Perceived Benefits of Automation and Artificial Intelligence in the AEC Sector: An Interpretive Structural Modeling Approach
- Department of Quantity Surveying and Construction Management, The Centre of Applied Research and Innovation in the Built Environment (CARINBE), University of Johannesburg, Johannesburg, South Africa
Increasing demand for infrastructure amidst the surge in the urbanization of cities and newly emerging commercial nerves has spurred the need to reinvent and rethink traditional approaches for delivering infrastructure. This has been identified as even more critical given the global drive and discourse on the sustainability of the construction sector and its health and safety performance. Given the potential gains of adopting construction automation and AI in infrastructure delivery, stakeholders’ convincing appreciation of its benefit is vital to its widespread adoption in the AEC sector. This explored and evaluated the critical benefits of integrating automation in construction processes in the architectural, engineering, and construction sector and the use of artificial intelligence (AI) in driving its systems and workflows. The study adopts an interpretive structural modeling approach based on interviews of construction stakeholders in diverse countries to develop a hierarchical model of the interrelationships of the benefits. Furthermore, the Matrice d’Impacts croises-multiplication applique a classement analysis (MICMAC) was used to categorize the benefits. Highlighted perceived benefits such as improved project quality, simplification of construction tasks, workflow improvements, and safety performance, amongst others, were fractionalized into levels. The study’s findings are critical in satisfying a cost-benefit index of adopting automation and AI in the AEC sector. The results provide recommendations on effective approaches pivotal to driving automation and AI for practice and research. This is of further importance to construction stakeholders, policymakers, and local authorities in building strategies and roadmaps for proper integration of these systems and widespread adoption.
1 Introduction
Challenges such as declining construction productivity (Skibniewski and Hendrickson, 1990; Cai et al., 2020), increasing scarcity of skills, high incidence of construction hazards, and need to improve productivity and ensure quality project delivery have spurred research and development of technologies to give the built sector a competitive edge and improve infrastructure delivery. Central to these emerging technologies is the design and application of automation and AI in the built industry to improve construction tasks, workflows, and processes (Mamela et al., 2020; Lee et al., 2022). The difference touted by the adoption of automation and AI is in the simplicity of construction tasks, repetitiveness that relieves human stress and strain, improvement in construction safety, and enhanced productivity, amongst others (Darko et al., 2020; Abioye et al., 2021).
Despite the benefits of automation and AI, awareness and interest in adoption are low in the industry (Shukla et al., 2019; Cubric, 2020). Major reasons for this have been attributed to the perception of automation and AI in the industry as hype rather than practical usefulness, the emerging nature of the systems, technological requirement, and underestimating their benefits (Trujillo and Holt, 2020; Bademosi and Issa, 2021). Naghshbandi et al. (2021) attributed this perception to inadequate awareness of automation and AI potentials, further confounded by low awareness of the advances and successes these innovations have recorded in the built sector. Emaminejad et al. (2021) argued that advancing knowledge on the benefits of design and development of AI and automation holds immense opportunities in attracting the interests of industry practitioners for adoption considerations, generating more discussion, motivating further R&D funding to advance development in AI, and informing policy decisions. This view is supported by Prentice et al. (2020), who noted that low adoption has often been associated with unconvincing dialogue on the system’s gains despite its huge potential. Despite the diverse impactful and critical perceived benefits, the uptake of automation and AI in construction in industry and research is still relatively low (Pan et al., 2020; Pan and Zhang, 2021). These studies collectively identify the critical role of understanding the benefits of a system in its adoption, consequently requiring a broader perspective to highlight these benefits.
Olawumi and Chan (2019) showed that in the quest to advance the adoption of innovative systems, benefits must be explored to avail evidential support and justify adoptive decision-making in construction organizations. As the danger of these unchecked perceptions lies in its potential to create a technology with averse disposition toward automation and AI adoption in the industry, one of the most significant current discussions about automation and AI is justifying its cost-use benefits. Following the perspectives mentioned previously, these indicate a need to contribute to the gap in knowledge by identifying and assessing the practical benefits availed to stakeholders through a different approach from other studies and grounding the identified benefits on expert perspectives. To unravel and bring to the fore the critical perceived benefits of automation and AI in construction, it is imperative to aggregate the benefits to avail evidential backing to support clients and policymakers in automation and AI adoption and implementation process. Therefore, the study bridges the gap between knowledge and practice by highlighting critical benefits from the perspectives of industry professionals and stakeholders. Furthermore, the study attempts to categorize the highlighted automation and AI benefits while also recommending strategies to advance the development and adoption of automation and AI in construction imperative for policy, practice, research, design, and curriculum development.
The study’s writing is organized with Section 1 introducing automation and AI in the built environment, while Section 2 presents a succinct overview of current trends in automation and AI juxtaposed with the historical background and benefits identified from diverse literature. Section 3 discusses the methodological approach, interpretive structural modeling, and analysis methods, while Section 4 addresses the findings and Section 5 concludes the study.
2 Theoretical Background
2.1 Automation and Artificial Intelligence in Construction
The term “AI” came about in 1956 during a workshop held at Dartmouth College (Salehi and Burgueño, 2018) and has advanced to the foundation of the interaction of diverse disciplines such as cybernetics, computer science, and information theory. Research interests, design, and development in construction automation and AI has commenced since the 1960s, with Japan recording critical actions to advance automation studies (Skibniewski and Hendrickson, 1990; Manuel et al., 2019). Much of the literature since the 1990s has focused on automation and robotics for building work, mobility and navigation, expert systems, automating concrete placement and automation in material handling, Earth and foundation work, building inspection, and maintenance and tunneling work (Skibniewski and Hendrickson, 1990; Bademosi and Issa, 2021). Only in the past two decades, as literature on automation and AI considerably began addressing sensor data acquisition and processing (Bock and Christos, 2016; Darko et al., 2020), engineering domains in cybernetics, computer vision, pattern recognition, deep learning (Salehi and Burgueño, 2018), human–robot teams (Karimidorabati et al., 2016), automated service technologies (Fernandes and Oliveira, 2021), automation for steel beam assembly (Jung et al., 2013), automation for GPS guidance, automating existing heavy equipment, automating kits, automating site preparation (Melenbrink et al., 2020a), automating earthwork and substructure tasks (Melenbrink et al., 2020b; Naghshbandi et al., 2021), autonomous installation of check dams, and automated obstruction detection and classification using Lidar (Aghimien et al., 2019; Gargoum and Karsten, 2021) were developed. Development in the aforementioned areas has stimulated automation and AI’s applicability to processes and tasks in the built environment.
However, the adoption of automation and AI in the AEC sector relies heavily on stakeholders’ perception of their prospects in aiding productivity and achieving safe infrastructure delivery. Studies such as Manuel et al. (2019) categorized construction automation as off-site prefabrication systems, on-site automated and robotic systems, drones and autonomous vehicles, and exoskeletons. Also, Chui and Mischke (2019) identified construction automation technologies as machines able to execute built environments, tasks, and processes through robotic systems, doing them faster, repetitively, and better with little to no human intervention. Bock and Christos (2016) further statedit as a new set of technologies and processes that will fundamentally change the whole course and idea of construction. Along this line, Ruggiero et al. (2016), Mohapatra and Kumar (2019), and Bademosi and Issa (2021) pinpoint distinct characteristics of automation systems. However, previous studies have not established the collective benefits automation and AI offer stakeholders in justifying the high investment cost required for adoption. With the introduction of new systems into the built sector, Salehi and Burgueño (2018) argue that clarity must be offered on how beneficial these systems are to stakeholders to solve engineering problems in the AEC sector.
