The Use of Mobile Near-Infrared Spectroscopy for Real-Time Pasture Management
- School of Biosciences, The University of Nottingham, Sutton Bonington, Loughborough, United Kingdom
Changes in pasture nutrients over the growing season are typically not monitored but doing so may help farmers improve how effectively they utilize forage. The aim of this research was to assess the use of real-time near-infrared spectroscopy (NIRS) for monitoring seasonal changes in nutrient concentrations of different pasture types used for grazing and silage production. Three permanent pastures and three temporary ley pastures (3 years old) grazed by cattle or sheep and/or used for silage production were monitored weekly for 20 weeks from April to August 2017 in the UK. Five pasture samples per field were obtained per week for NIRS analysis and estimation of fresh and dry matter herbage cover (both kg per hectare). Herbage height was also measured each week. Permanent pastures included a diverse range of native UK grass species, and temporary ley pastures were predominantly perennial ryegrass (Lolium perenne) with either white (Trifolium repens) or red clover (Trifolium pretense). Effects of pasture type (permanent or temporary), phase of production (grazed or rested for regrowth) and month of year (April to August) on pasture nutrients [dry matter, crude protein, acid detergent fiber (ADF), neutral detergent fiber (NDF), water soluble carbohydrate (WSC), ash, digestible organic matter (DOMD), and dry matter digestibility (DMD)] were assessed by fitting a linear mixed model. Considerable variation was observed in pasture production and in the concentrations of dry matter, crude protein and WSC in pastures. This study suggests that grazing pastures to a mean height of below 7 cm results in a significantly reduced concentration of crude protein, DOMD, and DMD, which may be detrimental to the grass intake and protein intake of the grazing animal. The DOMD and DMD of pasture were positively correlated with herbage height and herbage cover crude protein concentration. An approach of real-time nutrient monitoring will facilitate more timely adaptive pasture management than currently feasible for farmers. This should lead to productivity gain.
Introduction
Grassland is the dominant agricultural land use type in the UK, covering 12.3 million ha (66% of total agricultural area). The UK grassland area can be subdivided into 1.4 million ha of temporary grassland, 5.8 million ha of permanent pasture, and 5.1 million ha of rough grazing (Defra, 2018). The diversity of grasslands and their spatial arrangement within a farm, and the landscape, have major importance for the sustainability of the environment and ruminant livestock systems (Gibon, 2005). Grass provides a cheap and affordable source of nutrients for ruminants, with pasture providing approximately 70% of the 42 million tons of forage dry matter consumed by ruminants (Wilkinson, 2011). Furthermore, feed costs can represent as much as 70% of the variable costs of livestock production (Redman, 2016), and therefore ways to manage forage efficiently will enhance the use of this valuable resource. Grazed pasture can supply over half of the protein and energy needed by ruminants (Waghorn and Clark, 2004; Hopkins and Wilkins, 2006). Understanding seasonal changes in pasture nutrient concentrations can enhance ruminant production systems and management. The poor matching of nutrient supply with animal requirements can reduce livestock performance (Dillon, 2006), increase the demand for land and reduce nutrient use efficiency. Timely information on supply and nutrient concentrations of pasture, and its associated variability, will allow farmers to better match nutrient supply with animal demand.
Tools that measure herbage height and cover have been used for a long time, such as a rising plate meter or cut and weigh methods (French et al., 2015). The value of pasture is a combination of not only pasture production but its nutrient quality. The maximizing of forage utilization in ruminant production systems is associated with reduced operating costs, such as inputs of supplementary feed as mentioned above (Dillon, 2006; Ramsbottom et al., 2015). In recent years the principal method of wet chemistry analysis in the laboratory for obtaining nutrient levels in forage has been replaced by NIRS analysis (Stergiadis et al., 2015), which is calibrated using wet chemistry data. The NIRS technique measures the spectrum of infrared energy reflected from a sample illuminated by white light. This approach to estimating nutrient levels in products has reduced the time taken for analysis (from about 16 h to less than a minute) and its cost (Stuth et al., 2003). Even with this quicker and lower cost approach, forage analysis is often done infrequently by farmers. Developments in NIRS devices that are smaller andmore mobile (Malley et al., 2005; Pullanagari et al., 2012) with data processing and storage, now allow real-time analysis on farms. The benefit of this mobile approach is that the technology allows frequent nutrient analysis and timelier decision making at a lower cost to sending samples for laboratory analysis, which should encourage more adoption on farms.
The objective of this study was to assess the use of real-time NIRS for monitoring seasonal changes in nutrient concentrations of different pasture types used for grazing and silage production. During this study, three permanent pastures and three temporary ley pastures were monitored during spring and summer months in the UK.
Materials and Methods
Field Data
The study was carried out at the University of Nottingham farm at Sutton Bonington over a 20-week period from April to August 2017. During the study, the lowest amount of rainfall (13 mm) and lowest average daily temperatures (9°C) were in April, and highest amount of rainfall (90 mm) and highest average daily temperatures (17°C) in July (17°C; Figure 1). During the study the average daily temperatures in May and June were noticeably higher than the average for the same months during the previous 10 years from 2007 to 2016. Total rainfall was also noticeably lower in April and higher in July during the study compared to the previous 10 years.
