- 1Center for Global Health, Division of Infectious Diseases, School of Medicine, University of New Mexico, Albuquerque, NM, United States
- 2National Mastitis Center, Kimron Veterinary Institute, Bet Dagan, Israel
- 3Environmental Assessment, U.S. Environmental Protection Agency, Seattle, WA, United States
- 4Department of Fisheries and Wildlife, Michigan State University, East Lansing, MI, United States
- 5Human Ecology, Centro de Investigación y de Estudios Avanzados (CINVESTAV), Mérida, México
- 6Department of Internal Medicine, School of Medicine, University of New Mexico, Albuquerque, NM, United States
- 7Department of Biopathology and Clinical Microbiology, Aeginition Hospital, Medical School, National and Kapodistrian University of Athens, Athens, Greece
- 8Department of Nursing, Faculty of Human Movement and Quality of Life Sciences, University of Peloponnese, Sparta, Greece
- 9Department of Veterinary Medicine, University of Milan, Milan, Italy
- 10Department of Computer Science, University of Cyprus, Nicosia, Cyprus
- 11Department of Biological Sciences, University of Cyprus, Nicosia, Cyprus
- 12Los Alamos National Laboratory, Biosecurity and Public Health, Los Alamos, NM, United States
- 13School of Biotechnology, Centre National de la Recherche Scientifique (CNRS), University of Strasbourg, Strasbourg, France
Evolution has conserved “economic” systems that perform many functions, faster or better, with less. For example, three to five leukocyte types protect from thousands of pathogens. To achieve so much with so little, biological systems combine their limited elements, creating complex structures. Yet, the prevalent research paradigm is reductionist. Focusing on infectious diseases, reductionist and non-reductionist views are here described. The literature indicates that reductionism is associated with information loss and errors, while non-reductionist operations can extract more information from the same data. When designed to capture one-to-many/many-to-one interactions—including the use of arrows that connect pairs of consecutive observations—non-reductionist (spatial–temporal) constructs eliminate data variability from all dimensions, except along one line, while arrows describe the directionality of temporal changes that occur along the line. To validate the patterns detected by non-reductionist operations, reductionist procedures are needed. Integrated (non-reductionist and reductionist) methods can (i) distinguish data subsets that differ immunologically and statistically; (ii) differentiate false-negative from -positive errors; (iii) discriminate disease stages; (iv) capture in vivo, multilevel interactions that consider the patient, the microbe, and antibiotic-mediated responses; and (v) assess dynamics. Integrated methods provide repeatable and biologically interpretable information.
Reductionism and Its Historical Background
Numerous calls have asked for new methods applicable to infectious disease research. They are motivated by: (i) insufficient information on host-microbial interactions; (ii) obsolete microbial classifications—including “pathogenic” and “non-pathogenic” species; (iii) the need to distinguish “infectiveness” from “virulence”; (iv) the apparent end of the antibiotic era; and (v) requests for more reliable medical diagnoses than those based on research involving a single factor (1–12). Hoping to foster biologically grounded methods, this mini review describes the properties of infectious disease-related data, as well as reductionism—the belief that biology can be reduced to few and simple variables.
Three types of reductionism (ontological, epistemological, and methodological) have been described (13). While the first two types involve abstract (non-measurable) concepts, reductionist methods utilize concrete (measurable) operations. Because conceptualizations precede operationalizations, invalid concepts may promote invalid methods. Therefore, the validity of methods already applied or expected to be used in infectious diseases, in the future, should be determined.
Biology has adopted methods used in Physics (14, 15). Most notably, reductionist approaches have been followed in the field of molecular biology (12, 15–17). While such a fact should not be construed to imply that physics is reductionist per se, the opposite can be emphasized: in contrast to many physical systems, biological systems are generally complex, requiring approaches that far exceed the study of isolated component parts (18).
While biological reductionism has been successful, it has also been associated with failure and cognitive stagnation (12). For instance, after 20,000 publications on sepsis, only one new drug has been legally approved (1, 19). At least two facts suggest that reductionism has hampered vaccine development: (i) more than a thousand synthetic peptide vaccines have been generated but none has been approved, and (ii) reverse vaccinology has not yet produced effective HIV vaccines (10, 13, 17). The high percentage (up to 42%) of research funding reported to be wasted may be due to inadequate methods, which include reductionism (15, 20).
Reductionism has prevailed since Descartes published “The discourse on the method” (15). It is based on deductions, as when Halley predicted, in 1705, that a comet would be seen in 1758 (21). In contrast, Biology thrives on inductions made after data are collected (17).
While Descartes has been viewed as the founder of reductionism (Movie S1 in Supplementary Material), that is not what he proposed: in 1637, he described four rules, reductionism being only the second rule of a method that also included (i) data analysis (first rule), (ii) integration (the third rule), and (iii) comprehensive assessments (the fourth rule). Descartes’ third and fourth rules have not yet been applied in Biology (22).
Two centuries later, Claude Bernard championed biomedically grounded methods (23). He proposed to study the internal milieu—today known as homeostasis or feedback processes. Later, von Bertalanffy showed that biological systems are not closed, but open (24). Thus, “internal” and “external” factors—e.g., host–microbial interactions—should be investigated.