While adoption of automation and AI in the AEC sector has been relatively low, the emerging use of automation in the built sector is seen in commercial bricklaying robots, building and delivery drones, robotics for monitoring and inspection, and automated bulldozers, amongst various others (Oesterreich and Teuteberg, 2016). With improving developments such as knowledge-based systems in AI directed toward machine decision-making based on existing knowledge from domain expert knowledge, past cases or experiences, or other relevant sources with merits of increasing productivity. It is valuable for clients to understand and appreciate the value automation and AI offers to encourage adoption. Other value propositions such as the efficiency of easy access and interactions with large requisite domain knowledge (Abioye et al., 2021) and creating computational models that mimic the linguistic capabilities of human beings with AI are essential in future industrialized construction projects. Along these lines, the application of optimized decision-making systems driven by AI is essential in resource and waste management, value-driven services, supply chain management, health and safety, AI-driven construction contract analytics, voiceuser interfaces, and AI-driven audit systems for construction financials (Dagnaw, 2020; Abioye et al., 2021).
Considering all these benefits and the relatively small body of literature concerned with aggregating the benefits of this emerging development, it is useful to help support stakeholders’ decision-making in adopting innovative systems to improve construction productivity. It is imperative to highlight the benefits of these advancements. The issue has grown in importance in light of recent debate highlighting strategic technology proliferation in the AEC sector as being underpinned by stakeholders understanding its critical benefits (Chen et al., 2018). The following section highlights the benefits of automation and AI in construction.
2.2 Benefits of Automation and Artificial Intelligence in Construction
Despite the nascent research stage in automation and AI in construction, studies such as Lu et al. (2012) and Bademosi and Issa (2021) have noted significant output in these technologies, improving construction productivity, efficiency, and quality of infrastructure. Furthermore, their adoption is central to reducing the high incidence of waste generated on-site, achieving better quality in construction project delivery, improving construction workflow and productivity, and achieving sustainable cost in the long term (Akinradewo et al., 2018; Chowdhury et al., 2019; Manuel et al., 2019; Dwivedi et al., 2021). Comprehensive benefits are presented in Table 1, and aggregated benefits are presented in Table 2.
3 Methods
The aim of the study was to highlight the benefits of automation and artificial intelligence through the interpretive structural modeling approach as adopted in previous built environment studies (Mathiyazhagan et al., 2013; Shen et al., 2016; Wuni and Shen, 2019; Saka and Chan, 2020; Eshun and Chan, 2021; Obi et al., 2021; Shoar et al., 2021). To date, diverse methods have been adopted and introduced to measure the benefits of innovative systems. The interpretive structural modeling approach is a well-established method given its strength in studying complex system dynamics, such as adopting innovative systems (e.g., automation and AI). It was decided that the best method to adopt for this study was the ISM approach as it is beneficial in study areas with few experts. This is considered appropriate given the few experts in construction automation and AI. The survey approach was not considered as it would have required sufficient and valid responses which would not be achievable given few numbers of experts in the area (Shoar and Chileshe, 2021). As identified by Saka & Chan (2020), its reliance on expert experience and quality of feedback rather than quantity makes ISM practical and reliable, especially in emerging study areas with low expertise. Therefore, the study adopts a qualitative three-stage approach integrating variables identified from extant literature and expert perspectives. The three-stage approach is based on the system prescribed by Saka and Chan (2020) and Eshun and Chan (2021).
3.1 ISM Research Process
The ISM research approach is conducted in three stages; stage 1 involves identifying the benefits of automation and AI in the built industry. To accumulate comprehensive benefits from the literature, the study reviewed published materials from Scopus, Web of Science, and Google Scholar with considerations for all publications published in English to adequately assess the perceived benefits recorded by built professionals in construction automation and AI and avoid bias (Saka and Chan, 2020).
In stage II, the identified benefits were aggregated from the literature and presented to three experts with over a decade of experience to check the validity, clarity, and representativeness of the factors, as shown in Table 2. The ISM approach is advanced in developing interconnection matrices in structural modeling (Warfield, 1974). The system is proposed to utilize the experience and knowledge of experts in decomposing complex systems into multiple subsystems (Shen et al., 2016; Saka and Chan, 2020). Thereby quality of feedback from surveyed experts is primary in the approach to appropriate the structure of the relationship between the models. Thus, the ISM method is conducted with few knowledgeable and experienced experts (Ravi and Shankar, 2005; Saka and Chan, 2020). As adopted in other built industry studies such as Shoar et al. (2021) and Obi et al. (2021), they have been used primarily for systems with little expertise and emerging discussions to gain from the knowledge of experts in the field. The research process is presented in Figure 1.
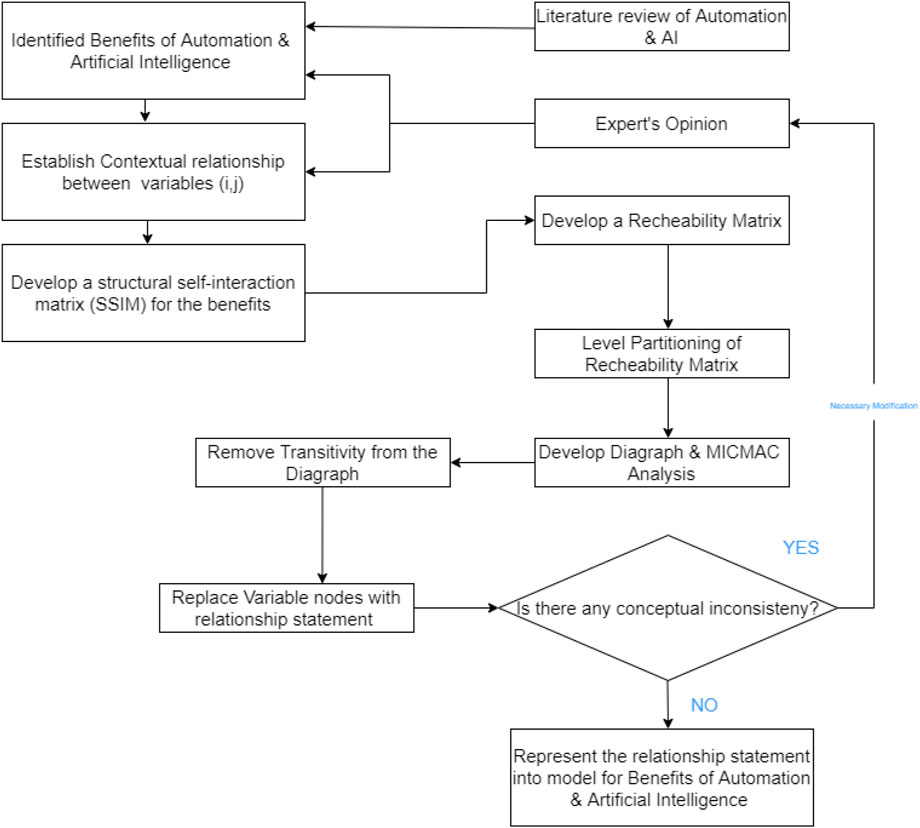
FIGURE 1. ISM methodology as adapted from Mandal and Deshmukh (1994).
The ISM approach is then followed by establishing the hierarchical levels of the factors from the reachability and intersection values. The driving power and the dependence-power of the highlighted factors are then used in building the “Matrice d’Impacts croises-multiplication applique a classement (MICMAC)” as proposed by (Duperrin and Godet, 1973) and adopted by Shen et al. (2016), Wuni and Shen (2019), and Saka and Chan (2020).