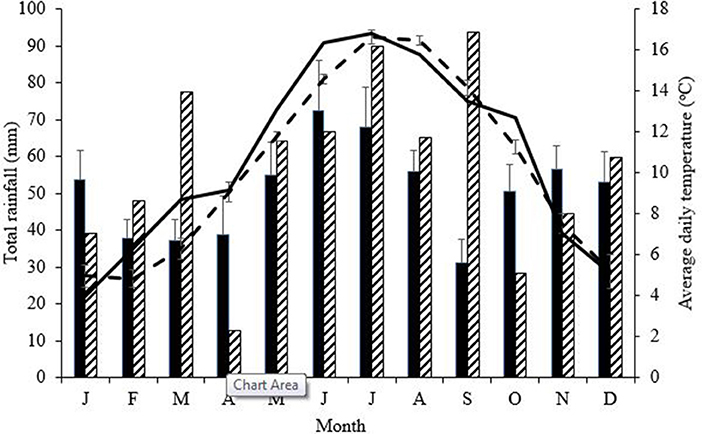
Figure 1. Total rainfall and average daily temperatures for the months January to December during the years 2007 to 2016 (solid column and line respectively) and during the study year of 2017 (dashed column and line respectively). Standard error bars are ahown for months during years 2007 to 2016.
Grassland at the farm consisted of permanent and temporary ley pastures used for cattle or sheep grazing and/or silage production. Six fields were selected for this study, with three being permanent pastures and three being temporary ley pastures. The permanent pastures (Fields A, B and C; Table 1) have never been cultivated and contain a diverse botanical composition of native UK grass species of perennial ryegrass (Lolium perenne), timothy (Phleum pretense), Yorkshire fog (Holcus lanatus), cocksfoot (Dactylis glomerata), common bent (Agrostis capillaris), and meadow grass (Poa annua). The temporary ley pastures were part of a crop rotation and are cultivated after 3 to 5 years of production. The temporary leys consisted of predominantly perennial ryegrass, with either white clover (Trifolium repens, Fields D and F; Table 1) or red clover (Trifolium pretense, Field E).
Prior to commencement of the study all six fields were rested for four or more weeks, as prior to this fields were intermittently grazed by sheep over the winter months. During this period the temporary pasture fields of D, E and F received an application of inorganic fertilizer, and fields A and B received dirty water, which is produced after removing solid organic material from cattle slurry (the amount of nutrient inputs are shown in Table 1). Field C didn't have any nutrients applied during the study. Fields D, E and F had dirty water applied after each harvest of silage, and during this week no grass measurements were taken. During the study, ewes and their lambs intermittently grazed fields C and F and dairy heifers grazed fields A and B. Management of each field during the weeks of the study are shown in Table 2, with periods highlighted when pasture is rested for regrowth, grazed and harvested for silage.
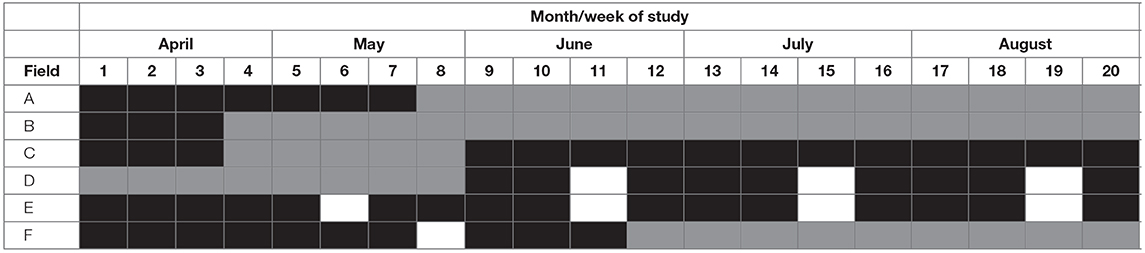
Table 2. Illustration showing periods of pasture regrowth (black), grazing (gray), and silage harvest (white) for each field (A to F) during the 20 weeks of the study.
Measurements
The herbage height, fresh and dry matter herbage cover and nutrient concentrations of each field were measured during the study. No grass measurements were taken during weeks when silage was harvested and dirty water was applied, as the application of dirty water prevented accurate NIRS analysis of nutrient concentrations. Grass measurements avoided dung, urine and dense weed patches when taken.
Pasture Sampling
Pasture measurements were conducted on the same day each week. In each field 5 grass samples were cut to ground level and within a 36 cm diameter wire ring (0.1 m2) randomly placed on the ground. To ensure representative coverage of each field, the 5 grass samples were taken in a W-pattern (Wilkinson et al., 2014). The total weight (grams) of pasture within the ring was multiplied by 100 (i.e., 1 hectare = 10,000 m2) to estimate the fresh herbage cover (kg fresh weight/hectare). The fresh pasture cover value was then multiplied by the percentage of dry matter measured by NIRS to derive the dry matter herbage cover (kg DM/hectare).
A rising plate meter (F400; Farmworks Precision Farming Systems Ltd, Feilding, NZ) was used to measure sward height (Figure 2). The pasture height of each field was estimated from the average of 30 “spot” measurements taken in a W-pattern across the field.
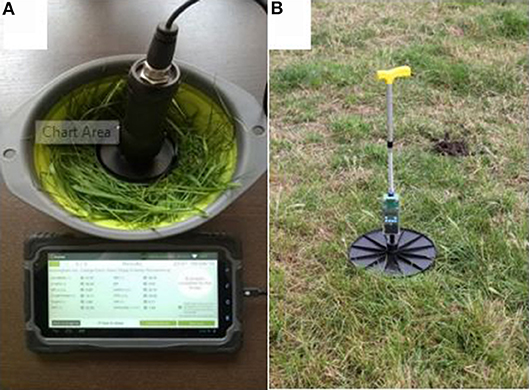
Figure 2. Pasture measurement devices of (A) Tablet near-infrared spectroscopy scanner and tablet for nutrient analysis and (B) rising plate meter for herbage height.
Nutrient Analysis
A mobile NIRS device (NIR4; Aunir, Towcester, UK) was used to scan cut pasture samples for their nutrient concentrations (Figure 2). The NIR4 takes four replicate scans, consisting of a spectrum of infrared energy reflected from the pasture sample illuminated by the scanner, from which nutrient concentrations are estimated from the average of the four scans. The scan results are uploaded to a tablet and secure server for further analysis. The nutrient concentrations measured were: dry matter, crude protein, acid detergent fiber (ADF), neutral detergent fiber (NDF), water soluble carbohydrate (WSC), ash, digestible organic matter (DOMD), and dry matter digestibility (DMD; all expressed as grams per kilogram of dry matter).