Reductionism-Related Errors and Information Loss
The difference between immunogenicity and antigenicity illustrates why reductionism, in Biology, is failure prone (17). Antigenicity is simply the chemical capacity of a protein (e.g., a viral protein) to bind some preexisting antibodies. In contrast, immunogenicity is the in vivo capacity of the immune system to respond against an immunogen (e.g., a viral antigen) when it is introduced into an animal with the purpose of producing antibodies directed against the antigen. While the complex immune system elicits poly-reactive antibodies that recognize numerous antigens, only some antibodies may neutralize the infectivity of the pathogen (25).
The previous concepts explain why reductionist attempts to design vaccine immunogens by molecular engineering usually fail (17). Two errors explain such failures: (i) because the neutralization capacity of a polyclonal antiserum depends on many and different antibodies, outcomes cannot be predicted from the structure of any one antibody; and (ii) because in vivo interactions involve the pathogen, antibodies, and some but not all host cells, outcomes depend on multifactor, in vivo relationships, which are not considered by synthetic approaches (26–31).
Reductionism is unintentionally practiced in many fields. For example, computer sciences are influenced by the “curse of dimensionality”—a term that refers to the large number of calculations that computers may need to perform (32). To avoid millions of calculations, the number of dimensions to be analyzed may be reduced (33). Fields that reduce dimensions lose valuable information, e.g., in epidemiology, controlled trials do not assess comorbidities, even though they play major roles in infectious diseases (34–37).
Some quantitative traditions also limit the analysis of host–microbial interactions, e.g., correlation analysis neither explains nor predicts (38). Network analysis (a static method) cannot capture dynamics (39). While classic statistics assume linearity, independence, and also regard as constant the meaning of any numerical assessment, these beliefs do not apply to immunomicrobial data: leukocytes are neither linearly distributed nor independent, and numbers derived from immune cells may have different interpretations at different times. That is, leukocyte data can be non-informative or ambiguous (40, 41).
Errors also happen due to inadequate procedures—such as those commonly used with “compositional” data (e.g., leukocyte percentages). Because the same ratio value may be found in different biological conditions, simple leukocyte ratios induce ambiguity (42–44). Errors are also generated by dichotomization: when a cutoff divides continuous data (e.g., leukocyte percentages) into two subsets and discontinuous labels—e.g., “infection-negative” and “-positive”—are assigned to each subset, false-positive and -negative errors invariably occur (45).
Toward Remedial Strategies (I): The Properties of Infectious Disease-Related Data
Infectious disease-related data reveal, at least, four properties: (i) circularity, (ii) heterogeneous temporal scales, (iii) ambiguity, and (iv) hidden structures (41, 43, 44). Understanding their features or consequences may prevent errors and information loss.
Data circularity is detected when three-dimensional (3D) interactions are explored—which become four-dimensional (4D) when time is also measured (43). The analysis of dynamics matters because what has occurred in the past will—or may—be repeated in the future (46). Because the circularity of temporal data shows neither beginning nor end, dynamics cannot be studied with approaches that utilize confidence intervals (43, 45, 47).
Because some processes occur within minutes or hours (e.g., early antimicrobial responses), while other responses—e.g., healing—take place over days or weeks (48, 49), the use of identical chronological units promotes information loss: any one unit may be too large or too small to detect all immune functions. To capture heterogeneous temporal scales, “biological” (not chronological) units may be needed. Two examples of “biological” units include: (i) the increased neutrophil values that characterize early inflammatory responses (expressed as higher neutrophil/lymphocyte [N/L] ratio values), and (ii) the augmented mononuclear cell/neutrophil [MC/N] values (typically observed in the resolution phase). Such well-conserved immune profiles could act as the biological equivalents of “early and late hours” (43).
Ambiguity results when the same numerical value of the same variable is found in different biological conditions (41). Also known as spatial relativity, it occurs when data collected over short time frames (e.g., 1 day before and 1 day after a new infection develops) occupy a large portion of the space under analysis, and vice versa (50).
Because, in 3D/4D space, the number of data combinations may approach infinity, some data structures may be “compressed”, i.e., unobservable (51, 52). Hence, hidden information is a common consequence of the combinatorial properties that characterize Biology.
Toward Remedial Strategies (II): Methodological Foundations
Three traditions facilitate method development: (i) those grounded on theory, (ii) methods expressed with a mathematical language (“modeling”), and (iii) approaches that do not consider theories or models, but “mechanistic” (i.e., limited) explanations (14). Thus, methods that capture a major biological theory in their operations can be more explanatory than alternatives.
Accordingly, methods centered on “organizing principles” have been proposed (12, 53). New methods could capture critical (system-level) biological properties—not features derived from convenience or borrowed from other fields—e.g.: (i) “one-to-many/many-to-one” combinatorial features (39), (ii) complexity (15, 16), and (iii) three-/four-dimensional dynamics (41, 44). These properties are not necessarily different: they may express the same phenomena.
The “one-to-many/many-to-one” feature has two presentations: (i) any one element (e.g., a cell type) can participate in two or more functions, and (ii) to be performed, any one function requires two or more elements. For instance, macrophages promote or destroy neutrophils and, together with lymphocytes, conduct complex functions—for instance, antigen activation (54).
While complexity may be indefinable and defy human understanding (52, 55, 56), four features describe it: (i) emergence, (ii) irreducibility, (iii) unpredictability, and (iv) autonomy. Autonomy means non-linearity: effects are not proportional or linear (57). Emergent features—e.g., those of virulence—are observed when a highly complex structure is assembled (58). Emergence (distinct, non-random patterns) may be detected using dimensionless numbers derived from leukocyte data, which create complex (although hypothetical) data structures (52, 59). Because emergence can neither be reduced to, nor predicted from isolated variables, to detect it, “top-down” (not only “bottom-up”) approaches are needed (12, 13, 16, 58).
