Drug repurposing screening validated by experimental assays identifies two clinical drugs targeting SARS-CoV-2 main protease
- 1Laboratory of Bioactive Compound Research and Development (LIDeB), Faculty of Exact Sciences, National University of La Plata (UNLP), La Plata, Buenos Aires, Argentina
- 2Laboratory Redox Biology of Trypanosomes, Institut Pasteur de Montevideo, Montevideo, Uruguay
- 3Laboratorio de Química Aplicada, Facultad de Bioquímica y Ciencias Biológicas, Universidad Nacional del Litoral, Santa Fe, Argentina
- 4Laboratory of Immunovirology, Institut Pasteur de Montevideo, Montevideo, Uruguay
- 5Departamento de Inmunobiología, Facultad de Medicina, Universidad de la República, Montevideo, Uruguay
- 6Screening Discovery Platform, Institut Pasteur Korea, Seongnam, South Korea
The COVID-19 pandemic prompted several drug repositioning initiatives with the aim to rapidly deliver pharmacological candidates able to reduce SARS-CoV-2 dissemination and mortality. A major issue shared by many of the in silico studies addressing the discovery of compounds or drugs targeting SARS-CoV-2 molecules is that they lacked experimental validation of the results. Here we present a computer-aided drug-repositioning campaign against the indispensable SARS-CoV-2 main protease (MPro or 3CLPro) that involved the development of ligand-based ensemble models and the experimental testing of a small subset of the identified hits. The search method explored random subspaces of molecular descriptors to obtain linear classifiers. The best models were then combined by selective ensemble learning to improve their predictive power. Both the individual models and the ensembles were validated by retrospective screening, and later used to screen the DrugBank, Drug Repurposing Hub and Sweetlead libraries for potential inhibitors of MPro. From the 4 in silico hits assayed, atpenin and tinostamustine inhibited MPro (IC50 1 µM and 4 μM, respectively) but not the papain-like protease of SARS-CoV-2 (drugs tested at 25 μM). Preliminary kinetic characterization suggests that tinostamustine and atpenin inhibit MPro by an irreversible and acompetitive mechanisms, respectively. Both drugs failed to inhibit the proliferation of SARS-CoV-2 in VERO cells. The virtual screening method reported here may be a powerful tool to further extent the identification of novel MPro inhibitors. Furthermore, the confirmed MPro hits may be subjected to optimization or retrospective search strategies to improve their molecular target and anti-viral potency.
1 Introduction
The outbreak of COVID-19 (coronavirus disease of 2019) posed a threat to global health, with so far more than 580 million confirmed cases and over 6.5 million deaths, resulting in unparalleled human and economic losses in modern times (except for the 1918 influenza pandemic) (Chen et al., 2021; Wang et al., 2022). The rapid development of effective vaccines has improved the disease prognosis, resulting in a substantial drop in mortality (compared to estimations if no vaccines were available; Watson et al., 2022).
Complementarily, the scientific community has resorted to drug repurposing (i.e., the identification of new therapeutic uses for known drugs) to find therapeutics in an expedite manner (Ashburn and Thor 2004). This led to the approval of several repurposed drug treatments (such as remdesivir, favipiravir, and dexamethasone) in less than 2 years, with other repurposing candidates still in the pipeline (Bellera et al., 2021; Chakraborty et al., 2021; Venkadapathi et al., 2021; Rodrigues et al., 2022).
Effective treatments are, however, still needed, especially for people unvaccinated or that may not fully respond to vaccination (e.g., immunocompromised patients), and to cover viral mutations that may potentially elicit vaccine escape (Niknam et al., 2022). SARS-CoV-2 (Severe Acute Respiratory Syndrome Coronavirus 2) main protease (MPro) has received major attention as a potential pharmacological target, not only due to its role in viral replication, but also because no human proteases are known to share its substrate specificity (Ullrich and Nitsche 2020; Breidenbach et al., 2021). In fact, the first target-based drugs specifically developed and approved for COVID-19 treatment, namely ensitrelvir (Mukae et al., 2022) and nirmatrelvir (Lamb 2022), are MPro inhibitors. Ensitrelvir is a non-peptidic and multi-heterocyclic molecule that inhibits MPro non-covalently, whereas nirmatrelvir is a peptidomimetic and competitive inhibitor of MPro. Nirmatrelvir requires the co-administration of ritonavir, a potent inhibitor of the major human drug-metabolizing enzyme CYP3A4 (Lamb 2022).
Here, we report a target-focused computer-aided drug repurposing campaign to detect new inhibitors of MPro, and the experimental validation of a small subset of the identified in silico hits. Noteworthy, whereas several similar efforts have been described in literature, in many (if not most) cases no experimental confirmation of the in silico hits has been reported (Eleftheriou et al., 2020; Llanos et al., 2021; Pinzi et al., 2021; Silva et al., 2021; Omer et al., 2022).
2 Methods
2.1 Model development and validation
2.1.1 Dataset compilation and curation
A dataset of compounds with reported IC50 (half maximal inhibitory concentration) against MPro or reported residual enzyme activity at 10, 20 or 50 µM concentrations was compiled from different sources. These include 18 original articles found in the specialized literature (Akshita et al., 2020; Jin et al., 2020; Ma et al., 2020; Sacco et al., 2020; Shitrit et al., 2020; Su et al., 2020; Vuong et al., 2020; Wenhao et al., 2020; Zhang et al., 2020; Bai et al., 2021; Chun-Hui et al., 2021; Franco et al., 2021; Hattori et al., 2021; Hongbo et al., 2021; Isgrò et al., 2021; Liu et al., 2021; Mody et al., 2021; Rothan and Teoh 2021), the publicly available COVID Moonshot database (Moonshot 2021), and in-house acquired data from our group. The literature search and data compilation from the COVID Moonshot database were performed in February 2021. Compounds with IC50 < 10 µM or with percentage of enzyme inhibition >50% at 10 µM were labelled as ACTIVE compounds. Compounds with IC50 > 20 μM, percentage of inhibition <80% at 20 µM or 50 μM, or percentage of enzyme inhibition <50% at 10 µM were labelled as INACTIVE compounds. The dataset compounds, represented in SMILES format, were standardized through an in-house script using the Molecule Validation and Standardization (MolVS) package (MolVS 2021), by applying the following actions: the largest organic fragment of the molecule is chosen by fragment_parent method (e.g., in the case of organic salts), the most common isotopes are assigned to the atoms by isotope_parent method, the structure is neutralized by isotope_parent method, and stereochemistry information is removed by stereo_parent method of MolVS (as only conformation-independent molecular descriptors will be included in the models, as explained later). The in-house script for standardization is provided as Supplementary Material (a Python file is available upon request to the corresponding authors). Duplicated data and compounds with inconsistent labels across different sources were removed. Since only 0D-2D molecular descriptors would be used for modeling purposes, when data associated to different optical isomers were reported, only one of them was kept whenever both isomers belonged to the same activity class, and the compounds were disregarded if the isomers belonged to different activity classes. A total of 134 active compounds and 281 inactive compounds remained in the curated dataset. The heatmap shown in the Supplementary Material (Supplementary Figure S1) exhibits the molecular diversity of the dataset. The dataset compounds that compose the training, test and validation sets are provided as Supplementary Material, in csv format (Training_set_MPRO.csv, Test_set_MPRO.csv and Validation_set_MPRO.csv, respectively).
2.1.2 Dataset splitting into training and test sets
The dataset was representatively divided into three different sets: a training set, used to train quantitative structure-activity relationship (QSAR) classifiers; a test set, used for the validation of the individual QSAR models and to select which models (and how) would be combined into a model ensemble; and a validation set, used to assess the performance of model ensembles. It has been observed that representative sampling of datasets into training and validation sets tends to produce models with better predictivity estimations, especially when training and validation sets of different sizes are sampled (Golbraikh et al., 2003; Leonard and Roy, 2006; Martin et al., 2012), possibly because in this way the compositions of the training and validation sets are as diverse as possible. For that purpose, we resorted to iterative Random subspace Principal Component Analysis clustering (iRaPCA; Prada Gori et al., 2022). Briefly, iRaPCA is based on an iterative procedure that combines feature bagging on the pool of conformation-independent Mordred descriptors (Moriwaki et al., 2018), dimensionality reduction through Principal Component Analysis (PCA) and clustering through the K-means algorithm. One hundred randomly sampled subsets of 200 descriptors each are generated from a pool of 1,613 Mordred descriptors; feature normalization is performed using the MinMaxScaler function of scikit-learn (Pedregosa et al., 2011). Correlated descriptors (Pearson coefficient above 0.4) are subsequently removed from each random descriptor subset. PCA is then conducted for each subset to obtain the first two principal components. Subsequently, the K-means algorithm is applied to each subset, systematically varying the number of clusters (K) from 2 to 25. The scikit-learn implementation of the algorithm screens 10 different randomly selected initial centroid seeds per cluster and chooses the one that minimizes the within-cluster distance. The so formed clusters are evaluated by calculating the Silhouette score (SIL) (Rouseeuw, 1987) for each K value and each descriptor subset pair: the K value that provides the highest SIL is selected. After a round of clustering is completed, the molecules in a cluster/total clustered molecules ratio is calculated for each cluster. Clusters that exceed a 0.4 ratio value are subjected to a new clustering round with the same initial parameters. Clusters that do not exceed the selected cutoff are kept as they are. Compounds from the ACTIVE and INACTIVE categories were clustered separately.
