New developments and opportunities for AI in viticulture, pomology, and soft-fruit research: a mini-review and invitation to contribute articles
- 1Digital Agriculture, Food and Wine Research Group, School of Agriculture, Food and Ecosystem Sciences, Faculty of Science, The University of Melbourne, Melbourne, VIC, Australia
- 2Tecnologico de Monterrey, School of Engineering and Sciences, Monterrey, Mexico
Climate change constraints on horticultural production and emerging consumer requirements for fresh and processed horticultural products with an increased number of quality traits have pressured the industry to increase the efficiency, sustainability, productivity, and quality of horticultural products. The implementation of Agriculture 4.0 using new and emerging digital technologies has increased the amount of data available from the soil–plant–atmosphere continuum to support decision-making in these agrosystems. However, to date, there has not been a unified effort to work with these novel digital technologies and gather data for precision farming. In general, artificial intelligence (AI), including machine/deep learning for data modeling, is considered the best approach for analyzing big data within the horticulture and agrifood sectors. Hence, the terms Agriculture/AgriFood 5.0 are starting to be used to identify the integration of digital technologies from precision agriculture and data handling and analysis using AI for automation. This mini-review focuses on the latest published work with a soil–plant–atmosphere approach, especially those published works implementing AI technologies and modeling strategies.
1 Introduction
In the past two decades, agriculture in general has been affected by market challenges driven by climate change adversities and global consumer pressures pertaining to the quality and sustainability of agricultural products, which have forced the horticulture and agrifood industries to be more sustainable and ethical to minimize their environmental footprints. Implementing Agriculture 4.0 using new and emerging digital technologies has enhanced the application of precision agriculture (PA) through technologies such as remote sensing, robotics, digital sensor networks, and the Internet of Things (IoT). The latest technologies have helped to increase the efficiency of and sustainability targets for horticultural production (Javaid et al., 2022; Maffezzoli et al., 2022). However, digital technologies have not been broadly implemented throughout all horticulture and agrifood production and supply chains. There is still a disconnect and lack of feedback/forward information among agricultural processes, food processing, packaging, and consumer appreciation/acceptability (Fuentes et al., 2021b).
New and emerging technologies, such as artificial intelligence (AI) and related disciplines, including machine/deep learning, robotics, computer vision, biometrics for sensory and consumer analysis, and digital twins, can help to fill the gaps within the agrifood sectors and production and supply chains. By implementing AI, a new agrifood revolution, or Agriculture 5.0, can be discussed. These advances reflect the latest figures reported on AI, which suggest that in nearly 98% of scientific fields, including agriculture and horticulture, AI has already been implemented in some capacity, with 5.7% of all peer-reviewed research papers published worldwide focused on AI applications (Hajkowicz et al., 2022). Furthermore, it is expected that AI implementation in agriculture, with the main objectives of monitoring crops, soil analysis, increasing crop yield, and, ultimately, reducing costs, will grow by 26% globally between 2019 and 2025 (Research Markets, 2020). Nowadays, some type of technology for precision agriculture is being used in 15%–40% of large farms in the United States, 20% of those in Australia and Canada, 85% of those in Scotland, 43% of those in Ireland, and 30% of those in Germany, along with 68% of small farms in Western Europe (Kinhal, 2022).
In line with findings from the aforementioned report, there has been a considerable increase in the number of publications related to digital agriculture/horticulture in the past 5 years that contain descriptions of new sensor technologies applied to the agrifood sector, from production to processing, and acceptability by consumers using sensory analysis and biometrics (Gonzalez Viejo et al., 2019). However, much of the research has been limited to digital technologies and model development for only one or two crops and specific research sites, with minimal or no deployment of AI models. Hence, there is a need for future research implementing AI to focus on the independent deployment options for the different applications and models developed.
This mini-review focuses on the latest published work based on a soil–plant–atmosphere approach, especially those published works implementing AI technologies and modeling strategies. It discusses the advantages and disadvantages of the methodologies proposed and how they should be tested, validated, and integrated throughout agrifood production and supply chains.
2 Digital technologies implemented for viticulture, pomology, and soft fruits
This mini-review was based on research papers published in the past 5 years. As mentioned before, due to the number of publications related to digital technologies in the previous 10 years, it would be impossible to cover all the research on crops and cultivars that has been conducted so far. Hence, this review focuses on the information from new and emerging technologies obtained from the latest papers related to the specific areas of viticulture (Table 1), pomology (Table 2), and soft fruits (Table 2).
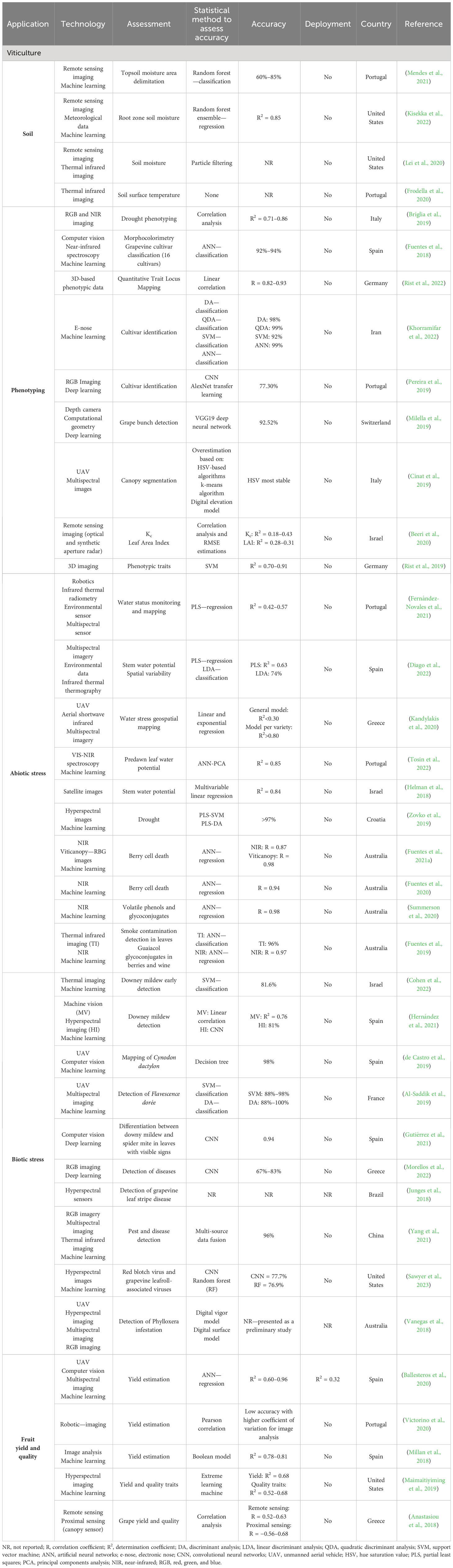
Table 1 Recent applications of digital technologies to viticulture displaying the technology used, the accuracy of the methods or models used, and details regarding deployment experiments (no = not conducted; % = deployment accuracy).