While reductionism measures variables in isolation, combinatorial (non-reductionist) approaches capture spatial–temporal relationships. Distinct patterns emerge when, in 3D/4D space, dimensionless indicators converge, not when a single variable changes (60, 61). Because multidimensional pattern recognition does not require numerical cut-offs, it prevents errors associated with dichotomization, “compositional” data, circularity, and ambiguity (39, 40, 44).
While “organizing properties” are necessary, they are not sufficient to prevent two problems: (i) data variability and (ii) the multiple scales of temporal data (48). Both problems may be addressed with structures that reveal a single (one data point-wide) line of observations. Such structures eliminate variability from all dimensions—except along the line—and detect temporal changes that occur along the line, even when such changes are numerically small and/or the individuals being tested include “slow” and “fast” responders (41, 51).
Visualization of Reductionist and Non-Reductionist Paradigms
Figure 1 outlines both reductionist and non-reductionist paradigms. It shows how combinations of few elements (cell types) can create numerous structures. Discrimination depends on pattern recognition—which, in turn, depends on complexity, i.e., the more spatial–temporal relationships captured, the higher the chances of differentiating data subsets. These concepts are explained with a mundane example: written language. While any “letter”, alone, lacks information (the left side of Figure 1), combinations of increasing complexity (“words”, “sentences”, “paragraphs”, “books”) possess meaning. When distinct spatial patterns emerge—such as the two perpendicular subsets exhibited by the upper 3D plot of Figure 1—and temporal data are considered (the lower plot shown on the right side of Figure 1, which displays 4D information), inferences can be based on arrows (spatial–temporal data directionality). While some inferences are visually obvious (Figures 2A,B), not all 4D patterns are interpretable, e.g., the use of the three basic “words” (M–L, N–L, and M–N interactions, expressed as ratios) cannot distinguish dynamics that take place within 2 weeks (Figure 2C). Yet, the spatial–temporal patterns shown in Figures 1 and 2A,B support the detection of, at least, six immunological data subsets (Figure 2D).
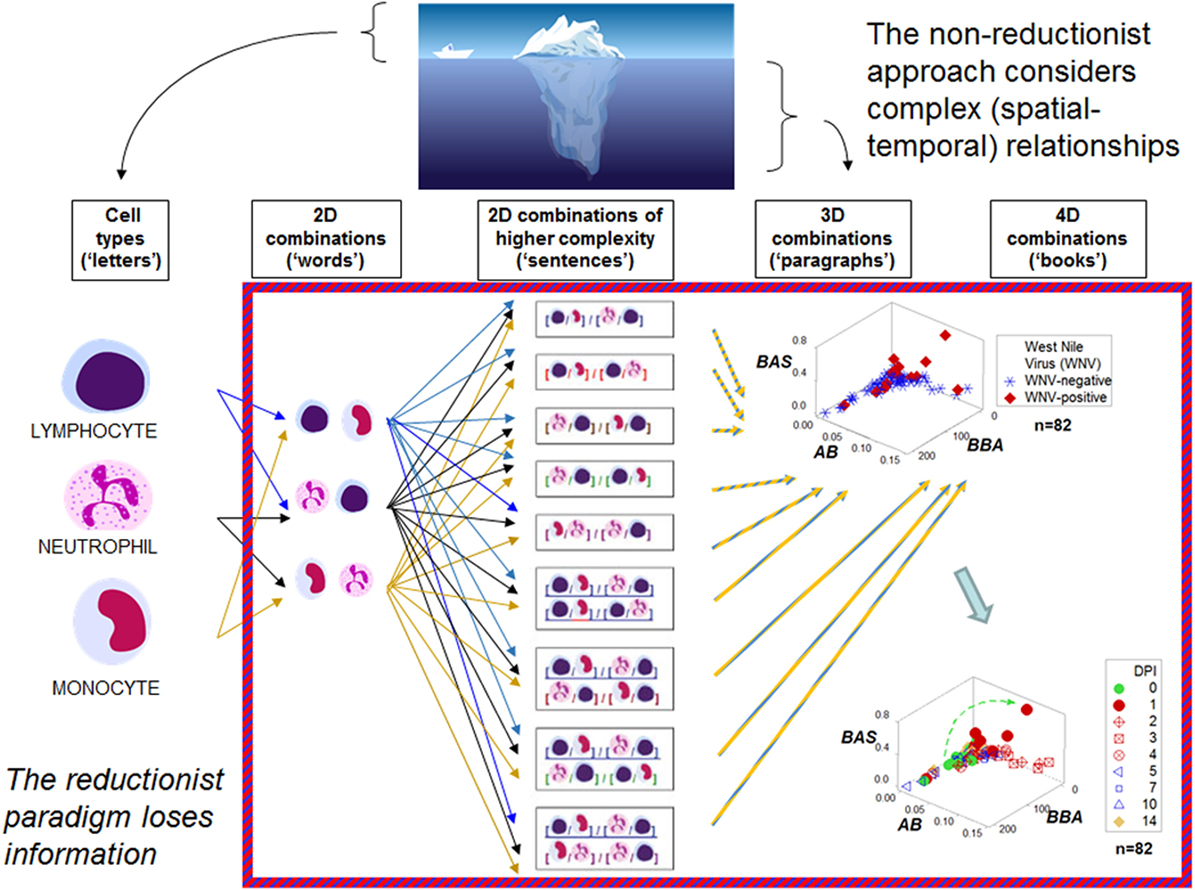
Figure 1. Reductionist and non-reductionist views. An iceberg is used to describe (i) reductionism (the “tip of the iceberg”, i.e., an easily measured entity that does not express all the available information), and (ii) non-reductionism (a combinatorial and spatial–temporal analysis of biological complexity and dynamics, i.e., the area “below the surface”). These concepts are illustrated with an analogy that refers to written language. While simple elements (“letters”) lack meaning, combinations of increasing complexity (“words”, “sentences”, “paragraphs”, “books”) exhibit distinct patterns that facilitate the partitioning of the data into subsets. The hypothetical indicators measured in the three-dimensional (3D)/four-dimensional (4D) plots shown on the right side in the figure—a set taken from the large group of dimensionless indicators shown in the central column—are identified with descriptors that lack any known biological meaning: “BAS”, “AB”, and “BBA.” One example of a dimensionless indicator is the result from calculating: [M/L * N/M]/[N/L * L/M] over [M + L/N] * [L + N/M]/[N + M]/L * [M/N]. DPI: day(s) postinoculation with West Nile virus. Data source: Ref. (43).