2.1.3 Molecular descriptor calculation, modelling procedure and model validation
1,613 conformation-independent descriptors were computed using the Mordred package (Moriwaki et al., 2018). Descriptors with a variance below 0.05 across the training set were excluded from the descriptor pool. A random subspace approach (Yu et al., 2012; El Habib Daho and Chikh 2015) was applied on the remaining ones to obtain 1,000 subsets of 200 descriptors each. It was observed that increasing the number of random subsets up to 10,000 did not provide any benefit in terms of early enrichment metrics such as the Boltzmann-Enhanced Discrimination of ROC (BEDROC; ROC: Receiver Operating Characteristic) and the Enrichment Factor in the top-ranked 1% (EF0.01). Moreover, a higher number of descriptors per random subset was not allowed to reduce the probability of spurious relationships (Topliss and Costello 1972). Highly correlated descriptors (Pearson correlation above 0.85) were not allowed within a given subset. A dummy dependent variable was introduced, which took values of 1 for compounds within the ACTIVE class and values of 0 for compounds belonging to the INACTIVE class. 1,000 linear classifiers, one per subset, were obtained using a Forward Stepwise procedure. A maximum of 16 descriptors per model was allowed to prevent over-parametrization and overfitting (only 1 molecular descriptor per 10 training examples was allowed into the model).
The probability of spurious correlations and the robustness of the models were assessed through randomization and Leave-Group-Out (LGO) cross-validation. In each LGO round, random stratified subsets of 10% of the total training examples were removed from the training set. 500 randomizations and 500 LGO folds were considered. The results were reported as the average accuracy across the 500 rounds and compared with the accuracy of the model inferred from the original training set, and also to the No-Model error rate (NOMER) (Gramatica 2013). The predictive ability of each individual model was finally assessed through external validation.
2.1.4 Retrospective screens
To estimate the enrichment performance of our models in a realistic virtual screening setting, two retrospective virtual screening experiments were performed. The first retrospective screen was performed by seeding the test set among a high number of decoys generated using the LIDEB’s Useful Decoys (LUDe) tool (Fallico et al., 2022). LUDe is conceptually similar to the Directory of Useful Decoys enhanced (Mysinger et al., 2012), but additional filters have been implemented to assure the topological dissimilarity between the decoys and the active compounds that are used as queries. Different enrichment metrics have been calculated to assess the enrichment behavior of the models: the Area Under the Receiver Operating Characteristic curve (AUC ROC), BEDROC, the Area Under the Precision Recall curve (AUPR), and EF0.01 (Truchon and Bayly 2007; Saito and Rehmsmeier 2015). The best-performing individual models in this first screening were combined as described in the following subsection, and the performance of the resulting ensembles was assessed using a second retrospective screen, where the validation set was seeded among a large number of decoys, also obtained via LUDe. The distribution of the enrichment metrics was estimated using stratified random sampling without replacement (75% of the libraries used in the retrospective screens were sampled, 100 times). Because normality and/or equal variances assumptions were not met by two of the enrichment metrics used, the performances of individual models and the best model ensemble were statistically compared using the Yuen-Welch test (Wilcox, 2012).
2.1.5 Ensemble learning
Given that combination of individual classifiers into meta-classifiers frequently provides better predictivity (Min 2016; Hyun et al., 2020), we have selectively combined the best individual classifiers, judging from the performance in the first retrospective screen. Five different combination schemes were tried: the average (AVE), minimum (MIN), and product (PROD) score, the average ranking (RANK) provided by the ensembled model, and the average vote (VOT) as computed by Zhang and Muegge (2006). MIN assigns, as the ensemble score, the lowest score across ensembled models. AVE provides as the ensemble score the mean of all the scores of the ensembled models. PROD provides, as score, the product of each of the combined models’ individual scores. RANK computes the average ranking provided by the ensembled models in the screened chemical library. Finally, the vote provided by each ensembled model i to a compound j is computed as voteij = max [0, int (11 −rankij/0.02N)], where rankij corresponds to the ranking assigned though model i to compound j when the screened library is ordered in descending order according to the model i score, and N is the number of compounds in the entire screened library. This procedure gives 10 votes to the first 2% of the ranked compounds, 9 votes for the next 2%, and so on. At last, for compounds in the 18%–20% rank bracket, 1 vote is given. Compounds in the bottom 80% of the ranked list receive no vote. The votes given for each compound by each individual models are then averaged over all models in the ensemble.
2.2 Prospective virtual screening
The model ensemble that showed the best performance in the second retrospective screen was used in the prospective screening of three repurposing-oriented chemical libraries: DrugBank 5.1.6 (Wishart et al., 2018), the Drug Repurposing Hub (Corsello et al., 2017), and SweetLead (Novick et al., 2013). The compounds in each database were standardized as previously described for the datasets. The optimal cutoff value for the ensemble score was chosen by analyzing Positive Predictive Value (PPV) surfaces (Bélgamo et al., 2020). As a final selection criterion, we assessed whether the in silico hits belonged to the applicability domain of the model, using the leverage approach (Yasri and Hartsough 2001), with 3d/n defined as critical value, d being the number of descriptors included in each model and n being the number of training set compounds.
2.3 Expression and purification of SARS-CoV-2 recombinant proteases
The expression and purification of the recombinant form of SARS-CoV-2 MPro and the Papain-like protease (PLPro) were conducted as essentially described in Zhang et al., 2020 and Shin et al., 2020, respectively. For MPro, the fractions eluted from the Mono Q column containing recombinant protein with high purity were pooled and subjected to buffer exchange (20 mM Tris, 150 mM NaCl, 1 mM EDTA, 1 mM DTT, pH 7.8) using a PD-10 desalting column. For PLPro, the affinity chromatography was performed using a Nickel (His-trap, GE-Healthcare) instead of a cobalt-based (TALON) column.
2.4 Protease screening and/or kinetic assays
2.4.1 MPro
MPro activity was determined by de-quenching of Edans fluorescence (5-((2-Aminoethyl)amino)naphthalene-1-sulfonic acid) upon proteolytic cleavage of a synthetic peptide (Dabcyl-KTSAVLQ↓SGFRKM-E(Edans)-NH2; United Biosystems-USA). The assay was performed in a 96-well black microplate (total assay volume 200 μl) and read using a Varioskan Lux microplate reader (Ex/Em = 340 nm/490 nm). Different parameters were routinely controlled for validating the assay (signal to background ratio >7, Z′ factor > 0.75 and relative fluorescence units >10). All samples were analyzed at least in duplicate.
For compound screening, the drugs (25 μM; Haloxon and INT131 were purchased from SIGMA whereas the synthesis of atpenin and tinostamustine was ordered to InvivoChem) were incubated with MPro (90 nM) in reaction buffer (Tris 20 mM, pH 7.8, 150 mM NaCl, 1 mM EDTA, 5% v/v DMSO) for 60 min at 25°C. Then, the peptidic substrate (5 μM) was added and fluorescence monitored for at least 30 min. Blank (reaction buffer + substrate), full-activity (Mpro + substrate) and inhibition (Mpro treated with 25 μM ebselen or acetamide + substrate) controls were run in parallel. Due to the intrinsic fluorescence of tinotamustine that overlaps that of the substrate, protease inhibition was assessed from the slope of the reaction and not from end-point measurements. Drugs that under such conditions inhibited Mpro activity ≥50% were considered hits and their IC50 values determined by measuring enzyme activity at different compound concentrations (7–8 concentration points from 25 to 0.0034 μM prepared in serial dilutions) and under the conditions described above. The data were fitted to the best linear or nonlinear equations using GraphPad Prism software (version 6.0) to obtain the IC50.
To evaluate the effect of the hits on Mpro KM (Michaelis´ constant: substrate concentration at which the reaction rate is half of its maximal value) and Vmax (maximal rate of the reaction), the initial velocity (60 s) of the enzyme-catalyzed reaction was determined at six different substrate concentrations (from 3.5 to 350 µM) while the concentration of atpenin (50 µM), tinostamustine (100 µM) and enzyme (0.1 µM) was fixed. The assay was performed in the same reaction buffer used for compound screening, in duplicate samples, and in a 384-well black microplate (total assay volume 100 μl). For kinetic measurements, a calibration curve and inner-filter effect corrections were applied following the procedures described in detail in (Zhang et al., 2020). Fluorescence was measured using a Varioskan Lux microplate reader (Ex/Em = 340 nm/490 nm) and data analyzed with Origin-Pro software.
