- 1Pediatrics, Women and Infants Hospital, Providence, RI, United States
- 2Pediatrics, Warren Alpert Medical School, Brown University, Providence, RI, United States
- 3Center for Computation and Visualization, Brown University, Providence, RI, United States
- 4Computational Biology of Human Disease, Brown University, Providence, RI, United States
- 5Center for Computational Molecular Biology, Brown University, Providence, RI, United States
Preeclampsia is a hypertensive disorder of pregnancy, which complicates up to 15% of US deliveries. It is an idiopathic disorder associated with several different phenotypes. We sought to determine if the genetic architecture of preeclampsia can be described by clusters of patients with variants in genes in shared protein interaction networks. We performed a case-control study using whole exome sequencing on early onset preeclamptic mothers with severe clinical features and control mothers with uncomplicated pregnancies between 2016 and 2020. A total of 143 patients were enrolled, 61 women with early onset preeclampsia with severe features based on ACOG criteria, and 82 control women at term, matched for race and ethnicity. A network analysis and visualization tool, Proteinarium, was used to confirm there are clusters of patients with shared gene networks associated with severe preeclampsia. The majority of the sequenced patients appear in two significant clusters. We identified one case dominant and one control dominant cluster. Thirteen genes were unique to the case dominated cluster. Among these genes, LAMB2, PTK2, RAC1, QSOX1, FN1, and VCAM1 have known associations with the pathogenic mechanisms of preeclampsia. Using bioinformatic analysis, we were able to identify subsets of patients with shared protein interaction networks, thus confirming our hypothesis about the genetic architecture of preeclampsia.
Introduction
Preeclampsia is a hypertensive disorder of pregnancy. It is associated with a higher risk of hypertension and cardiovascular disease later in life. Women who had preeclampsia have a twofold increased risk of death from cardiovascular diseases (Bokslag et al., 2016; Neerukonda et al., 2020). There is evidence that preeclampsia originates in part from genetic causes that include contributions from the maternal, paternal and fetal genome (Zusterzeel et al., 2002; Cnattingius et al., 2004; Nilsson et al., 2004; Kobayashi, 2015; Than et al., 2018). The heritability of preeclampsia is up to 52% (Salonen Ros et al., 2000; Chappell and Morgan, 2006). The role of genetics in preeclampsia is also supported by family-based observations (Chappell and Morgan, 2006; Nejatizadeh et al., 2008) with more than 100 studies showing a 2- to 5-fold increased risk among family members of affected women (Chesley et al., 1968; Sutherland et al., 1981; Arngrimsson et al., 1990; Cincotta and Brennecke, 1998; Mutze et al., 2008; Ward, 2008). The recurrence risk for preeclampsia in the daughters of either eclamptic or preeclamptic patients is 20–40% (Serrano, 2006; Genc and Schantz-Dunn, 2007). Nonetheless, there is no current consensus among the published results in regards to associated genes and the pathogenesis of the disease.
The genetic basis for complex diseases involves the interaction of multiple genes in discrete networks and pathways (Loscalzo et al., 2007). Although complex diseases show increased recurrence risk in families, they do not follow a simple Mendelian pattern of inheritance (Smith and Ebrahim, 2003). Computational methods have been used to analyze networks of genes and to find biological subnetworks due to the genetic heterogeneity of e diseases that are linked to a variety of disorders (Wall et al., 2009). There are several studies employing computational methods to identify important genes associated with hypertension. Ran et al. analyzed the protein protein interaction (PPI) network topology and molecular connectivity between protein pathways to identify associations with hypertension (Ran et al., 2013). Researchers have also developed a machine-learning algorithm to predict novel hypertension associated genes (Li et al., 2017).
We hypothesize that the genetic architecture of complex diseases like preeclampsia is described by clusters of patients with variants in genes in shared PPI networks. We sought to test this hypothesis using whole exome sequencing in carefully selected patients with early onset preeclampsia with severe clinical features. We compared variants identified in women with early onset, idiopathic severe preeclampsia with term controls without personal or family history of pregnancy related hypertensive disorders. We built and implemented Proteinarium, a novel multi-sample PPI tool, to identify clusters of patients with shared PPI networks.
Materials and Methods
Study Population
Women & Infants Hospital of Rhode Island (WIH) is the only provider of high-risk perinatal services in Rhode Island, northeastern Connecticut and southeastern Massachusetts. We used this population-based service to enroll preeclamptic mothers with early onset, severe features, based on ACOG criteria, as well as term mothers with no history of preeclampsia (Roberts et al., 2013).
