the glasphalt mixes, Figures 6A and B indicate that the flow increases as the maximum size of used aggregate and glass cullet increases in the mix. This increase indicates that the larger the MAS and MSGF, the better the flexibility of the glasphalt mixes since the flow represents the total amount of vertical deformation of a test specimen. Figures 6C and 6H also show that the glasphalt flow increases linearly as the penetration grade of the used asphalt cement and the VMA% increases. This increase in the flow is expected since a higher penetration grade of asphalt cement indicates a lower viscosity of asphalt cement. Also, higher VMA% indicates a compacted mix with more spaces filled with non-absorbed asphalt cement and air voids, which provides better flexibility to the mixes. Figure 6D shows that the flow increases gradually as the AC% achieves 6%, and then no considerable change in the flow values was noticed.
Opposite to the stability trends, the flow values stood generally at a certain level as the waste glass content (WGC%) increases in the glasphalt mixes, as shown in Figure 6E. The flow values were also decreased gradually by increasing the bulk density of the glasphalt mixes
Conclusion
As the awareness on environmental and natural resource issues increases globally, the waste glass cullet is used as an aggregate substitute in asphalt pavements. Asphalt mixture comprising waste crushed glass as an aggregate is referred to as “glasphalt”. This study aims to investigate the feasibility of utilizing the ANN learning machine tool to predict new and effective models to predict the Marshall stability and Marshall flow of the dense-graded glasphalt mixes. The developed ANN models utilized a wide range of related Marshall test results collected from previous studies available in the literature. Eight independent variables were selected in this study as inputs for the models. They include the maximum aggregate size (MAS), the maximum size of waste glass fractions (MSGF), average penetration grade of the used asphalts cement (AP), asphalt cement content (AC%), waste glass content (WGC%), bulk specific gravity of the glasphalt mixture
The proposed ANN models proved their high accuracy to predict the Marshall stability and Marshall flow of the dense glasphalt mixes. The results show an experimental-to-predicted ratio of 1.00 and 1.00, coefficient of variation (CoV) of 8.6% and 8.7%, RMSE of 1.63 kN and 0.54 mm, and R-squared of 93.6% and 85.7% for the glasphalt stability and flow models, respectively. The sensitivity of each variable on the Marshall stability and flow was investigated by conducting parametric analyses, and the following conclusions were obtained:
1) The desired maximum size of the used aggregate and waste crushed glass in glasphalt mixes is about 12.5 and 4.75 mm, respectively, where maximum stability values are achieved.
2) Using low viscosity asphalt cement would provide better coating and uniform dispersion of the used angular crushed glass and aggregate in the mix, resulting in high stability.
3) Glasphalt stability increases to a certain limit and then decreases gradually as the asphalt cement content and the bulk specific gravity increase for the mix.
4) The optimal content for incorporating waste crushed glass as an aggregate substitute in asphalt mixes is limited to 50%. This may refer to the high angularity and less bearing capability of the crushed glass compared to the natural aggregate, which limits mixing asphalt mixes with only waste crushed glass as an aggregate.
5) A 4.0% is the typical design content for the air voids in glasphalt mixes.
6) The stability increases as the VMA% increases. However, the minimum VMA% value that is required to assure a sufficient asphalt film thickness around the mix’s ingredients should be considered while designing.
7) The flow increases as the maximum size of crushed glass and aggregate, the penetration grade of asphalt cement, asphalt cement content, and VMA% increase.
8) Varying the content of the waste glass in glasphalt mixes does not affect the flow values, whereas increasing the mix density reduces the flow values.
The developed ANN models are very important in the sense that they provide accurate, rational, general, and simple visualization for the performance of the dense glasphalt mixes in terms of Marshal stability and flow considering a wide range of different parameters. They are also helpful in saving time and effort to estimate the Marshall test properties of glasphalt mixes. It would be recommended to develop mathematical equations for estimating the performance of dense glasphalt mixes based on a wide range of design variables.
Data Availability Statement
The raw data supporting the conclusions of this article will be made available by the authors, without undue reservation.
Author Contributions
Concept: YJ, AT, and YM. Data collection: YJ, RA, and YM. Analysis: AT, YM, YJ, and RA. Writing: YJ, RA, AT, and YM.
Conflict of Interest
The authors declare that the research was conducted in the absence of any commercial or financial relationships that could be construed as a potential conflict of interest.
