Modeling Potential Impacts of Climate Change on the Distribution of Wooly Wolf (Canis lupus chanco)
- 1Wildlife Institute of India, Dehradun, India
- 2Department of Biogeography and Global Change, National Museum of Natural Sciences, Madrid, Spain
- 3Department of Ecology, French Institute of Pondicherry–IFP, Pondicherry, India
- 4Department of Ecological Modelling, Helmholtz Centre for Environmental Research, Leipzig, Germany
The Central Asian wolves form a cohort within the wolf-dog clade known as the wooly wolf (Canis lupus chanco). These wolves are poorly studied and their current extent and distribution remain unknown. Apex predators already existing at higher elevations like wooly wolves can be severely affected by climate change because of the absence of suitable refuge. Concomitantly, in the era of Anthropocene, the change in land use land cover (LULC) is rapidly increasing. Even the most adaptable species occurring in human-dominated landscapes may fail to survive under the combined impact of both climate change and human pressure. We collected 3,776 presence locations of the wooly wolf across its range from published literature and compiled 39 predictor variables for species distribution modeling, which included anthropogenic factors, climatic, vegetation, and topographic features. We predicted the change in their distribution under different anthropogenic factors, climate change, and land-use land-cover change scenarios. Wolf showed affinity toward areas with low to moderately warm temperatures and higher precipitations. It showed negative relationships with forests and farmlands. Our future projections showed an expansion of wolf distribution and habitat suitability under the combined effects of future climate and LULC change. Myanmar and Russia had the introduction of high and medium suitability areas for the wooly wolf in future scenarios. Uzbekistan and Kazakhstan showed the consistent loss in high suitability areas while Mongolia and Bhutan had the largest gain in high suitability areas. The study holds great significance for the protection and management of this species and also provides opportunities to explore the impact on associated species.
Introduction
Climate change triggers stark effects on species geographic ranges, leading to range shifts and disruptions in the functioning of ecosystems (Colwell et al., 2008; Lenoir and Svenning, 2015; Lenoir et al., 2017; Pecl et al., 2017). Climate change also causes regional weather fluctuations with respect to precipitation, affecting resource distribution and availability and consequently impacting habitat and ecological processes of faunal species (Parmesan, 2006; Chen et al., 2011; Schewe and Levermann, 2012; Jayasankar et al., 2015). Although species are known to adapt both genetically and behaviorally to circumstances, their adaptive responses are often insufficient due to rapid changes in anthropogenic land-use patterns and their synergistic effects with climate change (Brodie, 2016) thus, threatening them to the verge of extinction (Jump and Penuelas, 2005; Bradshaw and Holzapfel, 2006; Radchuk et al., 2019).
The extinction of large carnivores may have cascading effects on the functioning of an ecosystem in multiple ways (Terborgh et al., 2010). However, when conserved, large carnivores can recover and colonize even in human-dominated landscapes (Chapron et al., 2014). Anthropogenic activities leading to change in land use may also promote large carnivore populations in the future (Milanesi et al., 2017). Studies from the Scandinavian countries and the United States reported that wolves have not only recovered but have also started exploring new habitats in human-dominated landscapes (Mladenoff et al., 1995; Wabakken et al., 2001; Gurarie et al., 2011; Carricondo-Sanchez et al., 2020).
Wolves are known as charismatic as well as umbrella species that have been extensively studied in America and Europe (Mech and Boitani, 2003) with only a few studies from Asia (Khan et al., 2022). The Holarctic gray wolf is also known as Himalayan or Tibetan wolf in the central and south-east Asian countries continues to be an enigma concerning its nomenclature due to the novel genetic insights from across its range (Sharma et al., 2004; Aggarwal et al., 2007; Shrotriya et al., 2012; Fan et al., 2016). Since different terms were used for these wolves according to the different regions where they were found, the term wooly wolf (Arnold, 2016; Joshi et al., 2020; Lyngdoh et al., 2020) was first used by Pocock (1941) to address these wolves. They are known for their hypoxic adaptations for surviving at extremely high altitudes of the Tibetan Plateau, Himalaya, Mongolia, China, and Manchuria (Zhang et al., 2014; Werhahn et al., 2018). Recent genomic studies have suggested that the south Asian region is an important center for the evolution of the gray wolf and the Tibetan wolf is an ecologically significant unit (ESU) (Hennelly et al., 2021).
Existing literature on the wooly wolf focuses majorly on its genetics (Aggarwal et al., 2007; Werhahn et al., 2017), diet (Chetri et al., 2017; Werhahn et al., 2019; Lyngdoh et al., 2020; Reshamwala et al., 2021), public perception (Bhatia et al., 2017; Kusi et al., 2020) and conflict with humans, habitat suitability, and distribution in localized regions (Kabir et al., 2017; Subba et al., 2017; Rana et al., 2018). However, its global geographical extent and potential habitat remain unknown. In this study, we explore two aspects of the wooly wolf distribution. First, we evaluate the current available wooly wolf habitat across its entire distribution. Further, we predict the changes in their suitable habitat under future climatic and land-use change scenarios. A protected area (PA) network plays an important role in the conservation of species regionally. Therefore, we also evaluated the extent and importance of the current PA network for wolf conservation in the range countries. To the best of our knowledge, this is the first study that shows the global extent of wooly wolves and helps in finding key priority areas that may be of management importance in the face of climate change and other anthropogenic factors. Thus, this study holds great conservation and management significance for this species.
Materials and Methods
Study Area
We considered all wolf-ranging regions from Central Asia, Mongolia, Chinese Turkestan, and Tian Shan mountains to the high-altitude plateaus of Tibet, Qinghai, Shensi, Szechwan, Yunnan, and the Himalaya in this study. The topographical features of these regions mainly consist of deserts, grasslands, glaciers, mountains, and river basins. We delineated the study area boundary by creating a polygon surrounding the extreme extents of the above-mentioned regions in Google Earth (Figure 1). Parts of the following countries were included in the intensive study area—Afghanistan, Pakistan, Kazakhstan, Kyrgyzstan, Tajikistan, Uzbekistan, India, Nepal, Bhutan, Myanmar, China, Mongolia, and Russia. The study area comprised about 18.63 million km2. The broad habitat types of the study area include alpine tundra, alpine steppe, wetlands, open grasslands, sparse shrubs, and coniferous forests (Werhahn et al., 2020). In addition to wooly wolves, other predator species in the study area include snow leopard (Panthera uncia), red fox (Vulpes vulpes), Tibetan fox (Vulpes ferrilata), Pallas’s cat (Otocolobus manul), Eurasian lynx (Lynx lynx), and brown bear (Ursus arctos). The major prey species of the study area are urial (Ovis vignei), ibex (Capra ibex), kiang (Equus kiang), blue sheep (Pseudois nayaur), Tibetan gazelle, Tibetan argali (Ovis ammon hodgsoni), white-lipped deer (Cervus albirostris), Himalayan marmot, wooly hare, several species of pika (Ochotona spp.), and rodents. Livestock also adds a considerable amount to the diet of predators in the study area (Lyngdoh et al., 2020; Khan et al., 2022).