This case/control study was approved by the Institutional Review Board of WIH (Project ID: WIH 16–0031). Between the years 2016–2020, we reviewed the records of all early-onset preeclamptic mothers with severe features delivering <34 weeks. Following informed consent, we asked explicit questions about preeclampsia in mother, grandmother, first order relatives and also paternal relatives. Gestational age was determined by best obstetrical estimate. In almost all cases, this was by first trimester ultrasound. Clinical history, with an emphasis on additional risk factors including medical illnesses and drug use was recorded. Hypertensive disorders include a broad range of different phenotypes. Again, in order to leverage the likelihood of genetic discovery associated with preeclampsia, we excluded preeclamptic mothers with personal or family history of other hypertensive disorders. Controls were mothers who delivered ≥37 weeks’ gestation for whom the formal genetic interview revealed no history of preterm birth or pregnancy related hypertensive disorders on either the maternal or paternal side of the pedigree. A total of 143 patients were enrolled, 61 women with early onset preeclampsia with severe features, and 82 control women at term, matched for race and ethnicity.
Whole Exome Sequencing
EDTA stabilized, residual maternal whole blood was obtained from each mother and stored at -80 °C. Samples were sent to an outside facility for whole exome sequencing that was blind to disease status. The library was sequenced on an Illumina HiSeq 4000 using 150 bp paired-end protocols.
Sequence Data
For variant discovery we used the Gene Analysis Tool Kit (GATK) V4 to analyze the sequence reads (Van der Auwera et al., 2013). Haplotype Caller was applied for variant detection (Poplin et al., 2018). Variants were flagged as low quality and filtered using established metrics: if three or more variants were detected within 10bp; if four or more alignments mapped to different locations equally well; if coverage was less than ten reads; if quality score <30; if low quality for a particular sequence depth (variant confidence/unfiltered depth <1.5); and if strand bias was observed (Phred-scaled p-values using Fisher’s Exact Test >200).
Genotype Testing
To identify variants that were differentially abundant between cases and controls, we used a Markov Chain Monte Carlo (MCMC) Fisher Exact Test to compare the frequency of the homozygous reference, homozygous alternative, and the heterozygous genotypes between cases and controls. Eigenstrat detected no significant population stratification (Price et al., 2006). None of the eigenvectors were associated with race/ethnicity.
Univariate Analysis: Variant Annotation
We applied a strict filter-based annotation using ANNOVAR (Wang et al., 2010). We identified deleterious variants with Polyphen 2 HDIV, SIFT and CADD (Ng and Henikoff, 2003; Adzhubei et al., 2010; Kircher et al., 2014; Genomes Project et al., 2015). We used the following thresholds: Polyphen 2 HDIV prediction if a change is damaging (≥0.957), a SIFT score (<0.05), a CADD score >15, and minor allele frequency (MAF) < 0.05 from the 1,000 Genome Project (Genomes Project et al., 2015).
Multivariate Protein Protein Interaction Analysis
We hypothesized that the genetic architecture underlying complex disorders is best explained by subsets of patients with variants in shared networks and pathways sufficient to express the phenotype. To analyze our whole exome sequencing data, we developed Proteinarium, a novel multi-sample PPI analysis and visualization tool (Armanious et al., 2020). For each sample, the top 60 genes, corresponding to the most significant, differentially abundant variants (ranked by genotype testing p value) were used as the seed genes for input into Proteinarium. The pipeline for Proteinarium is described in great detail in Armanious et al., 2020. In brief, these seed genes were used to create a PPI network for each patient. After generating the network graphs for each patient, Proteinarium calculates the similarity between each pair of graphs using the Jaccard distance. This similarity matrix is then used as the input to cluster the set of graphs. Clustering is performed hierarchically using Unweighted Pair Group Method with Arithmetic Mean (UPGMA). Proteinarium outputs the results of the clustering algorithm as a dendrogram and shared interaction network graphs. For each cluster, the abundance of cases is compared to that of the controls using Fisher’s exact test. Each cluster can be visualized as a layered graph of its constituent networks. Proteinarium was implemented with the minimum path length parameter set to 2, to include only those pathways in which seed proteins are connected directly to each other and/or via a single intermediary protein. We refer to these intermediary connecting proteins as imputed proteins.
Network Separation Testing
Separation testing is a computational approach for determining the genetic similarity between diseases by comparing their protein protein interaction networks from the interactome (Menche et al., 2015). It compares the shortest distances between network proteins within each disease or network to the shortest distances between the disease networks. A positive separation score indicates that there is a physical separation between networks within the interactome. We computed the separation score between the layered network graphs of clusters identified as associated to a specific phenotype via the Fisher exact test by Proteinarium (Armanious et al., 2020).