The handling editor YM declared a past co-authorship with the author AT.
Publisher’s Note
All claims expressed in this article are solely those of the authors and do not necessarily represent those of their affiliated organizations, or those of the publisher, the editors, and the reviewers. Any product that may be evaluated in this article, or claim that may be made by its manufacturer, is not guaranteed or endorsed by the publisher.
References
Aashish, A., and Tamrakar, G. B. S. (2019). Effect of Adding Waste Crushed Glass to Asphalt Mix. Int. J. Adv. Eng. Manag. 4 (4), 10–18.
Al Bodour, W., Hanandeh, S., Hajij, M., and Murad, Y. (2022). Development of Evaluation Framework for the Unconfined Compressive Strength of Soils Based on the Fundamental Soil Parameters Using Gene Expression Programming and Deep Learning Methods. J. Mater. Civ. Eng. 34 (2), 04021452. doi:10.1061/(asce)mt.1943-5533.0004087
Al-Jameel, H. A. E., and Al-Saeedi, B. J. K. (2018). Sustainable Performance of Iraqi Asphalt Base Course Using Recycled Glass as Aggregate Replacement. Jubes 26 (5), 63–80. doi:10.29196/jub.v26i5.869
Alhassan, H. M., Yunusa, G. H., and Sanusi, D. (2018). Potential of Glass Cullet as Aggregate in Hot Mix Asphalt. Nig. J. Tech. 37 (2), 338–345. doi:10.4314/njt.v37i2.8
Almuhmdi, A., Muhmood, A., and Salih, A. (2021). Effects of Crushed Glass Waste as a Fine Aggregate on Properties of Hot Asphalt Mixture. Tikrit J. Eng. Sci. 28 (3), 129–145. doi:10.25130/tjes.28.3.10
Anochie-Boateng, J., and George, T. (2016). Use of Waste Crushed Glass for the Production of Hot-Mix Asphalt. Sustain. Constr. Mater. Technol. (SCMT). doi:10.18552/2016/scmt4s198
Arabani, M. (2011). Effect of Glass Cullet on the Improvement of the Dynamic Behaviour of Asphalt Concrete. Constr. Build. Mater. 25 (3), 1181–1185. doi:10.1016/j.conbuildmat.2010.09.043
Arabani, M., and Kamboozia, N. (2013). The Linear Visco-Elastic Behaviour of Glasphalt Mixture under Dynamic Loading Conditions. Constr. Build. Mater. 41, 594–601. doi:10.1016/j.conbuildmat.2012.12.023
Arabani, M., Mirabdolazimi, S. M., and Ferdowsi, B. (2012). Modeling the Fatigue Behaviors of Glasphalt Mixtures. Sci. Iran. 19 (3), 341–345. doi:10.1016/j.scient.2012.02.021
Arabani, M., Tahami, S. A., and Taghipoor, M. (2017). Laboratory Investigation of Hot Mix Asphalt Containing Waste Materials. Road Mater. Pavement Des. 18 (3), 713–729. doi:10.1080/14680629.2016.1189349
Azarhoosh, A. R., Hamedi, G. H., and Abandansari, H. F. (2018). Providing Laboratory Rutting Models for Modified Asphalt Mixes with Different Waste Materials. Period. Polytech. Civ. Eng. 62 (2), 308–317. doi:10.3311/ppci.10684
Brown, E. R., Kandhal, P. S., Roberts, F. L., Kim, Y. R., Lee, D. Y., and Kennedy, T. W. (2009). Hot Mix Asphalt Materials, Mixture Design, and Construction. MD, United States: NAPA research and education foundation.
Dalloul, K. N. (2013). Study of the Effect of Crushed Waste Glass as Coarse Sand and Filler in the Asphalt Binder Course. Master Thesis (Gaza: University of Gaza).