Table 2 Recent applications of digital technologies to pomology displaying the technology used accuracy of the methods or models and deployment (No = not conducted; % = deployment accuracy).
3 Discussion
The research presented in this paper is a fair sample of the latest research on digital technologies including AI in horticulture. However, most of it did not report any attempt at deployment of the models developed, and the majority of the studies that did include it reported low performance (R2 < 0.52; Tables 1, 2), with the exception of two studies with deployments on yield prediction ~85% (Table 2). These results reflect the main concern of and criticism articulated by AI scientists, who state that “even a system that appears to perform spectacularly in training can make terrible predictions when presented with novel data in the world” (Crawford, 2021). Therefore, deploying AI models in horticulture should be a must for future publications.
Creating a successful AI pilot model starts with identifying Goldilocks problems in horticulture that can be solved by the application of AI modeling techniques based on digital sensors and technologies (Rochwerger and Pang, 2021). Most research is focused on technologies that address problems at the block, orchard, or regional scales that do not offer significant advantages compared with other more established technologies from PA, remote sensing, or data analysis from meteorological stations (i.e., evapotranspiration estimation for irrigation scheduling or biotic stress management). On the other hand, AI models offering assessments of targets at the plant-by-plant scale or sub-meter scales offer little practical management information if the management is at a block, orchard, or regional scale. These Goldilocks problems can be identified for specific crops and environments. One of the most crucial resources in the production of horticultural crops that must be managed efficiently is water. Hence, an increased number of models have been developed to accurately estimate plant water consumption and increase water use efficiency, and this has direct implications for fruit yield and fruit quality traits (Tables 1, 2). The other common targets for AI modeling are fertilization, canopy management and vigor assessment, pest and disease detection and management, phenotyping for fruit quality estimations and crop improvement and yield, among other things. Moreover, as mentioned before, the management scale, in terms of temporal and spatial scales, should be similar to the one considered by the AI model development.
The most common and efficient inputs for AI modeling are based on data that is relatively easy to collect at the orchard level, either historically (i.e., management and phenology history, meteorological data, soil–plant–atmosphere-based sensor technologies) or through the implementation of new and emerging sensor technologies based on remote sensing employing long-range remote sensing via unmanned aerial vehicles (UAV) of short/proximal range, or manned or unmanned terrestrial vehicles (UTV) (Fuentes and Gago, 2022). In addition, growers should know if they have the correct data to assess the targets of interest at the required temporal and spatial resolution. For example, the use of AI models based on Landsat multispectral data (30 m× 30 m pixel) to assess the incidence of water stress at the plant-per-plant level of a tomato crop would be ineffective, since at the spatial resolution scale the pixel footprint considers over 200 plants, and from the temporal resolution having an image every 15 days (satellite overpass) may not be appropriate for detecting water stress with daily fluctuations.
One of the main principles to consider when modeling using AI is the parsimony of input data compared with the targets considered. In other words, the inputs for AI modeling should be simpler to acquire than the targeted information. Furthermore, AI models developed should offer a certain level of automation in data acquisition, processing, and decision-making information to growers.
Many early criticisms of AI modeling were that they were “black boxes”, in the sense that there was no option to see how models treated the data that arrived at specific targets, especially in cases of unsupervised machine learning or deep learning, in which the machine automatically extracts parameters of importance from inputs to model target responses. However, the advances made in machine/deep learning have made this argument obsolete. The latter statement is less applicable in the case of supervised machine-learning modeling since an essential initial step is parameter engineering, in which the modeler decides which parameters/data are more relevant to model the patterns of behavior for a specific target (i.e., specific meteorological data for specific biotic/abiotic stress detection). Hence, modelers should have detailed knowledge of the physical and biological processes affecting particular crops and their effects on the fruit yield and fruit quality traits required.
Growers should also be aware of the realistic steps involved in the production of AI models and the level of dependence for the maintenance and modification of the models implemented. Currently, these services are offered by several digital and AI agricultural companies, which makes access to specific models complex and accompanied by the risk that applicability may not be the most efficient for particular grower conditions. However, this last bottleneck could be solved in the next decade since high-ranking educational institutions and universities are offering more and more agricultural science and agronomy educational programs that incorporate digital agriculture principles and specific training on digital technologies, sensors, and remote sensing platforms, including data analysis using AI and decision-making automation through the use of digital twins (Ahmad et al., 2022).
Finally, one of the most common bottlenecks for AI technology adoption by growers has historically been the ownership of data. Even before full-scale research on AI modeling strategies for horticulture and other digital technologies was conducted, data ownership was a concern for PA from the mid-1980s. However, it has been proposed that this issue can be solved by treating data as currency through blockchain technology and implementing a digital ledger that will allow growers to know how the data obtained from their orchards have been used and who is using them, to grant permissions and relevant rights through licenses, and to obtain royalties (Fuentes and Gago, 2022).