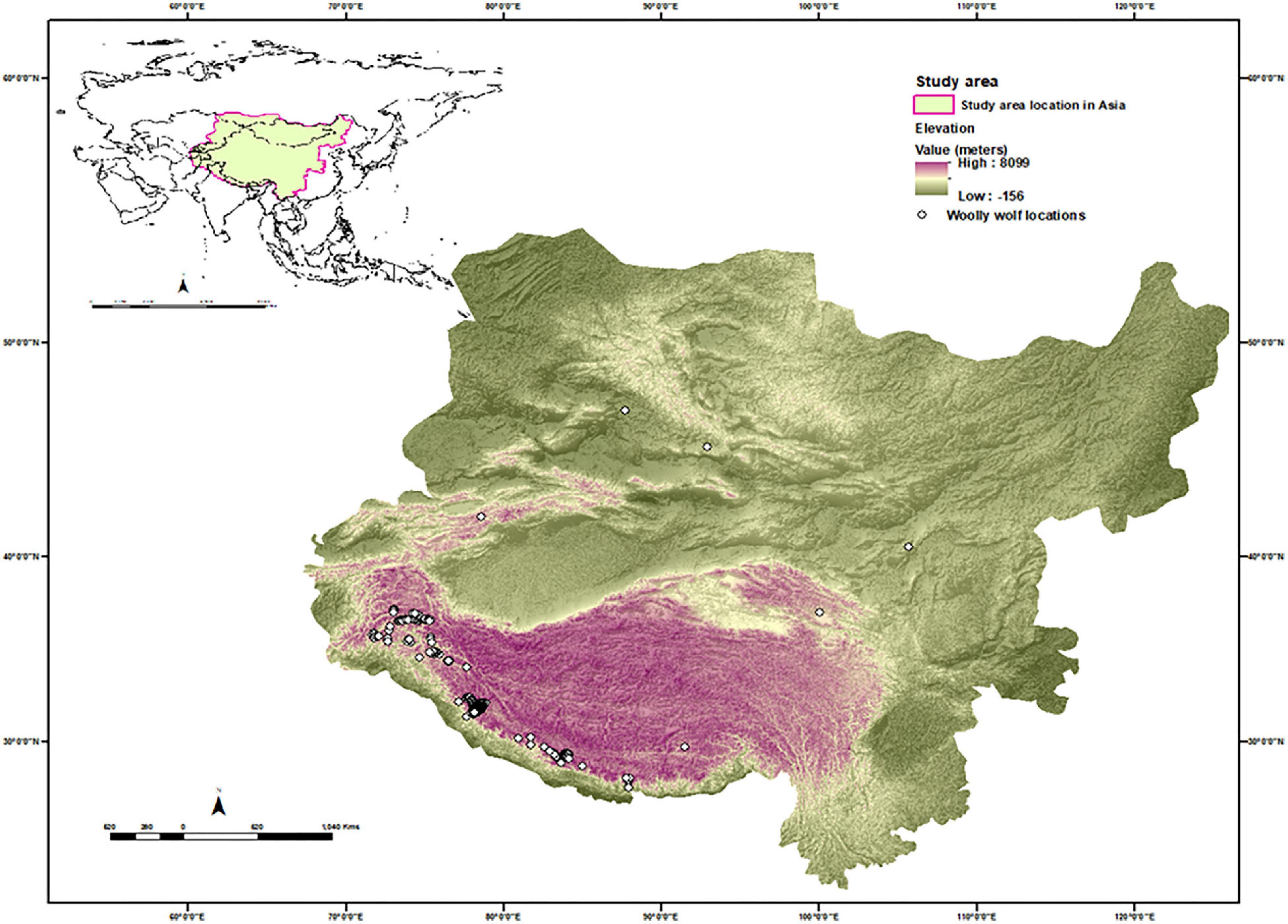
Figure 1. Map of the study area along with collated presence locations used for distribution modeling of the wooly wolf.
Wooly Wolf Location Data
Presence locations of wooly wolves (both direct and indirect evidence) were obtained from published literature and publicly available dissertations. We collected 3,776 presence locations of woolly wolves (Figure 1 and Supplementary Table 1) for Afghanistan, Pakistan, Kyrgyzstan, India, Nepal, Bhutan, China, and Mongolia which included data from our telemetry study and sightings on wooly wolves.
Preparation of Geospatial Layers
We gathered a total of 39 predictor variables from various sources to develop species distribution model for wooly wolves for current and future prediction (Supplementary Table 2). We selected topographic, vegetation, and anthropogenic variables that may govern the distribution of the wooly wolf. These factors included climatic variables, aridity Index (AI), potential evapotranspiration (PET), cloud cover, normalized difference vegetation index (NDVI), digital elevation model (DEM), land use land cover (LULC), slope, aspect, topographic position index, terrain ruggedness, vector ruggedness, hill shade, distance to the nearest water source, distance to nearest glaciers, distance to nearest roads, human footprint, and population density. We did a Pearson’s correlation test between the 39 variables prior to species distribution modeling and eliminated highly correlated variables (>0.6). Thus, we finalized 18 variables for final analyses in the current and future scenarios (Supplementary Table 2). We then extracted the values of all the variables according to the intensive study area boundary. We overlaid boundaries of PAs pertaining to the intensive study area which was available from https://www.protectedplanet.net/en/thematic-areas/wdpa to understand the suitable habitat of the wooly wolf inside and outside PAs. Though this is the only database available of comprehensive information for PAs across the globe, we want to point out that the above data have certain discrepancies regarding the coverage of all PAs of the countries included in the study. We acquired an updated PA layer for India from https://indiabiodiversity.org/map and merged it with the existing information. However, for other countries, such updated information was not available.
Analysis
Modeling Current Distribution
We used MaxEnt (version 3.4.1k) for developing species distribution models for the wooly wolf. MaxEnt is based on occurrence/presence records (locations where the species has been found) together with environmental variables or constraints for the surrounding study area (Phillips et al., 2006; Phillips and Dudík, 2008) and is one of the recent approaches which can be used (Hernandez et al., 2006; Wisz et al., 2008). We developed six MaxEnt models using the default settings, except for the feature classes, to check for initial model fitting using all locations for different grid divisions within the study area. We used linear, quadratic, and hinge feature classes, best suited for small sample sizes (Morales et al., 2017) for our models to help smooth the variable responses and reduce the noise (Elith et al., 2011; Merow et al., 2013; Morales et al., 2017). Hinge features provide at least as much flexibility in the fitted response to predictor variables as threshold features, while tending to reduce overfitting to the training data. To account for sampling bias, we applied the bias correction method by creating bias grid files that can be fed into the MaxEnt software (Dudík et al., 2005; Phillips et al., 2009). In a biased file, the cell values reflect the sampling effort and give a weight to random background data used for modeling (Fourcade et al., 2014). We produced these sampling probability surfaces by deriving the Gaussian Kernel Density of sampling localities (Elith et al., 2010). Previous studies have shown that correcting sampling bias has yielded improved model fitting especially with smaller sample sizes (Fourcade et al., 2014). The spatial distance used to quantify the region of spatial bias was kept at 50 and 100 km, respectively.
To avoid clustering of presence locations, we divided the entire study area into grids of 1 × 1 km, 2 × 2 km, 3 × 3 km, 5 × 5 km, 7 × 7 km, and 10 × 10 km and selected one random point location falling in each grid using R software (R Core Team). For each grid size, separate analyses were performed. Such divisions were selected to incorporate all possible scales and values of variable contributions for analysis. Accordingly, we finalized 774, 486, 357, 254, 206, and 162 presence locations, respectively, for further analysis. We conducted the analyses with all the divisions using both 50 and 100 km bias files (12 models). Concurrence between model accuracy and output decreased with an increased grid size (Seo et al., 2009). The models with increased grid sizes selected more area as potential distribution range. We assume that the small grid size combination incorporated all possible fine-scale values of variables used and provide a significant spatial distribution output. Otherwise, an overestimated predicted range might lead to inappropriate selection of conservation priority areas (Seo et al., 2009). Moreover, the analysis was conducted with a small sample size over a large study area, it was important to include all possible fine-scale information available for a robust output. Hence, we used results for only 1 × 1 km grid size with 100 km bias.
We then developed five different models with combinations of regularization multipliers (0.25, 0.5, 1, 1.5, and 2) and selected the most significant combination based on the area under the receiver operating characteristic curve (AUC) value. Regularization multiplier is a modifiable parameter that adds new constraints to the model and is thus used to evaluate the best potential combination of parameters teaming up with the feature classes (Morales et al., 2017). This is used to prevent the over-fitting of the model by controlling the intensity of the chosen feature classes (Elith et al., 2010). Previous studies have shown that for small sample sizes it is best to use intermediate regularization multipliers for better model fitting (Radosavljevic and Anderson, 2014; Morales et al., 2017). We assessed the model performance by the mean AUC value (Hosmer et al., 2000) and visual inspection of the identified suitable area of the output maps for each model. We finalized regularization multiplier 2 to be the most significant for further analysis as the AUC graph and the output map coincided in showing meaningful representations in concurrence to the species’ ecology (Radosavljevic and Anderson, 2014). We then partitioned our presence locations randomly into 5 sets of training and testing samples. All the training sets included rare locations from the far-off regions such as Mongolia. We opted for this approach to train the models with a wider set of information. We developed models with the above sets of training and testing samples (5 models) and the model performance was assessed by the AUC values. We calculated mean variable response curves and Jackknife of regularized training gain test to evaluate the importance of each predictor and percent contribution and permutation importance (Supplementary Figure 1). We applied a 10-percentile training presence logistic threshold to generate the species distribution maps. We calculated the average of the 5 distribution models to predict the current distribution of wooly wolves. The final layer was classified into equal intervals (0–33%, 33–66%, 66–100% suitability) of three suitability areas—low, medium, and high and tabulated the area for each class.
Projecting Future Distributions
We used two future scenario settings to assess the likely changes in suitable habitats for wooly wolves for climatic variables and LULC for RCP4.5 and RCP8.5. We used the A1B scenario of the future LULC layer as this scenario had data balanced across all resources (Li et al., 2017). We used the same environmental layers for both the current and future distribution models. We used the MICROC 5 model of the Global Climatic Model (GCM) for the future climate scenarios as the warmest future change is obtained through this model (Wazneh et al., 2020). We used 29 models of various combinations to generate the future projections (Supplementary Table 3). Similar to the analysis for current distribution (see section “Modeling Current Distribution”), we generated the average model for each scenario, classified them into suitability areas, and tabulated the areas for each class. We projected the future distributions for two timelines—2050 and 2070. We developed a total of 63 MaxEnt models (Supplementary Table 3) using 774 locations and 18 environmental variables to predict the potentially suitable habitat for wooly wolves.