Results
The clinical characteristics and the race/ethnicity distribution of the patients are shown in Table 1. As can be seen, the gestational age at delivery, systolic blood pressure, frequency of proteinuria, impaired liver function, thrombocytopenia, cerebral visual symptoms and fetal growth retardation were all significantly different between the groups, which was expected by our definition of severe preeclampsia.
High quality sequence data with a Phred score ≥30 from well-balanced pools with over 19, 000, 000 reads/patient, 40X average depth of coverage, with more than 80% of sequence reads with at least 20X coverage were observed. We identified a total of 528,630 variants including 187,915 exonic variants. The work flow for the univariate analysis is shown in Figure 1. After application of the initial filters for coverage and variant pathogenicity, there were 8,868 predicted deleterious variants (available at Online_Supplementary Table S1). Among these, 21 variants were nominally associated with preeclampsia by genotype testing. All were non-synonymous, exonic variants (Table 2). Nonetheless, none of these variants met genome-wide significance after correction for multiple comparison testing.
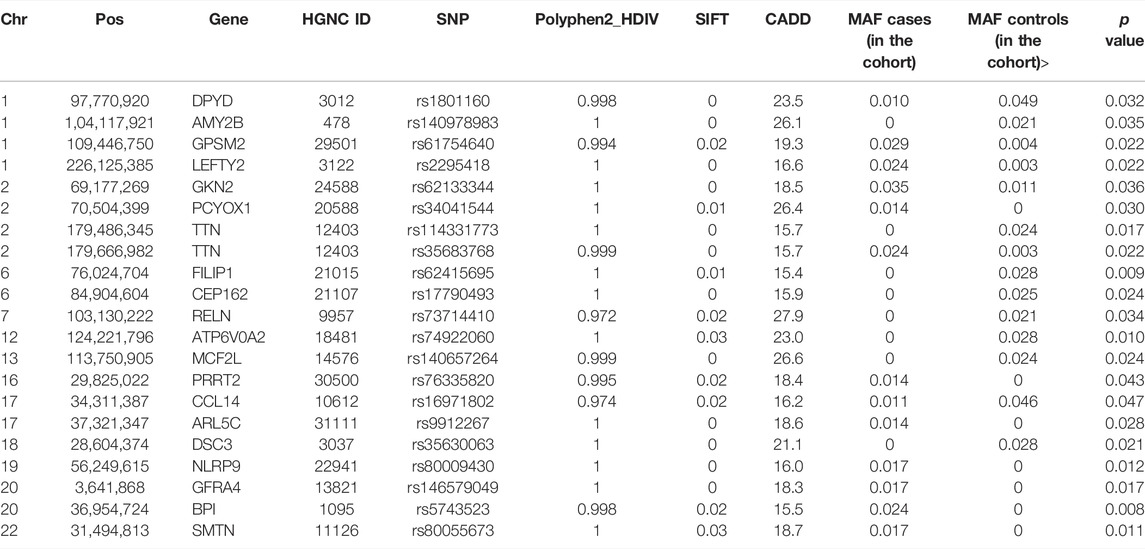
TABLE 2. Pathogenic, nominally significant (based on genotype testing, p < 0.05) gene variants identified by univariate analysis. Genomic positions are based on Human Feb. 2009 (GRCh37/hg19) Assembly. p value represents the genotype testing.
Proteinarium was used to carry out the multivariate protein protein interaction analysis to identify clusters of patients with shared networks associated with severe preeclampsia. The resulting, circularized dendrogram is shown in Figure 2A (Ciccarelli et al., 2006). Out of the 143 patients sequenced, 129 patients were assigned to two statistically significant clusters. (p < 0.0001). The inset in Figure 2A shows the number of cases and controls in each cluster. Cluster A had significantly more cases than controls, containing 47 of the 61 case subjects (Bastian et al., 2009). The layered network for the case-dominated Cluster A is shown in Figure 2B. There are 13 genes which are unique to Cluster A, highlighted in red in the layered network graph. Most have defined functional roles or implications for preeclampsia, Table 3. Cluster B had significantly more controls than cases, including 61 of the 82 subjects. The layered network for Cluster B is shown in Figure 2C. The unique genes from the layered network graph of Cluster B, shown in blue, are listed in Supplementary Table S1.