There is a growing interest in the use of drones and computer vision as aids to monitor farm conditions and to support management strategies to increase the quality traits of produce. These have been developed and offered by either researchers or external companies such as Blue River Technology, Ilumina, and Trace Genomics based in California, United States, for famers, and these technologies have contributed to farmers obtaining higher yields and achieving higher-quality production (Walch, 2019; USM, 2022). The latter applications, using digital technologies and remote sensing, are collectively known as Agriculture 4.0. Currently, the implementation of AI in agriculture in the form of data handling and modeling using machine/deep learning has been successful in enabling farmers to handle large amounts of historical and real-time data (big data), such as those on weather information, soil conditions, and water usage (among other management strategies), which have aided in their timely decision-making. Farmers have also been using AI in Precision Agriculture for pests and diseases, nutrition needs detection, and management strategies. Precision Agriculture is considered an advancement on Agriculture 4.0, and combining AI with digital agriculture has advanced the terminology to Agriculture 5.0 (Fuentes et al., 2023).
The implementation of AI in the future could be ubiquitous and necessary to deal with an increased amount of data produced by new and emerging digital sensor technologies applied to the horticulture and agrifood sectors. This could be the case for producing horticultural crops using vertical farming systems, in which fully controlled conditions can be simulated using digital twins to manipulate the phenotype and genotype plasticity of different crops to vary fruit quality traits (Kugler, 2022; Siregar et al., 2022). These technologies and AI applications can not only decrease world hunger by increasing the efficiency needed to handle the growing demand for food based on the forecasted population growth (Revanth, 2019), maximizing fruit production efficiency and minimizing food waste and the environmental footprint associated with food production, but also be the basis for food production outside Earth. For long-term space missions, such as the NASA Artemis program from Earth to the Moon (by 2030) and from the Moon to Mars (by 2040), the use of advanced biological and genetic technologies will be required if plants are to be grown in space. Food, beverages, materials, and pharmaceuticals should then be produced using AI digital twins developed using research based on the experience of Agriculture 5.0. The latter plan may seem extremely futuristic; however, these are the current aims of the Australian Research Council (ARC) Centre of Excellence in Plants for Space with the University of Melbourne, Australia, as one of the five Australian universities with more than 38 additional partners, including international universities and space agencies (e.g., Australian Space Agency and NASA), and companies such as Axiom (ARC, 2022).
Author contributions
SF: Investigation, Writing – original draft, Writing – review & editing. ET: Writing – review & editing. CGV: Investigation, Writing – original draft, Writing – review & editing.
Funding
The author(s) declare that no financial support was received for the research, authorship, and/or publication of this article.
Conflict of interest
The authors declare that the research was conducted in the absence of any commercial or financial relationships that could be construed as a potential conflict of interest.
The authors CGV and SF declared that they were editorial board members of Frontiers, at the time of submission. This had no impact on the peer review process and the final decision.
Publisher’s note
All claims expressed in this article are solely those of the authors and do not necessarily represent those of their affiliated organizations, or those of the publisher, the editors and the reviewers. Any product that may be evaluated in this article, or claim that may be made by its manufacturer, is not guaranteed or endorsed by the publisher.
References
Abd-Elrahman A., Guan Z., Dalid C., Whitaker V., Britt K., Wilkinson B., et al. (2020). Automated canopy delineation and size metrics extraction for strawberry dry weight modeling using raster analysis of high-resolution imagery. Remote Sens. 12, 3632. doi: 10.3390/rs12213632
Abdulridha J., Ehsani R., Abd-Elrahman A., Ampatzidis Y. (2019). A remote sensing technique for detecting laurel wilt disease in avocado in presence of other biotic and abiotic stresses. Comput. Electron. Agric. 156, 549–557. doi: 10.1016/j.compag.2018.12.018
Ahmad A., Noor S. E., Cassinello P. C., Núñez V. M. (2022). Artificial Intelligence (AI) as a complementary technology for agricultural Remote Sensing (RS) in plant physiology teaching. ReiDoCrea: Rev. electrónica investigación y docencia creativa 11, 695–701. doi: 10.30827/Digibug.77656
Al-Saddik H., Simon J.-C., Cointault F. (2019). Assessment of the optimal spectral bands for designing a sensor for vineyard disease detection: the case of ‘Flavescence dorée’. Precis. Agric. 20, 398–422. doi: 10.1007/s11119-018-9594-1
Anastasiou E., Balafoutis A., Darra N., Psiroukis V., Biniari A., Xanthopoulos G., et al. (2018). Satellite and proximal sensing to estimate the yield and quality of table grapes. Agriculture 8, 94. doi: 10.3390/agriculture8070094
Appiah S. A., Li J., Lan Y., Darko R. O., Alordzinu K. E., Al Aasmi A., et al. (2022). Real-time assessment of mandarin crop water stress index. Sensors 22, 4018. doi: 10.3390/s22114018
ARC (2022) ARC Centre of Excellence. Available at: https://www.arc.gov.au/funding-research/discovery-linkage/linkage-program/arc-centres-excellence/arc-centre-excellence-plants-space.
Bagheri N. (2020). Application of aerial remote sensing technology for detection of fire blight infected pear trees. Comput. Electron. Agric. 168, 105147. doi: 10.1016/j.compag.2019.105147
Ballesteros R., Intrigliolo D. S., Ortega J. F., Ramírez-Cuesta J. M., Buesa I., Moreno M. A. (2020). Vineyard yield estimation by combining remote sensing, computer vision and artificial neural network techniques. Precis. Agric. 21, 1242–1262. doi: 10.1007/s11119-020-09717-3
Bardhan S., Bagchi S., Jenamani M., Routray A. (2020). “Non-Invasive method using Contact-less Sensors and Embedded Platform for Monitoring Quality determining factors of Indian Mangoes,” in IECON 2020 The 46th Annual Conference of the IEEE Industrial Electronics Society. 18-21 Oct. 2020. (Singapore: IEEE), 2281–2285.
