Editorial: Data sciences in transportation and transit systems
- 1Chulalongkorn University, Bangkok, Thailand
- 2Fulbright University Vietnam (FUV), Ho Chi Minh City, Vietnam
- 3Norwegian University of Science and Technology, Trondheim, Norway
- 4Department of Mechanical Engineering, Nihon University, Tokyo, Japan
- 5School of Civil Engineering, Birmingham, United Kingdom
Editorial on the Research Topic
Data sciences in transportation and transit systems
Disruptions in the operation of our countries’ infrastructure may put the functioning of our societies and their economies at risk. Such disruptions may result from many kinds of hazards and physical and/or cyberattacks on installations and systems. Recent events have demonstrated the increased interconnection among the impact of hazards, of the two kinds of attacks and, conversely, the usefulness for operators of combining cyber and physical security solutions to protect installations of the critical infrastructure globally (Kaewunruen et al., 2016). New ideas and innovation for comprehensive yet installation-specific approaches are necessary to secure the integrity of existing or future, public or private, connected and interdependent assets, installations, and infrastructure systems.
This Research Topic on “Data Science in Transportation and Transit Systems” enables transparent, fair, rapid communication of research that highlights the role of big data, data sciences, artificial intelligence, and engineering in multidisciplinary areas across materials science, physics, and engineering. Emphasis is on the impact, depth, and originality of new concepts, methods, and observations at the forefront of applied sciences and engineering technologies. This topic will help us to achieve our carbon neutrality roadmap (as illustrated in Figure 1) by deploying specialist technology to create a digital twin of the infrastructure system to identify the optimal pathway to net zero, which will be achieved by combining digital sensor and analytic technologies, artificial intelligence, environmental and energy footprints, and concepts that help change users’ behaviour to transform transportation and transit systems.
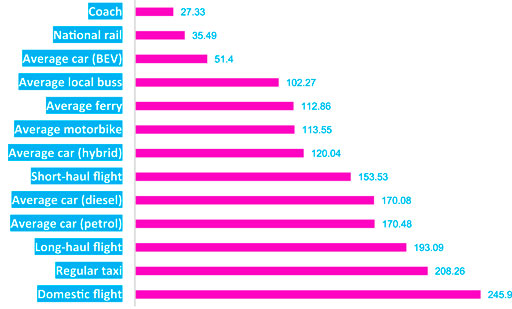
FIGURE 1. Carbon footprint of travel per kilometer in the UK 2022, by transportation mode (in grams of carbon dioxide equivalent per kilometer). (source: https://www.statista.com/statistics/826799/carbon-emission-sources-united-kingdom-uk/).
Along this line, Kaewunruen et al. discuss and assess the reliability of mast structures as a part of ensuring structural safety. The mast structure is a critical aspect of the overhead line electrification equipment (OHLE) used in railway systems. This structure is relatively important as the failure of structure could lead to the failure of an electric system that supplies the power to the trains. This study then analyses the reliability index of the mast, stay tube, and bracket tube structures. The insights guide the structural safety criteria of the railway mast structure and improve the standard design to mitigate the risks and unplanned maintenance due to the uncertainties.
Borjigin et al. share a new development of digital twins capable of assessing the whole life cycle performance of Precast Advanced Track (PCAT) light rail systems in a virtual environment, which is timesaving, flexible, and highly accurate. In this study, two sustainability aspects of the system are addressed: environmental and economic impacts. The study demonstrates a new finding that unrecyclable materials such as bitumen and grout account for a large portion of lifecycle CO2 emissions and costs. It also recommends a re-design of a new track slab system with less grout material and lesser perturbation to formation, which can yield positive structural changes that can minimize carbon impacts throughout the life cycle.
Kaewsawasvong et al. highlight an artificial neural network (ANN) model capable of predicting the stability of rectangular tunnels in rock masses based on the Hoek–Brown (HB) failure criterion. The optimal ANN models provide great accuracy in predicting the stability of the rectangular tunnels based on the HB failure criterion. The application to assess the safety and stability of tunnels has been demonstrated in the study. It clearly demonstrates the capability of ANN for limit analyses of tunnel construction.
Huang and Kaewunruen present a novel machine learning model for real time assessment of passenger ride comfort on trains. The method complies with ISO 2631 and UIC 513, which are based on body vibrations in three dimensions. The study proposes the use of smart phones as crowdsourcing sensors (Azzoug and Kaewunruen, 2017). The novel machine learning model can also determine the jerking rate and the rates of change in both superelevation and cant deficiency. Elizabeth Line has been selected to demonstrate the advanced capability of the machine learning model. This study has clearly demonstrated the robustness of the machine learning model for applications to both surface and underground trains. By data assimilation and automated retraining, the artificial intelligence model can fully address and determine the ride quality considering both ride comfort and rolling motions.
These papers provide exciting new insights and state-of-the-art knowledge for actions towards intelligent transportation and transit systems. The topic editors are grateful to the review editors and associated editors.
Author contributions
All authors listed have made a substantial, direct, and intellectual contribution to the work and approved it for publication.
Conflict of interest
The authors declare that the research was conducted in the absence of any commercial or financial relationships that could be construed as a potential conflict of interest.
Publisher’s note
All claims expressed in this article are solely those of the authors and do not necessarily represent those of their affiliated organizations, or those of the publisher, the editors and the reviewers. Any product that may be evaluated in this article, or claim that may be made by its manufacturer, is not guaranteed or endorsed by the publisher.
References
Azzoug, A., and Kaewunruen, S. (2017). RideComfort: A development of crowdsourcing smartphones in measuring train ride quality. Front. Built Environ. 3, 3. doi:10.3389/fbuil.2017.00003
Keywords: data science, transportation, transit system, decarbonisation, digital transformation (DT)
Citation: Ngamkhanong C, Huynh N, Kassa E, Tsunashima H and Kaewunruen S (2022) Editorial: Data sciences in transportation and transit systems. Front. Built Environ. 8:1066433. doi: 10.3389/fbuil.2022.1066433
Received: 10 October 2022; Accepted: 18 October 2022;
Published: 28 October 2022.
Edited and reviewed by:
Panagiotis Ch. Anastasopoulos, University at Buffalo, United StatesCopyright © 2022 Ngamkhanong, Huynh, Kassa, Tsunashima and Kaewunruen. This is an open-access article distributed under the terms of the Creative Commons Attribution License (CC BY). The use, distribution or reproduction in other forums is permitted, provided the original author(s) and the copyright owner(s) are credited and that the original publication in this journal is cited, in accordance with accepted academic practice. No use, distribution or reproduction is permitted which does not comply with these terms.
*Correspondence: Sakdirat Kaewunruen, s.kaewunruen@bham.ac.uk