- 1Feed and Farm Systems Group, DairyNZ Ltd., Lincoln, New Zealand
- 2Department of Global Value Chains and Trade, Faculty of Agribusiness and Commerce, Lincoln University, Lincoln, New Zealand
There are increasing opportunities to use smart farming technologies for improved management of farming systems. However, there is limited understanding of how the potential can be translated into effective use in the farming sector. Previous studies have highlighted the role that uncertainty plays in technological innovation systems. In this paper, we present the results of an international survey investigating the impact of innovation uncertainty on adoption of a smart farming technology, automatic milking systems (AMS). The objective of this study was to review adoption of AMS internationally and propose lessons for developing institutional knowledge and effective networks of practice in emerging smart farming innovation systems. We used an online survey of AMS experts globally and received 81 completed survey responses. The main countries represented were Canada, The Netherlands, USA, Denmark, and the UK. Respondents identified a range of adoption trends in their country and some of the reasons behind these adoption profiles were suppression of uptake due to low milk prices, financial markets, and issues with early installations and perceptions of these issues by other farmers. In terms of the impact of uncertainty, technological uncertainty was historically an important issue around the early development of AMS, with decommissioning occurring in some cases due to perceived technology issues. Political uncertainty also impacted adoption, with implications of food safety regulations or rules around herd testing systems. Our study highlighted the potential impact of negative experiences associated with new technologies from farmers who struggle with the adaptation process as such occurrences may act to stall the uptake of smart farming technologies. If public policy organizations are to realize the desired impacts of smart farming technology, there needs to be greater focus on understanding where (and which) technologies can have an actual impact on farm as opposed to technologies that only create greater farmer distrust and uncertainty. Our study highlights that to reduce uncertainty with emerging smart technologies, greater public and private R&D collaboration is required to foster knowledge development and exchange.
Introduction
There are increasing opportunities to use smart farming technologies for improved management of farming systems (Shepherd et al., 2018). Potential management improvements are related to enhanced collection of data to manage animals, plants, and the wider farming environment (Eastwood et al., 2017a). However, there is limited understanding of how the potential can be translated into widespread adoption in the farming sector, which has been slow to date (Gargiulo et al., 2018). The uptake of smarter farming approaches often represents more than a “plug and play” process for farmers (Jago et al., 2013). Successful use of these new tools depends on aspects of technology fit-for-purpose, on-farm adaptation, learning about data-driven decision-making, and social learning within a farmer's network of practice (Eastwood et al., 2012; Rose et al., 2016; Higgins et al., 2017; Klerkx et al., 2019). To turn the opportunity of smarter farming into a reality on-farm, we need to better understand the wider issues affecting a farmer's investment decision making (Rutten et al., 2018).
Previous studies have highlighted the role that uncertainty plays in the functioning of technological innovation systems. For example, Meijer et al. (2007b) identified the importance of technological, resource, competitive, supplier, consumer, and political uncertainty. The use of farm system-changing smart farming technologies such as automatic milking systems (AMS) [see Rodenburg (2017) for a description of AMS technology] requires not only a reconfiguration of farming practice, but also in the systems that operate around the farmer, for example, knowledge of veterinarians on how to maintain reproductive performance under AMS or structural changes to herd testing protocols (Svennersten-Sjaunja and Pettersson, 2008; Hansen, 2015; Rodenburg, 2017). The success of an innovation system can depend on minimizing the uncertainty around the innovation (Meijer et al., 2007b; Kuehne et al., 2017). Poor or haphazard innovation system reconfiguration can increase the uncertainty that farmers or their advisors have about a technology and impact on its successful uptake and implementation.
Within this context, the objective of this current study was to understand drivers for adoption of AMS internationally and propose lessons for developing institutional knowledge and effective networks of practice in emerging smart farming innovation systems. In this paper, we present the results of an international survey investigating the impact of innovation uncertainty within AMS support networks across different institutional environments. First, we outline the conceptual framework based around innovation uncertainty, and then we present the methods and results of the survey and discuss them in relation to the conceptual framework. The novel contribution of this paper is 2-fold: first, it adds to knowledge of the specific factors influencing farmer adoption of smarter farming technologies such as AMS, and second, it adds to the limited literature that empirically explores the role of various factors of uncertainty in technological innovation systems.
Conceptual Framework
Adoption of agricultural technologies has been extensively studied and perspectives vary from a diffusion of innovations perspective (Rogers, 1962) to the more holistic concepts of agricultural innovation systems (Klerkx et al., 2010). The agricultural innovation systems (AIS) approach considers the role of institutional change within agricultural innovation and potential benefits from different ways of organizing within such systems (Morriss et al., 2006; Klerkx et al., 2010). Successful agricultural innovations depend on factors such as technology development, institutional change, supply chain reorganization, market development, and creating societal acceptance (Klerkx et al., 2010). The AIS concept has value as an analytical framework to “improve everyday innovation capacity” (Spielman and von Grebmer, 2006). One feature of AIS is the role of uncertainty in the uptake and use of technologies. Meijer et al. (2007b) identify six forms of uncertainty that might occur: technological, resource, competitive, supplier, consumer, and political. Individuals (including farmers or service providers) within AIS may have little ability to influence the uncertainty existing around an innovation. Uncertainty within innovation systems can potentially reduce the uptake of a technology, affect its integration into the farm system or industry, and can prevent some actors from engaging in the innovation system (Meijer et al., 2007b).
While the sources of uncertainty cited by Meijer et al. (2007b) focus on the formation of innovation projects, and in particular the impact on entrepreneurial action, the framework could be applied to the actions of farmers and advisors in respect to new system-changing innovations such as AMS. An ongoing process of AMS innovation could therefore be viewed as dependent on not just the technology or its developers, but also the farmers, distributors, milk companies, researchers, consultants, and regulatory agencies that also operate in the AMS space. Through the AIS approach, the actors involved in an innovation system can be identified, along with possibilities for different ways of organizing the actors. Innovation systems analysis can highlight the development of agency in actors and the reduction of uncertainty in the environment in which the actors operate.
Uncertainty surrounding a smart farming innovation can occur during the early development phase, including uncertainty related to available support and finance, and around best practice when using the technology. While innovation developments are rarely associated with low uncertainty, too much uncertainty can cause stagnation in respect to the ongoing innovation process, or lead to “failure” of an innovation (Kuehne et al., 2017). Meijer et al. (2007b) describes a framework for analyzing “perceived uncertainty” in the early stage of an innovation (Figure 1). Few empirical studies have applied the innovation uncertainty framework to case studies (Meijer et al., 2007a; Roper and Tapinos, 2016); therefore, the novelty of our study is in relation to both the empirical survey of uncertainty factors and explaining longitudinal adoption trends.
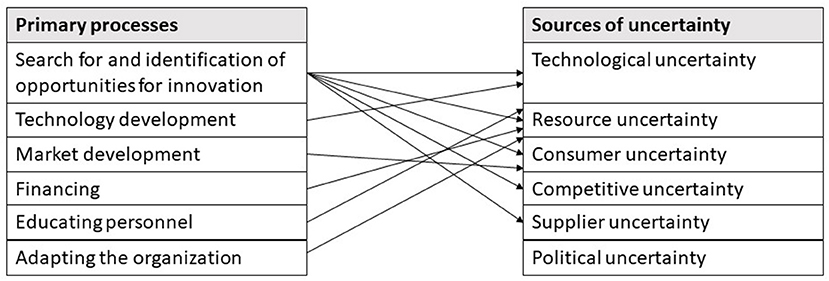
Figure 1. How the primary processes are linked to six sources of uncertainty (adapted from Meijer et al., 2006).
Adoption of AMS in Different Dairy Farming Countries
AMS involve the milking of dairy cows without human labor and are based on robotics and sensor technology. Since the first commercial AMS units were installed on a dairy farm in The Netherlands in 1992, there has been a range of adoption rates across different dairy farming countries. No single organization maintains statistics of the milking installations across different countries, and the information is held tightly by AMS retailers; however, some publications have provided data on installations over the last two decades (de Koning, 2010; Barkema et al., 2015; Tse et al., 2017). By 2015, there was up to 25,000 dairy farms using AMS worldwide, with the technology most popular in The Netherlands and Scandinavia (Rodenburg, 2017).