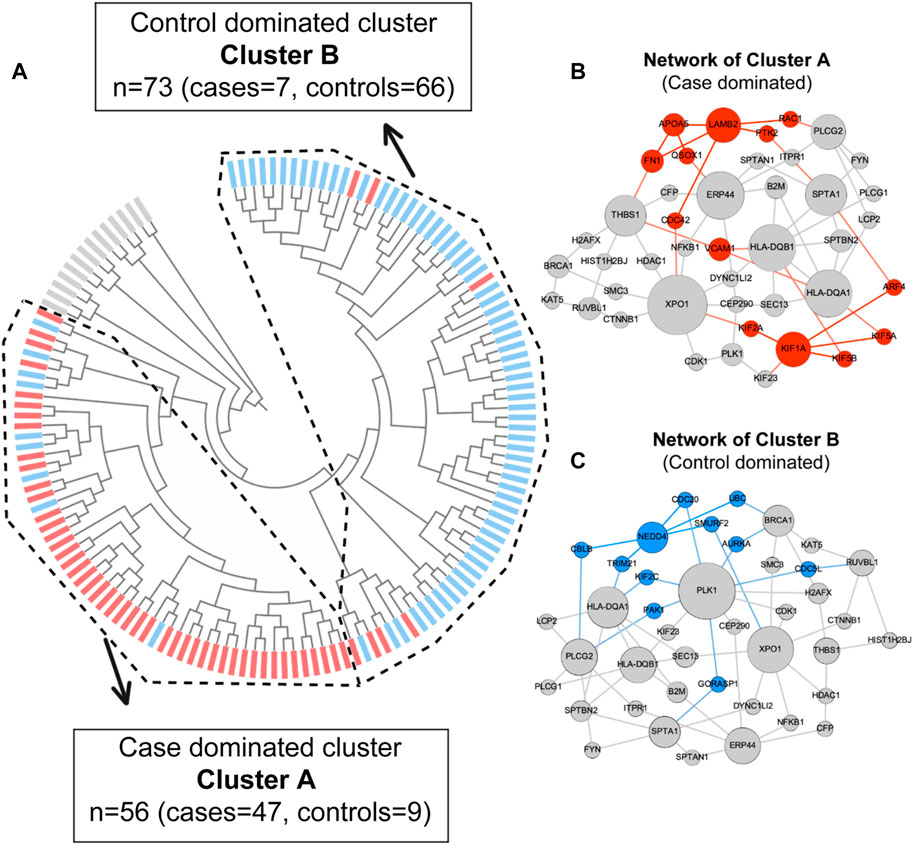
FIGURE 2. (A) Dendrogram shows statistically significant (p < 0.05) clusters of patients. Case dominated cluster (Cluster A) and control dominated cluster (Cluster B) are presented by dashed lines. Cases are represented in red and controls are represented in blue color. (B) Layered network graphs for the case dominated cluster A are presented. 13 unique genes of cluster A are in red color. (C) Layered network graphs for the control dominated cluster B are presented. 11 unique genes of cluster B are in blue color.
We compared the sequence data of the samples not assigned to clusters with those that were assigned and we did not find significant differences in the average depth of coverage. Likewise, there were no significant differences in clinical/phenotypic characteristics when comparing the subjects in the significant clusters with the subjects that were not in these clusters (data not shown).
The comparison of the unique genes from the case and the control dominated clusters revealed a positive separation score, confirming that the layered PPI networks of these two patient subgroups indeed exist in distinct areas of the interactome. We ran GO term analysis using DAVID software on all genes of the network from Cluster A and from Cluster B, Supplementary Table S2 (Huang da et al., 2009b; a). We found significantly enriched biological processes, molecular functions and cellular components based on Bonferroni corrected p-value for the case and control dominated networks. Prominent among the biological processes and molecular functions associated with preeclampsia were antigen processing and presentation, cellular movement (axon guidance and microtubules) and T cell receptor signaling.
We previously reported the database for Preeclampsia (dbPEC) which archives a curation-based collection of genes associated with preeclampsia and their association with clinical features and concurrent conditions (Triche et al., 2014). We compared the genes from our univariate analysis and the genes from both case and control dominated layered networks to those in the database. We found two overlapping genes from the univariate gene list (TTN and CCL14) that were included in dbPEC. We also found three overlapping genes from the layered network of Cluster A (FN1, KIF2A, VCAM1). By over representation analysis, Cluster A is significantly enriched for genes previously shown to be associated with preeclampsia in dbPEC (p < 0.0033).
Discussion
Preeclampsia is a life-threatening, multi-system hypertensive disorder of pregnancy, which complicates up to 15% of US deliveries (Chappell and Morgan, 2006; Valenzuela et al., 2012; Bornstein, 2020; Steinthorsdottir et al., 2020). The incidence is increasing (Bornstein, 2020). It is recognized as a leading cause of maternal and fetal morbidity and mortality worldwide (Valenzuela et al., 2012). Preeclampsia is characterized by varying degrees of maternal symptoms including elevated blood pressure, proteinuria and fetal growth restriction (Jebbink et al., 2012). Many clinicians believe that preeclampsia, severe preeclampsia, and early vs late preeclampsia are different disorders (Carreiras et al., 2002; Raymond and Peterson, 2011; American College of et al., 2013). Previously, using bioinformatic methods, we showed that there are discrete gene sets associated with these different phenotypes of preeclampsia (Triche et al., 2014).