Beeri O., Netzer Y., Munitz S., Mintz D. F., Pelta R., Shilo T., et al. (2020). Kc and LAI estimations using optical and SAR remote sensing imagery for vineyards plots. Remote Sens. 12, 3478. doi: 10.3390/rs12213478
Bellvert J., Adeline K., Baram S., Pierce L., Sanden B. L., Smart D. R. (2018). Monitoring crop evapotranspiration and crop coefficients over an almond and pistachio orchard throughout remote sensing. Remote Sens. 10, 2001. doi: 10.3390/rs10122001
Blanco V., Blaya-Ros P. J., Castillo C., Soto-Vallés F., Torres-Sánchez R., Domingo R. (2020). Potential of UAS-based remote sensing for estimating tree water status and yield in sweet cherry trees. Remote Sens. 12, 2359. doi: 10.3390/rs12152359
Bleasdale A., Blackburn G., Whyatt J. (2022). Feasibility of detecting apple scab infections using low-cost sensors and interpreting radiation interactions with scab lesions. Int. J. Remote Sens. 43, 4984–5005. doi: 10.1080/01431161.2022.2122895
Briglia N., Montanaro G., Petrozza A., Summerer S., Cellini F., Nuzzo V. (2019). Drought phenotyping in Vitis vinifera using RGB and NIR imaging. Scientia Hortic. 256, 108555. doi: 10.1016/j.scienta.2019.108555
Carrasco-Benavides M., Viejo C. G., Tongson E., Baffico-Hernández A., Ávila-Sánchez C., Mora M., et al. (2022). Water status estimation of cherry trees using infrared thermal imagery coupled with supervised machine learning modeling. Comput. Electron. Agric. 200, 107256. doi: 10.1016/j.compag.2022.107256
Chakraborty M., Khot L. R., Sankaran S., Jacoby P. W. (2019). Evaluation of mobile 3D light detection and ranging based canopy mapping system for tree fruit crops. Comput. Electron. Agric. 158, 284–293. doi: 10.1016/j.compag.2019.02.012
Chalupowicz D., Veltman B., Droby S., Eltzov E. (2020). Evaluating the use of biosensors for monitoring of Penicillium digitatum infection in citrus fruit. Sensors actuators B: Chem. 311, 127896. doi: 10.1016/j.snb.2020.127896
Chan C., Nelson P. R., Hayes D. J., Zhang Y.-J., Hall B. (2021). Predicting water stress in wild blueberry fields using airborne visible and near infrared imaging spectroscopy. Remote Sens. 13 1425. doi: 10.3390/rs13081425
Chaudhary M., Gastli M. S., Nassar L., Karray F. (2021). “Transfer learning application for berries yield forecasting using deep learning,” in 2021 International Joint Conference on Neural Networks (IJCNN). 18-22 July 2021. (Shenzhen, China: IEEE), 1–8.
Chen Y., Hou C., Tang Y., Zhuang J., Lin J., He Y., et al. (2019a). Citrus tree segmentation from UAV images based on monocular machine vision in a natural orchard environment. Sensors 19, 5558. doi: 10.3390/s19245558
Chen Y., Lee W. S., Gan H., Peres N., Fraisse C., Zhang Y., et al. (2019b). Strawberry yield prediction based on a deep neural network using high-resolution aerial orthoimages. Remote Sens. 11, 1584. doi: 10.3390/rs11131584
Chen R., Zhang C., Xu B., Zhu Y., Zhao F., Han S., et al. (2022). Predicting individual apple tree yield using UAV multi-source remote sensing data and ensemble learning. Comput. Electron. Agric. 201, 107275. doi: 10.1016/j.compag.2022.107275
Choudhury R., Hazarika S., Sarma U. (2019). Detection of water stress in Khasi mandarin orange plants from volatile organic compound emission profile implementing electronic nose. Int. J. Eng. Adv. Technol. 9, 133–137. doi: 10.35940/ijeat.A1086.109119
Cinat P., Gennaro Di, Berton A., Matese A. (2019). Comparison of unsupervised algorithms for Vineyard Canopy segmentation from UAV multispectral images. Remote Sens. 11, 1023. doi: 10.3390/rs11091023
Cohen B., Edan Y., Levi A., Alchanatis V. (2022). Early detection of grapevine (Vitis vinifera) downy mildew (Peronospora) and diurnal variations using thermal imaging. Sensors 22, 3585. doi: 10.3390/s22093585
Crawford K. (2021). The atlas of AI: Power, politics, and the planetary costs of artificial intelligence (New Haven, Connecticut, USA: Yale University Press).