The MICMAC assessment is carried out based on the driving power and dependence power of the aggregated variables and classified into an independent category, linkage category, autonomous category, and dependent category. To organize the variables based on MICMAC, the sum of the horizontal values is measured as the driving power. In contrast, the sum of the vertical values is calculated as the dependence power.
3.2 Interpretive Structural Modeling-Based Analysis
The literature does not agree on nor compels a certain number of experts to participate in the ISM methodology. It does not require many respondents and primarily pays attention to the quality of response (Eshun and Chan, 2021). Previous studies such as Ravi & Shankar (2005) and Debnath & Shankar (2012) presented their findings based on two experts, and five experts were deemed sufficient by Shen et al. (2016) and Liu et al. (2018). Furthermore, Ahuja et al. (2017) adopted seven respondents, and Eshun and Chan (2021) surveyed thirteen experts using ISM to develop a relationship between project risk dynamics in Sino-Africa public infrastructure delivery. In contrast, Saka and Chan (2020) interviewed 16 experts to build a model representing the barriers to BIM adoption in SMEs. Twenty professionals with expertise in automation and AI were invited to participate in the survey, but eleven responses were received. All the participants had a minimum of ten years of experience in their fields. Eligibility criteria that have been included in the study were based on years of industry experience or academic experience in construction automation knowledge areas and/or design. These criteria are well-established in extant studies using ISM in the built environment (Saka and Chan, 2020; Eshun and Chan, 2021; Shoar and Chileshe, 2021; Onososen and Musonda, 2022). A total of three groups of respondents participated in the survey; the first group involved selected academic researchers with minimum of a PhD degree in construction automation and AI industry experts with consulting and contractual experience who have utilized automated systems on-site. The third group involved respondents with experience in automation and AI design and systems. The respondents’ profile is presented in Table 3.
The SSIM survey was responded to by the experts based on causality between the variables; this ensures that the deep-rooted knowledge of experts in the domain is reflected in the model (Sushil, 2018).
The structural self-interaction matrix (SSIM) presents the relationships between benefits of automation and AI through a pairwise comparator in which columns and rows are identified using i and j, respectively.
V, A, X, and O are utilized in signifying the relationship between the benefits of automation and AI. The aggregated benefits from the literature were presented in a matrix and placed on the x- and y-axis to demonstrate the interaction between variables “i” and variables “j”. As adopted from the literature, VAXO signifies:
V: Benefits i influence j, and j does not influence i.
A: Benefits j influence i, and i does not influence j.
X: Benefits i influence j, and j also influences i.
O: Benefits i and j have no links.
3.3 Reachability Matrix
Consequently, the surveyed expert’s response indicated by the VAXO matrix was converted into a binary matrix (1, 0). Conditions to satisfy the binary conversion are as follows:
If the cell (i, j) is V, then cell (i, j) entry is 1 and cell (j, i) entry is 0.
If the cell (i, j) is A, then cell (i, j) entry is 0 and cell (j, i) entry is 1.
If the cell (i, j) is X, then cell (i, j) entry is 1 and cell (j, i) entry is 1.
If the cell (i, j) is O, then cell (i, j) entry is 0 and cell (j, i) entry is 0.
The initial reachability matrix is produced from satisfying the conditions of this rule, and the final reachability matrix is constructed from integrating transitive relations into the initial reachability matrix. Transitivity is checked using the following rule: if variable A is related to B and B is related to C, then A is necessarily related to C (Eshun and Chan, 2021; Obi et al., 2021; Shoar and Chileshe, 2021; Onososen and Musonda, 2022). Table 4 shows the received response from the SSIM survey.
3.4 Final Reachability Matrix
The final reachability matrix analysed after the initial reachability matrix as shown in Table 5 with transitivity incorporated is presented in Table 6.
3.5 Hierarchical Structure
The hierarchy of the factors is extracted from classifying the elements according to the reachability set, antecedent set, and intersection set. The reachability set for a variable “i” involves the benefit itself and other reachable benefits (benefits with 1 in its row on the final reachability matrix). The antecedent matrix for a variable is similar to the benefit itself and other reached benefits (benefits with a value of 1 in its column on the final reachability matrix). The benefits common to the reachability and antecedent set for the benefits is the intersection set.
To check the validity and correctness of the hierarchical structure, Sushil (2018) stated that checking elements identified from the literature are well represented, checking the contextual relationship between the variables and their interpretation showcases the model’s intent. Therefore, emphasis is placed on not only just developing a model but also adequately representing a quality relationship between the variables. Also, in making sure the interpretive logic knowledge base is unbiased, variables with both way relationships in which “i” to “j” is “1” and “j” to “i” is “1” were cross-checked again with the experts to confirm if the relationship goes both ways. This is essential as Sushil (2018) states that a one-way relationship may be stronger, and the other way may be comparatively weak. If there is no unconvincing relationship, the interpretation of the variables is treated as “0” or No. Furthermore, the majority decision was adopted in variables with conflicting relationships.
3.6 Level Partitioning
After the hierarchical structure is developed, level partitioning is carried out to classify the variables based on dependent and independent relationships. Benefits with similar reachability and intersection sets are classified to levels during each iteration of reachability, antecedent, and intersection sets. Following the ISM principle, β3 (improvement in construction health and safety), β5 (simplification of construction tasks), and β9 (automatic self-learning improving processes) have similar reachability and intersection sets and were thus partitioned as Level I as shown in Table 7. The partitioned Level I benefits were removed from the matrix table and repeated until all variables were iterated and finalized at Level VI.
Benefits partitioned in Level I are β3 (improvement in construction health and safety), β5 (simplification of construction tasks), and β9 (automatic self-learning improving processes), based on the similarity of the reachability set to the intersection set.
The steps were conducted to categorize the remaining benefits resulting in variables for Level II as shown in Table 8. β10 (on-site and off-site connectivity), β11 (improved collaboration, data sharing, and communication), β12 (workflow improvements; accuracy, reliability, transparency, and leaner procurement), β13 (improved socio-economic and environmental sustainability), β14 (simplified and improved inspection, monitoring, and control), β15 (optimized project planning and scheduling), and β16 (elimination of material wastage).
After eliminating the identified variables from Level II, the iteration was repeated for Level III as shown in Table 9. The identified categories for Level III are β1 (improvement in construction health and safety) and β4 (mitigation of construction risk).
Similarly, benefits β2 (timely project delivery and cost savings) and β17 (reduction in litigation, claims, and contract dispute) were partitioned to Level IV as shown in Table 10.
Likewise, β6 (newer job opportunities) was identified for Level V based on the similarity of reachability and intersection sets and is presented in Table 11.
Equally, β7 (stakeholders’ engagement and satisfaction) and β8 (competitive advantage) were partitioned based on the similarity between the reachability and intersection sets to yield Level VI categorization as presented in Table 12.
3.7 ISM for Benefits of Automation and Artificial Intelligence
This study seeks to identify the perceived benefits of construction automation and AI in improving infrastructure delivery. The ISM model developed as shown as follows reveals the dominant benefits, and this result is helpful for construction organization stakeholders to make adoption easier by identifying the ranked benefits in the model in line with their specific business objectives and organizational goals. Organizations mostly require cost-use benefits to justify investments in innovative or new systems. The ranked model (Figure 2) reveals the most important and dominant benefits that motivate stakeholders to adopt automation and AI. The model is quite revealing in several ways. Unlike other studies, it reveals the dominant benefits vital for organizational needs in faster infrastructure delivery, which amounts to cost-saving and improved the overall value offered to clients.