In the current paper, we focus on the “box-type” AMS rather than the robotic rotary systems that are also commercially available. In Table 1, we present data drawn from several sources to highlight the AMS adoption trends in dairy-producing countries where there were sufficient data from 2002 to 2018. Through to 2018, Iceland and Sweden had the greatest percentage of farms using AMS, at around 30% of farms, followed by another cluster of countries between 20 and 25% including Denmark, The Netherlands, Norway, Belgium, and Switzerland (Hogenkamp, 2018; Sigurdsson et al., 2019; Vik et al., 2019). Less data are published for other dairy countries; however, Canada (7% of farms) has seen a steady increase in installations (Tse et al., 2017). Limited data are available for the UK and USA; however, it is estimated that around 7% of farms in the UK (Hogenkamp, 2018) and 3% of farms in USA were using AMS by 2018 (Reed, 2018). There are few farms using AMS in Australia or NZ (<1% of farms). Interestingly, the data show that, in recent years, the percentage of farms using AMS in Denmark has peaked and is now declining, in part due to increasing farm sizes making other milking parlors more cost-effective (Sigurdsson et al., 2019).
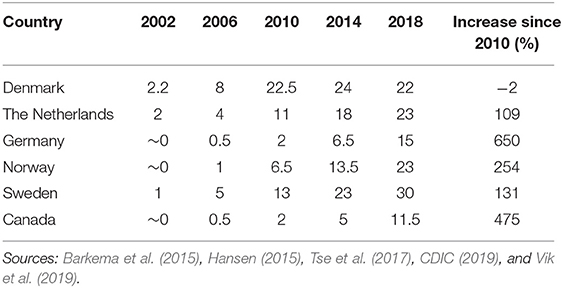
Table 1. Automatic milking system adoption rates from 2002 to 2018 in several dairy producing countries (% of total farms in each country, rounded to nearest 0.5%).
Methods
An online survey was designed to capture processes around AMS uptake, through three phases of the adoption process: (1) when farmers are initially thinking of investing in AMS, (2) when farmers have made the decision to invest, and (3) after they have installed and are using the AMS. The survey was conducted online via the SurveyMonkey™ platform. Closed questions were primarily used, with the number of open-ended questions limited to minimize survey length (Bryman, 2001). There were 116 questions, including demographic questions and questions on the role of the respondents and their organization in AMS development and extension, respondents' experience with AMS adoption, and patterns of AMS adoption in their country. In the current paper, we focus on a subset relevant to innovation uncertainty. The questions were developed based on AMS-related studies that had been published prior to survey design (Meskens et al., 2001; Shephard, 2004; Svennersten-Sjaunja and Pettersson, 2008; de Koning, 2010; Khanal et al., 2010). Below, we discuss the drivers for selecting AMS-related questions for each of the innovation uncertainty factors. The survey respondent answers to the questions are then listed in Table 2.
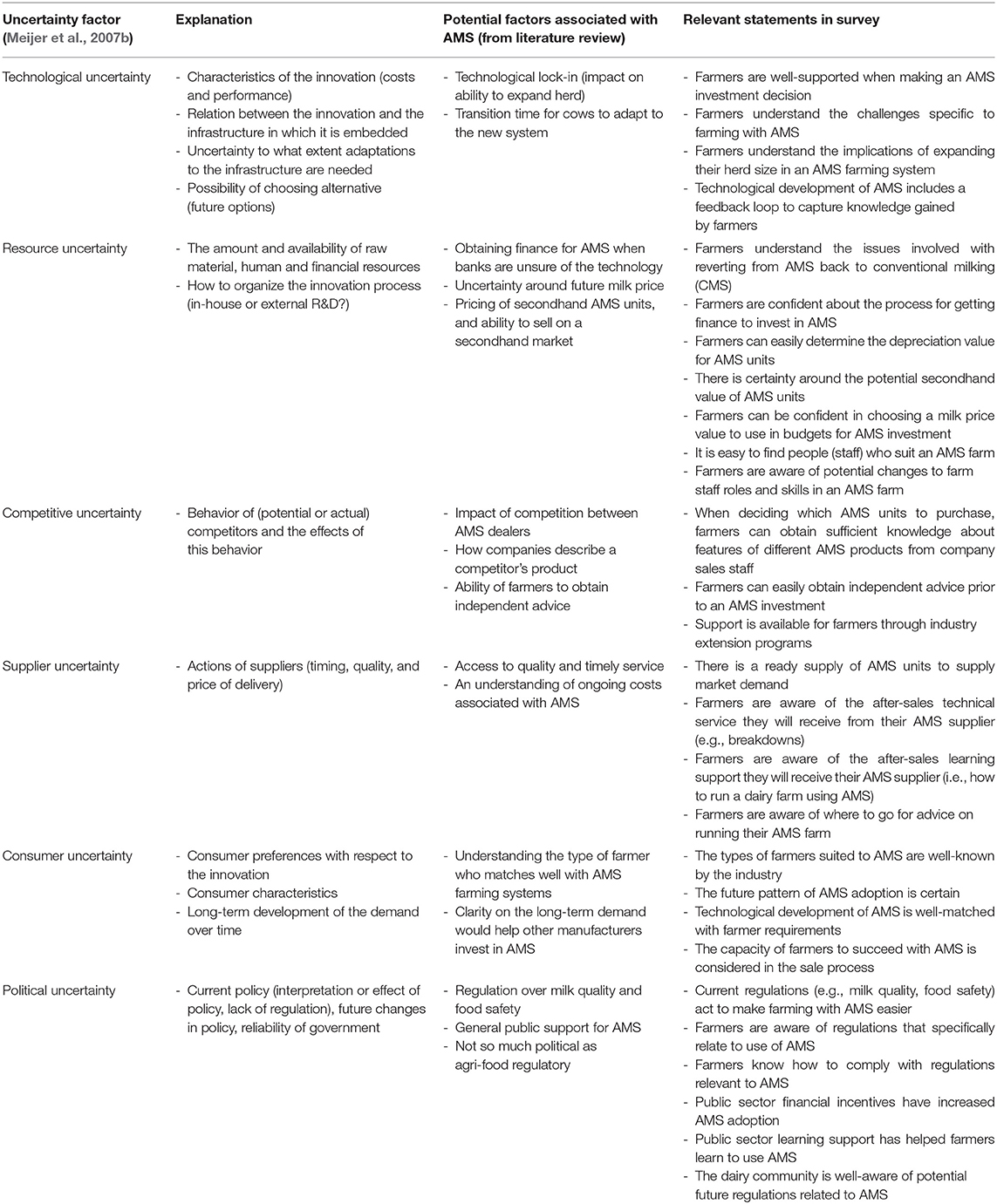
Table 2. Survey questions asked in relation to the six forms of uncertainty within innovation systems [adapted from Meijer et al. (2007b)].
Technological Uncertainty
Uncertainty around the characteristics of an innovation, related infrastructural implications, the level of adaptation required, and the impact on future options are all aspects of technological uncertainty (Meijer et al., 2007b; Klerkx et al., 2010; Tomy and Pardede, 2018). Relevant factors to AMS could be the support available to farmers when making investment decisions, the degree of technological lock-in (including the ability to expand herd sizes), and the specific challenges of adapting farming systems to AMS.
Resource Uncertainty
This factor focuses on the availability of resources, such as human, financial, and material, and also encompasses organization of the process of innovation (Meijer et al., 2007b). The uncertainty around forecasting resources and capital required for the innovation involves factors such as availability of knowledge and skills, required R&D expenditure, and potential revenue streams (Tomy and Pardede, 2018). In an AMS context, resource uncertainty could relate to the ability to get finance for the AMS investment, uncertainty around milk price and its impact on viability, along with other factors such as uncertain pricing of secondhand AMS technology and how to revert to previous milking methods.