We performed whole exome sequencing on women with idiopathic early-onset preeclampsia with severe features and singleton births <34 weeks’ gestation and compared them to term controls with no family history of preeclampsia. We developed Proteinarium, a novel multi-sample PPI analysis and visualization tool, to identify clusters of patients with shared protein protein interaction networks (Armanious et al., 2020). Unlike other tools which use the differentially expressed genes or the phenotype associated variants to build a single network for all samples, or clustering models based solely on gene expression, Proteinarium builds individual networks for each patient based on the STRING database. The similarities between individual PPI networks are then evaluated using a distance metric for clustering the samples. We identified a single, significant, large cluster of patients with a predominance of cases with early-onset, severe features of preeclampsia. We also identified a single control-dominated cluster. The separation test of the unique genes from case and control dominated clusters confirmed that the two subnetworks forming clusters A and B exist in the different regions of the interactome. These results support our hypothesis that the genetic architecture of complex diseases is characterized by clusters of patients that have variants in shared gene networks and provide insights into the genetics of severe preeclampsia.
Several of the unique genes from the case dominated network have very plausible mechanistic connections to preeclampsia. Laminin β2 (LAMB2) is a glomerular basement membrane (GBM) component, required for proper functioning of the glomerular filtration barrier. It has a role in proteinuria (Zhang and Huang, 2012) and serum laminin levels in preeclamptic patients are significantly higher than those in normal pregnancy (Furuhashi et al., 1993). Hypoxia-induced upregulation of Quiescin Sulfhydryl oxidase 1 (QSOX1) and an elevation in intracellular H2O2 leads to increased apoptosis in the placentae of pregnancies complicated by preeclampsia (Li et al., 2019). QSOX1 protein is found in circulating extracellular vesicles of both preeclampsia and healthy pregnant women (Tan et al., 2014). Fibronectin 1 (FN1) might promote the development of preeclampsia by modulating differentiation of human extravillous trophoblasts, as well as formation of focal adhesions (Brubaker et al., 1992; Auer et al., 2010; Zhao et al., 2017). Vascular Cell Adhesion Molecule 1 (VCAM1) is involved in cellular adhesion and serum concentrations of sVCAM-1 are significantly elevated in both mild and severe preeclampsia (Kim et al., 2004). Invasion of maternal decidua and uterine spiral arteries by extravillous trophoblasts is required for establishment of normal placenta. Human trophoblast migration requires Rac Family Small gtpase 1 (RAC1) and Cell Division Cycle 42 (CDC42) (Grewal et al., 2008). Lower levels were found in preeclampsia samples than in normal term pregnancy samples, and decline significantly in severe preeclampsia (Fan et al., 2016). Protein tyrosine kinase 2 (PTK2) (focal adhesion kinase) is differentially expressed in preeclampsia and reported as among the promising biomarkers for preeclampsia (Sado et al., 2011). In the case-dominated subnetwork we observed Kinesin Family Member 2A (KIF2A) which is upregulated in the preeclamptic placenta (Kobayashi, 2015). Up-regulated genes in the preeclampsia placenta have been shown to be associated with the regulation of diverse cellular processes, including matrix degradation, trophoblast cell invasion, migration and proliferation (Kobayashi, 2015).
There have been several sequencing efforts, including whole genome, whole exome and targeted sequencing, on an array of preeclampsia phenotypes from diverse populations (Johnson et al., 2012; Emmery et al., 2016; Kaartokallio et al., 2016; Thomsen et al., 2017; Gammill et al., 2018; Hansen et al., 2018; Soellner et al., 2018; Glotov et al., 2019; Melton et al., 2019; Steinthorsdottir et al., 2020; Zhang et al., 2020). There is no consensus among the published results in regards to associated genes and variants. Since preeclampsia is a complex, polygenic disease, the lack of a consensus among these univariate comparisons might be expected in these early-stage studies. Among the 20 genes identified in our univariate analysis, only Titin (TTN) was identified in prior studies (Gammill et al., 2018; Zhang et al., 2020). Protein-altering mutations in TTN have been identified in patients with cardiomyopathy and women with preeclampsia are more likely to carry TTN mutations associated with idiopathic cardiomyopathy and peripartum cardiomyopathy (Gammill et al., 2018). Additionally, we found 2 genes, Major Histocompatibility Complex, Class II, DQ Alpha 1 (HLA-DQA1) and Inositol 1,4,5-Trisphosphate Receptor Type 1 (ITPR1) that were reported in previous studies of preeclampsia (Emmery et al., 2016; Hansen et al., 2018). None of these overlapping genes were among the unique genes identified in the shared layered network from the case-dominated cluster. Likewise, no overlapping variants or genes were found in a recent genome-wide association meta-analysis investigating genetic predispositions associated with preeclampsia (Steinthorsdottir et al., 2020).