Interestingly, stakeholders and competitive advantage fall in Level VI, which signifies that achieving the benefits highlighted in the higher-ranked models already gives the organization an edge competitively. The enhanced infrastructure delivery process further ensures that stakeholders are satisfied. This implies that stakeholders are satisfied in achieving levels 1 to 4 of the ISM hierarchy as their concerns are catered for between these levels.
Second, given the need to increase resilience and responsiveness of infrastructure, especially with emerging shock events, the benefits identified in levels 1 to 5 are accrued to the stakeholders and take into cognizance the social responsiveness of the infrastructure to the needs of users. Therefore, integrating AI aids the design and development of infrastructure that fosters social justice. As shown in Figure 2, the model has revealed order and direction in the complex relationships between beneficial factors of automation and AI in the built environment, aiding in understanding the interrelationships of benefits and their levels of interdependence.
3.8 Matrice d’Impacts Croises-Multipication Applique a Classement Analysis
The MICMAC is adopted to classify the benefits into autonomous, dependent, linkage, and independent categories depending on their dependence and driving power. The highest dependence and driving power value is 17 on the x and y-axis, and the minimum x-axis is 3. Therefore, the axis ranges between 3 and 17 (14 units), and the half is 7, which is used in setting the axis value for the two-dimensional diagram (digraph) as shown in Figure 3.
• Autonomous category: This category incorporates weak driving and weak dependence power benefits. They are removed from the central system and have few connections, but this study has no autonomous values.
• Dependent category: This category incorporates weak driving and strong dependence power. The class is dependent on other benefits and can be achieved by enhancing related benefits. This benefit is a “competitive advantage.”
• Independent category: This category incorporates both strong driving and weak dependence power. They are adjudged as critical benefits and are “automatic self-learning improving processes.”
• Linkage category: This category incorporates both strong driving and dependence power benefits. They impact other benefits and have feedback on themselves. They are “improvement in construction health and safety; timely project delivery and cost savings; improved project quality, operations, and productivity; mitigation of construction risk; simplification of construction tasks; newer job opportunities; stakeholders’ engagement and satisfaction; on-site and off-site connectivity; improved collaboration, data sharing, and communication; workflow improvements (accuracy, reliability, transparency, and leaner procurement); improved socio-economic and environmental sustainability; simplified and improved inspection, monitoring, and control; optimized project planning and scheduling; elimination of material wastage; and reduction in litigation, claims, and contract dispute.”
3.9 Discussion of Findings
Automation and AI hold immense contributions to sustainable infrastructure delivery that is resilient, responsive, and socially just. While interests in automation and AI are not new, with discussions emerging in the 1960s, recent advances in technology have only reignited the push for what is possible with automation and AI in the built industry. While the merits are immense, interests and willingness to adopt and implement are low amongst industry practitioners, policymakers, and academia. Thus, this study intends to bridge available advancement in automation and AI with industrial needs by bringing to the fore critical benefits, and its adoption can contribute in significant and concrete terms to the AEC sector. Benefits collated from extant literature were aggregated and tested with experts knowledgeable in automation and AI, resulting in partitioning the benefits into six levels with outputs represented in a model and digraph using the MICMAC technique. The absence of an autonomous variable signifies that all the benefits are significant, and benefits as autonomous variables do not have much influence on the system.
Level I benefits categorized are improved project quality, operations, and productivity, simplification of construction tasks, and automatic self-learning improving processes. As stated by Akinosho et al. (2020), project delay and dwindling productivity in the built sector have further driven the need to adopt automation and AI to simplify construction tasks and improve quality by executing processes better, faster, more sustainably, and improving processes through self-learning of the automated systems needing little to no human intervention (Karimidorabati et al., 2016; Saidi et al., 2016; Aste et al., 2017).
The model in Figure 2 shows that Level II signifying the second hierarchy in the adoption benefits is reportedly more significant than the other levels. The benefits in this group are on-site and off-site connectivity, improved collaboration, data sharing, and communication. Also, others include workflow improvements (accuracy, reliability, transparency, and leaner procurement), improved socio-economic and environmental sustainability, simplified and improved inspection, monitoring, and control, optimized project planning and scheduling, and elimination of material wastage. These benefits are vital in aiding faster construction time than the conventional construction methods. The essence of this has not been much appreciated until the COVID-19 pandemic that resulted in urgent infrastructure needs to support quarantining but with the gross inability of the built industry to meet the demand within the required time (Alawad and Kaewunruen, 2021; El Jazzar et al., 2021). Infrastructure not provided within the required time leads to loss of opportunities. Therefore, the adoption of automation and AI is imperative for the future industrialized construction workflow and clients’ demands (Oesterreich and Teuteberg, 2016). In heavy engineering works and multiple construction projects with little manpower for monitoring, inspection, and quality assurance, automating the components and processes ensures transparency in the quality of work the system can deliver without fail (Oesterreich and Teuteberg, 2016; Manzoor et al., 2021; Pillai and Matus, 2021). While ensuring that quality is achieved, the system can provide real-time feedback and update on the progress of work and identify clash areas needing prompt attention and quick resolution to avoid delays and compromise quality. Heavy engineering works and the nature of infrastructure development in the AEC sector heavily depend on the quality of information and timeliness in sharing the knowledge and reliability of the data. Adopting construction automation and AI can eliminate redundancy in how information is shared by ensuring smooth collaboration between project participants, transparency, and availability of required information necessary for successful infrastructure development. Moreover, as stated by Oesterreich and Teuteberg (2016), Darko et al. (2020), and Dwivedi et al. (2021), big data analytics can assist construction project managers to handle well-informed decisions based on historical data effectively. As stated by Li et al. (2019), Bogue (2018), and O'Grady et al. (2021), transparency of data in automated systems helps in freely increasing collaboration and trust between parties. Workflow improvement is achieved through increased collaboration and transparency, resulting in accountability and project control (Melenbrink et al., 2020a).
Improvement in construction health and safety and mitigation of construction risk are the third-ranked level categorization in automation and AI benefits. The construction industry is popular for the high incidences of hazards, which has had grave consequences on drastically reducing the ability of the industry to attract new entrants, thereby leading to a dearth of vital skills; with the adoption of automation and AI in virtual safety training, AI hazard avoidance, AI risk maps updated in real-time and communicating impending risks to safety managers with automated safety robotics clearing hazardous paths and fixing safety issues, safety is made more central and efficient. Other applications also see the use of smart glasses or smart helmets and wearable technology that can predict safety risks, douse the hazard preventively, and protect human contact (Oesterreich and Teuteberg, 2016; Melenbrink et al., 2020b; Hansapinyo et al., 2020). Therefore, the findings demonstrate that the primary benefits envisioned for the adoption of automation and AI are that construction safety is significantly enhanced, tasks are made simpler, and processes can self-learn to improve performance.