Competitive Uncertainty
The behavior of competitors in the innovation system can affect its success (Meijer et al., 2007b). Factors behind this uncertainty can include level of market share, the impact of leading competitors, and the type of competition in the market (Tomy and Pardede, 2018). This relates to the competition between retailers of AMS technology (i.e., is there sufficient competition in a market dominated by two main players?) and how each competitor might refer to each other's product. We assessed this by asking questions around the availability of independent advice on technology, the adequacy of advice provided by technology retailers, and what industry support was available to farmers.
Supplier Uncertainty
This source of uncertainty relates to perceptions around the reliability of the supplier (Meijer et al., 2007b). In respect to AMS, we asked questions around the availability of AMS technology (was there sufficient supply to match demand), the access that farmers had to back-up service for both technical and learning support, and whether farmers knew where to access advice about farming with AMS.
Consumer Uncertainty
Consumer uncertainty concerns the preferences consumers might have for an innovation, the characteristics of consumers, and the development of demand for the technology (Meijer et al., 2007b). It also includes factors associated with knowledge of consumer acceptance of the technology, and the potential changes in demographics of the target population and therefore potential market size (Tomy and Pardede, 2018). These features are more applicable to entrepreneurs looking to work with consumers (farmers) rather than the consumers themselves. Therefore, for this factor, we asked industry-related questions such as uncertainty around future patterns of AMS adoption, the nature of technological development, whether farmer ability to succeed was included in the design and sale process, and the fit of AMS with farmer typologies. This is one aspect of the framework that potentially has less applicability when taking the farmer perspective in an AMS innovation system.
Political Uncertainty
The policy environment can have a major impact on the innovation process, for example, the interpretation of policy, existence of regulations, and uncertainty regarding government and policy changes (Meijer et al., 2007b). It also includes the potential impact of government support for the innovation, the impact of exchange rates, and taxation that may relate to the innovation (Tomy and Pardede, 2018). In respect to AMS, this may include the implications of milk quality and food safety regulations, general political and community support for AMS, the awareness of regulations, and the existence of incentives.
The survey design incorporated the framework derived from Meijer et al. (2007b) and Klerkx et al. (2010) to assess the impact of uncertainty in the AMS innovation system. For each of the uncertainty factors, a series of statements were developed by the project team (Table 2), and participants were asked to indicate to what extent they agreed or disagreed with each statement, based on a five-point Likert scale from 1 = strongly disagree to 5 = strongly agree.
A pilot of the survey was run with five experienced AMS researchers from New Zealand, The Netherlands, USA, England, and Australia. Feedback from the pilot group was incorporated into the final survey design. Participants in the full survey were chosen to represent a range of those in the network of practice of AMS farmers internationally including: AMS researchers, technology developers, and sales/support representatives. Actors in the research and service/support sectors were targeted primarily due to language differences across the countries surveyed. The project team decided that people from these sectors were more likely to engage and complete the extensive English-based survey. The survey was therefore designed for these actors to use their knowledge of both farmer experiences, and innovation system-wide issues, to answer the questions posed.
Contacts were sourced initially through researcher networks, and then a snowball method was used (Bryman, 2001). The study was approved by the University of Melbourne Human Research Ethics Committee (HREC), and a plain language statement outlining the project aims, funders, use of data, and key contacts was provided on the opening survey page. If participants consented to participate, they were invited to click “next” to enter the survey.
The survey weblink was sent out twice to those on the contact list. Results were exported as a.csv file and imported into Microsoft Excel™ Data were reviewed for quality and completeness and any erroneous responses were removed. The data were analyzed for interactions using multivariate statistics, but no strong associations were found; therefore, we focused the analysis on counts and summary statistics.
Results
In this section we outline the key results, beginning with an overview of the survey participant demographics, their experience and opinions related to AMS, and finally the results of questions related to the uncertainty factors.
Survey Participants
There were 84 survey responses of which three were removed due to incomplete responses; therefore, 81 responses were used in the analysis. The major countries represented were Canada (24), The Netherlands (14), USA (10), Denmark (7), and the UK/Ireland (6). Other countries represented were Germany (4), Sweden (3), Israel (2), Norway (2), and Switzerland (2). There was one representative from each of Finland, France, Ireland, New Zealand, India, Iceland, and Japan. The respondents were primarily male (89%) and 63% were aged 35–54.
There was a range among respondents when it came to their day-to-day experience with AMS farmers with 27 stating it was “a major part of my job,” 27 said it was “often part of my job,” and 26 a “small part of my job.” There was a similar mix in respect to years of experience that respondents had with AMS farms and farmers with 27 having over 10 years' experience, 27 had 5–10 years' experience, and 27 had <5 years' experience. There were 36 respondents from AMS retailers and 45 respondents not from AMS retailers (36 from public or industry funded research and advisory organizations, and 9 from privately funded advisors or consultants). In answering the survey questions, the respondents drew from experience that ranged from working with 1 farm to 1,000 farms. Most respondents interacted with between 20 and 100 farmers. The responses for each country, grouped by role (retailer vs. non-retailer) and experience, are shown in Table 3.
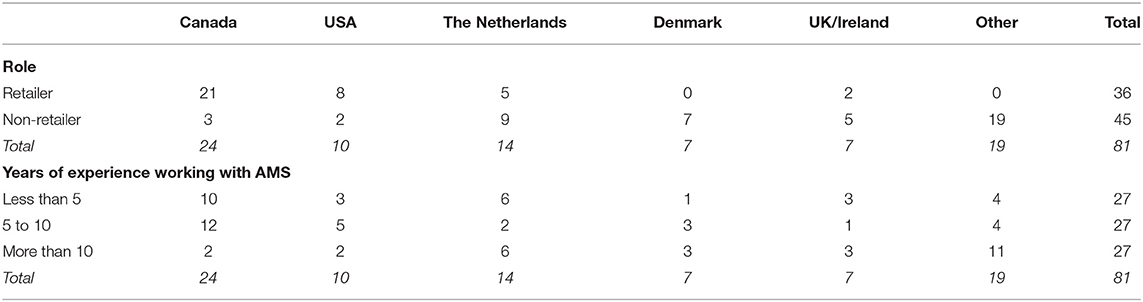
Table 3. Number of respondents by country where they are primarily based compared to role and years of experience with AMS.
Of non-retailer respondents who were in a publicly funded research/advisory position, or identified as consultants, most were aligned with research. Many also provided general farm management advice to farmers and to a lesser extent helped farmers before and after AMS installation. Providing technical support was generally a small part of their role.
Survey Responses
How Respondents Perceived Their Role in the AMS Innovation System
The AMS retailer representatives who completed the survey primarily described their role as helping farmers learn to use AMS, along with providing technical support. A smaller part of their jobs in general was actively selling AMS or installing the equipment. They indicated that the organization they worked for had a wider role from installing equipment, providing pre-sale and after-sales support. In terms of their AMS skill base, the company representatives indicated they learned slightly more through practical experience and interacting with farmers than via specific training. They were generally happy with their skill levels but felt they could learn more about farm management. The roles of non-retailers were less focused on technical support, and more on delivering AMS-related research and development knowledge, providing farmers with support prior to AMS investment, and providing general farm management advice. Few non-retailers (22%) had been specifically trained in supporting farmers using AMS, compared to 77% of retailers. Around half of non-retailers (49%) agreed that they were happy with their skills and knowledge related to AMS, compared to 71% of retailers. Both cohorts agreed they learned through interacting with AMS farmers (non-retailers 86% and retailers 91%). Additionally, they also agreed that they needed to learn more about farm management associated with AMS (non-retailers 67% and retailers 71%).
Respondents were asked about their opinions of the impact and future role of AMS in the dairy sector (Table 4). Most (87%) agreed that AMS required farmers to make significant changes to their farm systems, and responses were relatively consistent between retailers and non-retailers. Respondents also agreed (80%) that AMS represented the biggest transformation in dairy farming in the last 50 years. When asked if AMS would become the dominant milk harvesting method, most agreed (69%), and more retailers strongly agreed (53% compared with 30%).