For determining if the inhibition of MPro by tinotamustine is reversible or irreversible, 1 μM of the protease was incubated (1 h at 25°C) in reaction buffer in the absence (control sample) or presence of an excess of the drug that produces a ∼50% enzyme inhibition. Upon assessing the remaining enzyme activity (assay conditions described in the second paragraph, above), both samples were subjected to three cycles of diafiltration (Amicon Ultra-0.5, 10 kDa-cut-off filter) in reaction buffer. Protease concentration was determined by the bicinchoninic acid assay (standard plot prepared with bovine serum albumin: 1:2 serial dilutions from 100 to 6.25 μg/ml). MPro activity was assessed as described above except that the reaction was performed in a quartz cuvette and signal read with a Cary Eclypse Fluorescence Spectrometer (Agilent). All samples were tested in duplicate and MPro activity was normalized to protein concentration and, when corresponding, the fluorescence of tinotamustine subtracted.
2.4.2 PLPro
The assay conditions (buffer, pre-incubation, assay volume, microplate, temperature, etc.) for determining PLPro activity were almost identical to those described above for compound screening against Mpro. PLPro (0.1 µM) was pre-incubated for 60 min with compounds (25 µM in 5% DMSO v/v) and then the reaction started by adding the peptidic substrate (5 μM; Z-RLRGG↓-AMC, Bachem, Switzerland). For normalization purposes, full and null PLPro activity controls included protease samples treated with vehicle (5% v/v DMSO, 100% activity) or lacking substrate (0% activity), respectively. Product formation was measured by the increase of fluorescence (excitation/emission, 340/440 nm) on a 96-well microplate reader (Varioskan). All samples were tested in duplicate.
2.5 SARS-CoV-2 cell infection assay
The anti-SARS-CoV-2 activity of the hits was determined using a 384-wells microplate fluorescent-based cell infection assay (Jeon et al., 2020). The experiments were performed in compliance with the guidelines of the Korean National Institutes of Health, using enhanced Biosafety Level 3 (BSL-3) containment procedures in laboratories approved for use by the Korea Disease Control and Prevention Agency (KDCA). Vero cells were sourced from American Type Culture Collection (ATCC CCL-81) and grown in Dulbecco’s Modified Eagle Medium (DMEM; Welgene) supplemented with 10% v/v fetal bovine serum (FBS; Gibco) and 1X Antibiotic-Antimycotic solution (Gibco) at 37°C and a 5% CO2 atmosphere. Vero cells were seeded at 12,000 cells per well in DMEM, supplemented with 2% FBS and 1X Antibiotic-Antimycotic solution in black 384-well, μClear plates (Greiner Bio-One), 24 h prior to the experiment. Then, the compounds or reference drugs (ten-point concentrations) and SARS-CoV-2 (βCoV/KOR/KCDC03/2020; MOI = 0.0125) were added to the wells and incubation extended for additional 24 h. Chloroquine diphosphate (Sigma), remdesivir (MedChemExpress) and lopinavir (Selleckchem) were the reference drugs. After 24 h incubation, the cells were fixed and analyzed by immunofluorescence using an anti-SARS-CoV-2 nucleocapsid (N) protein antibody (Sino Biological Inc.) and an Alexa Fluor 488 goat anti-rabbit IgG (H + L) secondary antibody. Cell nucleus was stained with Hoechst 33,342 (Molecular Probes). The fluorescence microscopy images were taken with an Operetta CLS (PerkinElmer) and analyzed using Columbus™ (PerkinElmer) to quantify the number of cells and infection ratios. Antiviral activity is normalized to positive (mock no virus with 0.5% v/v DMSO) and negative (virus with 0.5% v/v DMSO) controls in each assay plate. IC50 values were calculated from data fit to sigmoidal equations using XLfit (Version 5.5) or GraphPad Prism (Version 8) Software. The quality of each assay was controlled by Z′-factor and the coefficient of variation in percent (%CV).
3 Results
3.1 Ensemble learning led to improved behaviour in retrospective screens
Table 1 shows the composition of the training, test, and validation sets, and how the test and validation sets, respectively, were enriched with putative inactive compounds to provide the chemical libraries used in retrospective screening 1 (to validate the enrichment performance of individual models and to train the model ensembles) and retrospective screening 2 (to validate the enrichment performance of the ensembles).

TABLE 1. Active and inactive compound composition of the training, test and validation sets. The test and validation sets were expanded with decoys to provide chemical libraries to be used in retrospective screens 1 and 2, respectively. Ya (yield of active compounds) refers to the proportion of active compounds in the corresponding set.
1,000 individual models (i.e., 1,000 individual classifiers) were generated from the training set by applying a combination of feature bagging and Forward Stepwise on a pool of 1,613 Mordred descriptors. The individual classifiers were validated internally and externally, initially employing a score cutoff value of 0.5 to discriminate between active and inactive compounds. Internal validation results for the five best individual classifiers, according to their AUC ROC in the first retrospective screening, are summarized in Table 2. The five best individual models and the meaning of their molecular descriptors have been included as Supplementary Material.
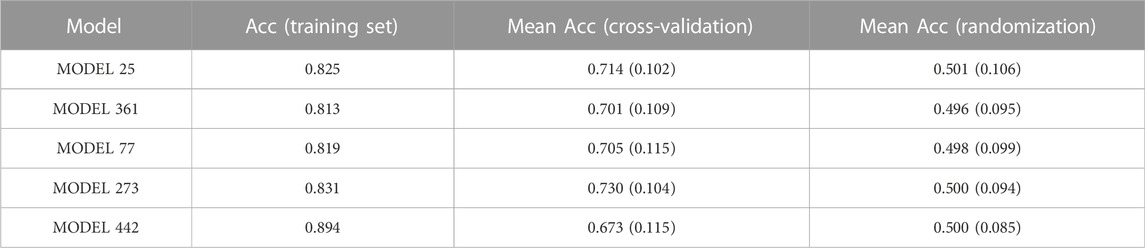
TABLE 2. Internal validation of the 5 best individual models in the first retrospective screen. For the cross-validation and randomization tests, the mean accuracy across the 500 rounds is informed; the standard deviation of the mean is presented in parentheses. The models have been ordered according to their performance in the first retrospective screening.
Due to the suboptimal results of the individual classifiers in the cross-validation, we resorted to ensemble learning, expecting to improve robustness. The performance of the individual models and the model ensembles has been comparatively assessed in two retrospective screening campaigns, where known MPro inhibitors were seeded among (known and putative) non-inhibitors. The best individual model displayed an AUC ROC of 0.934 ± 0.007, a BEDROC of 0.274 ± 0.057, an AUPR of 0.221 ± 0.038, and an EF0.01 of 0.29 ± 0.07 in the first retrospective screen, indicating that there was plenty room for improvement (note that, despite the good AUC ROC, the early enrichment metrics clearly had suboptimal values).
The systematic combination of the 2 to 100 individual models was performed, using five different operators to combine the scores of the individual models (Figure 1A). The model ensemble obtained by combining 22 models via the MIN operator (MIN-22) provided the best results across different metrics, greatly improving the early and overall enrichment. Its results in both retrospective screens are shown in Table 3; for comparative purposes, the results of the best individual model (MODEL 25) are also included.
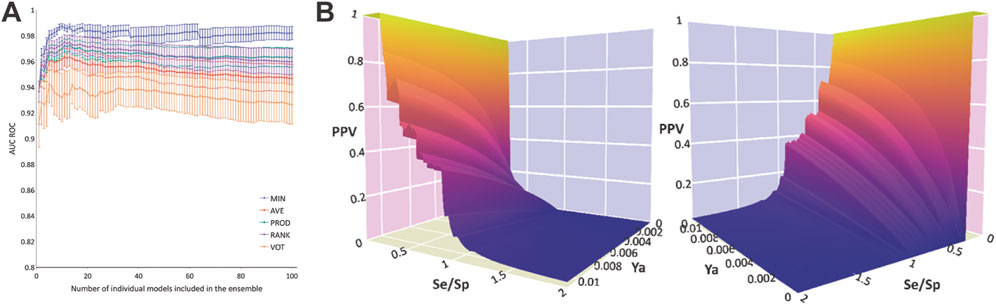
FIGURE 1. Data plots from retrospective and prospective in silico screening against MPro. (A) AUC ROC obtained in the retrospective screening as a function of the number of combined models for each operator. (B) Two different views of the PPV surface of the MIN-22 ensemble. Se/Sp refers to the Sensitivity/Specificity ratio.