Level IV is partitioned into timely project delivery and cost savings and reduction in litigation, claims, and contract disputes. Automating equipment and materials tracking through embedded sensors significantly reduces material costs (Oesterreich and Teuteberg, 2016). The automation of construction workflow processes also results in a substantial reduction in labor which cuts costs and, more importantly, removes the void associated with the shortage of skilled workers in the industry (Oesterreich and Teuteberg, 2016; Dwivedi et al., 2021). Despite the introduction of budget delivery and cost management tools, there is still a huge potential for the process to be improved through automation and AI. By decreasing project quality time, reducing material waste, and enabling a collaborative space with an easy flow of communication, sustainable cost management is one of the system’s benefits (Oesterreich and Teuteberg, 2016). With automation and AI incorporating several other technologies, the stakeholders are engaged and satisfied with the project as reporting and monitoring are flexibly available in real-time with cost, time, and schedule information available at their fingertips. With augmented reality, virtual reality and mixed reality in conjunction with mobile devices or wearable computing, construction companies can avail project clients greater insight into the detail and design of a building before it is built and during delivery (Melenbrink et al., 2020a). Level V and Level VI include newer job opportunities, stakeholders’ engagement and satisfaction, and competitive advantage. Construction site analytics improves productivity, integrating IoT sensors and other digital technologies in a smart working environment. AI can also structure a large amount of data in a short time in files, images, and videos, aggregate them in BIM and optimize them for site decision-making. The data are fed into the system for proactive project control on construction sites from predictive analytics. The prevalence of automation technologies in future industrialized construction would create entirely new roles to assimilate and reskill the displaced workers in the industry. The more recent job opportunities include construction AI researchers, trainers, and engineers, while low to medium-skilled workers could serve as trainers and testers for those systems replacing them (International Federation of Robotics (IFR), 2017; Abioye et al., 2021). Activity-driven control has emerged with more interest with proposed algorithms to enable the integration of AI in automated systems in construction so that the designs are prediction-based via learning a model that learns automatically as project and scenario increase, thereby gradually eliminating the need to solely rely on user presence information (Shahandashti et al., 2011; Ahmadi-Karvigh et al., 2019). This also enables the automation to adjust itself to user preferences, thereby helping increase stakeholders’ satisfaction and trust in the system.
Furthermore, cloud-based adoption of real-time project monitoring will ensure transparency by allowing stakeholders to access the performance. This is essential in resolving litigations, contractual claims, and disputes. Automation and AI also avail collaborative working, aiding project participants to collaborate and participate from various locations on a project through document sharing and virtual meetings facilities enabled by cloud computing (Bello et al., 2021). Similarly, Abioye et al. (2021) iterated waste reduction through proactive data-driven approaches, i.e., waste analytics (WA), which minimizes waste through design.
MICMAC analysis and the digraph classified the benefits into autonomous, dependent, linkages, and independent benefits. The dependent category incorporates “competitive advantage” and can be achieved by enhancing related benefits. Independent categories are adjudged as critical benefits. For this study, the critical benefits are “automatic self-learning improving processes,” while linkage categories impact other benefits and have feedback on themselves. They are “improvement in construction health and safety; timely project delivery and cost savings; improved project quality, operations, and productivity; mitigation of construction risk; simplification of construction tasks; newer job opportunities; stakeholders’ engagement and satisfaction; on-site and off-site connectivity; improved collaboration, data sharing, and communication; workflow improvements (accuracy, reliability, transparency, and leaner procurement); improved socio-economic and environmental sustainability; simplified and improved inspection, monitoring, and control; optimized project planning and scheduling; elimination of material wastage; and reduction in litigation, claims, and contract dispute”.
These presented benefits are as per the study’s objectives to present a structured contextual hierarchy of perceived benefits of construction automation and AI. The study’s implications, limitations, and future directions are discussed in the following section.
3.10 Implications of Findings
To assess a realistic evaluation of the impact of innovative systems such as construction automation and AI, particularly toward achieving a resilient and responsive infrastructure delivery, stakeholders must understand its cost-benefit analysis (Boktor et al., 2014). Moreover, Olawumi and Chan (2019) also suggested formulation of structural benefits is essential for organizations to tap the gains derivable from innovative systems such as construction automation and AI. The findings of this study have several important implications for practice and research. The theoretical implication of the ISM lies in decomposing complex systems into specific relationships and overall structures as portrayed in the digraph model. This helps to impose order and direction on the complexity of relationships among the various benefits identified.
The practical implication of these findings is that the identified model of benefits in aiding the adoption of construction automation and AI allows decision-makers to identify cost-effective technological solutions that align with organizational goals and objectives. This is further important given the increase in innovative technologies available in the construction industry and the managerial decisions challenge it brings to firms in deciding best-fit approaches to improve organizational competitiveness. Policymakers looking for critical areas vital to achieving an industrialized construction operation that is resiliently benefited from the structured model of benefits in affirming the importance of the system if widely adopted in the built environment. Some of the issues emerging from these findings motivate researchers’ keen interest to explore and conduct further studies.
3.11 Limitations and Future Studies
Overall, this study fills a gap in the perceived benefits of construction automation and AI by providing structured contextual relationships between the benefits. However, this has not been without limitations opening insights into further studies. First, other traditional approaches for analyzing benefits use mean value, weighted score, and relative importance index, which require eliciting data from a large pool of surveys. Compared to ISM, these methods are weak for studies in emerging systems with few experts with sufficient knowledge and experience and hence were not considered fit for the study. Second, the generalizability of these results is subjected to certain limitations of the ISM approach, for instance, the bias of the experts who decide the benefits in the final ISM model. Since the contextual relationships among the variables always depend on the users’ knowledge and familiarity with the firm, its operations, and its industry, this was controlled by ensuring adequacy in participants’ expertise and majority agreement in selecting contextual relationships between the benefits.
Finally, the ISM approach does not provide the level of influence that each benefit exerts on the other, and future studies can therefore investigate the level of influence of the benefits by adopting social network analysis (SNA) or decision-making trial and evaluation laboratory (DEMATEL). Despite these limitations, it transforms the undefined system models into well-defined models through nominal technique, brainstorming of experts, and affinity diagraming in explaining the contextual relationships among the variables. Future studies could include extending the model in case studies to show real-life applicability. Also, the future improvement could adopt fuzzy ISM or TISM to enhance the binary ISM and can be statistically validated using tools such as structural equation modeling to assign weights to the variables.
4 Conclusion
The primary purpose of this study was to assess the critical benefits of automation and AI in the built industry to advance perceptions on the gains of automation and AI from hype to the practical significance and consequently improve positive disposition to its adoption. A toatl of seventeen benefits were examined through the ISM method and MICMAC technique to categorize them in perceived importance and contribution to the built sector. The ISM approach is primarily championed based on expertise, knowledge, and experience that go into participants deciding on relationships between the variables that create the model. The key benefit of automation and AI from the study is represented as improved project quality, operations and productivity, simplification of construction tasks, and automatic self-learning improving processes. This is consistent with previous studies that have advanced the adoption of automation and AI on the premise of improving construction productivity and achieving sustainable infrastructure delivery. The next level of benefits is categorized as on-site and off-site connectivity, improved collaboration, data sharing, and communication, workflow improvements (accuracy, reliability, transparency, and leaner procurement), improved socio-economic and environmental sustainability, simplified and improved inspection, monitoring, and control, optimized project planning and scheduling, and elimination of material wastage. It is thus recommended that in proposing automation and AI adoption for industry use, important attention be primarily focused on the key benefits highlighted (improved project quality, operations and productivity, simplification of construction tasks, and automatic self-learning improving processes). While adoption might differ from region to region, the gains are similar across project types and locations. The classified and identified benefits motivate construction organizations, project teams, local authorities, and other key stakeholders toward enhancing the uptake of automation and AI in the built sector. Therefore, collaborative efforts between national policymakers, industry stakeholders, and local authorities must be availed to ensure the benefits are achieved.