Table 4. Respondent opinions regarding the impact of AMS on dairy farming. Results are presented as percentages, with retailer, non-retailer in brackets, respectively.
Perceptions on AMS Investment, Past, and Future
The most significant reasons for past AMS investment by farmers were identified as reducing total farm labor, reducing hours spent milking, more family time, and to reduce physical work. Improving milk quality, increasing production, and sustaining the farm business were not seen as overly important. Main reasons for farmers not investing were identified by respondents to be the cost of technology and to a lesser extent issues around herd expansion, difficulty obtaining finance, fit with farm system, and experiences of other farmers. Less of an issue were farmer perceptions of management issues during transition and availability of advice for farmers during the investment decision.
Respondents noted some decommissioning of AMS farms, although most respondents classified it as a “rare” occurrence. Countries where more than 10 decommissioned AMS farms had been observed included Denmark, The Netherlands, and the UK but this needs to be viewed in the context of the total number of AMS units in these countries and their position as sites of early adoption in the 1990s before the technology was mature. Reasons for decommissioning suggested by respondents included economic factors leading to bankruptcy (farm or company), initial lack of knowledge about using AMS and availability of support, initial technology issues (mostly in the 1990s), need for large herd flexibility, and lack of fit with farmers (incompatible expectations or skills). Specific comments of respondents highlighted the range in adoption trajectories in the first 10–15 years of AMS use (i.e., up to 2010). Below, we outline comments of respondents from The Netherlands (a mature market) and Canada (an emerging market) in relation to the adoption trajectories.
In The Netherlands, a mature AMS market at the time of surveying, respondents identified that in the early installations, there were technical problems and skepticism among farmers that automated milking was possible. Factors that led to greater uptake were more trust in the technology, a period of higher milk prices, the ability to have increased work flexibility, and more understanding among farmers of how to run AMS-based farm systems. There were up to five different AMS suppliers in The Netherlands. Comments made by some respondents were as follows:
“At the moment approximately 1 out of 2 new [milking] machines is an AMS, quite popular among the family farms. Larger farms (>200 cows) often decide to have a rotary parlor or large rapid exit side by side or herringbone parlors.” (Netherlands, non-retailer)
“In the first 5–10 years, AMS was bought mainly by early adopters, sometimes farmers who were very interested in the technology. In the recent decade this changed to farmers interested in optimizing individual cow management by using this technology.” (Netherlands, non-retailer)
In Canada, an emerging AMS market at the time of surveying, respondents noted a pattern of some installations, then a “tapering off” or some deinstallation, followed by a more rapid increase around the time of the survey. This was for a variety of reasons including after-sales service quality, poor understanding of the farm systems (in particular feeding) changes required, and farm economic issues. There were three different AMS suppliers in Canada. Comments made by some respondents were as follows:
“In the beginning we did not understand robotic milking correctly in Canada. We had to learn how to be successful. Robots were pulled out and this slowed the sales process for about three years. Robot knowledge then became better and the units themselves continued to progress. We now know that robotics work. We also know that it only works with some farmers and we have to be very careful who we sell to.” (Canada, retailer)
“The first robots were pulled out because we did not understand robotic milking fully. A learning curve had to happen and feeding strategies in Canada needed to be implemented to make sure the robots ran successfully” (Canada, retailer)
We also asked questions about predictions for future adoption and the predicted adoption trajectories are presented in Table 5. Across all respondents, 62% of respondents thought AMS uptake in their country would increase over the next 5 years, and another 23% thought it would rapidly increase. There was more confidence in the level of increased AMS adoption among retailers in comparison to non-retailers, with 35 and 14% expecting a rapid increase, respectively. Expectations of a rapid increase were also greater in the emerging markets of Canada and USA, when compared with the more mature markets of Denmark and The Netherlands. Increased labor costs were seen as a major future driver, along with increased confidence in AMS. Possible factors holding back AMS use was incompatibility with increased herd size and fluctuations in milk price or the farm economic environment.

Table 5. Respondent opinions regarding the future adoption of AMS from 2011 to 2015, presented as percentages.
Comments of respondents from The Netherlands (mature market) indicated that future adoption would be driven by higher farm labor costs, a desire to maintain family farming, and well-being factors. One limitation to adoption identified in The Netherlands was farm expansion, with herds of over 200 cows seen as a point where farmers considered other milking technologies such as a rotary or herringbone parlor. Canadian respondents felt that there would be more installations in the future. The reasons behind this were related to generational change on farm (younger farmers would invest in AMS to have more social time), it has become more reliable and trusted among farmers, and a lack of available agricultural workforce. One respondent noted that “With new anti-expansion quota policies several larger dairies are now considering robots as well.”
Role of Innovation Uncertainty on AMS Adoption Trends
Responses to survey questions related to different uncertainty factors are summarized in Table 6 and displayed in Figures 2–4. The results are summarized across all respondents, by role, and by country. The countries listed represent those with the most responses in the survey, and show mature AMS markets (The Netherlands, Denmark) and emerging markets (UK/Ireland, USA, Canada). Below, each individual factor is numbered and we refer to them in the text from F1 to F28.
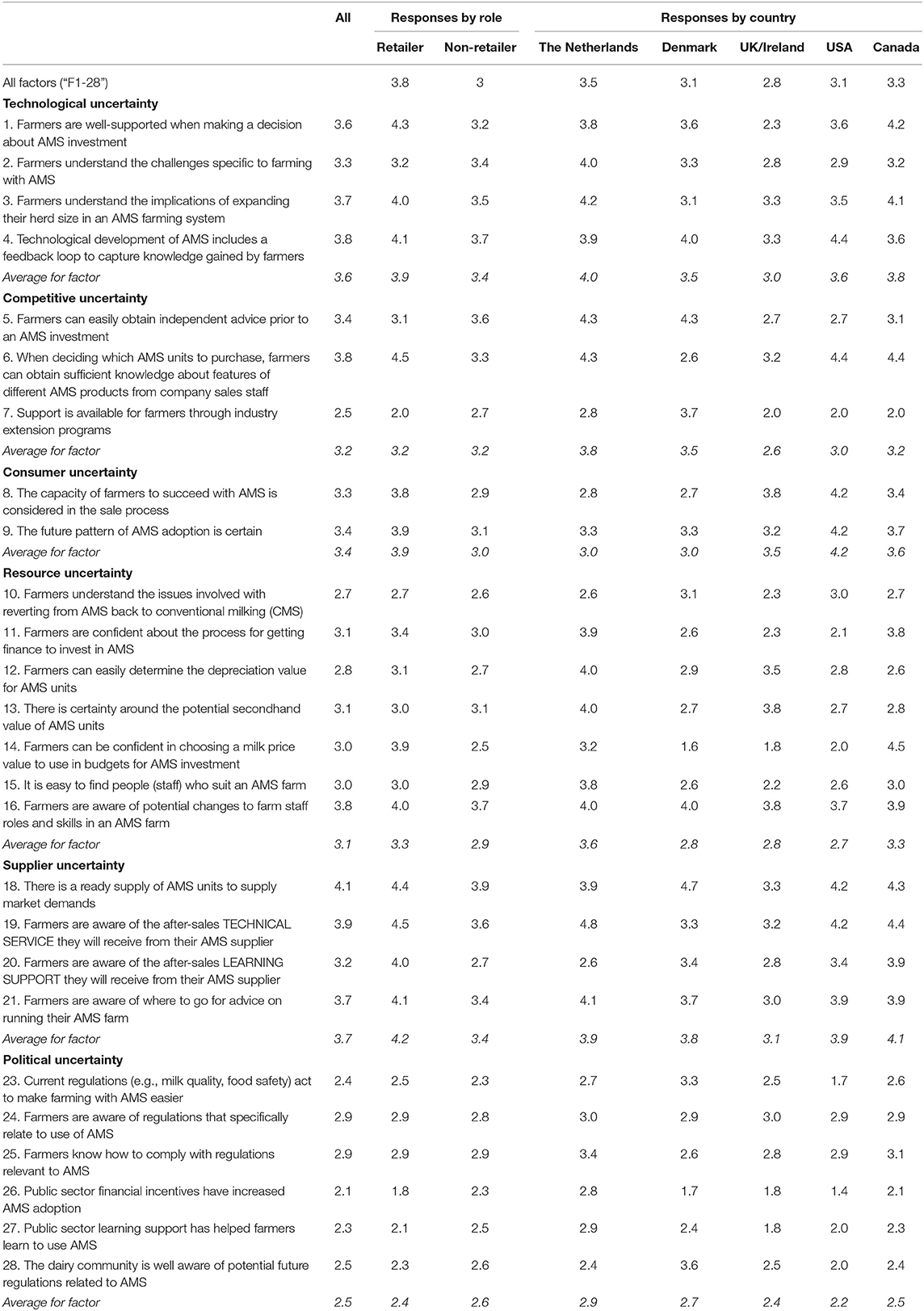
Table 6. Average responses to questions for the six innovation uncertainty categories, by role and country (scale of 1–5 where 1 = strongly disagree, 5 = strongly agree).