Statistical Analysis
The mean value for the 5 weekly measurements of herbage cover and nutrient concentrations per field was used for further analysis. This produced a total of 112 weekly records for all fields (20 weeks × 6 fields with the 8 weekly values when silage was harvested not included). Data were analyzed using a linear mixed model in Genstat software (version 18.1; VSN International Ltd., Hemel Hempstead, UK). Equation (1) was used to assess the effect of pasture type (permanent or temporary), phase of production (grazed or rested for regrowth) and month of year (April to August) on pasture nutrients:
where yijkl is the dependent variable; μ = overall mean; Mi = fixed effect of month of year (i = April to August); Pj = fixed effect of pasture type (j = permanent or temporary); Sk = fixed effect of phase of production (k = grazed or regrowth); Fl = fixed effect of field (l = A to F); eijkl = random error term.
Equation (1) was also used to assess differences in fresh pasture cover, dry matter cover and herbage height without the fixed effect of field. Predicted means for pasture type (permanent or temporary), phase of production (grazed or rested for regrowth), month of year (April to August) and field (A to F; for analysis of nutrient concentrations) and pasture type × phase of production were presented. The interaction between pasture type × phase of production and month of year are not presented since influenced by field management (i.e., grazing, silage cut or regrowth; Table 2). Pearson correlation coefficient (r) was used to test the association between herbage height, herbage cover (fresh and dry matter per hectare) and pasture nutrient concentrations. Significant differences were attributed at P < 0.05.
Results
Differences in Pasture Height, Herbage Cover, and Pasture Nutrients
There was considerable variation in herbage height (coefficient of variation = 46%), and fresh and dry matter herbage cover (coefficient of variation = 78 and 71%, respectively) across fields studied (Table 3). For pasture nutrients, there was more variability in measured dry matter, crude protein and WSC concentrations in pasture (coefficient of variation ranging from 18 to 23%) than observed for NDF, DOMD and DMD concentrations (coefficient of variation ranging from 2 to 5%).
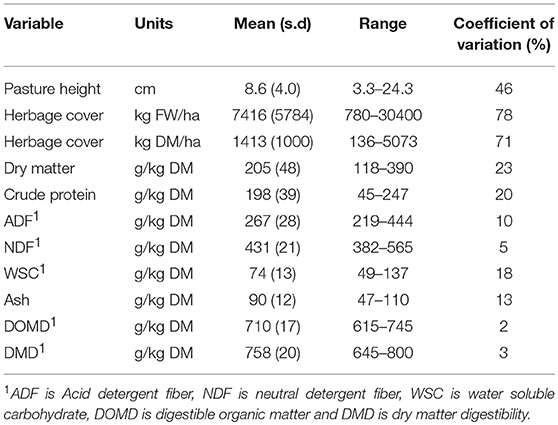
Table 3. Average pasture height, herbage cover, and nutrient concentrations across fields, and weeks of the study.
On average, herbage height and cover (fresh and dry matter) were lower during periods of grazing (all P < 0.001) and declined from April/May to June/July/August (P < 0.05 or greater; Table 4). An interaction was found between temporary ley pastures that were grazed, with lower herbage height and cover (fresh and dry matter) than other combinations of pasture type × phase of production (all P < 0.05). There was no effect of pasture type on herbage height and herbage cover (both P > 0.05).
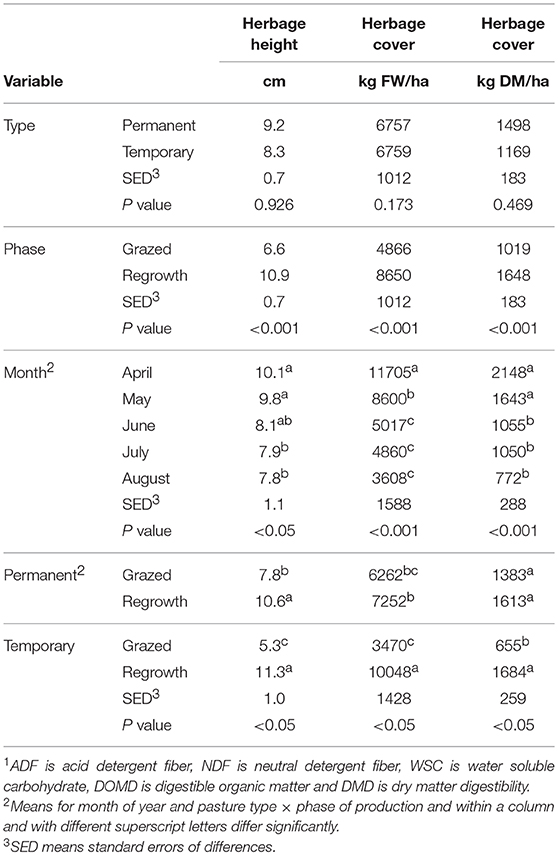
Table 4. Effect of pasture type (permanent or temporary), phase of production (grazed or rested for regrowth), and month of year (April to August) on herbage height, and fresh weight (FW) and dry matter (DM) herbage cover.
Permanent pastures were associated with significantly higher concentrations of dry matter, WSC (both P < 0.001) and NDF (P < 0.05) but lower crude protein, ADF (both P < 0.05) and ash (P < 0.001) than temporary ley pastures (Table 5). Periods when pastures were grazed were associated with higher dry matter, NDF and WSC (all P < 0.001) but lower crude protein, ash (all P < 0.001), DOMD, and DMD (both P < 0.05). During July, the concentrations of dry matter, ADF and NDF were at their highest and concentrations of crude protein, DOMD and DMD at their lowest, and significantly different to the months of April/May (all P < 0.001). The concentrations of WSC varied from April to August (P < 0.05). With adjustment for fixed effects, the permanent pasture fields of B and C had higher ash concentrations, and Field C had lower DOMD and DMD compared to other fields (all P < 0.05). An interaction was found between temporary ley pastures that were grazed, which had a higher mean NDF concentration (P < 0.001) and lower DOMD and DMD concentrations (both P < 0.05) than other combinations of pasture type × phase of production.