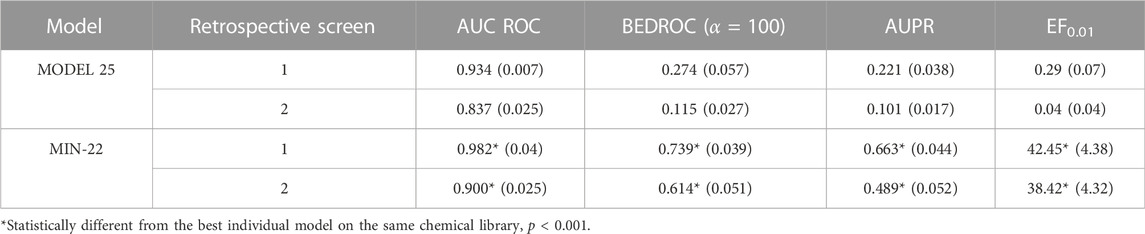
TABLE 3. Performance of the best individual model and the best model ensemble in retrospective screening experiments. The standard deviation of the enrichment metrics (obtained through bootstrapping) is presented in parentheses.
3.2 Prospective virtual screening
By analyzing PPV surfaces (Figure 1B) built upon the first retrospective screening, an optimized score cutoff value of 0.546 was chosen for the MIN-22 ensemble to identify in silico hits, corresponding to an estimated specificity of 0.998 and a minimum PPV value of 0.634 for a hypothetic Ya of 0.01. This indicates that, for that Ya, more than one every two in silico hits would confirm the prediction when submitted to experimental confirmation. If a Ya of 0.1% is assumed, the same score cutoff value would determine a minimum PPV of 0.147, meaning more than 1 in 10 in silico hits would theoretically confirm the predicted activity. Note that the true Ya of a library is ignored a priori.
The MIN-22 was applied in the virtual screening of three different chemical libraries oriented to drug repurposing, DrugBank, the Drug Repurposing Hub and Sweetlead, and 43 molecules were selected as potential inhibitors of MPro. The identity of these hits is shown in Supplementary Table S1, as well as their estimated PPV, their original indication, and whether they have already been tested against MPro according to ChEMBL (Gaulton et al., 2017), PubChem Bioassay (Wang et al., 2017) and PostEra Moonshot (about half the hits had been assayed against MPro between the time we initiated this study but before the moment of in silico hit acquisition) and if they presented any Pan-Assay INterference compoundS (PAINS) (Baell and Holloway 2010; Magalhães et al., 2021). The structures of the 43 hits are shown in the Supplementary Figure S2, together with the structures of the 80 active molecules of the Training set (Supplementary Figure S3).
3.3 Experimental validation
3.3.1 Atpenin and tinostamustine inhibit MPro but do not affect the in vitro proliferation of SARS-CoV-2
The following criteria were taken into account for selecting the in silico predicted MPro hits to be assayed against the viral proteases: i) promiscuous scaffold and PAINS were disregarded; ii) drugs with well-known molecular target(s) and physiological effects; iii) good solubility and pharmacodynamics profile; iv) whether they have been tested against the target at the moment of compound acquisition (already tested hits were disregarded); v) commercial availability; vi) price. Four candidates meeting all these desired features were purchased and tested (Figure 2).
In order to allow the detection of slow-binding and low-affinity competitive inhibition of MPro, the drugs (25 μM) were incubated for 1 h with the enzyme and, next, the reaction was started by adding the substrate to a 4-fold sub-KM concentration, respectively. Under these conditions, atpenin and tinostamustine reduced MPro activity to less than 50% whereas the inhibitory effect exerted by INT131 (22% inhibition) and haloxon (2% inhibition) was marginal and null, respectively (Table 4). The control drug ebselen, a well-known covalent inhibitor of MPro (Menéndez et al., 2020), produced full inhibition of the protease. In order to verify the specificity of the in silico approach to identify MPro inhibitors in a selective manner, the four drugs were assayed against the second cysteine (papain-like) protease of SARS-CoV-2, namely PL-Pro. Except for the promiscuous thiol-inactivating agent ebselen, a reported covalent inhibitor of PL-Pro (Weglarz-Tomczak et al., 2021), the drugs did not (INT131 and atpenin) or only marginally (17% and 21% inhibition at 25 μM for haloxon and tinotamustine, respectively) affected PL-Pro activity (Table 4). The drug concentration causing 50% inhibition of MPro was within the same order of magnitude for atpenin (IC50 = 1 μM) and tinostamustine (IC50 = 4 μM), and >20-fold lower for ebselen (IC50 = 50 nM; Table 4 and Figure 3A).
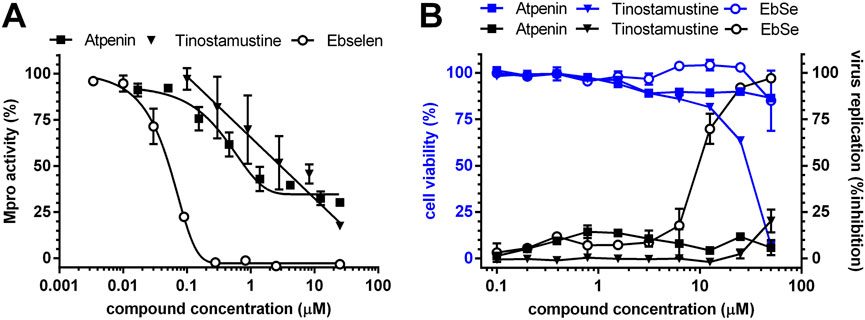
FIGURE 3. Drug testing against MPro and SARS-CoV-2. (A) Concentration-response plots for MPro inhibitors. The data were fitted to non-linear (sigmoidal, for atpenin and ebselen) or linear (tinostamustine) equations. (B) Concentration-response plots for anti-viral and cytotoxic activity against SARS-CoV-2 Vero-infected cells. Viral replication and cell viability were quantified by measuring levels of SARS-CoV-2 nucleocapsid protein and host nuclear DNA by fluorescence microscopy. For further details see Section 2.4.1 and Section 2.5.
The concentration-response curves show that atpenin is a partial inhibitor of MPro whereas the inhibition exerted by tinostamustine shows a linear correlation with drug concentration (Figure 3A), though within the concentration range tested full enzyme inhibition is not achieved.
In contrast to ebselen and tinostamustine, MPro inhibition by atpenin achieved an apparent plateau at concentrations above 1 μM (Figure 3A). This effect cannot be ascribed to a limited solubility of the compound (e.g., at concentrations higher than 5 μM) because the theoretical LogP (10-based logarithm of the octanol-water partition coefficient of a molecule) and LogS (10-based logarithm of the water solubility of a molecule) values for atpenin (2.89 and −2.88, respectively) are lower than those estimated for tinostamustine (3.92 and −2.97, respectively), a compound not showing saturation of enzyme inhibition within a three-orders of magnitude concentration range. Moreover, atpenin entails only a minor reduction (16%) in MPro’s Vmax and apparent KM for substrate (Table 4), suggesting that this drug is an acompetitive inhibitor of MPro.
For tinotamustine, kinetic analysis revealed a 4-fold reduction in MPro reaction velocity and not significant alteration in the apparent substrate KM (Table 4), which is suggestive of a non-competitive (reversible) or an irreversible inhibition mechanism. In order to determine by which of both mechanisms the drug is inhibiting MPro, the enzyme was treated with a stoichiometry excess of tinotamustine that yields ∼50% enzyme inhibition, and then the compound was removed by diafiltration. Before and after ultrafiltration the activity of untreated and tinomastutine-treated MPro was 100% ± 14% and 43% ± 9%, and 100% ± 9% and 46% ± 6%, respectively. Since MPro activity could not be restored upon removal of the drug, this result indicates that tinostamustine exerts an irreversible inhibition of the viral protease.
The capacity to inhibit the proliferation of SARS-CoV-2 (Wuhan strain) was assayed for atpenin and tinostamustine in a cell infection model. Ebselen was included as compound control whereas chloroquine, lopinavir, and remdesivir were included as clinical drug controls. All molecules were tested at concentrations embracing three-orders of magnitude. As shown in Figure 3B, at none of the concentrations tested, atpenin and tinostamustine significantly affected the proliferation of SARS-CoV-2. In contrast, ebselen (IC50 = 9.7 μM) and the control clinical drugs (plots not shown; IC50 = 10.4 μM for chloroquine, IC50 = 14.2 μM for lopinavir, and IC50 = 9.9 μM for remdesivir) displayed anti-viral activity in the low micro-molar range. In contrast with atpenin and ebselen, tinostamustine resulted markedly cytotoxic at concentrations above 10 μM (Figure 3B).