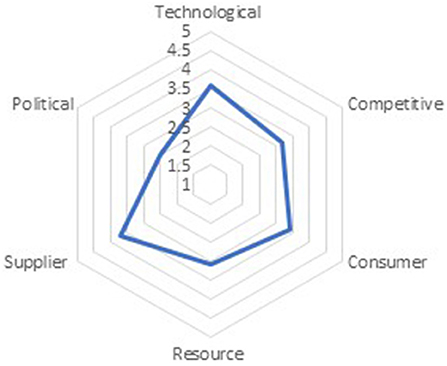
Figure 2. Average factor scores for the six uncertainties across all responses (scale of 1–5 where 1 = strongly disagree, 5 = strongly agree).
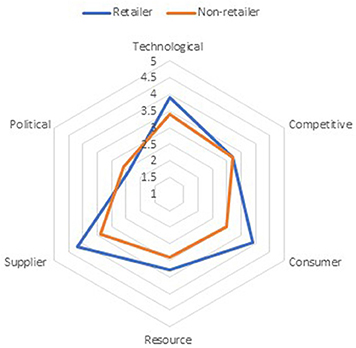
Figure 3. Average factor scores for the six uncertainties across roles (retailer and non-retailer) (scale of 1–5 where 1 = strongly disagree, 5 = strongly agree).
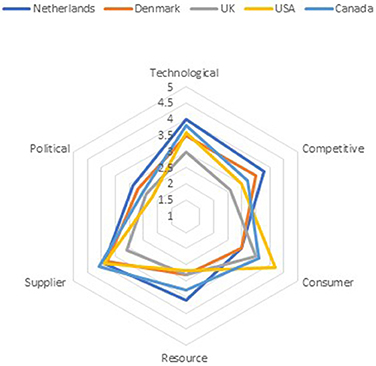
Figure 4. Average factor scores for the six uncertainties across countries (scale of 1–5 where 1 = strongly disagree, 5 = strongly agree).
Technological uncertainty
There were four questions related to technological uncertainty. Retailers were more positive when scoring these factors (average 3.9), compared with non-retailers (3.4), with the most divergence regarding whether farmers were well-supported when deciding to invest in AMS. The most agreement between these groups was in relation to farmers understanding the farm system challenges associated with AMS, with both cohorts being less positive for this factor (3.2 and 3.4). In terms of responses from different countries, respondents from The Netherlands and Canada had the most positive responses (4.0 and 3.8, respectively) with a 3.0 average for UK respondents. The UK respondents did not agree that farmers were well-supported in making investment decisions (2.3) or that farmers understood the challenges of AMS (2.8)—particularly compared with Dutch responses to these factors, 3.8 and 4.0, respectively.
Competitive uncertainty
Retailers and non-retailers provided the same average responses to the competitive uncertainty factors (3.2), but there was divergence among the individual factors, for example F6 where retailers agreed (4.5) that farmers can obtain sufficient knowledge about different AMS features, whereas non-retailers were more neutral (3.3). Conversely for F7, retailers (2.0) did not think support was available through industry extension initiatives, while non-retailers were more neutral (2.7). In respect to responses by country, UK respondents again were less positive on average (2.6), with The Netherlands most positive (3.8). The mature markets (The Netherlands 4.3, Denmark 4.3) agreed that farmers could easily obtain independent AMS advice (F5), compared with neutral responses from emerging markets (UK 2.7, USA 2.7, Canada 3.1). There was a similar trend for F7 on industry extension initiatives.
Consumer uncertainty
Retailers were more positive about the two consumer uncertainty factors (F8 and F9) than non-retailers, particularly in relation to whether the capacity for farmers to succeed with AMS was considered in the sale process. Additionally, respondents from countries in mature AMS markets provided overall neutral (3.0) responses while emerging markets showed a higher level of agreement with both factors (USA 4.2, Canada 3.6).
Resource uncertainty
There were seven factors related to resource uncertainty, and the average response for all respondents was neutral (3.1), with limited difference in the average between non-retailers and retailers. Dutch respondents (3.6) were slightly more positive than respondents from other countries. In terms of the individual factors, retailers (3.9) and non-retailers (2.5) differed most about whether farmers could confidently choose a milk price for their budgets. Overall, there was most disagreement (2.7) that farmers understood about reverting back to conventional milking, with respondents from UK/Ireland providing a rating of 2.3. Dutch respondents agreed most strongly that farmers were confident about getting finance (3.9), could determine depreciation values (4.0), were certain around secondhand AMS markets (4.0), and were aware of staff roles with AMS (4.0). Respondents from Denmark (1.6), UK/Ireland (1.8), and USA (2.0) most strongly disagreed that farmers could be confident on milk prices for their budgeting.
Supplier uncertainty
Retailers agreed more strongly with the factors related to supplier uncertainty, with an average rating of 4.2 compared with 3.4 for non-retailers. There was most divergence about whether farmers were aware of after-sales service by AMS suppliers (retailer 4.5, non-retailer 3.6) and the learning support they will receive from AMS suppliers (retailer 4.0, non-retailer 2.7). UK/Ireland respondents provided the lowest average rating (3.1) to supplier factors and, along with Dutch respondents, gave a low rating for farmer knowledge of learning support they would receive from AMS providers (2.8 UK/Ireland, 2.6 Netherlands). The most agreement for individual factors were from Danish respondents who felt there was a ready supply of AMS units (4.7) and Dutch respondents who agreed strongly that farmers were aware of the technical service they would receive (4.8).
Political uncertainty
The factors associated with political uncertainty were rated the lowest of all the uncertainty areas at an average rating of 2.5. There was little difference between retailers (2.4) and non-retailers (2.6). The lowest rated individual factor was F26, that public sector financial incentives have increased AMS adoption. On average, respondents from most countries disagreed that current regulations acted to make farming with AMS easier (F23), in particular USA (1.7) and UK/Ireland (2.5). Respondents across role and different countries were all neutral as to whether farmers were aware of regulations relating to AMS use (F24). While respondents from Denmark agreed (3.6) that the dairy community was aware of future regulations related to AMS (F28), respondents from all other countries disagreed (2.0–2.5). On average, respondents from USA disagreed the most (2.2) with the factors related to political uncertainty.
Discussion
In this paper, we aimed to understand the impact of innovation uncertainty adoption of AMS internationally and propose lessons for developing institutional knowledge and effective networks of practice in emerging smart farming innovation systems.