Results
The MaxEnt model used for wooly wolf distribution was reliable as statistical estimation of averaged accuracy for five models was 94.8% ± 0.54 SD and 94.5% ± 0.50 SD for training and test AUC, respectively. Regularization multiplier 2 was the most suitable for the wooly wolf to avoid overfitting of the models. Of the total study area, 5.31% was inside PA and 94.69% was outside PA.
Current Distribution
Among the variables used to predict the current distribution – DEM, vector ruggedness measure (VRM), mean diurnal temperature (Bio2), precipitation of coldest quarter (Bio19), precipitation of driest month (Bio14), and LULC were the most important factors driving the habitat suitability in the study area (Figure 2). Wolf showed a positive relation toward higher elevation, but the peak dropped after 5,000 m which is consistent with wolf ecology. However, the distribution did not show any variation with ruggedness indicating wolves’ preferences toward all kinds of terrain. Wolf showed affinity toward areas with lower to moderately warm temperatures and higher precipitations. It showed negative relations with forests and farmlands, reflecting its affinity more toward open barren areas. Only ∼12% of the study area corresponded to suitable area (Medium + High) for wooly wolf (Figure 3) of which only ∼1% is under PA and the rest outside PA. Among the countries, the most suitable habitat for wooly wolves was in China followed by Kyrgyzstan, India, Tajikistan, Pakistan, and Afghanistan.
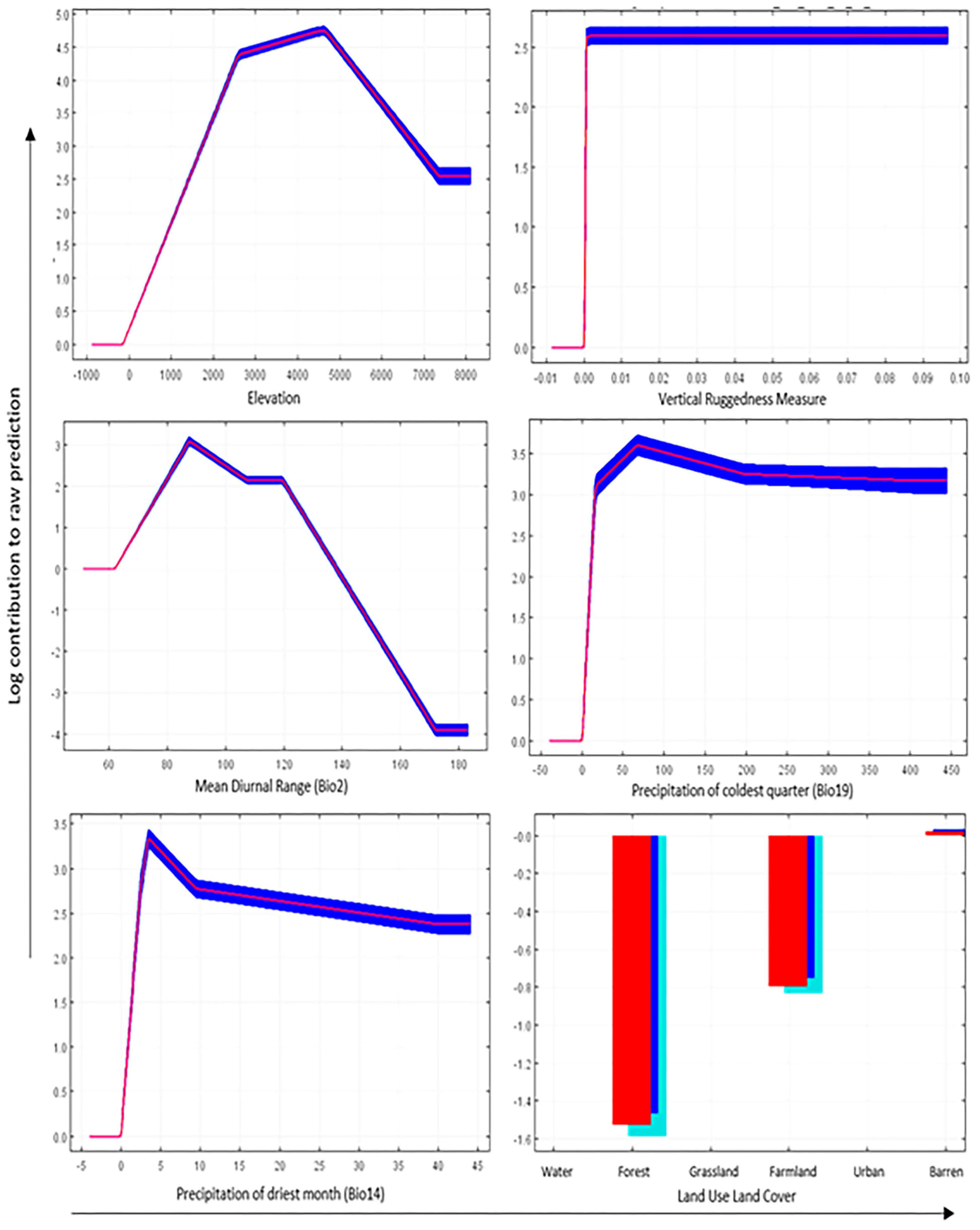
Figure 2. MaxEnt model response area curves of the predictor variables influencing wooly wolf distribution: elevation; vertical ruggedness; mean diurnal range (Bio2); precipitation of coldest quarter (Bio19); precipitation of driest month (Bio 14) and land use land cover.
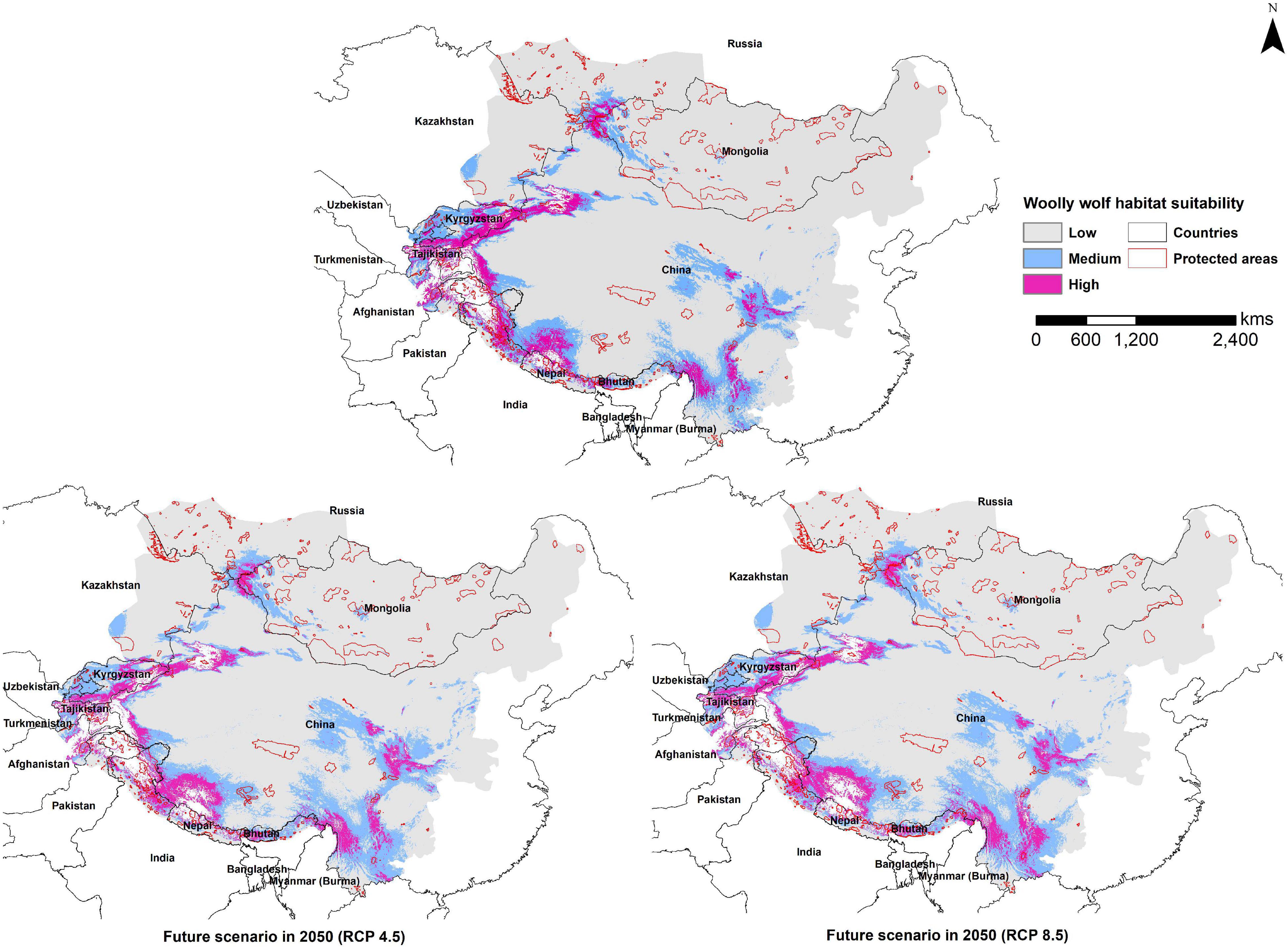
Figure 3. Wooly wolf distribution model showing current and future trends of suitable habitat for the species for two future climate scenarios (RCP 4.5 and 8.5) for the year 2050 with the countries and protected areas boundary within the intensive study area.