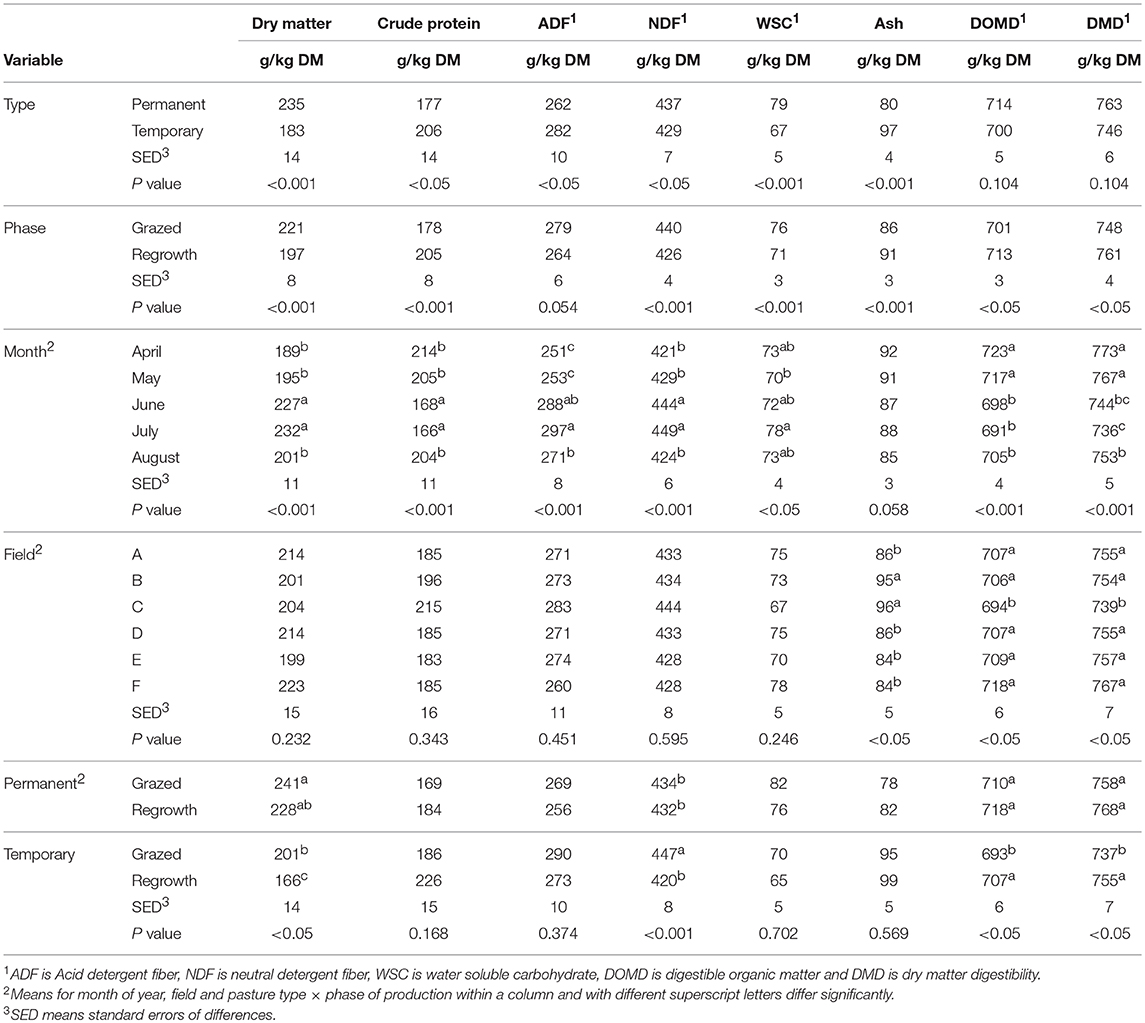
Table 5. Effect of pasture type (permanent or temporary), phase of production (grazed or rested for regrowth), month of year (April to August) and field (A to F) on pasture nutrient concentrations.
Relationship Between Pasture Height, Herbage Cover, and Pasture Nutrients
Fresh and dry matter herbage cover were highly correlated (r = 0.969) and both were highly correlated with pasture height (r = 0.870 and 0.842 respectively, both P < 0.001; Table 6). Pasture height and fresh herbage cover were positively correlated with concentrations of crude protein, ash (both P < 0.05), DOMD and DMD (both P < 0.001), and negatively correlated with concentrations of dry matter (P < 0.001), ADF, NDF (both P < 0.05) and WSC (P < 0.05 for pasture height only). Dry matter herbage cover was positively correlated with DOMD and DMD concentrations (both P < 0.001), and negatively correlated with ADF concentration (P < 0.001). The correlation between fresh herbage cover, pasture height and different pasture nutrients were of similar magnitude. The concentration of dry matter in the pasture was positively correlated with ADF, NDF and WSC, and negatively correlated with crude protein, ash, DOMD and DMD (all P < 0.001). The concentration of crude protein in the pasture was positively correlated with ash, DOMD and DMD, and negatively correlated with ADF, NDF and WSC (all P < 0.001). Concentrations of ADF and NDF were positively correlated (P < 0.001), and both were negatively correlated with DOMD and DMD (which are highly correlated; all P < 0.001). The concentration of WSC was positively correlated with concentration of ADF (P < 0.05) and negatively correlated with concentration of ash (P < 0.001).