Data Availability Statement
The datasets presented in this study can be found in online repositories. The names of the repository/repositories and accession number(s) can be found in the article/Supplementary Material.
Author Contributions
AO: conceptualization, writing, data collection, and data analysis; and IM: editing, supervision, and funding.
Funding
This work was supported by collaborative research at the Centre of Applied Research and Innovation in the Built Environment (CARINBE).
Conflict of Interest
The authors declare that the research was conducted in the absence of any commercial or financial relationships that could be construed as a potential conflict of interest.
Publisher’s Note
All claims expressed in this article are solely those of the authors and do not necessarily represent those of their affiliated organizations, or those of the publisher, the editors, and the reviewers. Any product that may be evaluated in this article, or claim that may be made by its manufacturer, is not guaranteed or endorsed by the publisher.
References
Abioye, S. O., Oyedele, L. O., Akanbi, L., Ajayi, A., Davila Delgado, J. M., Bilal, M., et al. (2021). Artificial Intelligence in the Construction Industry: A Review of Present Status, Opportunities and Future Challenges. J. Building Eng. 44, 103299. doi:10.1016/j.jobe.2021.103299
Aghimien, D. O., Aigbavboa, C. O., Oke, A. E., and Thwala, W. D. (2019). Mapping Out Research Focus for Robotics and Automation Research in Construction-Related Studies. J. Eng. Des. Technol. 18, 1063–1079. doi:10.1108/jedt-09-2019-0237
Ahmadi-Karvigh, S., Becerik-Gerber, B., and Soibelman, L. (2019). Intelligent Adaptive Automation: A Framework for an Activity-Driven and User-Centered Building Automation. Energy and Buildings 188-189, 184–199. doi:10.1016/j.enbuild.2019.02.007
Ahuja, R., Sawhney, A., and Arif, M. (2017). Prioritizing BIM Capabilities of an Organization: An Interpretive Structural Modeling Analysis. Proced. Eng. 196, 2–10. doi:10.1016/j.proeng.2017.07.166
Akanmu, A. A., Anumba, C. J., and Ogunseiju, O. O. (2021). Towards Next Generation Cyber-Physical Systems and Digital Twins for Construction. ITcon 26, 505–525. doi:10.36680/j.itcon.2021.027
Akinosho, T. D., Oyedele, L. O., Bilal, M., Ajayi, A. O., Delgado, M. D., Akinade, O. O., et al. (2020). Deep Learning in the Construction Industry: A Review of Present Status and Future Innovations. J. Building Eng. 32, 101827. doi:10.1016/j.jobe.2020.101827
Akinradewo, O., Oke, A., Aigbavboa, C., and Mashangoane, M. (2018). Willingness to Adopt Robotics and Construction Automation in the South African Construction Industry. Proc. Int. Conf. Ind. Eng. Oper. Manag. 2018, 1639–1646.
Alawad, H., and Kaewunruen, S. (2021). 5G Intelligence Underpinning Railway Safety in the COVID-19 Era. Front. Built Environ. 7, 1–5. doi:10.3389/fbuil.2021.639753
Aouad, G., Sun, M., and Faraj, I. (2002). Automatic Generation of Data Representations for Construction Applications. Construct Innov. 2, 151–165. doi:10.1191/1471417502ci034oa
Aste, N., Manfren, M., and Marenzi, G. (2017). Building Automation and Control Systems and Performance Optimization: A Framework for Analysis. Renew. Sustain. Energ. Rev. 75, 313–330. doi:10.1016/j.rser.2016.10.072
Bademosi, F., and Issa, R. R. A. (2021). Factors Influencing Adoption and Integration of Construction Robotics and Automation Technology in the US. J. Constr. Eng. Manag. 147. doi:10.1061/(asce)co.1943-7862.0002103
Bello, S. A., Oyedele, L. O., Akinade, O. O., Bilal, M., Davila Delgado, J. M., Akanbi, L. A., et al. (2021). Cloud Computing in Construction Industry: Use Cases, Benefits and Challenges. Automation in Construction 122, 103441. doi:10.1016/j.autcon.2020.103441
Bock, T., and Christos, G. (2016). Robotics in Construction. Berlin, Germany: Springer Handb. Robot, 1–2227.
Bogue, R. (2018). What Are the Prospects for Robots in the Construction Industry? Industrial Robot 45, 1–6. doi:10.1108/ir-11-2017-0194
Boktor, J., Hanna, A., and Menassa, C. C. (2014). State of Practice of Building Information Modeling in the Mechanical Construction Industry. J. Manage. Eng. 30, 78–85. doi:10.1061/(asce)me.1943-5479.0000176
Cai, S., Ma, Z., Skibniewski, M. J., and Guo, J. (2020). Construction Automation and Robotics: From One-Offs to Follow-Ups Based on Practices of Chinese Construction Companies. J. Constr. Eng. Manage. 146, 05020013. doi:10.1061/(asce)co.1943-7862.0001910
Chen, Q., García de Soto, B., and Adey, B. T. (2018). Construction Automation: Research Areas, Industry Concerns and Suggestions for Advancement. Automation in Construction 94, 22–38. doi:10.1016/j.autcon.2018.05.028
Chen, X., Chang-Richards, A. Y., Pelosi, A., Jia, Y., Shen, X., Siddiqui, M. K., et al. (2021). Implementation of Technologies in the Construction Industry: a Systematic Review. Eng. Constr. Archit. Manag. doi:10.1108/ecam-02-2021-0172
Chowdhury, T., Adafin, J., and Wilkinson, S. (2019). Review of Digital Technologies to Improve Productivity of New Zealand Construction Industry. ITcon 24, 569–587. doi:10.36680/j.itcon.2019.032
Cubric, M. (2020). Drivers, Barriers and Social Considerations for AI Adoption in Business and Management: A Tertiary Study. Technol. Soc. 62, 101257. doi:10.1016/j.techsoc.2020.101257
Dagnaw, G. (2020). Artificial Intelligence towards Future Industrial Opportunities and Challenges. African Conf. Inf. Syst.
Darko, A., Chan, A. P. C., Adabre, M. A., Edwards, D. J., Hosseini, M. R., and Ameyaw, E. E. (2020). Artificial Intelligence in the AEC Industry: Scientometric Analysis and Visualization of Research Activities. Automation in Construction 112, 103081. doi:10.1016/j.autcon.2020.103081
Darlow, G., Rotimi, J. O. B., and Shahzad, W. M. (2022). Automation in New Zealand's Offsite Construction (OSC): a Status Update. Bepam 12, 38–52. doi:10.1108/bepam-11-2020-0174
Duperrin, J. C., and Godet, M. (1973). Methodology for Prioritising Elements from a System. Rapp. Econ. Du CEA.
Dwivedi, Y. K., Hughes, L., Ismagilova, E., Aarts, G., Coombs, C., Crick, T., et al. (2021). Artificial Intelligence (AI): Multidisciplinary Perspectives on Emerging Challenges, Opportunities, and Agenda for Research, Practice and Policy. Int. J. Inf. Manage. 57, 101994. doi:10.1016/j.ijinfomgt.2019.08.002
El Jazzar, M., Schranz, C., Urban, H., and Nassereddine, H. (2021). Integrating Construction 4.0 Technologies: A Four-Layer Implementation Plan. Front. Built Environ. 7, 1–14. doi:10.3389/fbuil.2021.671408
Emaminejad, N., Akhavian, R., and Ph, D. (2021). Trustworthy AI and Robotics and the Implications for the AEC Industry: A Systematic Literature Review and Future Potentials. arXiv 2021, 1–29.