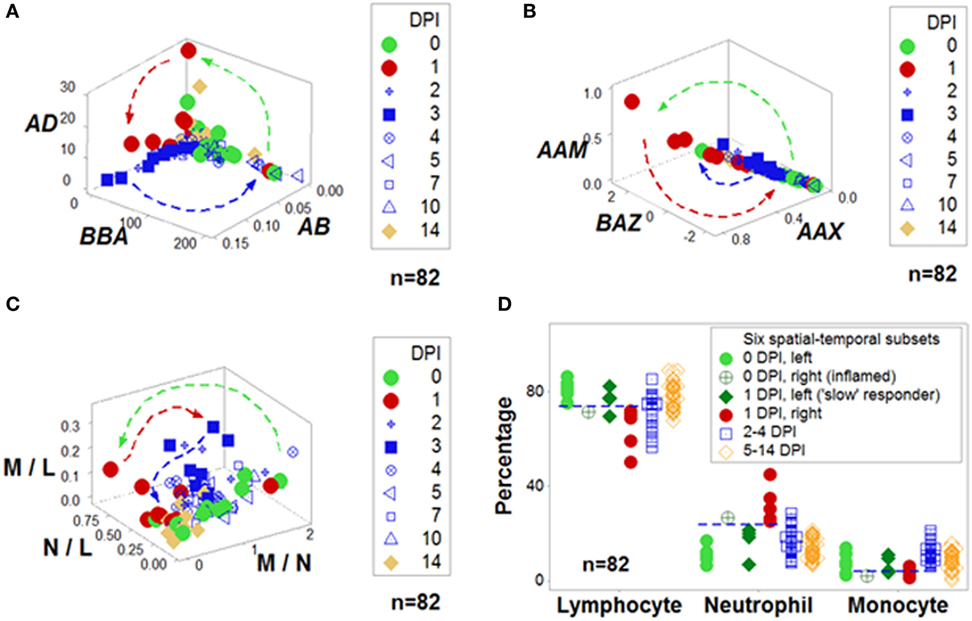
Figure 2. Integration of non-reductionism and reductionism. To both validate and interpret the non-reductionist graphic patterns (described in Figure 1), additional non-reductionist data analyses and reductionist (cell type-based) operations may be required. Highly complex data structures can demonstrate both discrimination and robustness (A,B). In contrast, data structures of lower complexity may fail to distinguish changes that occur within 2 weeks (C). Based on spatial–temporal patterns, numerous data subsets may be identified and interpreted. For instance, in this example, before challenge [0 day(s) postinoculation (DPI)], all birds but one were located on the left side of the plots displayed in Figure 1 [light green circles (D)]. In contrast, 24 h later (at 1 DPI), most challenged birds were on the right side [red symbols (D)]. However, some birds appeared to be “slow” responders: even at 1 DPI, they exhibited the profile of 0 DPI birds [dark green diamonds (D)]. The opposite profile was displayed by one 0 DPI animal, which revealed high neutrophil and low lymphocyte percentages [e.g., a profile indicative of an inflammation not due to the experimental challenge, dark, green circle with inserted cross (D)]. Inferences are facilitated by arrows that denote temporal data directionality (A–C) as well as non-overlapping data distributions [indicated by the horizontal lines (D)]. Because most data combinations have identical contents—except the three “words” [L and M, N and M, and L and N, shown in (C)], any other combination includes all data points of all three cell types (A,B), information does not depend on data inputs (identical for all but three indicators) but relationships, e.g., three-dimensional/four-dimensional (spatial–temporal) data “shapes”, which can be rapidly validated and analyzed—as shown in the Movie S1 in Supplementary Material. Data source: Ref. (43).
When emergent patterns are observed (which are not detected when reductionist approaches are utilized), one plausible inference is that they express immunological functions not previously recognized. As described in the Movie S1 in Supplementary Material, that hypothesis can be rapidly assessed.