Major Themes Associated With Predicted AMS Adoption
Survey responses in this current study indicated a range of influences on potential AMS adoption. While historical adoption had been negatively influenced by financial factors such as low milk prices and the 2008 financial crisis, there were examples of technological uncertainty affecting early installations and perceptions of these issues by other farmers. Respondents identified some examples of decommissioning, which created a level of uncertainty in the local farming population about the suitability of AMS. Farmers in early adopting countries (e.g., The Netherlands and Denmark) had some issues with learning to use AMS successfully, in some cases farmer skills and perceptions did not fit with AMS. The historical adoption factors associated with AMS identified in our study, such as reducing total farm labor, reducing hours spent milking, more family time, and reducing physical work, are supported by several authors (de Koning, 2010; Jacobs and Siegford, 2012; Hansen, 2015; Rodenburg, 2017; Vik et al., 2019).
In our study, we examined the predicted AMS adoption and the potential reasons for this among a group of experts. Almost all respondents predicted increased AMS adoption, with almost a quarter predicting a rapid increase. Understandably, this expectation of a rapid increase was higher among those selling AMS technology. Analyzed by country, respondents expected a slower adoption rate in Denmark and The Netherlands, when compared with Canada and USA. The data presented in Table 1 mostly agree with these predictions. Danish AMS installations have not increased from 2010 to 2018, and in recent years have actually decreased. However, installations in The Netherlands and Sweden doubled from 2010 to 2018, and there have been dramatic increases in countries such as Germany (650%), Canada (475%), and Norway (250%)—albeit off a relatively low 2010 base. Of the emerging markets in our survey, USA still shows a low level of adoption, with an estimated 3% of farms. This could be primarily due to the farm system types and sizes employed there, and relatively low labor costs. The number of large farms has previously been highlighted as a barrier to AMS adoption in USA by Jacobs and Siegford (2012), and increased farm size is also having an impact in countries such as Denmark and Norway (Sigurdsson et al., 2019; Vik et al., 2019). However, the information presented in our survey adds weight to the need for alternative automated milking approaches for larger farm systems, such as robotic rotaries and stand-alone robotic cup attachment systems that work in rotary parlors. We further explore the potential reasons for the different adoption trajectories in section The Impact of Uncertainty on AMS Adoption below.
The Impact of Uncertainty on AMS Adoption
Respondents were most positive toward factors associated with technological and supplier uncertainty. This indicates that at the time of the survey, the AMS technology was relatively mature and reliable, but in some countries, the knowledge associated with AMS use in different farm systems was not so developed. In this study, respondents felt that farmers were well-supported when making AMS investment decisions but were not always certain of the implications AMS had on farm systems challenges such as expanding their herd size, or reverting back to a conventional milking system (Hansen, 2015). The survey results therefore provide insights into the development and potential future adoption of AMS, including the need for greater certainty around issues of technological lock-in (where farmers face difficulties reversing technology investment decisions) and the forms of after-sales support required (for example, managing the farm systems changes related to AMS use).
There was most potential uncertainty around political factors, with ratings lowest among USA respondents. The political environment can have a large impact on innovations such as AMS through even apparently minor regulations or policies. One example highlighted in comments by respondents was food safety regulations in Europe requiring a person to be present at milking, which was not feasible under the 24 h milking cycle of AMS. Altering these regulations can take considerable effort and can act to discourage farmers. Other institutional arrangements can also be affected, for example, herd test protocols that require two milk samples at 12 h intervals have had impacts on the ability of AMS farmers to participate in herd improvement schemes (Eastwood et al., 2017b). Political factors have also been highlighted as driving industry structural change that can impact smart farming adoption such as AMS (Vik et al., 2019).
Respondents perceived a lack of awareness among farmers as to future regulations that may have an impact on AMS use and felt that farmers were moderately aware of the current regulations that are related to AMS use. There was a strong perception that current regulations did not act to make farming with AMS easier. There was also a perception that financial incentives at an industry level had not played a role in the uptake of AMS. In terms of the role of public and industry good organizations, respondents identified a lack of industry-level extension programs related to AMS use but perceptions were mixed as to whether there was a role for the dairy industry or public organizations in the learning support space. Smart farming technology is dominated by commercial interests, which has been shown to have implications for private and public R&D roles in terms of supporting adoption (Eastwood et al., 2017b; Klerkx et al., 2019). This tension was also highlighted by respondents in factors related to competitive uncertainty, with an indication that sourcing independent advice on AMS technology was difficult for farmers in the emerging markets of Canada, USA, and the UK. Farmer uncertainty about adopting AMS and the lack of service providers for technical support were also found to be an issue in USA by Jacobs and Siegford (2012).
Responses related to supplier uncertainty highlighted differences between retailers and non-retailers. Retailers were much more positive that farmers were receiving good technical and learning support. The ratings showed that while farmers could be certain about the extent of technical support they will receive, they may be less aware of the learning support available from their retailer. This retailer focus on technical support is common in the smart farming domain (Eastwood et al., 2016). However, this can be compensated by farmers having access to AMS farming system advice from other agents in the innovation system, but these skills take time to build. For example, a network of farm system advisors took two decades to develop in The Netherlands, primarily because a certain AMS market size was needed to make it worthwhile for advisory firms to upskill (Eastwood et al., 2017b).
When investing in smart farming technology, farmers need a clear value proposition and business case, which in turn requires more transparent sharing of financials and robot performance by farmers and retailers (Rojo-Gimeno et al., 2019). In terms of our factors related to resource uncertainty, respondents perceived relative certainty for farmers accessing finance for AMS, but that ascertaining the depreciation value of the technology was more difficult. This was more of an issue in the emerging markets, as the mature markets had more knowledge of AMS performance, and potentially more experience with use of secondhand AMS units. Additionally, in some countries, the uncertainty around future milk prices could impair the ability to create robust investment cases for smart technologies. It is interesting to note that AMS retailers indicated much more confidence in predicting milk prices than non-retailers, potentially indicating undue optimism in the sales process.
Usefulness of the Perceived Uncertainty Framework
The framework of Meijer et al. (2007b) provided a good lens with which to look at the AMS issues internationally. While most of the factors of uncertainty were relevant to the “farmer as entrepreneur” perspective adopted in our analysis, some of the factors relate better to other actors in the network. For example, consumer uncertainty relates more to technology providers and their uncertainty of the farmers' needs or to consultants and their uncertainty whether there is a business case for them to become involved. Use of the framework highlighted clear differences between technology retailers in specific aspects, and therefore, the framework could be used with other smart farming technologies to assess where potential issues occur between retailer and non-retail actors.
Difficulties we encountered with an empirical investigation using the framework factors involved first determining robust factors that related to each area of uncertainty. While we developed these within the research team, and tested them in a pilot, they would benefit from further refinement subsequent to this study. Additionally, with such survey methods in a niche research area, achieving sufficient responses is difficult. In our study, we concentrated on results from countries with the most respondents, but further empirical studies using this framework would benefit from greater targeting of respondents.
Implications for Minimizing Uncertainty Related to Smart Technologies in Agriculture
This current study indicates some lessons for the configuration of smart farming innovation systems. In the case of AMS, the dominant forms of uncertainty uncovered across all respondents were in the resource and political domains, a finding supported by another study of uncertainty in technological start-ups (Tomy and Pardede, 2018). In particular, we identify a need for further discussion regarding the role of private providers of advice to farmers, and the related role of public or industry good AMS support programs. Our study indicates that development of commercial roles for consultants in providing advice to AMS farmers took some time to occur in the established markets in Europe, a finding supported by Eastwood et al. (2017b). In relation to smart farming technologies generally, the potential role of farm advisors in reducing innovation uncertainty has been highlighted in other studies (Ayre et al., 2019; Eastwood et al., 2019). There exists a significant opportunity for farm advisors to support farmers, so they get the most from their technology investments, requiring more focus from public R&D in the smart farming domain.
Our survey results highlighted a difference in perceptions between technology retailers and other actors in the technological innovation system, particularly around factors related to supplier and consumer uncertainty. The smart farming domain is dominated by private R&D (Eastwood et al., 2017b; Klerkx et al., 2019) and therefore the pressures of being first to market, providing a return on agtech venture capital, and achieving sufficient sales in a niche market can lead to ambitious marketing. This may result in development of smart technologies without a full understanding of market (farmer) needs (i.e., consumer uncertainty) and lack of focus on after-sales service that helps farmers integrate the technology into their farming system context (i.e., supplier uncertainty). It is therefore vital that commercial interests, farm advisors, and public R&D actors foster a collaborative approach to development and support of smart farming technologies (Ayre et al., 2019; Phillips et al., 2019). The need for collaborative approaches is especially the case where technologies are brought together in platforms (e.g., via artificial intelligence) to solve dynamic and complex agricultural problems (Hermans et al., 2019).