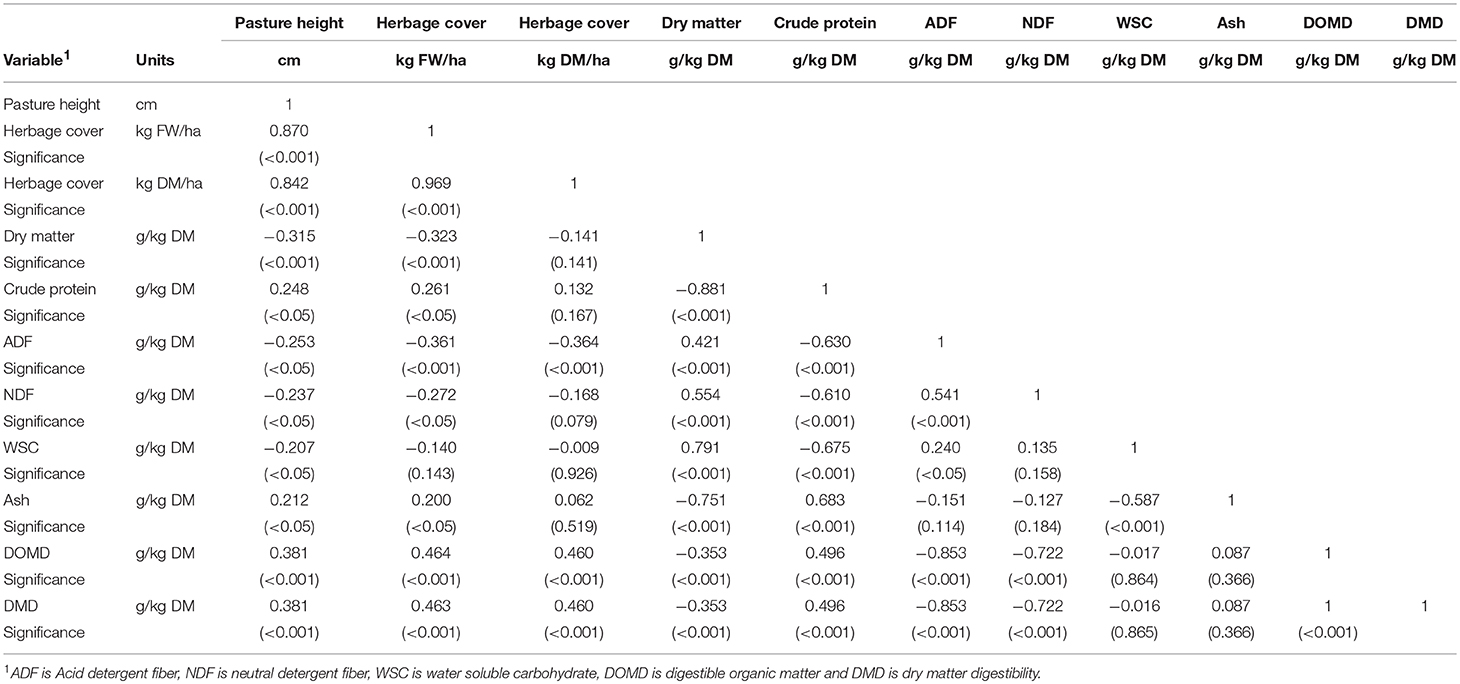
Table 6. Pearson correlation coefficients and significance of the relationship between pasture height, herbage cover (fresh and dry matter) and pasture nutrient concentrations.
Discussion
Precision Monitoring
As shown in this study, the use of real-time data on pasture dry matter concentration measured by NIRS can be combined with a measure of pasture production to determine the dry matter herbage cover. The alternative would be to dry the pasture samples in a microwave or oven to calculate the mass of dry plant material. Removing pasture samples from the field may allow the plant material to degrade and affect analysis results, as well as taking additional time to process the sample. The analysis of other pasture nutrient concentrations is typically done in the laboratory after being sent in the postal system, with results received after the grazing event. Unlike ensiled forages both oxidative degradation of carbohydrates and hydrolysis of peptides continues post-harvest in fresh grass (Binnie et al., 1997; Dale et al., 2016). Dale et al. (2016) suggested nutrient analysis should occur within 24 h to minimize degradation to plant material and changes in nutrient concentrations. This is unlikely to occur within a small country such as the UK, let alone larger countries, where the distance to analytical laboratories may be greater. Therefore, for perishable plant material, the implementation of real-time NIRS is better suited.
Monitoring Pasture Variability
Temporal and spatial changes in nutrient concentrations of pasture are important to the productivity of grazing animals and forage production (Miller et al., 2001; Wilkins and Humphreys, 2003; Colmenero and Broderick, 2006). Herbage digestibility (DOMD and DMD) has a major effect on herbage intake, with early-season management one of the overriding factors determining herbage digestibility (Ferris, 2007). Poor nutrient intake can impair the production and wellbeing of the animal, due to an inability to achieve required nutrient intakes.