Our analysis allowed us to identify a cluster of patients with shared PPI networks associated with severe preeclampsia. Within the significant cluster, there were unique imputed genes (RAC1, KIF5B, PTK2, KIF5A, FN1, QSOX1, ARF4, VCAM1, CDC42, KIF2A) that were not among the top 60 seed genes selected by genotype testing. Nonetheless, our approach allowed us to identify these influential genes in the mechanism(s) underlying preeclampsia that would not otherwise have been identified by whole genome univariate variant analysis.
We also examined the unique genes in the network of the control dominated cluster. Proteins in this network are associated with the ubiquitination process. They may serve a role that confers resilience against preeclampsia (Fredrickson and Gardner, 2012; Berryman et al., 2019). Although there are studies showing a relationship with hypertension - ubiquitination process and pregnancy, this still needs further investigation (Fredrickson and Gardner, 2012).
Whole exome sequencing, combined with a novel, multi-sample network analysis, and careful phenotyping contributed to our discovery despite the relatively modest size of our study. Concepts developed from network theory suggest that related diseases involve proteins in similar neighborhoods of the interactome (Menche et al., 2015). Based on these concepts, we hypothesized that the genetic architecture of preeclampsia is described by subgroups of patients with variants in shared genes in specific networks and pathways. We identified a significant subgroup of cases with shared PPI networks associated with severe preeclampsia. We believe that the careful phenotyping resulted in the high percentage of subjects being successfully assigned to significant clusters and the ability to observe distinct separation between the case and control dominated clusters. We acknowledge that genetic variation may not be the sole mechanism for preeclampsia, but rather epigenetic changes or protein conformational disturbances may also play a significant role.
While we were not expecting each patient to appear in a significant cluster and our study included only a modest sample size, we identified a significant subgroup of patients with shared PPI networks associated with severe preeclampsia. In order to leverage the likelihood of genetics discovery, we focused exclusively on women with severe, early-onset preeclampsia. Our analysis was restricted to evaluation of genetic variants in the maternal genome only. Future studies including fetal and/or paternal data will enhance the likelihood of genetic discovery.
Using our unique network analysis, we were able to identify subsets of patients with shared networks, thus confirming our hypothesis about the genetic architecture of preeclampsia. Strict phenotyping of both cases and controls improved the likelihood of identifying these otherwise difficult to find genetic associations. Our network analysis identified genes which were imputed from the interactome and these imputed genes provide insights for severe preeclampsia that may otherwise have not been identified. As such, these are important candidates to include in meta-analyses of genetic associations with preeclampsia. These results provide promise to further our understanding the mechanism underlying complex diseases like preeclampsia.
Data Availability Statement
The original contributions presented in the study are included in the article/Supplementary Material, further inquiries can be directed to the corresponding author.
Ethics Statement
The studies involving human participants were reviewed and approved by This case/control study was approved by the Institutional Review Board of WIH (Project ID: WIH 16–0031). The patients/participants provided their written informed consent to participate in this study.
Author Contributions
JS, JP, AU wrote the manuscript, interpreted the results, critically reviewed the manuscript for important intellectual content. GT enrolled patients and collected samples, perform analysis on phenotype data. VZ enrolled patients and collected samples. AA performed network separation testing. JS prepared samples for whole exome sequencing (WES). AR performed QC and variant calling for WES. JP and AU conceptualized and designed the study.
Funding
This work was supported by grants from the National Institutes of Health 5P20GM109035-05 and 5P20GM121298-05.
Conflict of Interest
The authors declare that the research was conducted in the absence of any commercial or financial relationships that could be construed as a potential conflict of interest.
Publisher’s Note
All claims expressed in this article are solely those of the authors and do not necessarily represent those of their affiliated organizations, or those of the publisher, the editors and the reviewers. Any product that may be evaluated in this article, or claim that may be made by its manufacturer, is not guaranteed or endorsed by the publisher.
Acknowledgments
We thank the Kilguss Research Core at Women & Infants Hospital and The Center for Computation and Visualization (CCV) at Brown University.