We identified an impact of immature AMS technology being marketed to farmers, and AMS technology being sold to farmers who did not have the capability or mindset to adapt their farm systems to suit. This resulted in instances of decommissioning, or reverting to conventional milking technology, and had a subsequent impact on farmer (and advisor) confidence in the technology. This experience highlights an important consideration for smart farming innovation uncertainty. Agricultural NGOs and governments are increasingly viewing smart farming as a tool for improvements in productivity and sustainability of agriculture. However, our study highlighted the potential impact of negative experiences associated with new technologies from farmers who struggle with the adaptation process as such occurrences may act to stall the uptake of smart farming technologies. If public policy organizations are to realize the desired impacts of smart farming technology, there needs to be greater focus on understanding where (and which) technologies can have an actual impact on farm (Shepherd et al., 2018), as opposed to technologies that only create greater farmer distrust and uncertainty (Jakku et al., 2018; Klerkx et al., 2019).
Limitations of the Approach
The approach used in this paper involved an online survey with a targeted snowball method where domain experts were first identified and then asked to distribute the survey among their networks. The target population (researchers and professionals with knowledge of AMS) was relatively small and dominated by commercial technology and service providers who are often difficult to access in research projects.
This research represented an exploratory approach to use the innovation uncertainty framework to describe major influences on AMS adoption in different countries. The questions were developed by the project team, and tested in a pilot survey, and therefore represent a best design of the appropriate questions. However, the questions could be open to interpretation of individual participants.
Conclusions
In this paper, the concept of perceived uncertainty in innovation systems was used to examine the adoption of automated milking systems, a smart farming technology. The major drivers for farmers adopting AMS included reducing total farm labor, hours spent milking, and the amount of physical work, while also having more family time. Adoption was perceived to be negatively impacted by the cost of technology and issues around future herd expansion, difficulty obtaining finance, fit with farm system, and negative experiences of other farmers. This study adds to limited literature focused on empirical analysis of the role of uncertainty in using factors associated with perceived uncertainty; we were able to analyze the AMS innovation system across different countries and institutional contexts and use this to determine implications for smart farming technology adoption. We highlighted perceived impacts of political uncertainty, and the impact of technological uncertainty around not only immature smart farming technologies but also the on-farm adaptation that such technologies can require. We also suggest that to reduce uncertainty with emerging smart technologies, greater effort is required to foster knowledge development and exchange. In emergent markets for smart farming technologies, there is a public or industry-good role in delivering broad knowledge development and capability building programs focused on key actors such as nutritionists, veterinarians, banking finance representatives, and agricultural consultants.
Data Availability Statement
Restrictions apply to the datasets: The datasets for this article are not publicly available to protect the anonymity of respondents. Requests to access the datasets should be directed to Callum R. Eastwood, Y2FsbHVtLmVhc3R3b29kQGRhaXJ5bnouY28ubno=.
Ethics Statement
The studies involving human participants were reviewed and approved by Human Ethics Advisory Group—The University of Melbourne. The participants provided their written informed consent to participate in this study.
Author Contributions
Research method and data collection were undertaken by CE. Data analysis, writing, and revision of the manuscript were undertaken equally by CE and AR.
Funding
This research was conducted as a part of a program funded by Dairy Australia (Melbourne, Australia) through project UM13556 at The University of Melbourne. Writing of the paper was funded by the dairy farmers of New Zealand through DairyNZ Inc. (Hamilton, New Zealand), contract WEN1802 (Systems for People).
Conflict of Interest
The authors declare that this study received funding from Dairy Australia and DairyNZ Inc. These funders were not involved in the study design, collection, analysis, interpretation of data, the writing of this article, or the decision to submit it for publication.
Acknowledgments
The authors would like to thank those involved in piloting the survey and the AMS retailers, consultants, and researchers who took the time to answer the survey.
References
Ayre, M., Mc Collum, V., Waters, W., Samson, P., Curro, A., Nettle, R., et al. (2019). Supporting and practising digital innovation with advisers in smart farming. NJAS-Wagen. J. Life Sci. 90:100302. doi: 10.1016/j.njas.2019.05.001
Barkema, H. W., von Keyserlingk, M. A. G., Kastelic, J. P., Lam, T. J. G. M., Luby, C., Roy, J. P., et al. (2015). Invited review: changes in the dairy industry affecting dairy cattle health and welfare. J. Dairy Sci. 98, 7426–7445. doi: 10.3168/jds.2015-9377
CDIC (2019). Dairy Barns by Type in Canada. Canadian Dairy Information Centre. Available online at: https://www.dairyinfo.gc.ca/index_e.php?s1=dff-fcil&s2=farm-ferme&s3=db-el (accessed December 1, 2019).
de Koning, K. (2010). “Automatic milking - common practice on dairy farms,” in The First North American Conference on Precision Dairy Management (Toronto, ON).
Eastwood, C. R., Ayre, M., Nettle, R., and Dela Rue, B. (2019). Making sense in the cloud: farm advisory services in a smart farming future. NJAS-Wagen. J. Life Sci. 90:100298. doi: 10.1016/j.njas.2019.04.004
Eastwood, C. R., Chapman, D. F., and Paine, M. S. (2012). Networks of practice for co-construction of agricultural decision support systems: case studies of precision dairy farms in Australia. Agric. Syst. 108, 10–18. doi: 10.1016/j.agsy.2011.12.005
Eastwood, C. R., Jago, J. G., Edwards, J. P., and Burke, J. K. (2016). Getting the most out of advanced farm management technologies: roles of technology suppliers and dairy industry organisations in supporting precision dairy farmers. Anim. Prod. Sci. 56, 1752–1760. doi: 10.1071/AN141015
Eastwood, C. R., Klerkx, L., Ayre, M., and Dela Rue, B. (2017a). Managing socio-ethical challenges in the development of smart farming: from a fragmented to a comprehensive approach for responsible research and innovation. J. Agric. Environ. Ethics Online Early. 32, 741–768. doi: 10.1007/s10806-017-9704-5
Eastwood, C. R., Klerkx, L., and Nettle, R. (2017b). Dynamics and distribution of public and private research and extension roles for technological innovation and diffusion: case studies of the implementation and adaptation of precision farming technologies. J. Rural Stud. 49, 1–12. doi: 10.1016/j.jrurstud.2016.11.008
Gargiulo, J. I., Eastwood, C. R., Garcia, S. C., and Lyons, N. A. (2018). Dairy farmers with larger herd sizes adopt more precision dairy technologies. J. Dairy Sci. 101, 5466–5473. doi: 10.3168/jds.2017-13324
Hansen, B. G. (2015). Robotic milking-farmer experiences and adoption rate in Jæren, Norway. J. Rural Stud. 41, 109–117. doi: 10.1016/j.jrurstud.2015.08.004
Hermans, F., Geerling-Eiff, F., Potters, J., and Klerkx, L. (2019). Public-private partnerships as systemic agricultural innovation policy instruments – assessing their contribution to innovation system function dynamics. NJAS-Wagen. J. Life Sci. 88, 76–95. doi: 10.1016/j.njas.2018.10.001
Higgins, V., Bryant, M., Howell, A., and Battersby, J. (2017). Ordering adoption: materiality, knowledge and farmer engagement with precision agriculture technologies. J. Rural Stud. 55, 193–202. doi: 10.1016/j.jrurstud.2017.08.011
Hogenkamp, W. (2018). Groei Melkrobot Verschilt Sterk Per Land. Melkvee100plus. Available online at: https://www.melkvee100plus.nl/Artikelen/Automatisering/2018/4/Groei-melkrobot-verschilt-sterk-per-land-277025E/?intcmp=navigatiepijlen (accessed April 29, 2018).