4 Discussion
As shown in Table 2, the overall percentage (accuracy, Acc) of good classifications of the best performing individual models was acceptable (around 80%) for the training set. In all cases, the mean Acc of the randomized models was similar to the NOMER, which is 0.5 for our balanced training set. These results clearly demonstrate the low probability of spurious relationships. Regarding the cross-validation, the mean Acc across the folds falls about 10% (compared to the Acc on the training set) for four of the five best performing classifiers, suggesting some degree of overfitting and suboptimal robustness. For one of the individual models (model 442) this behavior is accentuated, as it displays the highest Acc on the training examples (0.894) but the lowest mean accuracy in the cross-validation (0.673). This justified resorting to model combination and it was convincingly shown that the selective combination of individual models into a meta-classifier improved the predictive ability, especially in terms of early enrichment in the retrospective screening experiments.
The in silico approach applied for the identification of inhibitors of MPro proved successful in identifying molecules with selective activity against the viral protease. Two drugs, a cardio-protective agent (atpenin) and an anti-cancer agent (tinostamustine) agent, out of a minor number (four) of candidates tested displayed low µM inhibitory activity and different inhibition mechanisms towards Mpro from SARS-CoV-2. Some key points in the modelling procedure that could explain the success of our virtual screening campaign are:
a) A careful curation of the database from which the linear classifiers were inferred (following the well-known “garbage in, garbage out” principle in computer science, which states that flawed input data produces poor output);
b) The fact that we chose to infer model classifiers, based on a binary classification of the training examples into “active” and “inactive” categories, rather than regression models aimed at fitting and predicting a quantitative measure of activity, e.g., Ki or pIC50. Moreover, we employed a “safety” window to label active and inactive compounds: for those data points with reported IC50 values, compounds with IC50 < 10 µM were flagged as ACTIVE compounds, and compounds with IC50 > 20 µM were considered as INACTIVE. In our opinion, these two decisions helped to mitigate noise related to mislabeling and inter-laboratory variability in the observed response (note that our dataset gathered data obtained in different labs).
c) The use of ensemble learning, which is known to improve the reliability of the predictions. Remarkably, the chosen model ensemble for the prospective virtual screen was based on the MIN operator, which is quite a conservative way of combining the ensembled models: if only one model provides a low score to a given compound, the compound will be labeled as inactive. In other words, an ensemble based on this operator will only provide a high score for a compound if all the combined models provide a high score, i.e., if they agree in the prediction made;
d) Finally, we believe that a critical point of our protocol is the use of two retrospective screening experiments to assess the enrichment behavior of the individual models and the model ensembles. For such purpose, we used not only to enrichment metrics that reflect the average enrichment (AUC ROC) but also early enrichment metrics (BEDROC, EF0.01), the latter being the most critical when performing a virtual screening campaign (Truchon and Bayly 2007).
Atpenin is an antifungal antibiotic isolated from Penicillium sp., with high affinity for the ubiquinone-binding site of succinate dehydrogenase (Miyadera et al., 2003). Due to the potent inhibition exerted by atpenin on the mitochondrial complex II, this drug has been used as cardioprotective agent for counteracting ischemia-reperfusion injury (Wojtovich and Brookes. 2009; Dröse et al., 2011). Preliminary kinetic characterization suggests that MPro inhibition by atpenin is acompetitive. SARS coronavirus MPro has been shown to undergo important conformational changes during and pre-catalysis, the last including substrate-induced protein dimerization (Chang. 2009; Wu et al., 2013; Zhang et al., 2020). Thus, a possible mechanism by which atpenin affects MPro activity may involve a perturbation of the monomer/dimer equilibrium (e.g. increase in dimer KD) and/or in the cooperativity between the dimer subunits.
Tinostamustine is a fusion molecule that combines the strong DNA damaging effect of bedamustine (Cheson and Leoni, 2011) with the pan-histone deacetylase inhibitor moiety of vorinostat, providing a first-in-class alkylating deacetylase inhibitor (Festuccia et al., 2018). Our experimental data is consistent with an irreversible inhibition of the viral protease by tinotamustine. Solvent accessible and un-protonated cysteine residues (i.e., thiolate) of MPro qualify as nucleophilic targets for alkylation by tinostamustine. From the five cysteines in thiolate state present in MPro (Cys22, 38, 44, 128, and 145), three of them are located close to the protein surface: Cys22, Cys128, and Cys145 (Kneller et al., 2020). Since kinetic assays with tinostamustine suggested a non-competitive inhibition of MPro (impairment in Vmax and not in substrate KM), the catalytic Cys145 is a top candidate for, probably, irreversible modification by this drug. Nonetheless, further assays are needed to elucidate if tinostamustine acts as a covalent or tight-binding (non-covalent) inhibitor of MPro.
The lack of antiviral activity observed for atpenin and tinostamustine could be explained by the impossibility, under our assay conditions, of reaching effective concentrations at intracellular level able to affect viral replication and not cell viability within 24 h. This requirement is fulfilled by ebselen, a control compound that inhibited by 50% the replication of SARS-CoV-2 at a concentration 200-folds higher than its IC50 against MPro (0.049 μM; Table 4). Assuming a similar behavior for atpenin (IC50 for MPro = 0.98 μM) and tinostamustine (IC50 for MPro = 4 μM), the antiviral EC50 would be 196 and 800 μM, respectively. These values are far higher than the maximal concentration tested (50 μM).
An additional factor that may contribute to the observed low in cellulo activity of the compounds might relate to the conditions of our infection assay, which is very demanding in terms of favoring the detection of highly active molecules. For instance, i) it relies on Vero cells, a cell line where SARS-CoV-2 (Wuhan strain) has been shown to display maximal replication kinetics compared to human colon (CaCo-2) or lung (Calu-3) cells (Mautner et al., 2022), ii) viral infection and compound treatment is initiated simultaneously, and iii) viral replication is determined after 24 h incubation. In line with this statement, and supporting our current finding that atpenin is targeting an essential viral protein, a recent study in pre-print (Renz et al., 2022) reported an EC50 of 0.45 and 0.68 μM for atpenin A5 against two SARS-CoV-2 strains infecting Calu-3 cells treated for 24 h prior to infection with the compound and then incubated for additional 48 h before assessing viral load (total assay time = 72 h).
With respect to tinotamustine, a novel repurposing strategy for COVID-19 emerged recently. The accumulation of highly acetylated histones induced by this drug (and compounds with a similar mode of action) results in induction of chromatin remodeling and modulation of gene expression, which has been shown to reset the deregulated immune reaction observed in severe COVID-19, particularly by lowering the uncontrolled inflammatory response (Ripamonti et al., 2022). Thus, the potential polypharmacological effect of tinotamustine in COVID-19, i.e., amelioration of host’s cytokine storm and inhibition of viral MPro, merits further investigation.
In conclusion, our results pave the way towards a retrospective examination of analogues (Selby et al., 2010; Krautwald et al., 2016; Wang H et al., 2017) and building-blocks (Mehrling and Chen 2016) of atpenin and tinotamustine. On the other hand, the high predictive rate of the in silico method applied to identify SARS-CoV-2 MPro hits, prompt to extent the experimental screenings towards the remaining in silico candidates.
Data availability statement
The datasets used for the in silico studies (training and validation data) have been released in the Supplementary Material as .csv files.
Author contributions
Conceptualization, AT and MAC; Formal analysis, DNPG, SR, MF, LNA, CLB, AT, DS, MAC; Investigation: DNPG, SR, MF, LNA, SP, JH, HL, K-HPP; Writing—original draft preparation: AT, MAC; Writing—review and editing: DNPG, MF, SP, K-HPP, OP, DS, MAC; Supervision: AT, DS, MAC; Funding acquisition: DS, MAC. All authors have read and agreed to the published version of the manuscript.
Funding
The financial support of the Urgence COVID-19 Fundraising Campaign of Institut Pasteur and of FOCEM (Fondo para la Convergencia Estructural del Mercosur, Grant Number COF 03/11) is gratefully acknowledged. Additional support was provided by the National Research Foundation of Korea (NRF) grant funded by the Korea Government (MSIT, No. NRF-2017M3A9G6068254).
Acknowledgments
We thank Hilgenfeld R. (German Center for Infection Research, University of Lübeck, Germany) and Dikic (Max Planck Institute of Biophysics, Frankfurt, Germany) for providing the MPro and PLPro expression vectors, respectively. SR acknowledges the support of the Consejo Nacional de Investigaciones Científicas y Técnicas (CONICET, Argentina) for postdoctoral fellowship.
Conflict of interest
The authors declare that the research was conducted in the absence of any commercial or financial relationships that could be construed as a potential conflict of interest.
Publisher’s note
All claims expressed in this article are solely those of the authors and do not necessarily represent those of their affiliated organizations, or those of the publisher, the editors and the reviewers. Any product that may be evaluated in this article, or claim that may be made by its manufacturer, is not guaranteed or endorsed by the publisher.