Thus, non-reductionist data structures help discover preexisting functions (propositional knowledge). To validate such propositions, new tools or methods (prescriptive knowledge) may be required to conduct operations previously unfeasible (27, 62, 63).
Non-Reductionist Applications
The postulates described in Figure 1 have been abundantly demonstrated (41, 44, 51, 52, 64). As shown in Figure 2C, data ambiguity may occur when structures of low complexity are used (41).
In contrast, new information emerges when highly complex data structures are utilized (Figures 2A,B; Movie S1 in Supplementary Material). The discriminant process follows the geometric criteria described by Gestalt psychologists 80 years ago, including similarity, proximity, continuity, closure, common fate, parallelism, and symmetry (61).
Non-reductionist, combinatorial approaches can both detect false-negative and -positive errors and differentiate early from late immune stages (51). They also distinguish subsets of septic patients that differ in mortality rates and immunological profiles (44).
Furthermore, non-reductionism can inform on patients empirically treated with antibiotics (41, 44). While reductionist tests do not evaluate antimicrobial potency and only provide in vitro (antimicrobial susceptibility test-based) data (4), non-reductionist methods can provide earlier (within 24 h) and in vivo information on antibiotic–immuno–microbial–temporal interactions (41). Because they may capture emergent (system-level) properties, non-reductionist analyses can yield more reliable results than those based on any one single factor (12).
Non-reductionist approaches can reveal interactions that involve cellular, supra-, and/or subcellular levels. Such approaches can simultaneously assess numerous functions, including (i) leukocyte activation, (ii) diapedesis, (iii) phagocytosis, (iv) early inflammation, and (v) the resolution phase of inflammation (64).
The Future: Integration of Non-Reductionist and Reductionist Operations
The one-to-many/many-to-one “organizing principle” is ubiquitous: all vertebrates are protected from thousands of microbes by up to five leukocyte types. Even if all cell types—estimated to be approximately 210 (18)—performed antimicrobial functions, they could not fend off tens of thousands of microbes should only “one-to-one” (immunomicrobial) relationships exist. Clearly, the reductionist “single structure/single sequence/single function” theory is implausible (65).
In contrast, multilevel functionality seems to be one of Biology’s “first principles” (23, 66). Because it increases the complexity of the data—and, therefore, extracts more information—multilevel functionality may be operationalized by one-to-many/many-to-one constructs (32, 67).
However, non-reductionist approaches may generate artifacts. To validate such methods, reductionist operations—e.g., statistical analyses that focus on individual cell types—may be required (41, 44, 51, 52, 64).
Given the problems associated with reductionist concepts, the previous statement seems contradictory. Yet, it is not: a non-reductionist paradigm (an abstract entity) may be partially implemented by operations (concrete entities) that include reductionist procedures.
Integrated (non-reductionist and reductionist) constructs may improve experimental designs (68). Because experimental reductionism is inherently closed (69), it usually misses valuable information. In contrast, experiments conducted as a double (non-reductionist and reductionist) series of studies could circumvent the limitations of experimental reductionism.
Conclusion
Because some properties of infectious disease-related data may possess undesirable consequences (e.g., data ambiguity prevents discrimination) and, in personalized medicine, decisions should be made even when the number of subjects n = 1, to diagnose and treat infectious diseases what is needed is not more data points (impossible when n = 1) but temporal data of greater complexity. To that end, immune profiles may be considered.
Because their repeatability can be easily determined—just a couple of studies can elucidate whether an observed immune pattern has been conserved across populations or species—immune profile-based inferences can measure emergence, i.e., patterns not shown by simple data structures that do not measure interactions—such as neutrophil percentages or counts—which may be revealed by 4D configurations of greater complexity (41).
A two-step procedure may detect and validate “emergence.” The first step is a non-reductionist, “top-down”, hypothesis-free, combinatorial process that creates numerous and complex indicators with the purpose of generating distinct (non-randomly distributed) data subsets (Figure 1). The second step is a reductionist (cell type-based) description of subsets meant to reveal, partially or totally, non-overlapping leukocyte data distributions which may also differ temporally. This double (spatial and temporal) data partitioning process is likely to be both immunologically interpretable and statistically analyzable (Figure 2D).
Integrated (non-reductionist and reductionist) approaches may fill the gap of the Oslerian bio-medical paradigm—which looks for correlations but does not investigate pathogenesis—and merge disciplines and technologies (30, 70, 71). Complex and dynamic (combinatorial) methods may be more predictable than classic (reductionist or linear) models (72, 73).
Hence, the major message of this review refers to the generation and interpretation of biological information. Because most combinations of immunological data include exactly the same contents (Figures 1 and 2; Movie S1 in Supplementary Material), information does not depend on data inputs but procedures that include non-reductionist and reductionist steps: (i) detection of distinct patterns, followed by (ii) biological validation and statistical analysis of the data subsets identified in the first step.
Author Contributions
Conceived the study: AR. Contributed reagents/materials/data: GL, MJ, MI, SC, AI, SB, RP, and JCF. Wrote the paper: AR, AH, AA, JF, YA, and MR.
Disclaimer
This study does not reflect the official positions and policies of the US EPA. Mention of products/trade names does not constitute recommendation for use by US EPA.
Conflict of Interest Statement
While none of the authors received, at any time, any payment or services from a third party for any aspect of the submitted work, they wish to declare that they used a proprietary algorithm subject to a pending patent.