Jacobs, J. A., and Siegford, J. M. (2012). Invited review: the impact of automatic milking systems on dairy cow management, behavior, health, and welfare. J. Dairy Sci. 95, 2227–2247. doi: 10.3168/jds.2011-4943
Jago, J., Eastwood, C. R., Kerrisk, K., and Yule, I. (2013). Precision dairy farming in Australasia: adoption, risks and opportunities. Anim. Prod. Sci. 53, 907–916. doi: 10.1071/AN12330
Jakku, E., Taylor, B., Fleming, A., Mason, C., Fielke, S., Sounness, C., et al. (2018). “If they don't tell us what they do with it, why would we trust them?” Trust, transparency and benefit-sharing in Smart Farming. NJAS-Wagen. J. Life Sci. 90:100285. doi: 10.1016/j.njas.2018.11.002
Khanal, A. R., Gillespie, J., and MacDonald, J. (2010). Adoption of technology, management practices, and production systems in US milk production. J. Dairy Sci. 93, 6012–6022. doi: 10.3168/jds.2010-3425
Klerkx, L., Aarts, N., and Leeuwis, C. (2010). Adaptive management in agricultural innovation systems: the interactions between innovation networks and their environment. Agric. Syst. 103, 390–400. doi: 10.1016/j.agsy.2010.03.012
Klerkx, L., Jakku, E., and Labarthe, P. (2019). A review of social science on digital agriculture, smart farming and agriculture 4.0: new contributions and a future research agenda. NJAS-Wagen. J. Life Sci. 90:100315. doi: 10.1016/j.njas.2019.100315
Kuehne, G., Llewellyn, R., Pannell, D. J., Wilkinson, R., Dolling, P., Ouzman, J., et al. (2017). Predicting farmer uptake of new agricultural practices: a tool for research, extension and policy. Agric. Syst. 156, 115–125. doi: 10.1016/j.agsy.2017.06.007
Meijer, I. S. M., Hekkert, M. P., and Koppenjan, J. F. M. (2007a). How perceived uncertainties influence transitions; the case of micro-CHP in the Netherlands. Technol. Forecast. Soc. Change. 74, 519–537. doi: 10.1016/j.techfore.2006.02.007
Meijer, I. S. M., Hekkert, M. P., and Koppenjan, J. F. M. (2007b). The influence of perceived uncertainty on entrepreneurial action in emerging renewable energy technology; biomass gasification projects in the Netherlands. Energy Policy. 35, 5836–5854. doi: 10.1016/j.enpol.2007.07.009
Meijer, I. S. M., Hekkert, M. P., Faber, J., and Smits, R. (2006). Perceived uncertainties regarding socio-technological transformations: towards a framework. Int. J. Foresight Innovat. Policy 2, 214–240. doi: 10.1504/IJFIP.2006.009316
Meskens, L., Vandermersch, M., and Mathijs, E. (2001). Implication of the Introduction of Automatic Milking on Dairy Farms Literature Review on the Determinants and Implications of Technology Adoption. Leuven: Departement of Agricultural and Environmental Economics, Katholieke Universiteit.
Morriss, S., Massey, C., Flett, R., Alpass, F., and Sligo, F. (2006). Mediating technological learning in agricultural innovation systems. Agric. Syst. 89, 26–46. doi: 10.1016/j.agsy.2005.08.002
Phillips, P. W. B., Relf-Eckstein, J.-A., Jobe, G., and Wixted, B. (2019). Configuring the new digital landscape in western Canadian agriculture. NJAS-Wagen. J. Life Sci. 90:100295. doi: 10.1016/j.njas.2019.04.001
Reed, E. (2018). The Cow-Milking Robots Keeping Small Farms in Business. The Atlantic. Available online at: https://www.theatlantic.com/business/archive/2018/10/young-dairy-farmers/567937/ (accessed October 11, 2018).
Rodenburg, J. (2017). Robotic milking: technology, farm design, and effects on work flow. J. Dairy Sci. 100, 7729–7738. doi: 10.3168/jds.2016-11715
Rojo-Gimeno, C., van der Voort, M., Niemi, J. K., Lauwers, L., Kristensen, A. R., and Wauters, E. (2019). Assessment of the value of information of precision livestock farming: a conceptual framework. NJAS-Wagen. J. Life Sci. 90:100311. doi: 10.1016/j.njas.2019.100311
Roper, S., and Tapinos, E. (2016). Taking risks in the face of uncertainty: an exploratory analysis of green innovation. Technol. Forecast. Soc. Change. 112, 357–363. doi: 10.1016/j.techfore.2016.07.037
Rose, D. C., Sutherland, W. J., Parker, C., Lobley, M., Winter, M., Morris, C., et al. (2016). Decision support tools for agriculture: towards effective design and delivery. Agric. Syst. 149, 165–174. doi: 10.1016/j.agsy.2016.09.009
Rutten, C. J., Steeneveld, W., Oude Lansink, A. G. J. M., and Hogeveen, H. (2018). Delaying investments in sensor technology: the rationality of dairy farmers' investment decisions illustrated within the framework of real options theory. J. Dairy Sci. 101, 7650–7660. doi: 10.3168/jds.2017-13358
Shephard, R. (2004). Automatic Milking System (AMS) Research Applicable to the Australian Dairy Industry. Ellinbank, VIC: National Milk Harvesting Centre.
Shepherd, M., Turner, J. A., Small, B., and Wheeler, D. (2018). Priorities for science to overcome hurdles thwarting the full promise of the ‘digital agriculture' revolution. J. Sci. Food Agric. doi: 10.1002/jsfa.9346. [Epub ahead of print].
Sigurdsson, S., Hettasch, T., Gretarsson, S., Kromann, H., Manninen, E., Nyman, K., et al. (2019). “Development of AMS in the Nordic countries, 1998-2018,” in IDF 2019 Mastitis Conference (Denmark: Copenhagen).
Spielman, D., and von Grebmer, K. (2006). Public–private partnerships in international agricultural research: an analysis of constraints. J. Technol. Transf. 31, 291–300. doi: 10.1007/s10961-005-6112-1
Svennersten-Sjaunja, K. M., and Pettersson, G. (2008). Pros and cons of automatic milking in Europe. J. Anim. Sci. 86, 37–46. doi: 10.2527/jas.2007-0527
Tomy, S., and Pardede, E. (2018). From uncertainties to successful start ups: a data analytic approach to predict success in technological entrepreneurship. Sustainability 10:602. doi: 10.3390/su10030602
Tse, C., Barkema, H. W., DeVries, T. J., Rushen, J., and Pajor, E. A. (2017). Effect of transitioning to automatic milking systems on producers' perceptions of farm management and cow health in the Canadian dairy industry. J. Dairy Sci. 100, 2404–2414. doi: 10.3168/jds.2016-11521
Keywords: automatic milking systems, innovation uncertainty, dairy, smart farming, advisors
Citation: Eastwood CR and Renwick A (2020) Innovation Uncertainty Impacts the Adoption of Smarter Farming Approaches. Front. Sustain. Food Syst. 4:24. doi: 10.3389/fsufs.2020.00024
Received: 14 December 2019; Accepted: 24 February 2020;
Published: 20 March 2020.
Edited by:
Matt Bell, University of Nottingham, United KingdomReviewed by:
David Rose, University of Reading, United KingdomYong Liu, Hunan Academy of Agricultural Sciences (CAAS), China
Copyright © 2020 Eastwood and Renwick. This is an open-access article distributed under the terms of the Creative Commons Attribution License (CC BY). The use, distribution or reproduction in other forums is permitted, provided the original author(s) and the copyright owner(s) are credited and that the original publication in this journal is cited, in accordance with accepted academic practice. No use, distribution or reproduction is permitted which does not comply with these terms.
*Correspondence: Callum R. Eastwood, Y2FsbHVtLmVhc3R3b29kQGRhaXJ5bnouY28ubno=