As shown in this study on a small number of fields used for grazing and silage production and observed by others studying thousands of pasture samples from farms across the UK (Wilkinson et al., 2014), considerable temporal and spatial variation in quality and quantity of pasture biomass exists, which is currently poorly understood particularly in the case of nutrient concentrations in different pasture types. In the current study, the coefficient of variation for fresh herbage cover was 78% and dry matter herbage cover was 71% across fields and weeks of the study (Table 3). The nutrient concentrations of dry matter, crude protein and WSC showed more variability across fields and weeks (coefficient of variation ranging from 18 to 23%) than other nutrient concentrations (coefficient of variation ranging from 2 to 13%). The mean and range of pasture nutrient concentrations observed in the current study were similar to values in other studies in the UK (Wilkinson et al., 2014). Wilkinson et al. (2014) found similar coefficients of variation for nutrient concentrations of dry matter, crude protein and WSC in pre-grazed pasture ranging from 22 to 27%, but greater variability in ADF and NDF (coefficient of variation ranging from 14 to 19% compared to 5 to 10% in the current study). Several factors can influence pasture quality including sward management (Curran et al., 2010; Crosse et al., 2015), maturity and season (Binnie et al., 1997; Frame and Laidlaw, 2011; Wilkinson et al., 2014), grass variety, sward botanical composition and soil properties (Frame and Laidlaw, 2011). During the wettest and warmer summer month of July (Figure 1), the concentrations of dry matter, ADF and NDF were higher and concentrations of crude protein, DOMD and DMD lower compared to the driest and coolest spring month of April. Seasonal changes in climate, pasture production and sward maturity may explain the seasonal changes observed for the majority of pasture nutrients measured; no seasonal effect on ash concentration was observed. Frame and Laidlaw (2011) reported no seasonal effect on ADF, NDF and WSC in the cooler and wetter climate of Northern Ireland for a dairy grazing system.
The use of real-time nutrient analysis in the current study allowed differences in pasture nutrients to be assessed under different management practices. Grazed pastures, and a decline in herbage height and cover, were associated with lower concentrations of crude protein, DOMD and DMD as the composition of the sward is more stem and residual plant material than vegetative leaf material. The seasonal decline in crude protein and digestibility are consistent with other studies over the same months (Frame and Laidlaw, 2011; Wilkinson et al., 2014). The current study found grazed (mean height of 6.6 cm) and temporary ley pastures that were grazed (mean height of 5.3 cm) had significantly lower DOMD and DMD and higher NDF concentrations than non-grazed and other combinations of pasture type × phase of production respectively. The higher mean herbage height of 7.8 cm for grazed permanent pastures (6.2 tons fresh herbage and 1.4 tons of dry matter herbage cover) had no significant impact on pasture digestibility (DOMD or DMD). This finding is supported by Hodgson (1990) who found the dry matter intake of cattle and sheep was reduced below about 7 cm, presumably due to similar changes in pasture digestibility. In the current study, due to availability of grazing for cattle and sheep at the farm studied it was not possible to assess differences between pastures grazed by different livestock species due to the lack of replication in the design of the study. Even with reduced nutrient intake due to herbage height (e.g., Hodgson, 1990), physical differences in muzzle size, mechanics of their bite, and body size mean that sheep are better able to graze shorter sward heights than cattle (Frame and Laidlaw, 2011) and therefore sheep grazing systems may benefit more from real-time nutrient analysis. Intensive grazing management has been found to reduce crude protein and DOMD concentrations (Hopkins and Holmes, 2000; McDonald et al., 2011). A review by Ferris (2007) highlighted that a number of studies have advocated grazing swards to a residual height of below 6 cm, which the current study suggests would be detrimental to pasture quality and animal performance due to a potentially restricted nutrient intake for intensively grazed systems. Further, research could use real-time NIRS to explore within-day variability in pasture species nutrients, timing of optimum grazing for cattle and sheep, and silage management. Orr et al. (2001) observed that dairy cows grazing after evening milking had a significantly longer evening meal compared to when grazing after morning milking, which they attributed to a higher dry matter (197 vs. 178 g/kg) and WSC (204 vs. 175 g/kg DM) concentration in herbage grazed in the evening. However, the authors found no overall improvement in milk production. Younger and genetically superior temporary pastures are supposed to be more productive, with better nutrient qualities and greater persistency than older pastures (Miller et al., 2001; Shalloo et al., 2011). The younger temporary ley pastures in the current study had higher crude protein, ADF and ash concentrations than permanent pastures, which had higher concentrations of dry matter, WSC and NDF. The higher concentrations of crude protein and minerals in the temporary ley pastures is possibly due to the presence of clover, which is rich in protein (due to its ability to fix atmospheric nitrogen into the soil) and minerals, and clover also helps increase these nutrients in the grass (Frame and Laidlaw, 2011). Surprisingly, there was no difference in overall digestibility (i.e., DOMD or DMD) between the predominantly perennial ryegrass temporary ley pastures and the permanent pastures, as perennial ryegrass is known to have a higher digestibility than grass species in the permanent pastures (e.g., timothy and cocksfoot), especially as they mature (Frame and Laidlaw, 2011). This lack of difference is presumably due to the grazing management of the pasture types. Reseeding is used to improve the overall productivity from grasslands, with fields selected for reseeding based typically on poor grass production or low perennial ryegrass quantity (Shalloo et al., 2011). Pastures containing high sugar grass varieties have become increasingly common, and were present in the temporary ley swards studied (Fields D to F), but interestingly the permanent pastures had a significantly higher mean concentration of WSC. The aim of high sugar varieties is to increase pasture palatability (with a reduced ADF content; Table 6) and overall animal productivity, which has been found to have variable success and this may be due to a marginal increase in actual overall WSC concentrations in the pasture (Ferris, 2007).