Cruz M., Mafra S., Teixeira E., Figueiredo F. (2022). Smart strawberry farming using edge computing and IoT. Sensors 22, 5866. doi: 10.3390/s22155866
de Castro A. I., Peña J. M., Torres-Sánchez J., Jiménez-Brenes F. M., Valencia-Gredilla F., Recasens J., et al. (2019). Mapping cynodon dactylon infesting cover crops with an automatic decision tree-OBIA procedure and UAV imagery for precision viticulture. Remote Sens. 12, 56. doi: 10.3390/rs12010056
Diago M. P., Tardaguila J., Barrio I., Fernández-Novales J. (2022). Combination of multispectral imagery, environmental data and thermography for on-the-go monitoring of the grapevine water status in commercial vineyards. Eur. J. Agron. 140, 126586. doi: 10.1016/j.eja.2022.126586
Dujak C., Jurado F., Aranzana M. J. (2023). Comprehensive Morphometric Analysis of Apple Fruits and Weighted Class Assignation using Machine Learning. 02 August 2023, PREPRINT (Version 1). doi: 10.21203/rs.3.rs-2860631/v1
Durfee N., Ochoa C. G., Mata-Gonzalez R. (2019). The use of low-altitude UAV imagery to assess western juniper density and canopy cover in treated and untreated stands. Forests 10, 296. doi: 10.3390/f10040296
Feng J., Zeng L., He L. (2019). Apple fruit recognition algorithm based on multi-spectral dynamic image analysis. Sensors 19, 949. doi: 10.3390/s19040949
Fernández-Novales J., Saiz-Rubio V., Barrio I., Rovira-Más F., Cuenca-Cuenca A., Santos Alves F., et al. (2021). Monitoring and mapping vineyard water status using non-invasive technologies by a ground robot. Remote Sens. 13, 2830. doi: 10.3390/rs13142830
Frodella W., Lazzeri G., Moretti S., Keizer J., Verheijen F. G. (2020). Applying infrared thermography to soil surface temperature monitoring: Case study of a high-resolution 48 h survey in a vineyard (Anadia, Portugal). Sensors 20, 2444. doi: 10.3390/s20092444
Fuentes S., Gago J. (2022). “Modern approaches to precision and digital viticulture,” in Improving sustainable viticulture and winemaking practices. (San Diego, USA: Elsevier).
Fuentes S., Gonzalez Viejo C., Hall C., Tang Y., Tongson E. (2021a). Berry cell vitality assessment and the effect on wine sensory traits based on chemical fingerprinting, canopy architecture and machine learning modelling. Sensors 21, 7312. doi: 10.3390/s21217312
Fuentes S., Hernández-Montes E., Escalona J., Bota J., Viejo C. G., Poblete-Echeverría C., et al. (2018). Automated grapevine cultivar classification based on machine learning using leaf morpho-colorimetry, fractal dimension and near-infrared spectroscopy parameters. Comput. Electron. Agric. 151, 311–318. doi: 10.1016/j.compag.2018.06.035
Fuentes S., Tongson E., Chen J., Gonzalez Viejo C. (2020). A digital approach to evaluate the effect of berry cell death on pinot noir wines’ Quality traits and sensory profiles using non-destructive near-infrared spectroscopy. Beverages 6, 39. doi: 10.3390/beverages6020039
Fuentes S., Tongson E. J., De Bei R., Gonzalez Viejo C., Ristic R., Tyerman S., et al. (2019). Non-invasive tools to detect smoke contamination in grapevine canopies, berries and wine: A remote sensing and machine learning modeling approach. Sensors 19, 3335. doi: 10.3390/s19153335
Fuentes S., Tongson E., Gonzalez Viejo C. (2023). “Artificial intelligence and big data revolution in the agri-food sector,” in Food Industry 4.0: Emerging Trends and Technologies in Sustainable Food Production and Consumption. Ed. Hassoun A. (San Diego, USA: Elsevier).
Fuentes S., Tongson E., Viejo C. G. (2021b). Novel digital technologies implemented in sensory science and consumer perception. Curr. Opin. Food Sci. 41, 99–106. doi: 10.1016/j.cofs.2021.03.014
Gao X., Han W., Hu Q., Qin Y., Wang S., Lun F., et al. (2023). Planting age identification and yield prediction of apple orchard using time-series spectral endmember and logistic growth model. Remote Sens. 15, 642. doi: 10.3390/rs15030642
Gao Z., Zhao Y., Hoheisel G.-A., Khot L. R., Zhang Q. (2021). Blueberry bud freeze damage detection using optical sensors: Identification of spectral features through hyperspectral imagery. J. Berry Res. 11, 631–646. doi: 10.3233/JBR-211506
Gao Z., Zhao Y., Khot L. R., Hoheisel G.-A., Zhang Q. (2019). Optical sensing for early spring freeze related blueberry bud damage detection: Hyperspectral imaging for salient spectral wavelengths identification. Comput. Electron. Agric. 167, 105025. doi: 10.1016/j.compag.2019.105025
García-Tejero I., Gutiérrez-Gordillo S., Ortega-Arévalo C., Iglesias-Contreras M., Moreno J., Souza-Ferreira L., et al. (2018). Thermal imaging to monitor the crop-water status in almonds by using the non-water stress baselines. Scientia Hortic. 238, 91–97. doi: 10.1016/j.scienta.2018.04.045
Gonzalez Viejo C., Torrico D. D., Dunshea F. R., Fuentes S. (2019). Emerging technologies based on artificial intelligence to assess the quality and consumer preference of beverages. Beverages 5, 62. doi: 10.3390/beverages5040062
Guan Z., Abd-Elrahman A., Fan Z., Whitaker V. M., Wilkinson B. (2020). Modeling strawberry biomass and leaf area using object-based analysis of high-resolution images. ISPRS J. Photogramm. Remote Sens. 163, 171–186. doi: 10.1016/j.isprsjprs.2020.02.021
Gutiérrez S., Hernández I., Ceballos S., Barrio I., Díez-Navajas A. M., Tardaguila J. (2021). Deep learning for the differentiation of downy mildew and spider mite in grapevine under field conditions. Comput. Electron. Agric. 182, 105991. doi: 10.1016/j.compag.2021.105991
Hajkowicz S., Naughtin C., Sanderson C., Schleiger E., Karimi S., Bratanova A., et al. (2022). “Artificial intelligence for science – Adoption trends and future development pathways,” in CSIRO Data61. (Brisbane, Australia: CSIRO).