Future Projections
The future climate scenarios RCP 4.5 and 8.5 both predict an increase in global temperature for the years 2050 and 2070. Our future projections showed an expansion of wolf distribution and habitat suitability under the combined effects of future climate and LULC (Figure 3). The results for both the scenarios for both timelines showed expansion of range in medium and high suitability classes (Table 1). Most expansions occurred in the southern and south western parts of the study area. This indicates that wooly wolf distribution was mainly affected by the change in future climatic conditions which in turn governed the changes in future land-use scenario. It was interesting to find that Myanmar and Russia had the introduction of high and medium suitable areas for wooly wolves in the future scenarios (Figure 4). Uzbekistan and Kazakhstan showed consistent loss in high suitable area while Mongolia and Bhutan had the highest gain in suitable area (Figure 4). Kyrgyzstan and Tajikistan had increase of suitable areas inside PA and decrease outside PA (Supplementary Table 4).
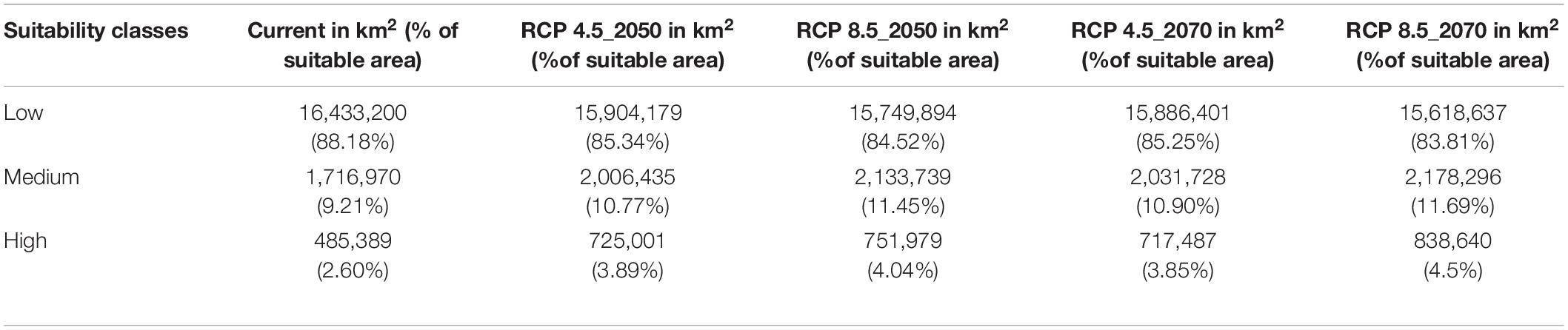
Table 1. Results showing patterns of current and future suitability areas for wooly wolves under different suitability classes.
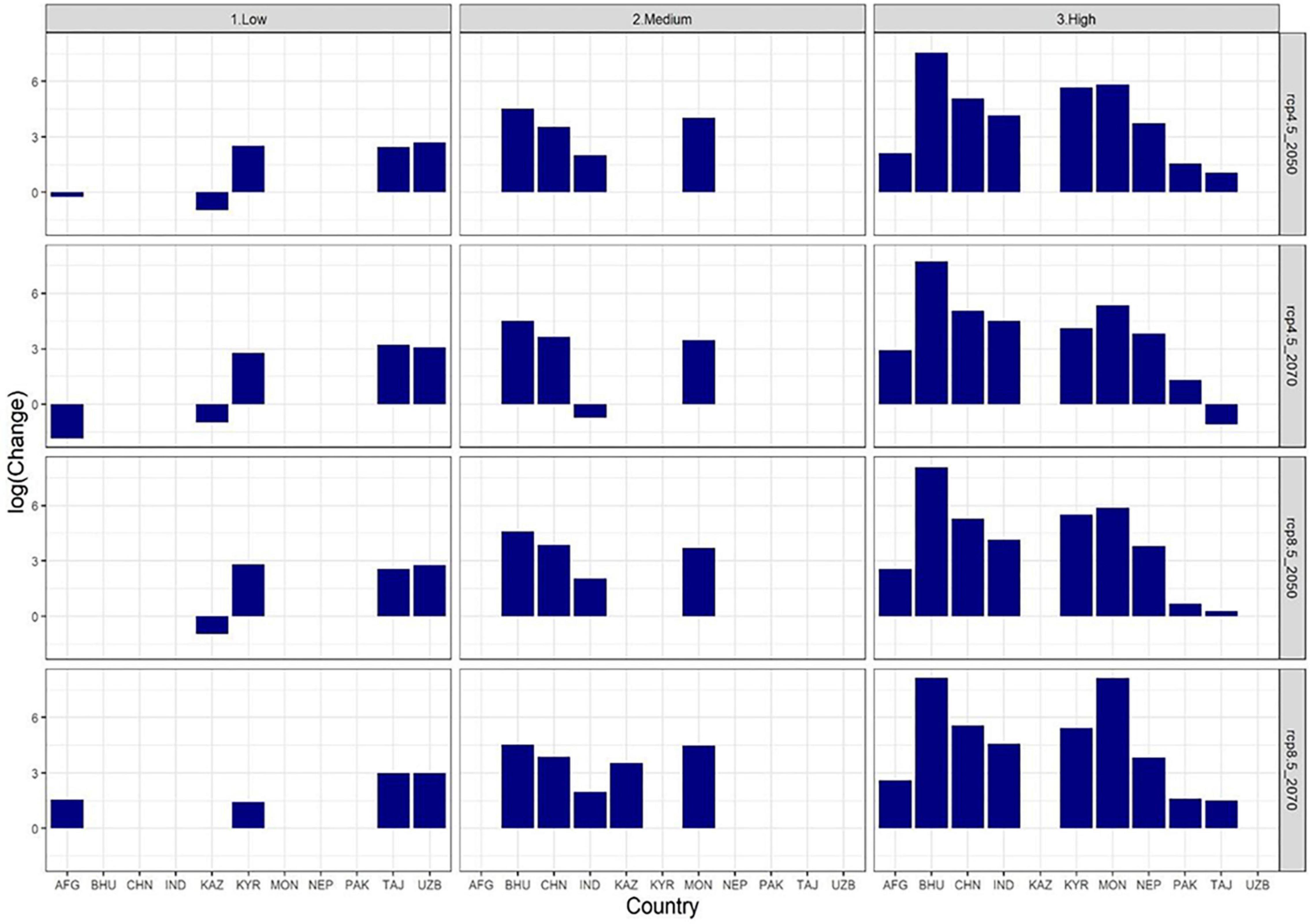
Figure 4. Country-wise gain/loss of wooly wolf suitable habitat in four future scenarios with respect to current suitable habitat in three suitability classes.
For the current scenario, we calculated the low, medium, and high habitat suitability of wooly wolves. The result showed that the maximum areas of PA in Nepal, India, China, and Mongolia were highly suitable for wooly wolves. Moreover, the highly suitable areas for wooly wolves were found more outside PAs in Uzbekistan, Afghanistan, Pakistan, Kazakhstan, Tajikistan, and Bhutan (Table 2).