When formulating a diet to be fed to livestock, the conventional approach is to determine the least-cost ration depending on the estimated nutrient requirement of the average animal in the group based on infrequent determination of diet nutrient concentrations. This means that some animals will be underfed, and others overfed. As highlighted in this study, in reality considerable variation can exist in quality of forage, and more precise and current determination of nutrient availability offers considerable productive, financial and environmental benefits from this timely information. Adoption of pasture NIRS analysis will presumably be greater if done on farms, and help improve pasture nutrient management, reduce production costs and reduce the potential for waste at the farm level with better practice. For ruminants, about 35% of energy consumed in the diet is lost in the form of enteric methane, feces or urine and 77% of nitrogen consumed is excreted in feces or urine (Bell et al., 2015). More precise feeding of animals to meet their requirements will improve utilization of consumed forage resources from available land, leading to less nutrients lost per unit product (Bell et al., 2015). The use of NIRS has been shown to provide a fast and reliable analytical method for analyzing forages, products of digestion and potentially provide a prediction of feed intake in grazing situations (Decruyenaere et al., 2009). Eastwood and Dela Rue (2017) found the important factors for adoption of grazing software by farmers were (1) the alignment of data for monitoring key performance indicators, (2) using data for benchmarking and reporting and (3) enabling farm team communication. Eastwood et al. (2013) proposed that the value proposition for farmers of using precision farming tools needs to be clear to encourage farmers to invest time and money toward the equipment and learning how to use the new information effectively. It is envisaged that the reduced analytical cost, speed, improved reliability of current NIRS analysis should encourage greater uptake of pasture analysis with more timely information for farm level decision making. Potentially, you could obtain 20 times more NIRS nutrient analysis results for the equivalent cost of forage wet chemistry nutrient analysis (assuming £0.60 per sample for NIRS and £12 per sample for wet chemistry analysis). Mobile NIRS offers not only the potential of more frequent analysis for farmers to monitor a wide range of key nutrients in forage when they need the information, but also soil and manure nutrients within a soil-plant-animal system. While aerial imaging can provide useful information on biomass production and nutrient concentrations of crude protein and metabolisable energy of grassland (Pullanagari et al., 2018), the proposed ground level analysis in the current study can be used to measure spatial and temporal variation in biomass and nutrient concentrations of dry matter, crude protein, fiber, WSC, ash, and the digestibility of pasture. Detailed information on the diversity in botanical composition of swards and replication in grazing livestock species would add to the study and provide further practical insight for adaptive management of pastures. This study assessed the use of mobile NIRS analysis for monitoring pasture nutrients in real-time. Considerable variation existed in herbage production and concentrations of dry matter, crude protein and WSC in pastures studied. When combined with a measure of herbage height or herbage cover, the measured real-time NIRS nutrient concentrations can help identify temporal (i.e., seasonal) or spatial (i.e., within or between fields) changes that may impact on grazing or silage production. Pastures grazed to a height of below 7 cm had significantly lower concentrations of crude protein, DOMD and DMD compared to taller and greater herbage covers, which may be detrimental to the productivity of the land. More precise monitoring of pastures will (1) improve production system sustainability by enhancing feed utilization efficiency, (2) improve productivity of livestock and conserved forages and (3) reduce the potential for wasted resources.
Author Contributions
MB, LM, and JD carried out the data collection and analysis, with JD and MB writing the paper.
Conflict of Interest Statement
The authors declare that the research was conducted in the absence of any commercial or financial relationships that could be construed as a potential conflict of interest.
Acknowledgments
The authors are grateful to Richard Wynn for providing the NIRS device and his support throughout. LM is grateful to the Erasmus Programme that enabled his research placement at The University of Nottingham.
References
Bell, M. J., Garnsworthy, P. C., Stott, A. W., and Pryce, J. E. (2015). The effect of changing cow production and fitness traits on profit and greenhouse gas emissions from UK Dairy systems. J. Ag. Sci. 153, 138–151. doi: 10.1017/S0021859614000847
Binnie, R. C., Kilpatrick, D. J., and Chestnutt, D. M. B. (1997). Effect of altering the length of the regrowth interval in early, mid and late season on the productivity of grass swards. J. Ag. Sci. 128, 303–309. doi: 10.1017/S0021859696004224
Colmenero, J. O., and Broderick, G. A. (2006). Effect of dietary crude protein concentration on milk production and nitrogen utilization in lactating dairy cows. J. Dairy Sci. 89, 1704–1712. doi: 10.3168/jds.S0022-0302(06)72238-X
Crosse, M., O'Donovan, M., Boland, T. M., Delaby, L., Ganche, E., and Kennedy, E. (2015). Using post-grazing sward height to impose dietary restrictions of varying duration in early lactation: its effects on spring-calving dairy cow production. J. Anim. Sci. 9, 592–603. doi: 10.1017/S1751731114002948
Curran, J., Delaby, L., Kennedy, E., Murphy, J. P., Boland, T. M., and O'Donovan, M. (2010). Sward characteristics, grass dry matter intake and milk production performance are affected by pre-grazing herbage mass and pasture allowance. Livest. Sci. 127, 144–154. doi: 10.1016/j.livsci.2009.09.004
Dale, A., Gordon, A. W., Archer, J., and Ferris, C. P. (2016). Impact of sampling and storage technique, and duration of storage, on the composition of fresh grass when analysed using near-infrared reflectance spectroscopy. Grass For. Sci. 72, 614–625. doi: 10.1111/gfs.12266
Decruyenaere, V., Lecomte, P.h., Demarquilly, C., Aufrere, J., Dardenne, P., Stilmant, D., et al. (2009). Evaluation of green forage intake and digestibility in ruminants using near infrared reflectance spectroscopy (NIRS): Developing a global calibration. Anim. Feed Sci. Technol. 148, 138–156. doi: 10.1016/j.anifeedsci.2008.03.007
Defra (2018). Annual Statistics About Agriculture in the United Kingdom to 2017. Available online at: https://assets.publishing.service.gov.uk/government/uploads/system/uploads/attachment_data/file/741062/AUK-2017-18sep18.pdf (Accessed august 2, 2018).
Dillon, P. (2006). “Achieving high dry-matter intake from pasture with grazing dairy cows,” in Fresh Herbage for Dairy Cattle, the Key to a Sustainable Food Chain, eds A. Elgersma, J. Dijkstra and S. Tamminga, (Dordrecht: Wageningen UR Frontis Series Volume 18. Springer), 1–26.
Eastwood, C., and Dela Rue, B. (2017). “Precision grazing management- understanding farmer uptake of grazing software,” in Proceedings of the 1st Asian-Australasian Conference on Precision Pastures and Livestock Farming, (Hamilton), October 16–18, 2017.