Hazarika S., Choudhury R., Montazer B., Medhi S., Goswami M. P., Sarma U. (2020). Detection of citrus tristeza virus in mandarin orange using a custom-developed electronic nose system. IEEE Trans. Instrumentation Measurement 69, 9010–9018. doi: 10.1109/TIM.2020.2997064
Helman D., Bahat I., Netzer Y., Ben-Gal A., Alchanatis V., Peeters A., et al. (2018). Using time series of high-resolution planet satellite images to monitor grapevine stem water potential in commercial vineyards. Remote Sens. 10, 1615. doi: 10.3390/rs10101615
Hernández I., Gutiérrez S., Ceballos S., Iñíguez R., Barrio I., Tardaguila J. (2021). Artificial intelligence and novel sensing technologies for assessing downy mildew in grapevine. Horticulturae 7, 103. doi: 10.3390/horticulturae7050103
Huang Y., Wang D., Liu Y., Zhou H., Sun Y. (2020). Measurement of early disease blueberries based on vis/nir hyperspectral imaging system. Sensors 20, 5783. doi: 10.3390/s20205783
Ionescu L., Mazare A., Serban G., Chitu E., Lita A. (2019). Intelligent monitoring and analysis system of soil moisture parameters and trunk diameter used in fruit tree culture. 2019 IEEE 25th International Symposium for Design and Technology in Electronic Packaging (SIITME). 23-26 October 2019. (Cluj-Napoca, Romania: IEEE), 252–255.
Javaid M., Haleem A., Singh R. P., Suman R. (2022). Enhancing smart farming through the applications of Agriculture 4.0 technologies. Int. J. Intell. Netw. 3, 150–164. doi: 10.1016/j.ijin.2022.09.004
Junges A. H., Ducati J. R., Lampugnani C. S., Almança M. A. K. (2018). Detection of grapevine leaf stripe disease symptoms by hyperspectral sensor. Phytopathol. Mediterr. 57, 399–406. doi: 10.14601/Phytopathol_Mediterr-22862
Kandylakis Z., Falagas A., Karakizi C., Karantzalos K. (2020). Water Stress Estimation in Vineyards from Aerial SWIR and multispectral UAV data. Remote Sens. 12, 2499. doi: 10.3390/rs12152499
Khorramifar A., Karami H., Wilson A. D., Sayyah A. H. A., Shuba A., Lozano J. (2022). Grape cultivar identification and classification by machine olfaction analysis of leaf volatiles. Chemosensors 10, 125. doi: 10.3390/chemosensors10040125
Kinhal V. (2022) Precision Agriculture Policy & Adoption Outlook 2023. Available at: https://cid-inc.com/blog/precision-agriculture-policy-adoption-outlook-2023/ (Accessed 9 March 2023).
Kisekka I., Peddinti S. R., Kustas W. P., Mcelrone A. J., Bambach-Ortiz N., Mckee L., et al. (2022). Spatial–temporal modeling of root zone soil moisture dynamics in a vineyard using machine learning and remote sensing. Irrigation Sci. 40, 761–777. doi: 10.1007/s00271-022-00775-1
Kugler L. (2022). Artificial intelligence, machine learning, and the fight against world hunger. Commun. ACM 65, 17–19. doi: 10.1145/3503779
Latha M., Jaya S. (2019). Detection of Septoria spot on blueberry leaf images using SVM classifier. ICTACT J. Image Video Process 9, 2015–2019. doi: 10.21917/ijivp.2019.0286
Lee S., Arora A. S., Yun C. (2022). Detecting strawberry diseases and pest infections in the very early stage with an ensemble deep-learning model. Front. Plant Sci. 4006. doi: 10.3389/fpls.2022.991134
Lei F., Crow W. T., Kustas W. P., Dong J., Yang Y., Knipper K. R., et al. (2020). Data assimilation of high-resolution thermal and radar remote sensing retrievals for soil moisture monitoring in a drip-irrigated vineyard. Remote Sens. Environ. 239, 111622. doi: 10.1016/j.rse.2019.111622
Ma H., Zhao K., Jin X., Ji J., Qiu Z., Gao S. (2019). Spectral difference analysis and identification of different maturity blueberry fruit based on hyperspectral imaging using spectral index. Int. J. Agric. Biol. Eng. 12, 134–140. doi: 10.25165/j.ijabe.20191203.4325
Macabiog R. E. N., Cruz J. C. D. (2019). “Soil moisture and rain prediction based irrigation controller for the strawberry farm of La Trinidad, Benguet,” in 2019 IEEE 11th International Conference on Humanoid, Nanotechnology, Information Technology, Communication and Control, Environment, and Management (HNICEM). 29 November 2019 - 01 December 2019. (Laoag, Philippines: IEEE), 1–6.
Maffezzoli F., Ardolino M., Bacchetti A., Perona M., Renga F. (2022). Agriculture 4.0: a systematic literature review on the paradigm, technologies and benefits. Futures 142, 102998. doi: 10.1016/j.futures.2022.102998
Mahmud M. S., Zaman Q. U., Esau T. J., Chang Y. K., Price G. W., Prithiviraj B. (2020). Real-time detection of strawberry powdery mildew disease using a mobile machine vision system. Agronomy 10, 1027. doi: 10.3390/agronomy10071027
Maimaitiyiming M., Sagan V., Sidike P., Kwasniewski M. T. (2019). Dual activation function-based Extreme Learning Machine (ELM) for estimating grapevine berry yield and quality. Remote Sens. 11, 740. doi: 10.3390/rs11070740
Mendes M. P., Matias M., Gomes R. C., Falcão A. P. (2021). Delimitation of low topsoil moisture content areas in a vineyard using remote sensing imagery (Sentinel-1 and Sentinel-2) in a Mediterranean-climate region. Soil Water Res. 16, 85–94. doi: 10.17221/101/2019-SWR
Milella A., Marani R., Petitti A., Reina G. (2019). In-field high throughput grapevine phenotyping with a consumer-grade depth camera. Comput. Electron. Agric. 156, 293–306. doi: 10.1016/j.compag.2018.11.026
Millán S., Casadesús J., Campillo C., Moñino M. J., Prieto M. H. (2019). Using soil moisture sensors for automated irrigation scheduling in a plum crop. Water 11 (10), 2061. doi: 10.3390/w11102061
Millan B., Velasco-Forero S., Aquino A., Tardaguila J. (2018). On-the-go grapevine yield estimation using image analysis and boolean model. J. Sensors 2018, 1–14. doi: 10.1155/2018/9634752
Mirnezami S. V., Hamidisepehr A., Ghaebi M., Hassan-Beygi S. R. (2020). “Apricot variety classification using image processing and machine learning approaches,” in Proceedings of the 2020 4th International Conference on Vision, Image and Signal Processing. 9-11 December 2020. (Bangkok, Thailand). 1–6.