Supplementary Material
The Supplementary Material for this article can be found online at: https://www.frontiersin.org/articles/10.3389/fgene.2021.765985/full#supplementary-material
References
Adzhubei, I. A., Schmidt, S., Peshkin, L., Ramensky, V. E., Gerasimova, A., Bork, P., et al. (2010). A Method and Server for Predicting Damaging Missense Mutations. Nat. Methods 7, 248–249. doi:10.1038/nmeth0410-248
American College of Obstetricians and Gynecologists (ACOG) (2020). Available: www.acog.org (Accessed October 8, 2020).
Armanious, D., Schuster, J., Tollefson, G. A., Agudelo, A., Dewan, A. T., Istrail, S., et al. (2020). Proteinarium: Multi-Sample Protein-Protein Interaction Analysis and Visualization Tool. Genomics 112, 4288–4296. doi:10.1016/j.ygeno.2020.07.028
Arngrimsson, R., Bjornsson, S., Geirsson, R. T., Bjornsson, H., Walker, J. J., and Snaedal, G. (1990). Genetic and Familial Predisposition to Eclampsia and Pre-Eclampsia in a Defined Population. BJOG:An Int. J. O&G 97, 762–769. doi:10.1111/j.1471-0528.1990.tb02569.x
Auer, J., Camoin, L., Guillonneau, F., Rigourd, V., Chelbi, S. T., Leduc, M., et al. (2010). Serum Profile in Preeclampsia and Intra-Uterine Growth Restriction Revealed by iTRAQ Technology. J. Proteomics 73, 1004–1017. doi:10.1016/j.jprot.2009.12.014
Bastian, M., Heymann, S., and Jacomy, M. (2009). “Gephi: An Open Source Software for Exploring and Manipulating Networks,” International AAAI Conference on Weblogs and Social Media 194 (4), 1031–1037. doi:10.1016/j.juro.2015.04.079
Berryman, K., Buhimschi, C. S., Zhao, G., Axe, M., Locke, M., and Buhimschi, I. A. (2019). Proteasome Levels and Activity in Pregnancies Complicated by Severe Preeclampsia and Hemolysis, Elevated Liver Enzymes, and Thrombocytopenia (HELLP) Syndrome. Hypertension 73, 1308–1318. doi:10.1161/hypertensionaha.118.12437
Bokslag, A., Van Weissenbruch, M., Mol, B. W., and De Groot, C. J. M. (2016). Preeclampsia; Short and Long-Term Consequences for Mother and Neonate. Early Hum. Develop. 102, 47–50. doi:10.1016/j.earlhumdev.2016.09.007
Bornstein, E. E., Chervenak, F. A., and Grünebaum, A. (2020). Concerning Trends in Maternal Risk Factors in the United States: 1989–2018. EClinicalMedicine 20, 29–30. doi:10.1016/j.eclinm.2020.100657
Brubaker, D. B., Ross, M. G., and Marinoff, D. (1992). The Function of Elevated Plasma Fibronectin in Preeclampsia. Am. J. Obstet. Gynecol. 166, 526–531. doi:10.1016/0002-9378(92)91663-u
Carreiras, M., Montagnani, S., and Layrisse, Z. (2002). Preeclampsia: A Multifactorial Disease Resulting from the Interaction of the Feto-Maternal HLA Genotype and HCMV Infection. Am. J. Reprod. Immunol. 48, 176–183. doi:10.1034/j.1600-0897.2002.01076.x
Ciccarelli, F. D., Doerks, T., von Mering, C., Creevey, C. J., Snel, B., and Bork, P. (2006). Toward Automatic Reconstruction of a Highly Resolved Tree of Life. Science. 311 (5765), 1283–1287. doi:10.1126/science.1123061
Chappell, S., and Morgan, L. (2006). Searching for Genetic Clues to the Causes of Pre-eclampsia. Clin. Sci. (Lond) 110, 443–458. doi:10.1042/cs20050323
Chesley, L. C., Annitto, J. E., and Cosgrove, R. A. (1968). The Familial Factor in Toxemia of Pregnancy. Obstet. Gynecol. 32, 303–311.