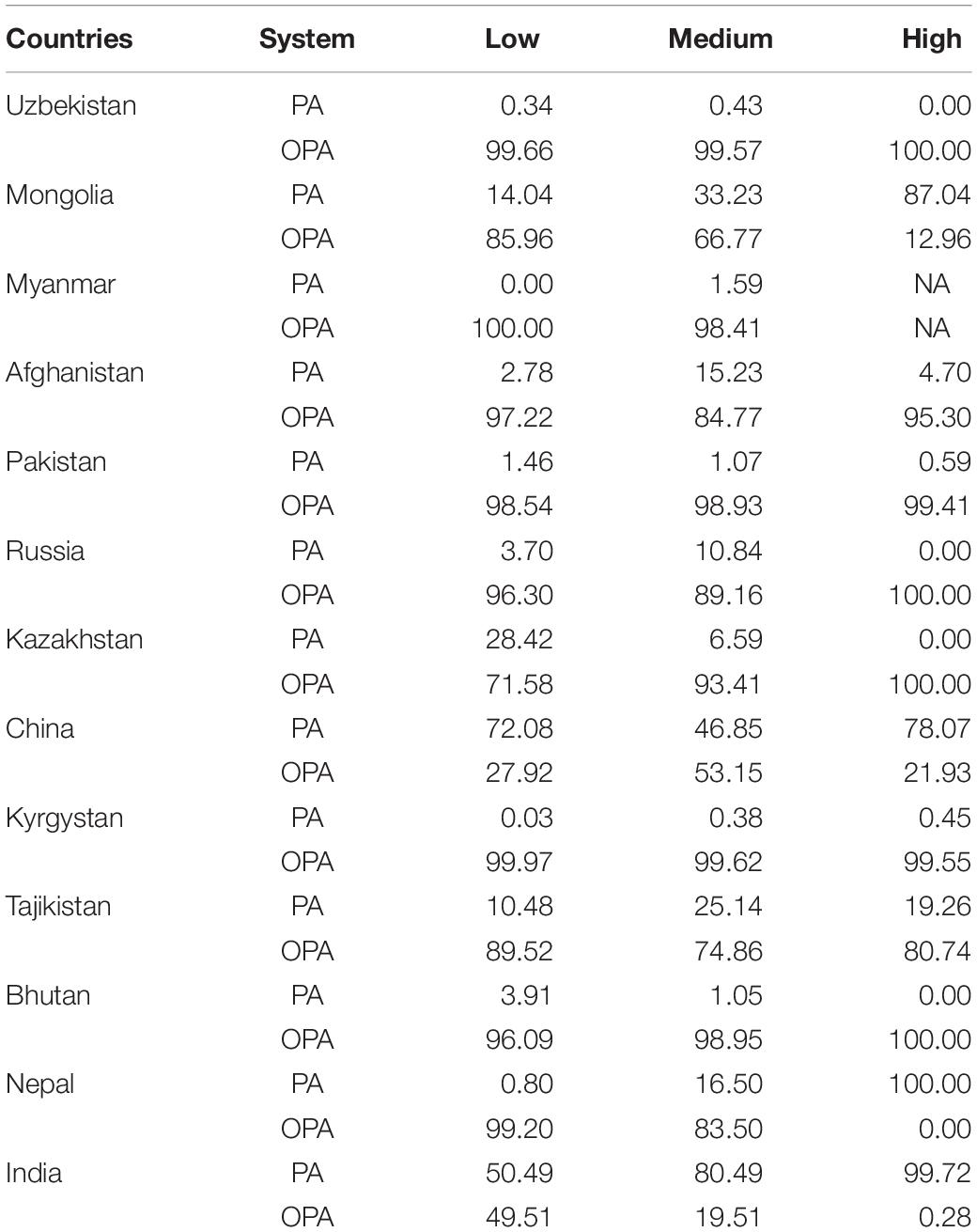
Table 2. Country-wise suitable habitat of wooly wolf suitable within (PA) and outside protected areas (OPA) in current scenarios.
Discussion
This study provided a probabilistic current distribution and predicted the likely changes in the suitable habitats for wooly wolves across 15 countries in Central Asia under climate change. The study shows an increase in habitat suitability of wooly wolves within its probable distribution range under the combined effects of climate and land-use changes in the future. The primary reason behind this could be the increase in the availability of barren areas and agricultural lands due to the melting of glaciers under rising temperatures. Both RCP 4.5 and RCP 8.5 emissions scenarios predict a rise in temperature in the years 2050 and 2070. The future land-use change for the year 2050 predicts increase in agricultural land use, high pressure on forest resources, and expansion of plantation forestry (Sleeter et al., 2012). Studies have shown that climate change due to rising temperature and expansion of agriculture along altitudinal gradient synergistically affect the niches of mammals (Brodie, 2016). Wolves could be affected by these factors and lead to changes in the distribution and behavioral patterns of the species. Our distribution model shows a strong negative relationship with forests than with agriculture and a positive relation with barren areas (Figure 2). This was further supported by the distribution of wooly wolf suitable habitats outside PAs in future scenarios (Table 1) and the introduction of suitable habitats in Myanmar and Russia. Our results show wooly wolves prefer moderately warmer and contiguous wet areas. It is known that warmer and wetter conditions may favor certain species expansion (Hof et al., 2012). We believe that such conditions could lead to land-use changes, especially the expansion of agriculture. It has been found that land-use changes (agriculture, plantations, etc.) can increase the structural, functional, and temporal connectivity to generalist species by providing refugia during less favorable climatic conditions facilitating range expansions (Auffret et al., 2015; Elmhagen et al., 2015).
We found that protected areas hardly provide suitable areas for wooly wolves throughout their distribution range. The future scenario is also similar except that the suitable area increased more inside the PAs in Kyrgyzstan and Tajikistan. Although our calculations of the PA network could be an underestimation due to the unavailability of recently updated datasets for all countries, the overall trend is unlikely to be affected by such errors. Therefore, the wolf conservation should be pivoted to community conservation areas outside the PA network. Large-ranging species require landscape-level planning and management, which are difficult to achieve through the existing PA network system. Our distribution modeling for wooly wolf further has potential drawbacks due to the lack of presence data from North and Eastern parts of its range. Almost no literature is available from the central Asia countries and much of the Chinese part. To avoid overprediction or underestimation of its habitat, we ensured to use all locations from these understudied regions for training in all our model datasets. However, more presence locations from these regions would aid in robust modeling and predictions.
Species that are generalist in nature with the ability to move far can colonize new areas and track their shifting areas according to climatic suitability (Colwell et al., 2008). Arctic and sub-arctic generalist mammal species have been known to expand their ranges due to the impact of climate change (Hof et al., 2012). It has been suggested in previous studies that species can make large elevational shifts over short linear distances in areas with steep topography. This is because contiguous habitats are found along elevational gradients (Colwell et al., 2008; Brodie, 2016). There are evidence of upslope range shifts due to climate change by snow leopards and Ethiopian wolves (Trouwborst and Blackmore, 2020). Our results showed major habitat suitability changes in the south and south-western parts of the study area that had the most variations in altitudes due to the presence of several mountain ranges.
Gray wolves are known to act as buffers in climate change by mediating and facilitating other animal species (Wilmers and Getz, 2005). Range expansion may lead to intra-guild as well as interspecific competition and predation as suggested by Pamperin et al. (2006) in their study on red foxes (Vulpes vulpes), which underwent range expansion in the higher altitudes leading to incidences of killing of arctic foxes (Alopex lagopus). The increase in habitat suitability of wooly wolves in the future can lead to habitat sharing or usurping of species of more or less similar guilds or niches such as snow leopards, brown bears, red foxes, and lynx. Both gray wolves and brown bears are reported to expand their ranges under the influence of climate change (Hof et al., 2012; Falcucci et al., 2013). Such range changes of top predators like wolves may affect prey populations due to limiting effects on other predator species.
Unfavorable weather conditions may affect ranges of certain animals in a positive or negative interaction with wolves affecting their distribution and range expansion. Again, land use changes might indirectly favor predator species such as wolves, and increase predation pressure and conflict (Festa-Bianchet et al., 2011; Elmhagen et al., 2015). Thus, the response of prey in range expansion of wolf would be an important aspect to be focused on in future because if prey does not respond in the same manner, it can lead to enhanced conflict or even mass extinction. Studies have shown that wild ungulates would become more susceptible to disease in the future due to climate change, habitat shrinking, increased concentration and movement of human, livestock within shared habitats of wild animals introducing various pathogens and vectors (Hu and Jiang, 2011). Prey species in Central Asia such as Przewalski’s gazelle (Procapra przewalskii), Mongolian gazelle (Procapra gutturosa), Siberian ibex (Capra sibirica) and goitered gazelle (Gazella subgutturosa), and saiga antelopes (Saiga tatarica) are prone to disease outbreaks due to the increasing temperature as well as shared rangelands with livestock (Pruvot et al., 2020; Khanyari et al., 2021). Due to climate change, such responses of prey species might lead to a decline in their population and the spread of disease amongst predators like wolves.