Disfani, M. M., Arulrajah, A., Bo, M. W., and Hankour, R. (2011). Recycled Crushed Glass in Road Work Applications. Waste Manag. 31 (11), 2341–2351. doi:10.1016/j.wasman.2011.07.003
Ektas, S., and Karacasu, M. (2012). Use of Recycled Concrete in Hot Mix Asphalt and an ANN Model for Prediction of Resilient Modulus. Ekoloji Derg. 21 (83). doi:10.5053/ekoloji.2012.836
Fadhil, T. H., Ahmed, T. M., and Al Mashhadany, Y. I. (2022). Application of Artificial Neural Networks as Design Tool for Hot Mix Asphalt. Int. J. Pavement Res. Technol. 15 (2), 269–283. doi:10.1007/s42947-021-00065-7
Finkle, I., and Ksaibati, K. (2007). “Recycled Glass Utilization in Highway Construction,” in Proceedings of the Transportation Research Board 86th Annual Meeting (University of Wyoming, Laramie, WY, United States), MPC Report No. 07-192.
Garcia, J., and Hansen, K. (2001). HMA Pavement Mix Type Selection Guide. MD, United States: National Asphalt Pavement Association.
Iftikhar, B., Alih, S. C., Vafaei, M., Elkotb, M. A., Shutaywi, M., Javed, M. F., et al. (2022). Predictive Modeling of Compressive Strength of Sustainable Rice Husk Ash Concrete: Ensemble Learner Optimization and Comparison. J. Clean. Prod. 348, 131285. doi:10.1016/j.jclepro.2022.131285
Issa, Y. (2016). Effect of Adding Crushed Glass to Asphalt Mix. Archives Civ. Eng. 62 (2), 35–44. doi:10.1515/ace-2015-0063
Jony, H. H., Al-Rubaie, M., and Jahad, I. (2011). The Effect of Using Glass Powder Filler on Hot Asphalt Concrete Mixtures Properties. Eng. Technol. J. 29 (1), 44–57.
Kandahl, P. (1992). Waste Materials in Hot Mix Asphalt. Auburn University, United States: National Center for Asphalt Technology. NCAT Report 92–06.
Keskin, M., and Karacasu, M. (2021). Artificial Neural Network Modelling for Asphalt Concrete Samples with Boron Waste Modification. J. Eng. Res. doi:10.36909/jer.8124
Kifile, D., Quezon, E. T., and Tesfaye, A. (2020). Effect of Partial Replacement of Crushed Stone Dust Filler with Waste Glass Powder in Hot Mix Asphalt Concrete Production. Xi'an Dianzi Keji Daxue Xuebao/Journal Xidian Univ. 14 (9).
Malisch, W. R., Day, D. E., and Wixson, B. G. (1970). Use of Domestic Waste Glass as Aggregate in Bituminous Concrete. Highw. Res. Rec. 307, 1–10.
Mansourian, A., Ghanizadeh, A. R., and Golchin, B. (2018). Modeling of Resilient Modulus of Asphalt Concrete Containing Reclaimed Asphalt Pavement Using Feed-Forward and Generalized Regression Neural Networks. J. Rehabilitation Civ. Eng. 6 (1), 132–147. doi:10.22075/JRCE.2017.10876.1177
Momani, Y., Tarawneh, A., Alawadi, R., and Momani, Z. (2022). Shear Strength Prediction of Steel Fiber-Reinforced Concrete Beams without Stirrups. Innov. Infrastruct. Solutions 7 (1), 1–15. doi:10.1007/s41062-021-00703-w
Murad, Y., Tarawneh, A., Arar, F., Al-Zu'bi, A., Al-Ghwairi, A., Al-Jaafreh, A., et al. (2021). Flexural Strength Prediction for Concrete Beams Reinforced with FRP Bars Using Gene Expression Programming. Structures 33, 3163–3172. Elsevier. doi:10.1016/j.istruc.2021.06.045
Othman, K. (2022). Artificial Neural Network Models for the Estimation of the Optimum Asphalt Content of Asphalt Mixtures. Int. J. Pavement Res. Technol., 1–13. doi:10.1007/s42947-022-00179-6
Ozgan, E. (2011). Artificial Neural Network Based Modelling of the Marshall Stability of Asphalt Concrete. Expert Syst. Appl. 38 (5), 6025–6030. doi:10.1016/j.eswa.2010.11.018
Ozturk, H. I., Saglik, A., Demir, B., and Gungor, A. G. (2016). “An Artificial Neural Network Base Prediction Model and Sensitivity Analysis for Marshall Mix Design,” in Proceedings of the 6th Eurasphalt & Eurobitume Congress (Prague, Czech Republic. doi:10.14311/ee.2016.224
Ozturk, H. I., and Kutay, M. E. (2014). An Artificial Neural Network Model for Virtual Superpave Asphalt Mixture Design. Int. J. Pavement Eng. 15 (2), 151–162. doi:10.1080/10298436.2013.808341
Pasetto, M., Baldo, N., and Manthos, E. (2019). Asphalt Concrete Mechanical Behavior Prediction by Artificial Neural NetworksBitum. Mix. Pavements VII. CRC Press, 252–261. doi:10.1201/9781351063265-38
Rahman, M. T., Mohajerani, A., and Giustozzi, F. (2020). Recycling of Waste Materials for Asphalt Concrete and Bitumen: A Review. Materials 13 (7), 1495. doi:10.3390/ma13071495
Salem, Z. T. A., Khedawi, T. S., Baker, M. B., and Abendeh, R. (2017). Effect of Waste Glass on Properties of Asphalt Concrete Mixtures. Jordan J. Civ. Eng. 11 (1).