Acknowledgments
The artwork and video production generated by Nicol Engberts Hoogesteyn, José Luis Febles, and Marinos Panayiotou are very much appreciated.
Funding
This research received no specific grant from any funding agency in the public, commercial, or not-for-profit sectors.
Supplementary Material
The Supplementary Material for this article can be found online at http://journal.frontiersin.org/article/10.3389/fimmu.2017.00612/full#supplementary-material.
Movie S1. Biological reductionism vs. biological complexity.
References
1. Cohen J, Vincent JL, Adhikari NKJ, Machado FR, Angus DC, Calandra T, et al. Sepsis: a roadmap for future research. Lancet Infect Dis (2015) 15:581–614. doi: 10.1016/S1473-3099(15)70112-X
2. Van Regenmortel MHV. Paradigm changes are required in HIV vaccine research. Front Immunol (2015) 6:326. doi:10.3389/fimmu.2015.00326
3. Casadevall A, Pirofski L. Host-pathogen interactions: redefining the basic concepts of virulence and pathogenicity. Infect Immun (1999) 67:3703–13.
4. Anuforom O, Wallace GR, Piddock LV. The immune response and antibacterial therapy. Med Microbiol Immunol (2015) 204:151–9. doi:10.1007/s00430-014-0355-0
5. Casadevall A, Pirofski L. Host-pathogen interactions: the basic concepts of microbial commensalism, colonization, infection, and disease. Infect Immun (2000) 68:6511–8. doi:10.1128/IAI.68.12.6511-6518.2000
6. Casadevall A, Pirofski LA. What is a host? Incorporating the microbiota into the damage-response framework. Infect Immun (2015) 83:2–7. doi:10.1128/IAI.02627-14
7. Pomorska-Mól M, Pejsak Z. Effects of antibiotics on acquired immunity in vivo – current state of knowledge. Pol J Vet Sci (2012) 15:583–9. doi:10.2478/v10181-012-0089-0
8. Pirofski L, Casadevall A. What is infectiveness and how is it involved in infection and immunity? BMC Immunol (2015) 16:13. doi:10.1186/s12865-015-0076-1
9. Wainwright M, Maisch T, Nonell S, Plaetzer K, Almeida A, Tegos GP, et al. Photoantimicrobials – are we afraid of the light? Lancet Infect Dis (2017) 17:e49–55. doi:10.1016/S1473-3099(16)30268-7
10. Esparza J. A new scientific paradigm may be needed to finally develop an HIV vaccine. Front Immunol (2015) 6:124. doi:10.3389/fimmu.2015.00124
11. Van Regenmortel MHV. An outdated notion of antibody specificity is one of the major detrimental assumptions of the structure-based reverse vaccinology paradigm, which prevented it from helping to develop an effective HIV-1 vaccine. Front Immunol (2015) 6:593. doi:10.3389/fimmu.2014.00593
12. Conti F, Valerio MC, Zbilut JP, Giuliani A. Will systems biology offer new holistic paradigms to life sciences? Syst Synth Biol (2007) 1:161–5. doi:10.1007/s11693-008-9016-1
13. Mazzocchi F. Complexity and the reductionism–holism debate in systems biology. Wiley Interdiscip Rev Syst Biol Med (2012) 2012(4):413–27. doi:10.1002/wsbm.1181
14. Ghilarov AM. The changing place of theory in 20th century ecology: from universal laws to array of methodologies. Oikos (2001) 92:357–62. doi:10.1034/j.1600-0706.2001.920218.x
16. Van Regenmortel MHV. Reductionism and complexity in molecular biology. EMBO Rep (2004) 5:1016–20. doi:10.1038/sj.embor.7400284
17. Van Regenmortel MHV. Basic research in HIV vaccinology is hampered by reductionist thinking. Front Immunol (2012) 3:194. doi:10.3389/fimmu.2012.00194
18. Macklem PT, Seely A. Towards a definition of life. Perspect Biol Med (2010) 53:330–40. doi:10.1353/pbm.0.0167
19. Deutschman CS, Tracey KJ. Sepsis: current dogma and new perspectives. Immunity (2014) 40:463–75. doi:10.1016/j.immuni.2014.04.001
20. Yordanov Y, Dechartres A, Porcher R, Boutron I, Altman DG, Ravaud P. Avoidable waste of research related to inadequate methods in clinical trials. BMJ (2015) 350:h809. doi:10.1136/bmj.h809
21. Wallis R. The glory of gravity – Halley comet 1759. Ann Sci (1964) 41:279–86. doi:10.1080/00033798400200271
22. Margineanu DG. Neuropharmacology beyond reductionism – a likely prospect. Biosystems (2016) 141:1–9. doi:10.1016/j.biosystems.2015.11.010