Morellos A., Pantazi X. E., Paraskevas C., Moshou D. (2022). Comparison of deep neural networks in detecting field grapevine diseases using transfer learning. Remote Sens. 14, 4648. doi: 10.3390/rs14184648
Ni X., Li C., Jiang H., Takeda F. (2021). Three-dimensional photogrammetry with deep learning instance segmentation to extract berry fruit harvestability traits. ISPRS J. Photogramm. Remote Sens. 171, 297–309. doi: 10.1016/j.isprsjprs.2020.11.010
Noguera M., Millán B., Pérez-Paredes J. J., Ponce J. M., Aquino A., Andújar J. M. (2020). A new low-cost device based on thermal infrared sensors for olive tree canopy temperature measurement and water status monitoring. Remote Sens. 12, 723. doi: 10.3390/rs12040723
Nooriman W., Abdullah A., Rahim N. A., Kamarudin K. (2018). Development of wireless sensor network for Harumanis Mango orchard's temperature, humidity and soil moisture monitoring. 2018 IEEE Symposium on Computer Applications & Industrial Electronics (ISCAIE). 28-29 April 2018. (Penang, Malaysia: IEEE), 263–268.
Oliveira L. S. D., Castoldi R., Martins G. D., Medeiros M. H. (2023). Estimation of strawberry crop productivity by machine learning algorithms using data from multispectral images. Agronomy 13, 1229. doi: 10.3390/agronomy13051229
Pereira C. S., Morais R., Reis M. J. (2019). Deep learning techniques for grape plant species identification in natural images. Sensors 19, 4850. doi: 10.3390/s19224850
Pérez-Bueno M. L., Pineda M., Vida C., Fernández-Ortuño D., Torés J., De Vicente A., et al. (2019). Detection of white root rot in avocado trees by remote sensing. Plant Dis. 103, 1119–1125. doi: 10.1094/PDIS-10-18-1778-RE
Poobalasubramanian M., Park E.-S., Faqeerzada M. A., Kim T., Kim M. S., Baek I., et al. (2022). Identification of early heat and water stress in strawberry plants using chlorophyll-fluorescence indices extracted via hyperspectral images. Sensors 22, 8706. doi: 10.3390/s22228706
Rahman M. M., Robson A., Bristow M. (2018). Exploring the potential of high resolution worldview-3 Imagery for estimating yield of mango. Remote Sens. 10, 1866. doi: 10.3390/rs10121866
Ravi R., Kennedy S., Pitchay D. (2020). Distribution of volatile compounds in kaffir lime (Citrus hystrix) leaves grown in soilless substrate analyzed using electronic nose. Int. J. Plant Soil Sci. 32 (9), 1–9. doi: 10.9734/ijpss/2020/v32i930321
Research Markets. (2020). Artificial Intelligence in Agriculture Market by Technology, Offering, Application, and Geography - Global Forecast to 2026 - ResearchAndMarkets.com (Dublin, Ireland: Research Markets).
Revanth. (2019). Towards Future Farming: How Artificial Intelligence is Transforming the Agriculture Industry. Available at: https://www.wipro.com/holmes/towards-future-farming-how-artificial-intelligence-is-transforming-the-agriculture-industry/ (Accessed 17 April 2023).
Rist F., Gabriel D., Mack J., Steinhage V., Töpfer R., Herzog K. (2019). Combination of an automated 3D field phenotyping workflow and predictive modelling for high-throughput and non-invasive phenotyping of grape bunches. Remote Sens. 11, 2953. doi: 10.3390/rs11242953
Rist F., Schwander F., Richter R., Mack J., Schwandner A., Hausmann L., et al. (2022). Relieving the phenotyping bottleneck for grape bunch architecture in grapevine breeding research: implementation of a 3D-based phenotyping approach for quantitative trait locus mapping. Horticulturae 8, 907. doi: 10.3390/horticulturae8100907
Rochwerger A. S., Pang W. (2021). Real World AI: A Practical Guide for Responsible Machine Learning (Carson City, Nevada, USA: Lioncrest Publishing).