Eastwood, C., Trotter, M., and Scott, N. (2013). Understanding the user: learning from on-farm application of precision farming technologies in the australian livestock sector. Aust. J. Multi Discipl. Eng. 10, 41–50. doi: 10.7158/N12-AE04.2013.10.1
Ferris, C. P. (2007). Sustainable pasture based dairy systems–meeting the challenges. Can. J. Plant Sci. 87, 723–738. doi: 10.4141/CJPS06011
Frame, J., and Laidlaw, A. S. (2011). Improved Grassland Management. Marlborough: The Crowood Press.
French, P., O'Brien, B., and Shalloo, L. (2015). Development and adoption of new technologies to increase the efficiency and sustainability of pasture-based systems. Anim. Prod. Sci. 55, 931–935. doi: 10.1071/AN14896
Gibon, A. (2005). Managing grassland for production, the environment and the landscape. Challenges at the farm and the landscape level. Livest. Prod. Sci. 96, 11– 31 doi: 10.1016/j.livprodsci.2005.05.009
Hodgson, J. (1990). Grazing Management: Science Into Practice. Harlow: Longman Scientific and Technical.
Hopkins, A., and Holmes, W. (2000). Grass: Its production and utilization. Oxford: Blackwell Science.
Hopkins, A., and Wilkins, R. J. (2006). Temperate grassland: key developments in the last century and future perspectives. J. Ag. Sci. 144, 503–523. doi: 10.1017/S0021859606006496
Malley, D., McClure, C., Martin, P. D., Buckley, K., and McCaughey, W. P. (2005). Compositional analysis of cattle manure during composting using a field-portable NIRS. Commun. Soil Sci. Plant Anal. 36, 455–475. doi: 10.1081/CSS-200043187
McDonald, P., Edwards, R. A., Greenhalgh, J. F. D., Morgan, C. A., Sinclair, L. A., and Wilkinson, R. G. (2011). Animal Nutrition, 5th edn. Harlow: Longman Press.
Miller, L. A., Moorby, J. M., Davies, D. R., Humphrey, M. O., Scollan, N. D., MacRae, J. C., et al. (2001). Increased concentration of water-soluble carbohydrate in perennial ryegrass (Lolium perenne L.): milk production from late-lactation dairy cows. Grass For. Sci. 56, 383–394. doi: 10.1046/j.1365-2494.2001.00288.x
Orr, R. J., Rutter, S. M., Penning, P. D., and Rook, A. J. (2001). Matching grass supply to grazing patterns for dairy cows. Grass For. Sci. 56, 352–361. doi: 10.1046/j.1365-2494.2001.00284.x
Pullanagari, R. R., Kereszturi, G., and Yule, I. J. (2018). Integrating airborne hyperspectral, topographic, and soil data for estimating pasture quality using recursive feature elimination with random forest regression. Remote. Sens. 10:1117. doi: 10.3390/rs10071117
Pullanagari, R. R., Yule, I. J., Tuohy, M. P., Hedley, M. J., Dynes, R. A., and King, W. M. (2012). In-field hyperspectral proximal sensing for estimating quality parameters of mixed pasture. Precis. Agric. 13, 351–369. doi: 10.1007/s11119-011-9251-4
Ramsbottom, G., Horan, B., Berry, D. P., and Roche, J. R. (2015). Factors associated with the financial performance of spring-calving, pasture-based dairy farms. J. Dairy Sci. 98, 3526–3540. doi: 10.3168/jds.2014-8516
Redman, G. (2016). John Nix Farm Management Pocketbook. Melton Mowbray: Agro Business Consultants Ltd.
Shalloo, L., Creighton, P., and O'Donovan, M. (2011). The economics of reseeding on a dairy farm. Irish J. Agr. Food Res. 50, 113–122. doi: 10.2307/41348159
Stergiadis, S., Allen, M., Chen, X. J., Wills, D., and Yan, T. (2015). Prediction of nutrient digestibility and energy concentrations in fresh grass using nutrient composition. J. Dairy Sci. 98, 3257–3273. doi: 10.3168/jds.2014-8587
Stuth, J., Jama, A., and Tolleson, D. (2003). Direct and indirect means of predicting forage quality through near infrared reflectance spectroscopy. Field Crops Res. 84, 45–56. doi: 10.1016/S0378-4290(03)00140-0
Waghorn, G. C., and Clark, D. A. (2004). Feeding value of pastures for ruminants. NZ Vet. J. 52, 320–331. doi: 10.1080/00480169.2004.36448
Wilkins, P. W., and Humphreys, M. O. (2003). Progress in breeding perennial forage grasses for temperate agriculture. J. Ag. Sci. 140, 129–150. doi: 10.1017/S0021859603003058
Wilkinson, J. M. (2011). Re-defining efficiency of feed use by livestock. Animal 5, 1014–1022. doi: 10.1017/S175173111100005X
Keywords: grasslands, technology, spectral data, predictions, management
Citation: Bell MJ, Mereu L and Davis J (2018) The Use of Mobile Near-Infrared Spectroscopy for Real-Time Pasture Management. Front. Sustain. Food Syst. 2:76. doi: 10.3389/fsufs.2018.00076
Received: 13 August 2018; Accepted: 22 October 2018;
Published: 15 November 2018.
Edited by:
Lynn V. Dicks, University of East Anglia, United KingdomReviewed by:
Emma Louise Burns, Australian National University, AustraliaSarah Evelyn Park, University of East Anglia, United Kingdom
Copyright © 2018 Bell, Mereu and Davis. This is an open-access article distributed under the terms of the Creative Commons Attribution License (CC BY). The use, distribution or reproduction in other forums is permitted, provided the original author(s) and the copyright owner(s) are credited and that the original publication in this journal is cited, in accordance with accepted academic practice. No use, distribution or reproduction is permitted which does not comply with these terms.
*Correspondence: Matt J. Bell, matt.bell@nottingham.ac.uk