This study across different countries showed that Bhutan, Mongolia, Kyrgyzstan, Nepal, China, and India had increased suitable habitat, mostly outside PAs. These countries already have existing conflict issues with wolves (Watanabe et al., 2010; Alexander et al., 2015; Karimov et al., 2018; Din et al., 2019). Synergistic positive effects of climate and land-use change can favor invasive species and expansion of temperate species to higher altitudes (Bellard et al., 2013; Elmhagen et al., 2015). Our results suggested that the elevation plays an important role in wooly wolf distribution in current and future scenarios without change in the preferred range of 2,500–5,000 m, which aligns with a similar previous study (Habib et al., 2013). We also predict the range expansion of wolves in Myanmar and Russia where there was low habitat suitability for wolf distribution before. This might lead to the colonization of a new predator species, disrupting the current prey-predator guild and the sudden rise of conflict with humans. Thus, this study shows the importance of predictive modeling, which could help in management planning for such scenarios priorly.
Our results indicate Kazakhstan to have decreased wooly wolf habitat suitability area in the future. Earlier studies have shown that the wolf population in this country has been declining due to a reduction in saiga population and hunting (Leontiev, 2018). Gray wolves, which were once the most widespread land carnivore (Paquet and Carbyn, 2003), have now vanished from 26% of their geographical extent (Wolf and Ripple, 2017). Similarly, the wooly wolf is subjected to human persecution and conflict for centuries, a consequence of thriving in a resource-scarce habitat (Lyngdoh et al., 2020). The main reasons for conflict with humans across their distribution range are due to livestock depredation and retaliation by humans (Mishra, 1997; Namgail et al., 2007; Jamtsho and Katel, 2019). We propose that changes in wolf distribution may aggravate the conflict situation across various wolf-ranging countries in the future, mainly because of their increasing presence in human-dominated landscapes. This study paves the way for future administrative mechanisms for wolf management in these countries.
Conclusion
Studies have shown that climate change has affected mammal species in the northern hemisphere more due to the combined effect of different drivers such as land-use change, anthropogenic food subsidies, and hunting. This study provides baseline information on how the distribution of a generalist top predator species would alter under the combined effects of climate and land-use change. Holistic spatial information about local populations of predators is important because of the knowledge gaps from lesser-known areas. While this study does not support the complete range shift for wooly wolves, we predict an increase in suitability area across their distribution range. This study provides insights regarding the gain or loss of suitable habitat in wolf range countries within and outside PAs. Based on such projections, conscious management decisions need to be taken regarding the conservation of this species in a country-specific manner. Hotspot areas where future conflict with humans could arise due to increased livestock numbers resulting in depredation should be considered priority areas for management. Understanding the spatial distribution of wooly wolves across its range is necessary to plan monitoring and management strategies. Lack of this understanding may also hinder our conservation efforts and mitigation strategies under climate change scenarios. Similar information on future trends of prey species and co-predators should be at hand to have overall information of the landscape ecosystem. Transboundary protocols based on such future trends are the need of the hour for the conservation of the wooly wolf.
Data Availability Statement
The raw data supporting the conclusions of this article will be made available by the authors, without undue reservation.
Ethics Statement
The animal study was reviewed and approved by the Wildlife Institute of India.
Author Contributions
SK, HR, and AB collected the data and wrote the manuscript. SS and SL helped in analysis and discussion. BH conceptualized, supervised, and edited the manuscript. SG and RK helped in discussion, analysis, and editing. All authors contributed to the article and approved the submitted version.
Funding
This research was supported by the Wildlife Institute of India. We are thankful to the Director and Dean, Wildlife Institute of India, for support and encouragement. This work was carried out under the project “Response to Anthropocene and Climate Change: Movement Ecology of Selected Mammal Species in the Indian Himalayan region” funded by the National Mission on Himalayan Studies (NMHS).
Conflict of Interest
The authors declare that the research was conducted in the absence of any commercial or financial relationships that could be construed as a potential conflict of interest.
Publisher’s Note
All claims expressed in this article are solely those of the authors and do not necessarily represent those of their affiliated organizations, or those of the publisher, the editors and the reviewers. Any product that may be evaluated in this article, or claim that may be made by its manufacturer, is not guaranteed or endorsed by the publisher.
Supplementary Material
The Supplementary Material for this article can be found online at: https://www.frontiersin.org/articles/10.3389/fevo.2022.815621/full#supplementary-material
References
Aggarwal, R. K., Kivisild, T., Ramadevi, J., and Singh, L. (2007). Mitochondrial DNA coding region sequences support the phylogenetic distinction of two Indian wolf species. J. Zool. Syst. Evol. Res. 45, 163–172. doi: 10.1111/j.1439-0469.2006.00400.x
Alexander, J., Chen, P., Damerell, P., Youkui, W., Hughes, J., Shi, K., et al. (2015). Human wildlife conflict involving large carnivores in Qilianshan, China and the minimal paw-print of snow leopards. Biol. Conserv. 187, 1–9. doi: 10.1016/j.biocon.2015.04.002
Arnold, C. (2016). Woolly Wolf Spotted in Nepal Is Likely a New Species. New York, NY: National Geographic.
Auffret, A. G., Plue, J., and Cousins, S. A. (2015). The spatial and temporal components of functional connectivity in fragmented landscapes. Ambio 44, 51–59. doi: 10.1007/s13280-014-0588-6
Bellard, C., Thuiller, W., Leroy, B., Genovesi, P., Bakkenes, M., and Courchamp, F. (2013). Will climate change promote future invasions? Glob. Change Biol. 19, 3740–3748. doi: 10.1111/gcb.12344
Bhatia, S., Redpath, S. M., Suryawanshi, K., and Mishra, C. (2017). The relationship between religion and attitudes toward large carnivores in northern India? Hum. Dimens. Wildl. 22, 30–42. doi: 10.1080/10871209.2016.1220034
Bradshaw, W. E., and Holzapfel, C. M. (2006). Evolutionary response to rapid climate change. Science (Washington) 312, 1477–1478. doi: 10.1126/science.1127000
Brodie, J. F. (2016). Synergistic effects of climate change and agricultural land use on mammals. Front. Ecol. Environ. 14:20–26. doi: 10.1002/16-0110.1
Carricondo-Sanchez, D., Zimmermann, B., Wabakken, P., Eriksen, A., Milleret, C., Ordiz, A., et al. (2020). Wolves at the door? Factors influencing the individual behavior of wolves in relation to anthropogenic features. Biol. Conserv. 244:108514. doi: 10.1016/j.biocon.2020.108514
Chapron, G., Kaczensky, P., Linnell, J. D., Von Arx, M., Huber, D., Andrén, H., et al. (2014). Recovery of large carnivores in Europe’s modern human-dominated landscapes. Science 346, 1517–1519. doi: 10.1126/science.1257553
Chen, J., Brissette, F. P., and Leconte, R. (2011). Uncertainty of downscaling method in quantifying the impact of climate change on hydrology. J. Hydrol. 401, 190–202. doi: 10.1016/j.jhydrol.2011.02.020
Chetri, M., Odden, M., and Wegge, P. (2017). Snow leopard and Himalayan wolf: food habits and prey selection in the central Himalayas, Nepal. PLoS One 12:e0170549. doi: 10.1371/journal.pone.0170549
Colwell, R. K., Brehm, G., Cardelús, C. L., Gilman, A. C., and Longino, J. T. (2008). Global warming, elevational range shifts, and lowland biotic attrition in the wet tropics. Science 322, 258–261. doi: 10.1126/science.1162547
Din, J. U., Nawaz, M. A., Mehmood, T., Ali, H., Ali, A., Adli, D. S. H., et al. (2019). A transboundary study of spatiotemporal patterns of livestock predation and prey preferences by snow leopard and wolf in the Pamir. Glob. Ecol. Conserv. 20:e00719. doi: 10.1016/j.gecco.2019.e00719
Dudík, M., Phillips, S., and Schapire, R. E. (2005). Correcting sample selection bias in maximum entropy density estimation. Adv. Neural Inf. Process. Syst. 18, 323–330.