23. Noble D. Claude Bernard, the first systems biologist, and the future of physiology. Exp Physiol (2008) 93:16–26. doi:10.1113/expphysiol.2007.038695
24. von Bertalanffy L. The theory of open systems in physics and biology. Science (1950) 111:23–9. doi:10.1126/science.111.2872.23
25. Van Regenmortel MHV. Specificity, polyspecificity amd heterospecificity of antigen-antibody recognition. J Mol Recognit (2014) 27:627–39. doi:10.1002/jmr.2394
26. Van Regenmortel MHV. Reductionism and the search for structure-function relationships in antibody molecules. J Mol Recognit (2002) 15:240–7. doi:10.1002/jmr.584
27. Van Regenmortel MHV. Structure-based reverse vaccinology failed in the case of HIV because it disregarded accepted immunological theory. Int J Mol Sci (2016) 17:1591. doi:10.3390/ijms17091591
28. Talmage DW. Immunological specificity. Science (1959) 129:1643–8. doi:10.1126/science.129.3364.1643
29. Richards FF, Konigsberg WH. Speculations. How specific are antibodies? Immunochemistry (1973) 10:545–53. doi:10.1016/0019-2791(73)90227-9
30. Schubert W. Systematic, spatial imaging of large multimolecular assemblies and the emerging principles of supramolecular order in biological systems. J Mol Recognit (2014) 27:3–18. doi:10.1002/jmr.2326
31. Berzofsky JA. Intrinsic and extrinsic factors in protein antigenic structure. Science (1985) 229:932–40. doi:10.1126/science.2410982
32. Robson B. The dragon on the gold: myths and realities for data mining in biomedicine and biotechnology using digital and molecular libraries. J Proteome Res (2005) 3:1113–9. doi:10.1021/pr0499242
33. Binder H, Blettner M. Big data in medical science—a biostatistical view. Dtsch Arztebl Int (2015) 112:137–42. doi:10.3238/arztebl.2015.0137
34. Greenhalgh T, Howick J, Maskrey N. Evidence based medicine: a movement in crisis? BMJ (2014) 348:g3725. doi:10.1136/bmj
35. Esper AM, Moss M, Lewis CA, Nisbet R, Mannino DM, Martin GS. The role of infection and comorbidity: factors that influence disparities in sepsis. Crit Care Med (2006) 34:2576–82. doi:10.1097/01.CCM.0000239114.50519.0Eg3725
36. Ronacher K, Joosten SA, van Crevel R, Dockrell HM, Walzl G, Ottenhoff THM. Acquired immunodeficiencies and tuberculosis: focus on HIV/AIDS and diabetes mellitus. Immunol Rev (2015) 264:121–37. doi:10.1111/imr.12257
37. Ford N, Shubber Z, Meintjes G, Grinsztejn B, Eholie S, Mills EJ, et al. Causes of hospital admission among people living with HIV worldwide: a systematic review and meta-analysis. Lancet HIV (2015) 2:e438–44. doi:10.1016/S2352-3018(15)00137-X
39. Tieri P, Grignolio A, Zaikin A, Mishto M, Remondini D, Castellani GC, et al. Network, degeneracy and bow tie. Integrating paradigms and architectures to grasp the complexity of the immune system. Theor Biol Med Model (2010) 7:32. doi:10.1186/1742-4682-7-32
40. Amarasingham A, Geman S, Harrison MT. Ambiguity and nonidentifiability in the statistical analysis of neural codes. Proc Natl Acad Sci U S A (2015) 112:6455–60. doi:10.1073/pnas.1506400112
41. Iandiorio MJ, Fair JM, Chatzipanagiotou S, Ioannidis A, Trikka-Graphakos E, Charalampaki N, et al. Preventing data ambiguity in infectious diseases with four-dimensional, earlier, personalized, in vivo evaluations. PLoS One (2016) 11:e0159001. doi:10.1371/journal.pone.0159001
42. Katz JN, King G. A statistical model for multiparty electoral data. Am Polit Sci Rev (1999) 93:15–32. doi:10.2307/2585758
43. Rivas AL, Jankowski MD, Piccinini R, Leitner G, Schwarz D, Anderson KL, et al. Feedback-based, system-level properties of vertebrate-microbial interactions. PLoS One (2013) 8:e53984. doi:10.1371/journal.pone.0053984
44. Chatzipanagiotou S, Ioannidis A, Trikka-Graphakos E, Charalampaki N, Sereti C, Piccinini R, et al. Detecting the hidden properties of immunological data and predicting the mortality risks of infectious syndromes. Front Immunol (2016) 7:217. doi:10.3389/fimmu.2016.00217