Supplementary material
The Supplementary Material for this article can be found online at: https://www.frontiersin.org/articles/10.3389/fddsv.2022.1082065/full#supplementary-material
References
Akshita, G., Chitra, R., Pradeep, P., Viswanathan, V., Naval, V., Punit, K., et al. (2020). Structure-based virtual screening and biochemical validation to discover a potential inhibitor of the SARS-CoV-2 main protease. ACS Omega 5, 33151–33161. doi:10.1021/acsomega.0c04808
Ashburn, T., and Thor, K. (2004). Drug repositioning: Identifying and developing new uses for existing drugs. Nat. Rev. Drug Discov. 3, 673–683. doi:10.1038/nrd1468
Baell, J. B., and Holloway, G. A. (2010). New substructure filters for removal of pan assay interference compounds (PAINS) from screening libraries and for their exclusion in bioassays. J. Med. Chem. 53, 2719–2740. doi:10.1021/jm901137j
Bai, Y., Ye, F., Feng, Y., Liao, H., Song, H., Qi, J., et al. (2021). Structural basis for the inhibition of the SARS-CoV-2 main protease by the anti-HCV drug narlaprevir. Sig. Transduct. Target Ther. 6, 51. doi:10.1038/s41392-021-00468-9
Bélgamo, J. A., Alberca, L. N., Pórfido, J. L., Caram Romero, F. N., Rodríguez, S., Talevi, A., et al. (2020). Application of target repositioning and in silico screening to exploit fatty acid binding proteins (FABPs) from Echinococcus multilocularis as possible drug targets. J. Comput. Aided Mol. Des. 34, 1275–1288. doi:10.1007/s10822-020-00352-8
Bellera, C. L., Llanos, M., Gantner, M. E., Rodriguez, S., Gavernet, L., Comini, M., et al. (2021). Can drug repurposing strategies be the solution to the COVID-19 crisis? Expert Opin. Drug Discov. 16, 605–612. doi:10.1080/17460441.2021.1863943
Breidenbach, J., Lemke, C., Pillaiyar, T., Schäkel, L., Al Hamwi, G., Diett, M., et al. (2021). Targeting the main protease of SARS-CoV-2: From the establishment of high throughput screening to the design of tailored inhibitors. Angew. Chem. Int. Ed. Engl. 60, 10423–10429. doi:10.1002/anie.202016961
Chakraborty, C., Sharma, A. R., Bhattacharya, M., Agoramoorthy, G., and Lee, S. S. (2021). The drug repurposing for COVID-19 clinical trials provide very effective therapeutic combinations: Lessons learned from major clinical studies. Front. Pharmacol. 12, 704205. doi:10.3389/fphar.2021.704205
Chang, G. G. (2009). Quaternary structure of the SARS coronavirus main protease. Mol. Biol. SARS-Coronavirus, 115–128. doi:10.1007/978-3-642-03683-5_8
Chen, X., Gong, W., Wu, X., and Zhao, W. (2021). Estimating economic losses caused by COVID-19 under multiple control measure scenarios with a coupled infectious disease-economic model: A case study in wuhan, China. Int. J. Environ. Res. Public. Health. 18, 11753. doi:10.3390/ijerph182211753
Cheson, B. D., and Leoni, L. (2011). The evolving role of bendamustine in lymphoid malignancy: Understanding the drug and its mechanism of action—introduction. Clin. Adv. Hematol. Oncol. 9 (19), 1–S3. doi:10.1053/j.seminhematol.2011.03.001
Chun-Hui, Z., Stone, E. A., Deshmukh, M., Ippolito, J. A., Ghahremanpour, M. M., Tirado-Rives, J., et al. (2021). Potent noncovalent inhibitors of the main protease of SARS-CoV-2 from molecular sculpting of the drug perampanel guided by free energy perturbation calculations. ACS Cent. Sci. 7, 467–475. doi:10.1021/acscentsci.1c00039
Corsello, S. M., Bittker, J., Liu, Z., Gould, J., McCarren, P., Hirschman, J. E., et al. (2017). The drug repurposing Hub: A next-generation drug library and information resource. Nat. Med. 23, 405–408. doi:10.1038/nm.4306
Dröse, S., Bleier, L., and Brandt, U. (2011). A common mechanism links differently acting complex II inhibitors to cardioprotection: Modulation of mitochondrial reactive oxygen species production. Mol. Pharmacol. 79, 814–822. doi:10.1124/mol.110.070342
El Habib Daho, M., and Chikh, M. A. (2015). Combining bootstrapping samples, random subspaces and random forests to build classifiers. J. Med. Imag. Health Inf. 5, 539–544. doi:10.1166/jmihi.2015.1423
Eleftheriou, P., Amanatidou, D., Petrou, A., and Geronikaki, A. (2020). In silico evaluation of the effectivity of approved protease inhibitors against the main protease of the novel SARS-CoV-2 virus. Molecules 25, 2529. doi:10.3390/molecules25112529
Fallico, M., Alberca, L. N., Prada Gori, D. N., Gavernet, L., and Talevi, A. (2022). “Machine learning search of novel selective NaV1.2 and NaV1.6 inhibitors as potential treatment against dravet syndrome,” in Computational neuroscience. LAWCN 2021. Editors P. R. d. A. Ribeiro, V. R. Cota, D. A. C. Barone, and A. C. M. de Oliveira (Cham, Switzerland: Communications in Computer and Information Science), 1519, 101–118. doi:10.1007/978-3-031-08443-0_7
Festuccia, C., Mancini, A., Colapietro, A., Gravina, G. L., Vitale, F., Marampon, F., et al. (2018). The first-in-class alkylating deacetylase inhibitor molecule tinostamustine shows antitumor effects and is synergistic with radiotherapy in preclinical models of glioblastoma. J. Hematol. Oncol. 11, 32. doi:10.1186/s13045-018-0576-6
Franco, L. S., Maia, R. C., and Barreiro, E. J. (2021). Identification of LASSBio-1945 as an inhibitor of SARS-CoV-2 main protease (MPRO) through in silico screening supported by molecular docking and a fragment-based pharmacophore model. RSC Med. Chem. 12, 110–119. doi:10.1039/D0MD00282H
Gaulton, A., Hersey, A., Nowotka, M., Bento, A. P., Chambers, J., Mendez, , et al. (2017). The ChEMBL database in 2017. Nucleic Acids Res. 45, D945–D954. doi:10.1093/nar/gkw1074
Golbraikh, A., Shen, M., Xiao, Z., Xiao, Y. D., Lee, K. H., and Tropsha, A. (2003). Rational selection of training and test sets for the development of validated QSAR models. J. Comput. Aided Mol. Des. 17, 241–253. doi:10.1023/A:1025386326946
Gramatica, P. (2013). On the development and validation of QSAR models. Methods Mol. Biol. 930, 499–526. doi:10.1007/978-1-62703-059-5_21
Hattori, Si., Higashi-Kuwata, N., Hayashi, H., Allu, S. R., Raghavaiah, J., Bulut, H., et al. (2021). A small molecule compound with an indole moiety inhibits the main protease of SARS-CoV-2 and blocks virus replication. Nat. Commun. 12, 668. doi:10.1038/s41467-021-20900-6
Hongbo, L., Fei, Y., Qi, S., Hao, L., Chunmei, L., Siyang, L., et al. (2021). Scutellaria baicalensis extract and baicalein inhibit replication of SARS-CoV-2 and its 3C-like protease in vitro. J. Enzyme Inhibition Med. Chem. 36, 497–503. doi:10.1080/14756366.2021.1873977
Hyun, J. C., Kavvas, E. S., Monk, J. M., and Palsson, B. O. (2020). Machine learning with random subspace ensembles identifies antimicrobial resistance determinants from pan-genomes of three pathogens. PLoS Comput. Biol. 16, e1007608. doi:10.1371/journal.pcbi.1007608
Isgrò, C., Sardanelli, A. M., and Palese, L. L. (2021). Systematic search for SARS-CoV-2 main protease inhibitors for drug repurposing: Ethacrynic acid as a potential drug. Viruses 13, 106. doi:10.3390/v13010106
Jeon, S., Ko, M., Lee, J., Choi, I., Byun, S. Y., Park, S., et al. (2020). Identification of antiviral drug candidates against SARS-CoV-2 from FDA-approved drugs. Antimicrob. Agents Chemother. 64, e00819–e00820. doi:10.1128/AAC.00819-20
Jin, Z., Du, X., Xu, Y., Deng, Y., Liu, M., Zhao, Y., et al. (2020). Structure of Mpro from SARS-CoV-2 and discovery of its inhibitors. Nature 582, 289–293. doi:10.1038/s41586-020-2223-y