Elith, J., Kearney, M., and Phillips, S. (2010). The art of modelling range-shifting species. Methods Ecol. Evol. 1, 330–342. doi: 10.1111/j.2041-210X.2010.00036.x
Elith, J., Phillips, S. J., Hastie, T., Dudík, M., Chee, Y. E., and Yates, C. J. (2011). A statistical explanation of MaxEnt for ecologists. Divers. Distrib. 17, 43–57. doi: 10.1111/j.1472-4642.2010.00725.x
Elmhagen, B., Kindberg, J., Hellström, P., and Angerbjörn, A. (2015). A boreal invasion in response to climate change? Range shifts and community effects in the borderland between forest and tundra. Ambio 44, 39–50. doi: 10.1007/s13280-014-0606-8
Falcucci, A., Maiorano, L., Tempio, G., Boitani, L., and Ciucci, P. (2013). Modeling the potential distribution for a range-expanding species: wolf recolonization of the Alpine range. Biol. Conserv. 158, 63–72. doi: 10.1016/j.biocon.2012.08.029
Fan, Z., Silva, P., Gronau, I., Wang, S., Armero, A. S., Schweizer, R. M., et al. (2016). Worldwide patterns of genomic variation and admixture in gray wolves. Genome Res. 26, 163–173. doi: 10.1101/gr.197517.115
Festa-Bianchet, M., Ray, J. C., Boutin, S., Côté, S. D., and Gunn, A. (2011). Conservation of caribou (Rangifer tarandus) in Canada: an uncertain future. Can. J. Zool. 89, 419–434. doi: 10.1139/z11-025
Fourcade, Y., Engler, J. O., Rödder, D., and Secondi, J. (2014). Mapping species distributions with MAXENT using a geographically biased sample of presence data: a performance assessment of methods for correcting sampling bias. PLoS One 9:e97122. doi: 10.1371/journal.pone.0097122
Gurarie, E., Suutarinen, J., Kojola, I., and Ovaskainen, O. (2011). Summer movements, predation and habitat use of wolves in human modified boreal forests. Oecologia 165, 891–903. doi: 10.1007/s00442-010-1883-y
Habib, B., Shrotriya, S., and Jhala, Y. V. (2013). Ecology and Conservation of Himalayan Wolf. Technical Report No TR – 2013/01. Dehradun: Wildlife Institute of India. doi: 10.13140/RG.2.2.36012.87685
Hennelly, L. M., Habib, B., Modi, S., Rueness, E. K., Gaubert, P., and Sacks, B. N. (2021). Ancient divergence of Indian and Tibetan wolves revealed by recombination-aware phylogenomics. Mol. Ecol. 30, 6687–6700. doi: 10.1111/mec.16127
Hernandez, P. A., Graham, C. H., Master, L. L., and Albert, D. L. (2006). The effect of sample size and species characteristics on performance of different species distribution modeling methods. Ecography 29, 773–785. doi: 10.1093/bioinformatics/btaa255
Hof, A. R., Jansson, R., and Nilsson, C. (2012). Future climate change will favour non-specialist mammals in the (sub) arctics. PLoS One 7:e52574. doi: 10.1371/journal.pone.0052574
Hosmer, D. W., Lemeshow, S., and Sturdivant, R. X. (2000). Applied Logistic Regression. New York, NY: Wiley.
Hu, J., and Jiang, Z. (2011). Climate change hastens the conservation urgency of an endangered ungulate. PLoS One 6:e22873. doi: 10.1371/journal.pone.0022873
Jamtsho, Y., and Katel, O. (2019). Livestock depredation by snow leopard and Tibetan wolf: implications for herders’ livelihoods in Wangchuck Centennial National Park, Bhutan. Pastoralism 9, 1–10. doi: 10.1186/s13570-018-0136-2
Jayasankar, C. B., Surendran, S., and Rajendran, K. (2015). Robust signals of future projections of Indian summer monsoon rainfall by IPCC AR5 climate models: role of seasonal cycle and interannual variability. Geophys. Res. Lett. 42, 3513–3520. doi: 10.1002/2015GL063659
Joshi, B. D., Lyngdoh, S., Singh, S. K., Sharma, R., Kumar, V., Prakash, T. V., et al. (2020). Revisiting the woolly wolf (Canis lupus chanco) phylogeny in Himalaya: addressing taxonomy, spatial extent and distribution of an ancient lineage in Asia. PLoS One 15:e0231621. doi: 10.1371/journal.pone.0231621
Jump, A. S., and Penuelas, J. (2005). Running to stand still: adaptation and the response of plants to rapid climate change. Ecol. Lett. 8, 1010–1020. doi: 10.1111/j.1461-0248.2005.00796.x
Kabir, M., Hameed, S., Ali, H., Bosso, L., Din, J. U., Bischof, R., et al. (2017). Habitat suitability and movement corridors of grey wolf (Canis lupus) in Northern Pakistan. PLoS One 12:e0187027. doi: 10.1371/journal.pone.0187027
Karimov, K., Kachel, S. M., and Hackländer, K. (2018). Responses of snow leopards, wolves and wild ungulates to livestock grazing in the zorkul strictly protected area, Tajikistan. PLoS One 13:e0208329. doi: 10.1371/journal.pone.0208329
Khan, S., Shrotriya, S., Sadhukhan, S., Lyngdoh, S., Goyal, S. P., and Habib, B. (2022). Comparative ecological perspectives of two ancient lineages of grey wolves: woolly wolf (Canis lupus chanco) and Indian wolf (Canis lupus pallipes). Front. Ecol. Evol. 10:775612. doi: 10.3389/fevo.2022.775612
Khanyari, M., Robinson, S., Morgan, E. R., Brown, T., Singh, N. J., Salemgareyev, A., et al. (2021). Building an ecologically-founded disease risk prioritisation framework for migratory wildlife species based on contact with livestock. J. Appl. Ecol. 58, 1–16. doi: 10.1111/1365-2664.13937
Kusi, N., Sillero-Zubiri, C., Macdonald, D. W., Johnson, P. J., and Werhahn, G. (2020). Perspectives of traditional Himalayan communities on fostering coexistence with Himalayan wolf and snow leopard. Conserv. Sci. Pract. 2:e165.
Lenoir, J., Hattab, T., and Pierre, G. (2017). Climatic microrefugia under anthropogenic climate change: implications for species redistribution. Ecography 40, 253–266. doi: 10.1111/ecog.02788
Lenoir, J., and Svenning, J. C. (2015). Climate-related range shifts–a global multidimensional synthesis and new research directions. Ecography 38, 15–28. doi: 10.1111/ecog.00967
Li, X., Chen, G., Liu, X., Liang, X., Wang, S., Chen, Y., et al. (2017). A new global land-use and land-cover change product at a 1-km resolution for 2010 to 2100 based on human–environment interactions. Ann. Am. Assoc. Geogr. 107, 1040–1059. doi: 10.1080/24694452.2017.1303357
Lyngdoh, S. B., Habib, B., and Shrotriya, S. (2020). Dietary spectrum in Himalayan wolves: comparative analysis of prey choice in conspecifics across high-elevation rangelands of Asia. J. Zool. 310, 24–33. doi: 10.1111/jzo.12724
Mech, L. D., and Boitani, L. (2003). Wolves: Behaviour, Ecology and Conservation. Chicago, IL: University of Chicago Press.
Merow, C., Smith, M. J., and Silander, J. A. Jr. (2013). A practical guide to MaxEnt for modeling species’ distributions: what it does, and why inputs and settings matter. Ecography 36, 1058–1069. doi: 10.1111/j.1600-0587.2013.07872.x
Milanesi, P., Breiner, F. T., Puopolo, F., and Holderegger, R. (2017). European human-dominated landscapes provide ample space for the recolonization of large carnivore populations under future land change scenarios. Ecography 40, 1359–1368. doi: 10.1111/ecog.02223
Mishra, C. (1997). Livestock depredation by large carnivores in the Indian trans-Himalaya: conflict perceptions and conservation prospects. Environ. Conserv. 24, 338–343. doi: 10.1017/s0376892997000441
Mladenoff, D. J., Sickley, T. A., Haight, R. G., and Wydeven, A. P. (1995). A regional landscape analysis and prediction of favorable gray wolf habitat in the northern Great Lakes region. Conserv. Biol. 9, 279–294. doi: 10.1046/j.1523-1739.1995.9020279.x
Morales, N. S., Fernández, I. C., and Baca-González, V. (2017). MaxEnt’s parameter configuration and small samples: are we paying attention to recommendations? A systematic review. PeerJ 5:e3093. doi: 10.7717/peerj.3093
Namgail, T., Fox, J. L., and Bhatnagar, Y. V. (2007). Carnivore-caused livestock mortality in trans-Himalaya. Environ. Manage. 39, 490–496. doi: 10.1007/s00267-005-0178-2
Pamperin, N. J., Follmann, E. H., and Petersen, B. (2006). Interspecific killing of an arctic fox by a red fox at Prudhoe Bay, Alaska. Arctic 59, 361–364.
Paquet, P. C., and Carbyn, L. N. (2003). “Gray wolf,” in Wild Mammals of North America: Biology, Management, and Conservation, 2nd Edn, (Baltimore, MD: The Johns Hopkins University Press), 482–510.