Eshun, B. T. B., and Chan, A. P. C. (2021). An Evaluation of Project Risk Dynamics in Sino-Africa Public Infrastructure Delivery; a Causal Loop and Interpretive Structural Modelling Approach (ISM-CLD). Sustain 13. doi:10.3390/su131910822
Fernandes, T., and Oliveira, E. (2021). Understanding Consumers' Acceptance of Automated Technologies in Service Encounters: Drivers of Digital Voice Assistants Adoption. J. Business Res. 122, 180–191. doi:10.1016/j.jbusres.2020.08.058
Gargoum, S. A., and Karsten, L. (2021). Virtual Assessment of Sight Distance Limitations Using LiDAR Technology: Automated Obstruction Detection and Classification. Automation in Construction 125, 103579. doi:10.1016/j.autcon.2021.103579
Haas, C. T., and Kim, Y. S. (2002). Automation in Infrastructure Construction. Constr. Innov. 2, 191–210. doi:10.1108/14714170210814766
Hansapinyo, C., Latcharote, P., and Limkatanyu, S. (2020). Seismic Building Damage Prediction from GIS-Based Building Data Using Artificial Intelligence System. Front. Built Environ. 6, 1–11. doi:10.3389/fbuil.2020.576919
International Federation of Robotics (IFR) (2017). The Impact of Robots on Productivity, Employment and Jobs. Frankfurt, Germany: Positioning Paper.
Jung, K., Chu, B., and Hong, D. (2013). Robot-based Construction Automation: An Application to Steel Beam Assembly (Part II). Automation in Construction 32, 62–79. doi:10.1016/j.autcon.2012.12.011
Karimidorabati, S., Haas, C. T., and Gray, J. (2016). Evaluation of Automation Levels for Construction Change Management. Ecam 23, 554–570. doi:10.1108/ecam-01-2015-0013
Lee, D.-s., Chen, Y.-T., and Chao, S.-L. (2022). Universal Workflow of Artificial Intelligence for Energy Saving. Energ. Rep. 8, 1602–1633. doi:10.1016/j.egyr.2021.12.066
Li, J., Greenwood, D., and Kassem, M. (2019). Blockchain in the Built Environment and Construction Industry: A Systematic Review, Conceptual Models and Practical Use Cases. Automation in Construction 102, 288–307. doi:10.1016/j.autcon.2019.02.005
Liu, P., Li, Q., Bian, J., Song, L., and Xiahou, X. (2018). Using Interpretative Structural Modeling to Identify Critical Success Factors for Safety Management in Subway Construction: A China Study. Int. J. Environ. Res. Public Health 15, 1359. doi:10.3390/ijerph15071359
Lu, P., Chen, S., and Zheng, Y. (2012). Artificial Intelligence in Civil Engineering. Math. Probl. Eng. 2012, 1–22. doi:10.1155/2012/145974
Mamela, T. L., Sukdeo, N., and Mukwakungu, S. C. (2020). “The Integration of AI on Workforce Performance for a South African Banking Institution,” in 2020 International Conference on Artificial Intelligence, Big Data, Computing and Data Communication Systems IcABCD 2020 - Proceedings.
Mandal, A., and Deshmukh, S. (1994). Vendor Selection Using Interpretive Structural Modelling (ISM). Int. J. Oper. Prod. Manag. 14, 52–59.
Manuel, J., Delgado, D., Oyedele, L., Ajayi, A., Akanbi, L., Akinade, O., et al. (2019). Robotics and Automated Systems in Construction: Understanding Industry-specific Challenges for Adoption. J. Build. Eng. 26, 100868.
Manzoor, B., Othman, I., Durdyev, S., Ismail, S., and Wahab, M. H. (2021). Influence of Artificial Intelligence in Civil Engineering toward Sustainable Development—A Systematic Literature Review. Appl. Syst. Innov. 4, 1–17. doi:10.3390/asi4030052
Maskuriy, R., Selamat, A., Maresova, P., Krejcar, O., and David, O. O. (2019). Industry 4.0 for the Construction Industry: Review of Management Perspective. Economies 7, 1–14. doi:10.3390/economies7030068
Mathiyazhagan, K., Govindan, K., NoorulHaq, A., and Geng, Y. (2013). An ISM Approach for the Barrier Analysis in Implementing green Supply Chain Management. J. Clean. Prod. 47, 283–297. doi:10.1016/j.jclepro.2012.10.042
McNamara, A. J., and Sepasgozar, S. M. E. (2021). Intelligent Contract Adoption in the Construction Industry: Concept Development. Automation in Construction 122, 103452. doi:10.1016/j.autcon.2020.103452
Melenbrink, N., Rinderspacher, K., Menges, A., and Werfel, J. (2020a). Autonomous Anchoring for Robotic Construction. Automation in Construction 120, 103391. doi:10.1016/j.autcon.2020.103391
Melenbrink, N., Werfel, J., and Menges, A. (2020b). On-site Autonomous Construction Robots: Towards Unsupervised Building. Automation in Construction 119, 103312. doi:10.1016/j.autcon.2020.103312
Mitra Debnath, R., and Shankar, R. (2012). Improving Service Quality in Technical Education: Use of Interpretive Structural Modeling. Use interpretive Struct. Model. 20, 387–407. doi:10.1108/09684881211264019
Mohammadpour, A., Atefeh Karan, E., and Asadi, S. (2019). “Artificial Intelligence Techniques to Support Design and Construction,” in International Symposium on Automation and Robotics in Construction. doi:10.22260/ISARC2019/0172
Mohapatra, S., Kumar, A., and Kumar, A. (2019). Developing a Framework for Adopting Artificial Intelligence. Int. J. Comput. Theor. Eng. 11, 19–22. doi:10.7763/ijcte.2019.v11.1234
Naghshbandi, S. N., Varga, L., and Hu, Y. (2021). Technology Capabilities for an Automated and Connected Earthwork Roadmap. Constr. Innov. doi:10.1108/ci-02-2021-0022
Nazareno, L., and Schiff, D. S. (2021). The Impact of Automation and Artificial Intelligence on Worker Well-Being. Technol. Soc. 67, 101679. doi:10.1016/j.techsoc.2021.101679
Nikas, A., Poulymenakou, A., and Kriaris, P. (2007). Investigating Antecedents and Drivers Affecting the Adoption of Collaboration Technologies in the Construction Industry. Automation in Construction 16, 632–641. doi:10.1016/j.autcon.2006.10.003
Nishant, R., Kennedy, M., and Corbett, J. (2020). Artificial Intelligence for Sustainability: Challenges, Opportunities, and a Research Agenda. Int. J. Inf. Manage. 53, 102104.
Obi, L., Awuzie, B., Obi, C., Omotayo, T. S., Oke, A., and Osobajo, O. (2021). Bim for Deconstruction: An Interpretive Structural Model of Factors Influencing Implementation. Buildings 11. doi:10.3390/buildings11060227
Oesterreich, T. D., and Teuteberg, F. (2016). Understanding the Implications of Digitisation and Automation in the Context of Industry 4.0: A Triangulation Approach and Elements of a Research Agenda for the Construction Industry. Comput. Industry 83, 121–139. doi:10.1016/j.compind.2016.09.006
O’Grady, T., Chong, H. Y., and Morrison, G. M. (2021). A Systematic Review and Meta-Analysis of Building Automation Systems. Build. Environ. 195, 107770.