Kneller, D. W., Phillips, G., Weiss, K. L., Pant, S., Zhang, Q., O'Neill, H. M., et al. (2020). Unusual zwitterionic catalytic site of SARS-CoV-2 main protease revealed by neutron crystallography. J. Biol. Chem. 295, 17365–17373. doi:10.1074/jbc.AC120.016154
Krautwald, S., Nilewski, C., Mori, M., Shiomi, K., Ōmura, S., and Carreira, E. M. (2016). Bioisosteric exchange of Csp3 -chloro and methyl substituents: Synthesis and initial biological studies of atpenin A5 analogues. Angew. Chem. Int. Ed. Engl. 55, 4049–4053. doi:10.1002/anie.201511672
Lamb, Y. N. (2022). Nirmatrelvir plus ritonavir: First approval. Drugs 82, 585–591. doi:10.1007/s40265-022-01692-5
Leonard, J. T., and Roy, K. (2006). On selection of training and test sets for the development of predictive QSAR models. QSAR Comb. Sci. 25, 235–251. doi:10.1002/qsar.200510161
Liu, C., Boland, S., Scholle, M. D., Bardiot, D., Marchand, A., Chaltin, P., et al. (2021). Dual inhibition of SARS-CoV-2 and human rhinovirus with protease inhibitors in clinical development. Antivir. Res. 187, 105020. doi:10.1016/j.antiviral.2021.105020
Llanos, M. A., Gantner, M. E., Rodriguez, S., Alberca, L. N., Bellera, C. L., Talevi, A., et al. (2021). Strengths and weaknesses of docking simulations in the SARS-CoV-2 era: The main protease (Mpro) case study. J. Chem. Inf. Model. 61, 3758–3770. doi:10.1021/acs.jcim.1c00404
Ma, C., Sacco, M. D., Hurst, B., Townsend, J. A., Hu, Y., Szeto, T., et al. (2020). Boceprevir, GC-376, and calpain inhibitors II, XII inhibit SARS-CoV-2 viral replication by targeting the viral main protease. Cell Res. 30, 678–692. doi:10.1038/s41422-020-0356-z
Magalhães, P. R., Reis, P. B. P. S., Vila-Viçosa, D., Machuqueiro, M., and Victor, B. L. (2021). Identification of Pan-Assay INterference compoundS (PAINS) using an MD-based protocol. Methods Mol. Biol. 2315, 263–271. doi:10.1007/978-1-0716-1468-6_15
Martin, T. M., Harten, P., Young, D. M., Muratov, E. N., Golbraikh, A., Zhu, H., et al. (2012). Does rational selection of training and test sets improve the outcome of QSAR modeling? J. Chem. Inf. Model. 52, 2570–2578. doi:10.1021/ci300338w
Mautner, L., Hoyos, M., Dangel, A., Berger, C., Ehrhardt, A., and Baiker, A. (2022). Replication kinetics and infectivity of SARS-CoV-2 variants of concern in common cell culture models. Virol. J. 19 (1), 76. doi:10.1186/s12985-022-01802-5
Mehrling, T., and Chen, Y. (2016). The alkylating-hdac inhibition fusion principle: Taking chemotherapy to the next level with the first in class molecule edo-s101. Anticancer Agents Med. Chem. 16, 20–28. doi:10.2174/1871520615666150518092027
Menéndez, C. A., Byléhn, F., Perez-Lemus, G. R., Alvarado, W., and de Pablo, J. J. (2020). Molecular characterization of ebselen binding activity to SARS-CoV-2 main protease. Sci. Adv. 6, eabd0345. doi:10.1126/sciadv.abd0345
Min, S. H. (2016). A genetic algorithm-based heterogeneous random subspace ensemble model for bankruptcy prediction. Int. J. Appl. Eng. Res. 11, 2927–2931.
Miyadera, H., Shiomi, K., Ui, H., Yamaguchi, Y., Masuma, R., Tomoda, H., et al. (2003). Atpenins, potent and specific inhibitors of mitochondrial complex II (succinate-ubiquinone oxidoreductase). Proc. Natl. Acad. Sci. U. S. A. 100, 473–477. doi:10.1073/pnas.0237315100
Mody, V., Ho, J., Wills, S., Mawri, A., Lawson, L., Ebert, M. C. C. J. C., et al. (2021). Identification of 3-chymotrypsin like protease (3CLPro) inhibitors as potential anti-SARS-CoV-2 agents. Commun. Biol. 4, 93. doi:10.1038/s42003-020-01577-x
MolVS (2021). Molecule validation and standardization. Available at: https://molvs.readthedocs.io/en/latest/.
Moonshot (2021). Covid.Postera. Available at: https://covid.postera.ai/covid/activity_data (Accessed February 18, 2021).
Moriwaki, H., Tian, Y. S., Kawashita, N., and Takagi, T. (2018). Mordred: A molecular descriptor calculator. J. Cheminform. 10, 4. doi:10.1186/s13321-018-0258-y
Mukae, H., Yotsuyanagi, H., Ohmagari, N., Doi, Y., Imamura, T., Sonoyama, T., et al. (2022). A randomized phase 2/3 study of ensitrelvir, a novel oral SARS-CoV-2 3C-like protease inhibitor, in Japanese patients with mild-to-moderate COVID-19 or asymptomatic SARS-CoV-2 infection: Results of the phase 2a part. Antimicrob. Agents Chemother. 66, e0069722. doi:10.1128/aac.00697-22
Mysinger, M. M., Carchia, M., Irwin, J. J., and Shoichet, B. K. (2012). Directory of useful decoys, enhanced (DUD-E): Better ligands and decoys for better benchmarking. J. Med. Chem. 55, 6582–6594. doi:10.1021/jm300687e
Niknam, Z., Jafari, A., Golchin, A., Pouya, F. D., Nematiet, M., Rezaei-Tavirani, M., et al. (2022). Potential therapeutic options for COVID-19: An update on current evidence. Eur. J. Med. Res. 27, 6. doi:10.1186/s40001-021-00626-3
Novick, P. A., Ortiz, O. F., Poelman, J., Abdulhay, A. Y., and Pande, V. S. (2013). Sweetlead: An in silico database of approved drugs, regulated chemicals, and herbal isolates for computer-aided drug discovery. PLoS ONE 8, e79568. doi:10.1371/journal.pone.0079568
Omer, S. E., Ibrahim, T. M., Krar, O. A., Ali, A. M., Makki, A. A., Ibraheem, W., et al. (2022). Drug repurposing for SARS-CoV-2 main protease: Molecular docking and molecular dynamics investigations. Biochem. Biophys. Rep. 29, 101225. doi:10.1016/j.bbrep.2022.101225
Pedregosa, F., Varoquaux, G., Gramfort, A., Michel, V., Thirion, B., Grisel, O., et al. (2011). Scikit-learn: Machine learning in Python. J. Mach. Learn. Res. 12, 2825–2830. doi:10.5555/1953048.2078195
Pinzi, L., Tinivella, A., Caporuscio, F., and Rastelli, G. (2021). Drug repurposing and polypharmacology to fight SARS-CoV-2 through inhibition of the main protease. Front. Pharmacol. 12, 636989. doi:10.3389/fphar.2021.636989
Prada Gori, D. N., Llanos, M. A., Bellera, C. L., Talevi, A., and Alberca, L. N. (2022). iRaPCA and SOMoC: Development and validation of web applications for new approaches for the clustering of small molecules. J. Chem. Inf. Model. 62, 2987–2998. doi:10.1021/acs.jcim.2c00265
Renz, A., Hohner, M., Breitenbach, M., Josephs-Spaulding, J., Dürrwald, J., Best, L., et al. (2022). Metabolic modeling elucidates phenformin and atpenin A5 as broad-spectrum antiviral drugs, 2022, 100223. doi:10.20944/preprints202210.0223.v1Preprints
Ripamonti, C., Spadotto, V., Pozzi, P., Stevenazzi, A., Vergani, B., Marchini, M., et al. (2022). HDAC inhibition as potential therapeutic strategy to restore the deregulated immune response in severe COVID-19. Front. Immunol. 13, 841716. doi:10.3389/fimmu.2022.841716
Rodrigues, L., Bento Cunha, R., Vassilevskaia, T., Viveiros, M., and Cunha, C. (2022). Drug repurposing for COVID-19: A review and a novel strategy to identify new targets and potential drug candidates. Molecules 27, 2723. doi:10.3390/molecules27092723
Rothan, H. A., and Teoh, T. C. (2021). Cell-based high-throughput screening protocol for discovering antiviral inhibitors against SARS-COV-2 main protease (3CLpro). Mol. Biotechnol. 63, 240–248. doi:10.1007/s12033-021-00299-7
Rousseeuw, P. J. (1987). Silhouettes: A graphical aid to the interpretation and validation of cluster analysis. J. Comput. Appl. Math. 20, 53–65. doi:10.1016/0377-0427(87)90125-7