Parmesan, C. (2006). Ecological and evolutionary responses to recent climate change. Annu. Rev. Ecol. Evol. Syst. 37, 637–669. doi: 10.1146/annurev.ecolsys.37.091305.110100
Pecl, G. T., Araújo, M. B., Bell, J. D., Blanchard, J., Bonebrake, T. C., Chen, I. C., et al. (2017). Biodiversity redistribution under climate change: impacts on ecosystems and human well-being. Science 355, 14–21. doi: 10.1126/science.aai9214
Phillips, S. J., Anderson, R. P., and Schapire, R. E. (2006). Maximum entropy modeling of species geographic distributions. Ecol. Modell. 190, 231–259. doi: 10.1016/j.ecolmodel.2005.03.026
Phillips, S. J., and Dudík, M. (2008). Modeling of species distributions with Maxent: new extensions and a comprehensive evaluation. Ecography 31, 161–175. doi: 10.1111/j.2007.0906-7590.05203.x
Phillips, S. J., Dudík, M., Elith, J., Graham, C. H., Lehmann, A., Leathwick, J., et al. (2009). Sample selection bias and presence-only distribution models: implications for background and pseudo-absence data. Ecol. Appl. 19, 181–197. doi: 10.1890/07-2153.1
Pocock, R. I. (1941). The Fauna of British India, Including Ceylon and Burma: Mammalia. II. London: Taylor & Francis.
Pruvot, M., Fine, A. E., Hollinger, C., Strindberg, S., Damdinjav, B., Buuveibaatar, B., et al. (2020). Outbreak of peste des petits ruminants among critically endangered mongolian saiga and other wild ungulates, mongolia, 2016–2017. Emerg. Infect. Dis. 26:51. doi: 10.3201/eid2601.181998
Radchuk, V., Reed, T., Teplitsky, C., Van De Pol, M., Charmantier, A., Hassall, C., et al. (2019). Adaptive responses of animals to climate change are most likely insufficient. Nat. Commun. 10, 1–14. doi: 10.1038/s41467-019-10924-4
Radosavljevic, A., and Anderson, R. P. (2014). Making better Maxent models of species distributions: complexity, overfitting and evaluation. J. Biogeogr. 41, 629–643. doi: 10.1111/jbi.12227
Rana, D. B., Shrestha, B., Karki, J. B., and Shrestha, H. L. (2018). Distribution Occupancy, Potential Habitat and Conservation Of Recolonized Himalayan Wolf In Upper Mustang, Annapurna Conservation Area, Nepal Doctoral Dissertation. Kathmandu: Tribhuvan University.
Reshamwala, H. S., Mahar, N., Dirzo, R., and Habib, B. (2021). Successful neighbour: interactions of the generalist carnivore red fox with dogs, wolves and humans for continued survival in dynamic anthropogenic landscapes. Glob. Ecol. Conserv. 25:e01446. doi: 10.1016/j.gecco.2020.e01446
Schewe, J., and Levermann, A. (2012). A statistically predictive model for future monsoon failure in India. Environ. Res. Lett. 7:044023. doi: 10.1088/1748-9326/7/4/044023
Seo, C., Thorne, J. H., Hannah, L., and Thuiller, W. (2009). Scale effects in species distribution models: implications for conservation planning under climate change. Biol. Lett. 5, 39–43. doi: 10.1098/rsbl.2008.0476
Sharma, D. K., Maldonado, J. E., Jhala, Y. V., and Fleischer, R. C. (2004). Ancient wolf lineages in India. Proceedings of the royal society of London. Series B Biol. Sci. 271(suppl. 3), S1–S4. doi: 10.1098/rsbl.2003.0071
Shrotriya, S., Lyngdoh, S., and Habib, B. (2012). Wolves in trans-Himalayas: 165 years of taxonomic confusion. Curr. Sci. 103, 885–887.
Sleeter, B. M., Sohl, T. L., Bouchard, M. A., Reker, R. R., Soulard, C. E., Acevedo, W., et al. (2012). Scenarios of land use and land cover change in the conterminous United States: utilizing the special report on emission scenarios at ecoregional scales. Glob. Environ. Change 22, 896–914. doi: 10.1016/j.gloenvcha.2012.03.008
Subba, S. A., Shrestha, A. K., Thapa, K., Malla, S., Thapa, G. J., Shrestha, S., et al. (2017). Distribution of grey wolves Canis lupus lupus in the Nepalese Himalaya: implications for conservation management. Oryx 51, 403–406. doi: 10.1017/S0030605316000296
Terborgh, J., Holt, R. D., Estes, J. A., Terborgh, J., and Estes, J. (2010). “Trophic cascades: what they are, how they work, and why they matter” in Trophic Cascades: Predators, Prey, and the Changing Dynamics of Nature, Vol. 1, eds J. Terborgh and J. A. Estes (Washington, DC: Island Press), 18.
Trouwborst, A., and Blackmore, A. (2020). Hot dogs, hungry bears, and wolves running out of mountain—international wildlife law and the effects of climate change on large carnivores. J. Int. Wildl. Law Policy 23, 212–238. doi: 10.1080/13880292.2020.1852671
Wabakken, P., Sand, H., Liberg, O., and Bjärvall, A. (2001). The recovery, distribution, and population dynamics of wolves on the Scandinavian peninsula, 1978-1998. Can. J. Zool. 79, 710–725. doi: 10.1139/cjz-79-4-710
Watanabe, T., Izumiyama, S., Gaunavinaka, L., and Anarbaev, M. (2010). Wolf depredation on livestock in the Pamir. Geogr. Stud. 85, 26–36. doi: 10.7886/hgs.85.26
Wazneh, H., Arain, M. A., and Coulibaly, P. (2020). Climate indices to characterize climatic changes across southern Canada. Meteorol. Appl. 27:e1861. doi: 10.1002/met.1861
Werhahn, G., Kusi, N., Li, X., Chen, C., Zhi, L., Martín, R. L., et al. (2019). Himalayan wolf foraging ecology and the importance of wild prey. Glob. Ecol. Conserv. 20:e00780. doi: 10.1016/j.gecco.2019.e00780
Werhahn, G., Liu, Y., Meng, Y., Cheng, C., Lu, Z., Atzeni, L., et al. (2020). Himalayan wolf distribution and admixture based on multiple genetic markers. J. Biogeogr. 47, 1272–1285. doi: 10.1111/jbi.13824
Werhahn, G., Senn, H., Ghazali, M., Karmacharya, D., Sherchan, A. M., Joshi, J., et al. (2018). The unique genetic adaptation of the Himalayan wolf to high-altitudes and consequences for conservation. Glob. Ecol. Conserv. 16:e00455. doi: 10.1016/j.gecco.2018.e00455
Werhahn, G., Senn, H., Kaden, J., Joshi, J., Bhattarai, S., Kusi, N., et al. (2017). Phylogenetic evidence for the ancient Himalayan wolf: towards a clarification of its taxonomic status based on genetic sampling from western Nepal. R. Soc. Open Sci. 4:170186. doi: 10.1098/rsos.170186
Wilmers, C. C., and Getz, W. M. (2005). Gray wolves as climate change buffers in yellowstone. PLoS Biol. 3:e92. doi: 10.1371/journal.pbio.0030092
Wisz, M. S., Hijmans, R. J., Li, J., Peterson, A. T., Graham, C. H., Guisan, A., et al. (2008). Effects of sample size on the performance of species distribution models. Divers. Distrib. 14, 763–773. doi: 10.1111/j.1472-4642.2008.00482.x
Wolf, C., and Ripple, W. J. (2017). Range contractions of the world’s large carnivores. R. Soc. Open Sci. 4:170052. doi: 10.1098/rsos.170052
Keywords: Central Asia, future prediction, habitat suitability, predator, species distribution model, global warming
Citation: Reshamwala HS, Bhattacharya A, Khan S, Shrotriya S, Lyngdoh SB, Goyal SP, Kanagaraj R and Habib B (2022) Modeling Potential Impacts of Climate Change on the Distribution of Wooly Wolf (Canis lupus chanco). Front. Ecol. Evol. 10:815621. doi: 10.3389/fevo.2022.815621
Received: 15 November 2021; Accepted: 17 March 2022;
Published: 18 April 2022.
Edited by:
Jay E. Diffendorfer, United States Geological Survey (USGS), United StatesReviewed by:
Robby R. Marrotte, Trent University, CanadaTainá Rocha, Rio de Janeiro Botanical Garden, Brazil
Copyright © 2022 Reshamwala, Bhattacharya, Khan, Shrotriya, Lyngdoh, Goyal, Kanagaraj and Habib. This is an open-access article distributed under the terms of the Creative Commons Attribution License (CC BY). The use, distribution or reproduction in other forums is permitted, provided the original author(s) and the copyright owner(s) are credited and that the original publication in this journal is cited, in accordance with accepted academic practice. No use, distribution or reproduction is permitted which does not comply with these terms.
*Correspondence: Bilal Habib, bh@wii.gov.in, bilalhabib1@gmail.com
†These authors have contributed equally to this work and share first authorship