Saltan, M., Öksüz, B., and Uz, V. E. (2015). Use of Glass Waste as Mineral Filler in Hot Mix Asphalt. Sci. Eng. Compos. Mater. 22 (3), 271–277. doi:10.1515/secm-2013-0135
Saltan, M., Öksüz, B., and Uz, V. E. (2015). Use of Glass Waste as Mineral Filler in Hot Mix Asphalt. Sci. Eng. Compos. Mater. 22 (3), 271–277. doi:10.1515/secm-2013-0135
Shafabakhsh, G. H., Ani, O. J., and Talebsafa, M. (2015). Artificial Neural Network Modeling (ANN) for Predicting Rutting Performance of Nano-Modified Hot-Mix Asphalt Mixtures Containing Steel Slag Aggregates. Constr. Build. Mater. 85, 136–143. doi:10.1016/j.conbuildmat.2015.03.060
Singh, D., Zaman, M., and Commuri, S. (2013). Artificial Neural Network Modeling for Dynamic Modulus of Hot Mix Asphalt Using Aggregate Shape Properties. J. Mat. Civ. Eng. 25 (1), 54–62. doi:10.1061/(asce)mt.1943-5533.0000548
Su, N., and Chen, J. S. (2002). Engineering Properties of Asphalt Concrete Made with Recycled Glass. Resour. conservation Recycl. 35 (4), 259–274. doi:10.1016/s0921-3449(02)00007-1
Tapkın, S., Çevik, A., and Uşar, Ü. (2010). Prediction of Marshall Test Results for Polypropylene Modified Dense Bituminous Mixtures Using Neural Networks. Expert Syst. Appl. 37 (6), 4660–4670. doi:10.1016/j.eswa.2009.12.042
Viswanathan, K. (1996). Characterization of Waste Recycled Glass as a Highway Material. Doctoral dissertation. TX, United States: Texas Tech University.
Xiao, F., Amirkhanian, S., and Juang, C. H. (2009). Prediction of Fatigue Life of Rubberized Asphalt Concrete Mixtures Containing Reclaimed Asphalt Pavement Using Artificial Neural Networks. J. Mat. Civ. Eng. 21 (6), 253–261. doi:10.1061/(asce)0899-1561(2009)21:6(253)
Keywords: waste glass, prediction models, glasphalt, Marshall stability, flow
Citation: Jweihan YS, Alawadi RJ, Momani YS and Tarawneh AN (2022) Prediction of Marshall Test Results for Dense Glasphalt Mixtures Using Artificial Neural Networks. Front. Built Environ. 8:949167. doi: 10.3389/fbuil.2022.949167
Received: 20 May 2022; Accepted: 09 June 2022;
Published: 13 July 2022.
Edited by:
Yasmin Murad, The University of Jordan, JordanReviewed by:
Taqwa Alhadidi, Al-Ahliyya Amman University, JordanBara’ Al-Mistarehi, Jordan University of Science and Technology, Jordan
Taher Ahmed, University of Anbar, Iraq
Copyright © 2022 Jweihan, Alawadi, Momani and Tarawneh. This is an open-access article distributed under the terms of the Creative Commons Attribution License (CC BY). The use, distribution or reproduction in other forums is permitted, provided the original author(s) and the copyright owner(s) are credited and that the original publication in this journal is cited, in accordance with accepted academic practice. No use, distribution or reproduction is permitted which does not comply with these terms.
*Correspondence: Yazeed S. Jweihan, y.jweihan@mutah.edu.jo