45. Cohen J. The cost of dichotomization. Appl Psychol Meas (1983) 7:249–53. doi:10.1177/014662168300700301
46. Bertuglia CS, Vaio F. Nonlinearity, Chaos and Complexity. Oxford: Oxford University Press (2005).
47. Gill J, Hangartner D. Circular data in political science and how to handle it. Polit Anal (2010) 18:316–36. doi:10.1093/pan/mpq009
48. Qu Z, Garfinkel A, Weiss JN, Nivala M. Multi-scale modeling in biology: how to bridge the gaps between scales? Prog Biophys Mol Biol (2011) 107:21–31. doi:10.1016/j.pbiomolbio.2011.06.004
49. Myers SR, Leigh IM, Navsaria H. Epidermal repair results from activation of follicular and epidermal progenitor keratinocytes mediated by a growth factor cascade. Wound Repair Regen (2007) 15:693–701. doi:10.1111/j.1524-475X.2007.00297.x
50. Auffrey C, Nottale L. Scale relativity theory and integrative systems biology 1. Founding principles and scale laws. Prog Biophys Mol Biol (2008) 97:79–114. doi:10.1016/j.pbiomolbio.2007.09.002
51. Fair JM, Rivas AL. Systems biology and ratio-based, real-time disease surveillance. Transbound Emerg Dis (2015) 62:437–45. doi:10.1111/tbed.12162
52. Leitner G, Blum S, Rivas AL. Visualizing the indefinable: three-dimensional complexity of ‘infectious diseases’. PLoS One (2015) 10:e0123674. doi:10.1371/journal.pone.01236742015
53. Wolkenhauer O, Green S. The search for organizing principles as a cure against reductionism in systems medicine. FEBS J (2013) 280:5938–48. doi:10.1111/febs.12311
54. Knowlton ER, Lepone LM, Li J, Rappocciolo G, Jenkins FJ, Rinaldo CR. Professional antigen presenting cells in human herpesvirus 8 infection. Front Immunol (2013) 3:427. doi:10.3389/fimmu.2012.00427
55. Burggren W, Monticino MG. Assessing physiological complexity. J Exp Biol (2005) 208:3221–32. doi:10.1242/jeb.01762
57. Van Regenmortel MHV. The rational design of biological complexity: a deceptive metaphor. Proteomics (2007) 7:965–75. doi:10.1002/pmic.200600407
58. Casadevall A, Fang FC, Pirofski LA. Microbial virulence as an emergent property: consequences and opportunities. PLoS Pathog (2011) 7:e1002136. doi:10.1371/journal.ppat.1002136
59. Klinke DJ. Validating a dimensionless number for glucose homeostasis in humans. Ann Biomed Eng (2009) 37:1886–96. doi:10.1007/s10439-009-9733-y
60. Osinga HM, Sherman A, Tsaneva-Atanasova K. Cross-currents between biology and mathematics: the codimension of pseudo-plateau bursting. Discrete Contin Dyn Syst Ser A (2012) 32:2853–77. doi:10.3934/dcds.2012.32.2853
61. Andreopoulos A, Tsotsos JK. 50 Years of object recognition: directions forward. Comput Vis Image Underst (2013) 117:827–91. doi:10.1016/j.cviu.2013.04.005
64. Rivas AL, Hoogesteijn AL, Piccinini R. Beyond numbers: the informative patterns of staphylococcal dynamics. Curr Pharm Des (2015) 21:2122–30. doi:10.2174/1381612821666150310104053
65. Uversky VN, Davé V, Iakoucheva LM, Malaney P, Metallo SJ, Pathak RR, et al. Pathological unfoldomics of uncontrolled chaos: intrinsically disordered proteins and human diseases. Chem Rev (2014) 114:6844–79. doi:10.1021/cr400713r
66. Noble D. Biophysics and systems biology. Phil Trans R Soc A (2010) 368:1125–39. doi:10.1098/rsta.2009.0245
67. Cedersund G, Roll J. Systems biology: model based evaluation and comparison of potential explanations for given biological data. FEBS J (2009) 276:903–22. doi:10.1111/j.1742-4658.2008.06845.x
68. Roberts AEL, Kragh KN, Bjarnsholt T, Diggle SP. The limitations of in vitro experimentation in understanding biofilms and chronic infection. J Mol Biol (2015) 427:3646–61. doi:10.1016/j.jmb.2015.09.002
69. Pilgrim D. The biopsychosocial model in health research: its strengths and limitations for critical realists. J Crit Realism (2015) 14:164–80. doi:10.1179/1572513814Y.0000000007
70. Loscalzo J, Barabasi AL. Systems biology and the future of medicine. Wiley Interdiscip Rev Syst Biol Med (2011) 3:619–27. doi:10.1002/wsbm.144
71. Panagi M, Georgila K, Eliopoulos AG, Apidianakis Y. Constructing personalized longitudinal holo’omes of colon cancer-prone humans and their modeling in flies and mice. Oncotarget (2015). doi:10.18632/oncotarget.6463
72. Skinner JE. Low-dimensional chaos in biological systems. Biotechnology (1994) 12:596–600. doi:10.1038/nbt0694-596
Keywords: methods, host–microbe interactions, reductionism, non-reductionism, pattern recognition
Citation: Rivas AL, Leitner G, Jankowski MD, Hoogesteijn AL, Iandiorio MJ, Chatzipanagiotou S, Ioannidis A, Blum SE, Piccinini R, Antoniades A, Fazio JC, Apidianakis Y, Fair JM and Van Regenmortel MHV (2017) Nature and Consequences of Biological Reductionism for the Immunological Study of Infectious Diseases. Front. Immunol. 8:612. doi: 10.3389/fimmu.2017.00612
Received: 29 March 2017; Accepted: 09 May 2017;
Published: 31 May 2017
Edited by:
Juarez Antonio Simões Quaresma, Federal University of Pará, BrazilReviewed by:
Ignazio Licata, Institute for Scientific Methodology (ISEM), ItalyAlbert Descoteaux, Institut national de la recherche scientifique, Canada
Sven Braeutigam, University of Oxford, United Kingdom
Copyright: © 2017 Rivas, Leitner, Jankowski, Hoogesteijn, Iandiorio, Chatzipanagiotou, Ioannidis, Blum, Piccinini, Antoniades, Fazio, Apidianakis, Fair and Van Regenmortel. This is an open-access article distributed under the terms of the Creative Commons Attribution License (CC BY). The use, distribution or reproduction in other forums is permitted, provided the original author(s) or licensor are credited and that the original publication in this journal is cited, in accordance with accepted academic practice. No use, distribution or reproduction is permitted which does not comply with these terms.
*Correspondence: Ariel L. Rivas, alrivas@unm.edu