Oke, A., Akinradewo, O., Aigbavboa, C., and Akinradewo, O. (2019). Benefits of Construction Automation and Robotics in the South African Construction Industry. IOP Conf. Ser. Earth Environ. Sci. 385. doi:10.1088/1755-1315/385/1/012063
Okpala, I., Nnaji, C., and Karakhan, A. A. (2020). Utilizing Emerging Technologies for Construction Safety Risk Mitigation. Pract. Period. Struct. Des. Constr. 25, 04020002. doi:10.1061/(asce)sc.1943-5576.0000468
Olawumi, T. O., and Chan, D. W. M. (2019). An Empirical Survey of the Perceived Benefits of Executing BIM and Sustainability Practices in the Built Environment. Construction Innovation 19, 321–342. doi:10.1108/ci-08-2018-0065
Onososen, A., and Musonda, I. (2022). Barriers to BIM-Based Life Cycle Sustainability Assessment for Buildings: An Interpretive Structural Modelling Approach. Buildings 12, 324. doi:10.3390/buildings12030324
Pan, M., Linner, T., Pan, W., Cheng, H., and Bock, T. (2020). Structuring the Context for Construction Robot Development through Integrated Scenario Approach. Autom. Constr. 114. doi:10.1016/j.autcon.2020.103174
Pan, Y., and Zhang, L. (2021). Roles of Artificial Intelligence in Construction Engineering and Management: A Critical Review and Future Trends. Automation in Construction 122, 103517. doi:10.1016/j.autcon.2020.103517
Paneru, S., Jahromi, F. F., Hatami, M., Roudebush, W., and Jeelani, I. (2021). Integration of Emergy Analysis with Building Information Modeling. Sustain 13. doi:10.3390/su13147990
Pillai, V. S., and Matus, K. J. M. (2021). Towards a Responsible Integration of Artificial Intelligence Technology in the Construction Sector. Sci. Public Pol. 47, 689–704. doi:10.1093/scipol/scaa073
Prentice, C., Dominique Lopes, S., and Wang, X. (2020). Emotional Intelligence or Artificial Intelligence- an Employee Perspective. J. Hospitality Marketing Manage. 29, 377–403. doi:10.1080/19368623.2019.1647124
Ravi, V., and Shankar, R. (2005). Analysis of Interactions Among the Barriers of Reverse Logistics. Technol. Forecast. Soc. Change 72, 1011–1029. doi:10.1016/j.techfore.2004.07.002
Ruggiero, A., Salvo, S., and St. Laurent, C. (2016). Robotics in Construction. Worcester, MA, USA: Worcester Polytechnic Institute.
Saidi, K. S., Bock, T., and Georgoulas, C. (2016). Robotics in Construction. Berlin, Germany: Springer Handb. Robot, 1493–1520. doi:10.1007/978-3-319-32552-1_57
Saka, A. B., and Chan, D. W. M. (2020). Profound Barriers to Building Information Modelling (BIM) Adoption in Construction Small and Medium-Sized Enterprises (SMEs). Construction Innovation 20, 261–284. doi:10.1108/ci-09-2019-0087
Salehi, H., and Burgueño, R. (2018). Emerging Artificial Intelligence Methods in Structural Engineering. Eng. Struct. 171, 170–189.
Shahandashti, S. M., Razavi, S. N., Soibelman, L., Berges, M., Caldas, C. H., Brilakis, I., et al. (2011). Data-fusion Approaches and Applications for Construction Engineering. J. Constr. Eng. Manage. 137, 863–869. doi:10.1061/(asce)co.1943-7862.0000287
Shen, L., Song, X., Wu, Y., Liao, S., and Zhang, X. (2016). Interpretive Structural Modeling Based Factor Analysis on the Implementation of Emission Trading System in the Chinese Building Sector. J. Clean. Prod. 127, 214–227. doi:10.1016/j.jclepro.2016.03.151
Shoar, S., and Chileshe, N. (2021). Exploring the Causes of Design Changes in Building Construction Projects: An Interpretive Structural Modeling Approach. Sustain 13. doi:10.3390/su13179578
Shoar, S., Yiu, T. W., Payan, S., and Parchamijalal, M. (2021). Modeling Cost Overrun in Building Construction Projects Using the Interpretive Structural Modeling Approach: a Developing Country Perspective. Eng. Constr. Archit. Manag. ahead-of-p. doi:10.1108/ecam-08-2021-0732
Shubha, R. (2019). Impact of Automation and Artificial Intelligence. Int. J. Hum. Comput. Stud. 2019, 14–17.
Shukla, A. K., Janmaijaya, M., Abraham, A., and Muhuri, P. K. (2019). Engineering Applications of Artificial Intelligence: A Bibliometric Analysis of 30 Years (1988-2018). Eng. Appl. Artif. Intelligence 85, 517–532. doi:10.1016/j.engappai.2019.06.010
Skibniewski, M., and Hendrickson, C. (1990). Automation and Robotics for Road Construction and Maintenance. J. Transp. Eng. 116, 261–271. doi:10.1061/(asce)0733-947x(1990)116:3(261)
Sobotka, A., and Pacewicz, K. (2017). Mechanisation and Automation Technologies Development in Work at Construction Sites. IOP Conf. Ser. Mater. Sci. Eng. 251. doi:10.1088/1757-899x/251/1/012046
Sushil, S. (2018). How to Check Correctness of Total Interpretive Structural Models? Ann. Oper. Res. 270, 473–487. doi:10.1007/s10479-016-2312-3
Trujillo, D., and Holt, E. (2020). Barriers to Automation and Robotics in Construction. Epic Ser. Built Environ. 1, 257–247.
Tussyadiah, I. (2020). A Review of Research into Automation in Tourism: Launching the Annals of Tourism Research Curated Collection on Artificial Intelligence and Robotics in Tourism. Ann. Tourism Res. 81, 102883. doi:10.1016/j.annals.2020.102883
Wang, B., Yuan, J., and Ghafoor, K. Z. (2021). Research on Construction Cost Estimation Based on Artificial Intelligence Technology. Scpe 22, 93–104. doi:10.12694/scpe.v22i2.1868
Warfield, J. N. (1974). Developing Interconnection Matrices in Structural Modeling. IEEE Trans. Syst. Man. Cybern. SMC-4, 81–87. doi:10.1109/tsmc.1974.5408524
Keywords: artificial intelligence, automation, benefits, construction industry, interpretive structural modeling (ism), MICMAC, AEC
Citation: Onososen AO and Musonda I (2022) Perceived Benefits of Automation and Artificial Intelligence in the AEC Sector: An Interpretive Structural Modeling Approach. Front. Built Environ. 8:864814. doi: 10.3389/fbuil.2022.864814
Received: 28 January 2022; Accepted: 21 March 2022;
Published: 20 April 2022.
Edited by:
Maria Rashidi, Western Sydney University, AustraliaReviewed by:
Venkata Santosh Kumar Delhi, Indian Institute of Technology Bombay, IndiaAmir Mahdiyar, University of Science Malaysia, Malaysia
Copyright © 2022 Onososen and Musonda. This is an open-access article distributed under the terms of the Creative Commons Attribution License (CC BY). The use, distribution or reproduction in other forums is permitted, provided the original author(s) and the copyright owner(s) are credited and that the original publication in this journal is cited, in accordance with accepted academic practice. No use, distribution or reproduction is permitted which does not comply with these terms.
*Correspondence: Adetayo Olugbenga Onososen, Onososen@outlook.com