Salgadoe A. S. A., Robson A. J., Lamb D. W., Dann E. K., Searle C. (2018). Quantifying the severity of phytophthora root rot disease in avocado trees using image analysis. Remote Sens. 10, 226. doi: 10.3390/rs10020226
Sawyer E., Laroche-Pinel E., Flasco M., Cooper M. L., Corrales B., Fuchs M., et al. (2023). Phenotyping grapevine red blotch virus and grapevine leafroll-associated viruses before and after symptom expression through machine-learning analysis of hyperspectral images. Front. Plant Sci. 14, 1117869. doi: 10.3389/fpls.2023.1117869
Schoofs H., Delalieux S., Deckers T., Bylemans D. (2020). Fire blight monitoring in pear orchards by unmanned airborne vehicles (UAV) systems carrying spectral sensors. Agronomy 10, 615. doi: 10.3390/agronomy10050615
Siregar R. R. A., Seminar K. B., Wahjuni S., Santosa E. (2022). Vertical farming perspectives in support of precision agriculture using artificial intelligence: A review. Computers 11, 135. doi: 10.3390/computers11090135
Summerson V., Viejo C. G., Torrico D. D., Pang A., Fuentes S. (2020). Detection of smoke-derived compounds from bushfires in Cabernet-Sauvignon grapes, must, and wine using Near-Infrared spectroscopy and machine learning algorithms. OENO One 54, 1105–1119. doi: 10.20870/oeno-one.2020.54.4.4501
Tosin R., Martins R., Pôças I., Cunha M. (2022). Canopy VIS-NIR spectroscopy and self-learning artificial intelligence for a generalised model of predawn leaf water potential in Vitis vinifera. Biosyst. Eng. 219, 235–258. doi: 10.1016/j.biosystemseng.2022.05.007
Uryasheva A., Kalashnikova A., Shadrin D., Evteeva K., Moskovtsev E., Rodichenko N. (2022). Computer vision-based platform for apple leaves segmentation in field conditions to support digital phenotyping. Comput. Electron. Agric. 201, 107269. doi: 10.1016/j.compag.2022.107269
USM (2022) AI In Agriculture– Use Cases, Benefits, and Future. Available at: https://usmsystems.com/ai-in-agriculture-applications-of-ai-its-tools/ (Accessed 18 April 2023).
Vanegas F., Bratanov D., Powell K., Weiss J., Gonzalez F. (2018). A novel methodology for improving plant pest surveillance in vineyards and crops using UAV-based hyperspectral and spatial data. Sensors 18, 260. doi: 10.3390/s18010260
Victorino G. F., Braga R., Santos-Victor J., Lopes C. M. (2020). Yield components detection and image-based indicators for non-invasive grapevine yield prediction at different phenological phases. OENO One 54, 833–848. doi: 10.20870/oeno-one.2020.54.4.3616
Walch K. (2019) How AI Is Transforming Agriculture. Available at: https://www.forbes.com/sites/cognitiveworld/2019/07/05/how-ai-is-transforming-agriculture/?sh=550cd0bf4ad1 (Accessed 18 April 2023).
Wen T., Zheng L., Dong S., Gong Z., Sang M., Long X., et al. (2019). Rapid detection and classification of citrus fruits infestation by Bactrocera dorsalis (Hendel) based on electronic nose. Postharvest Biol. Technol. 147, 156–165. doi: 10.1016/j.postharvbio.2018.09.017
Weng S., Yu S., Guo B., Tang P., Liang D. (2020). Non-destructive detection of strawberry quality using multi-features of hyperspectral imaging and multivariate methods. Sensors 20, 3074. doi: 10.3390/s20113074
Wenter A., Burger R., Hafner H., Thalheimer M. (2021). A pilot study of sensor-based soil moisture assessment for precise irrigation scheduling in apple. XII Int. Symposium Integrating Canopy Rootstock Environ. Physiol. Orchard Syst. 1346, 557–562. doi: 10.17660/ActaHortic.2022.1346.70
Wu D., Johansen K., Phinn S., Robson A., Tu Y.-H. (2020). Inter-comparison of remote sensing platforms for height estimation of mango and avocado tree crowns. Int. J. Appl. Earth Obs. Geoinf. 89, 102091. doi: 10.1016/j.jag.2020.102091
Xiao J.-R., Chung P.-C., Wu H.-Y., Phan Q.-H., Yeh J.-L. A., Hou M. T.-K. (2020). Detection of strawberry diseases using a convolutional neural network. Plants 10, 31. doi: 10.3390/plants10010031
Yang R., Lu X., Huang J., Zhou J., Jiao J., Liu Y., et al. (2021). A multi-source data fusion decision-making method for disease and pest detection of grape foliage based on ShuffleNet V2. Remote Sens. 13, 5102. doi: 10.3390/rs13245102
Zhang C., Serra S., Quirós-Vargas J., Sangjan W., Musacchi S., Sankaran S. (2021). Non-invasive sensing techniques to phenotype multiple apple tree architectures. Inf. Process. Agric. 10, 136–147. doi: 10.1016/j.inpa.2021.02.001
Zhao T., Yang Y., Niu H., Wang D., Chen Y. (2018). Comparing U-Net convolutional network with mask R-CNN in the performances of pomegranate tree canopy segmentation. Multispectral hyperspectral ultraspectral Remote Sens. technology techniques Appl. VII 10780, 210–218. SPIE. doi: 10.1117/12.2325570
Zheng C., Abd-Elrahman A., Whitaker V., Dalid C. (2022). Prediction of strawberry dry biomass from UAV multispectral imagery using multiple machine learning methods. Remote Sens. 14, 4511. doi: 10.3390/rs14184511
Keywords: climate change, Agriculture 5.0, digital agriculture, remote sensing, machine/deep learning
Citation: Fuentes S, Tongson E and Gonzalez Viejo C (2023) New developments and opportunities for AI in viticulture, pomology, and soft-fruit research: a mini-review and invitation to contribute articles. Front. Hortic. 2:1282615. doi: 10.3389/fhort.2023.1282615
Received: 24 August 2023; Accepted: 20 September 2023;
Published: 11 October 2023.
Edited by:
Gastón Gutiérrez Gamboa, Instituto de Investigaciones Agropecuarias, ChileReviewed by:
Jorge Alejandro Prieto, Instituto Nacional de Tecnología Agropecuaria, ArgentinaCopyright © 2023 Fuentes, Tongson and Gonzalez Viejo. This is an open-access article distributed under the terms of the Creative Commons Attribution License (CC BY). The use, distribution or reproduction in other forums is permitted, provided the original author(s) and the copyright owner(s) are credited and that the original publication in this journal is cited, in accordance with accepted academic practice. No use, distribution or reproduction is permitted which does not comply with these terms.
*Correspondence: Sigfredo Fuentes, sfuentes@unimelb.edu.au