Sacco, M. D., Ma, C., Lagarias, P., Gao, A., Townsend, J. A., Meng, X., et al. (2020). Structure and inhibition of the SARS-CoV-2 main protease reveal strategy for developing dual inhibitors against Mpro and cathepsin L. Sci. Adv. 6, eabe0751. doi:10.1126/sciadv.abe0751
Saito, T., and Rehmsmeier, M. (2015). The precision-recall plot is more informative than the ROC plot when evaluating binary classifiers on imbalanced datasets. PloS One 10, e0118432. doi:10.1371/journal.pone.0118432
Selby, T. P., Hughes, K. A., Rauh, J. J., and Hanna, W. S. (2010). Synthetic atpenin analogs: Potent mitochondrial inhibitors of mammalian and fungal succinate-ubiquinone oxidoreductase. Bioorg. Med. Chem. Lett. 20, 1665–1668. doi:10.1016/j.bmcl.2010.01.066
Shin, D., Mukherjee, R., Grewe, D., Bojkova, D., Baek, K., Bhattacharya, A., et al. (2020). Papain-like protease regulates SARS-CoV-2 viral spread and innate immunity. Nature 587, 657–662. doi:10.1038/s41586-020-2601-5
Shitrit, A., Zaidman, D., Kalid, O., Bloch, I., Doron, D., Yarnizky, T., et al. (2020). Conserved interactions required for inhibition of the main protease of severe acute respiratory syndrome coronavirus 2 (SARS-CoV-2). Sci. Rep. 10, 20808. doi:10.1038/s41598-020-77794-5
Silva, J. R. A., Kruger, H. G., and Molfetta, F. A. (2021). Drug repurposing and computational modeling for discovery of inhibitors of the main protease (Mpro) of SARS-CoV-2. RSC Adv. 11, 23450–23458. doi:10.1039/d1ra03956c
Su, H., Yao, S., Zhao, W-f., Li, M-j., Liu, J., Shang, W-j., et al. (2020). Anti-SARS-CoV-2 activities in vitro of Shuanghuanglian preparations and bioactive ingredients. Acta Pharmacol. Sin. 41, 1167–1177. doi:10.1038/s41401-020-0483-6
Topliss, J. G., and Costello, R. J. (1972). Change correlations in structure-activity studies using multiple regression analysis. J. Med. Chem. 15, 1066–1068. doi:10.1021/jm00280a017
Truchon, J. F., and Bayly, C. I. (2007). Evaluating virtual screening methods: Good and bad metrics for the “early recognition” problem. J. Chem. Inf. Model. 47, 488–508. doi:10.1021/ci600426e
Ullrich, S., and Nitsche, C. (2020). The SARS-CoV-2 main protease as drug target. Bioorg. Med. Chem. Lett. 30, 127377. doi:10.1016/j.bmcl.2020.127377
Venkadapathi, J., Govindarajan, V. K., Sekaran, S., and Venkatapathy, S. (2021). A minireview of the promising drugs and vaccines in pipeline for the treatment of COVID-19 and current update on clinical trials. Front. Mol. Biosci. 8, 637378. doi:10.3389/fmolb.2021.637378
Vuong, W., Khan, M. B., Fischer, C., Arutyunova, E., Lamer, T., Shields, J., et al. (2020). Feline coronavirus drug inhibits the main protease of SARS-CoV-2 and blocks virus replication. Nat. Commun. 11, 4282. doi:10.1038/s41467-020-18096-2
Wang, H., Huwaimel, B., Verma, K., Miller, J., Germain, T. M., Kinarivala, N., et al. (2017). Synthesis and antineoplastic evaluation of mitochondrial complex II (succinate dehydrogenase) inhibitors derived from atpenin A5. Chem. Med. Chem. 12, 1033–1044. doi:10.1002/cmdc.201700196
Wang, H., Paulson, K. R., Pease, S. A., Watson, S., Comfort, H., Zheng, P., et al. (2022). Estimating excess mortality due to the COVID-19 pandemic: A systematic analysis of COVID-19-related mortality, 2020-21. Lancet 399, 1513–1536. doi:10.1016/S0140-6736(21)02796-3
Wang, Y., Bryant, S. H., Cheng, T., Wang, J., Gindulyte, A., Shoemaker, B. A., et al. (2017). PubChem BioAssay: 2017 update. Nucleic Acids Res. 45, D955–D963. doi:10.1093/nar/gkw1118
Watson, O. J., Barnsley, G., Toor, J., Hogan, A. B., Winskill, P., and Ghani, A. C. (2022). Global impact of the first year of COVID-19 vaccination: A mathematical modelling study. Lancet Infect. Dis. 22, 1293–1302. doi:10.1016/S1473-3099(22)00320-6
Weglarz-Tomczak, E., Tomczak, J. M., Talma, M., Burda-Grabowska, M., Giurg, M., and Brul, S. (2021). Identification of ebselen and its analogues as potent covalent inhibitors of papain-like protease from SARS-CoV-2. Sci. Rep. 11, 3640. doi:10.1038/s41598-021-83229-6
Wenhao, D., Zhang, B., Jiang, X-M., Su, H., Li, J., Zhao, Y., et al. (2020). Structure-based design of antiviral drug candidates targeting the SARS-CoV-2 main protease. Science 368, 1331–1335. doi:10.1126/science.abb4489
Wilcox, R. R. (2012). “Chapter 5 - comparing two groups,” in Statistical modeling and decision science. Introduction to robust estimation and hypothesis testing. Third Edition (Academic Press), 137–213. doi:10.1016/B978-0-12-386983-8.00005-6
Wishart, D. S., Feunang, Y. D., Guo, A. C., Lo, E. J., Marcu, A., Grant, J. R., et al. (2018). DrugBank 5.0: A major update to the DrugBank database for 2018. Nucleic Acids Res. 46, D1074–D1082. doi:10.1093/nar/gkx1037
Wojtovich, A. P., and Brookes, P. S. (2009). The complex II inhibitor atpenin A5 protects against cardiac ischemia-reperfusion injury via activation of mitochondrial KATP channels. Basic Res. Cardiol. 104, 121–129. doi:10.1007/s00395-009-0001-y
Wu, C. G., Cheng, S. C., Chen, S. C., Li, J. Y., Fang, Y. H., Chen, Y. H., et al. (2013). Mechanism for controlling the monomer-dimer conversion of SARS coronavirus main protease. Acta Crystallogr. D. Biol. Crystallogr. 69, 747–755. doi:10.1107/S0907444913001315
Yasri, A., and Hartsough, D. (2001). Toward an optimal procedure for variable selection and QSAR model building. J. Chem. Inf. Comput. Sci. 41, 1218–1227. doi:10.1021/ci010291a
Yu, G., Zhang, G., Domeniconi, C., Yu, Z., and You, J. (2012). Semi-supervised classification based on random subspace dimensionality reduction. Pattern Recogn. 45, 1119–1135. doi:10.1016/j.patcog.2011.08.024
Zhang, L., Lin, D., Sun, X., Curth, U., Drosten, C., Sauerhering, L., et al. (2020). Crystal structure of SARS-CoV-2 main protease provides a basis for design of improved α-ketoamide inhibitors. Science 368, 409–412. doi:10.1126/science.abb3405
Keywords: atpenin, tinostamustine, in silico screening, cysteine proteases, COVID-19, drug repositioning, SARS-CoV-2
Citation: Prada Gori DN, Ruatta S, Fló M, Alberca LN, Bellera CL, Park S, Heo J, Lee H, Park K-HP, Pritsch O, Shum D, Comini MA and Talevi A (2023) Drug repurposing screening validated by experimental assays identifies two clinical drugs targeting SARS-CoV-2 main protease. Front. Drug. Discov. 2:1082065. doi: 10.3389/fddsv.2022.1082065
Received: 27 October 2022; Accepted: 14 December 2022;
Published: 05 January 2023.
Edited by:
Weiwei Xue, Chongqing University, ChinaReviewed by:
Luca Pinzi, University of Modena and Reggio Emilia, ItalyFloriano Paes Silva-Jr, Oswaldo Cruz Foundation (Fiocruz), Brazil
Copyright © 2023 Prada Gori, Ruatta, Fló, Alberca, Bellera, Park, Heo, Lee, Park, Pritsch, Shum, Comini and Talevi. This is an open-access article distributed under the terms of the Creative Commons Attribution License (CC BY). The use, distribution or reproduction in other forums is permitted, provided the original author(s) and the copyright owner(s) are credited and that the original publication in this journal is cited, in accordance with accepted academic practice. No use, distribution or reproduction is permitted which does not comply with these terms.
*Correspondence: Marcelo A. Comini, mcomini@pasteur.edu.uy; Alan Talevi, alantalevi@gmail.com
†These authors have contributed equally to this work and share first authorship
‡These authors have contributed equally to this work and share senior authorship