Cincotta, R. B., and Brennecke, S. P. (1998). Family History of Pre-Eclampsia as a Predictor for Pre-Eclampsia in Primigravidas. Int. J. Gynecol. Obstet. 60, 23–27. doi:10.1016/s0020-7292(97)00241-5
Cnattingius, S., Reilly, M., Pawitan, Y., and Lichtenstein, P. (2004). Maternal and Fetal Genetic Factors Account for Most of Familial Aggregation of Preeclampsia: A Population-Based Swedish Cohort Study. Am. J. Med. Genet. 130A, 365–371. doi:10.1002/ajmg.a.30257
Emmery, J., Hachmon, R., Pyo, C. W., Nelson, W. C., Geraghty, D. E., Andersen, A. M. N., et al. (2016). Maternal and Fetal Human Leukocyte Antigen Class Ia and II Alleles in Severe Preeclampsia and Eclampsia. Genes Immun. 17, 251–260. doi:10.1038/gene.2016.20
Fan, M., Xu, Y., Hong, F., Gao, X., Xin, G., Hong, H., et al. (2016). Rac1/β-Catenin Signalling Pathway Contributes to Trophoblast Cell Invasion by Targeting Snail and MMP9. Cell Physiol Biochem 38, 1319–1332. doi:10.1159/000443076
Fredrickson, E. K., and Gardner, R. G. (2012). Selective Destruction of Abnormal Proteins by Ubiquitin-Mediated Protein Quality Control Degradation. Semin. Cel Develop. Biol. 23, 530–537. doi:10.1016/j.semcdb.2011.12.006
Furuhashi, N., Kimura, H., Nagae, H., Yajima, A., Kimura, C., and Saito, T. (1993). Serum Laminin Levels in Normal Pregnancy and Preeclampsia. Gynecol. Obstet. Invest. 36, 172–175. doi:10.1159/000292620
Gammill, H. S., Chettier, R., Brewer, A., Roberts, J. M., Shree, R., Tsigas, E., et al. (2018). Cardiomyopathy and Preeclampsia. Circulation 138, 2359–2366. doi:10.1161/circulationaha.117.031527
Genc, M. R., and Schantz-Dunn, J. (2007). The Role of Gene-Environment Interaction in Predicting Adverse Pregnancy Outcome. Best Pract. Res. Clin. Obstet. Gynaecol. 21, 491–504. doi:10.1016/j.bpobgyn.2007.01.009
Genomes Project, C., Auton, A., Brooks, L. D., Durbin, R. M., Garrison, E. P., Kang, H. M., et al. (2015). A Global Reference for Human Genetic Variation. Nature 526, 68–74. doi:10.1038/nature15393
Glotov, A. S., Kazakov, S. V., Vashukova, E. S., Pakin, V. S., Danilova, M. M., Nasykhova, Y. A., et al. (2019). Targeted Sequencing Analysis of ACVR2A Gene Identifies Novel Risk Variants Associated with Preeclampsia. J. Maternal-Fetal Neonatal Med. 32, 2790–2796. doi:10.1080/14767058.2018.1449204
Grewal, S., Carver, J. G., Ridley, A. J., and Mardon, H. J. (2008). Implantation of the Human Embryo Requires Rac1-Dependent Endometrial Stromal Cell Migration. Proc. Natl. Acad. Sci. 105, 16189–16194. doi:10.1073/pnas.0806219105
Hansen, A. T., Bernth Jensen, J. M., Hvas, A.-M., and Christiansen, M. (2018). The Genetic Component of Preeclampsia: A Whole-Exome Sequencing Study. PLoS One 13, e0197217. doi:10.1371/journal.pone.0197217
Huang, D. W., Sherman, B. T., and Lempicki, R. A. (2009a). Bioinformatics Enrichment Tools: Paths toward the Comprehensive Functional Analysis of Large Gene Lists. Nucleic Acids Res. 37, 1–13. doi:10.1093/nar/gkn923
Huang, D. W., Sherman, B. T., and Lempicki, R. A. (2009b). Systematic and Integrative Analysis of Large Gene Lists Using DAVID Bioinformatics Resources. Nat. Protoc. 4, 44–57. doi:10.1038/nprot.2008.211
Jebbink, J., Wolters, A., Fernando, F., Afink, G., Van Der Post, J., and Ris-Stalpers, C. (2012). Molecular Genetics of Preeclampsia and HELLP Syndrome - a Review. Biochim. Biophys. Acta (Bba) - Mol. Basis Dis. 1822, 1960–1969. doi:10.1016/j.bbadis.2012.08.004
Johnson, M. P., Brennecke, S. P., East, C. E., Göring, H. H. H., Kent, J. W., Dyer, T. D., et al. (2012). Genome-Wide Association Scan Identifies a Risk Locus for Preeclampsia on 2q14, Near the Inhibin, Beta B Gene. PLoS One 7, e33666. doi:10.1371/journal.pone.0033666
Kaartokallio, T., Wang, J., Heinonen, S., Kajantie, E., Kivinen, K., Pouta, A., et al. (2016). Exome Sequencing in Pooled DNA Samples to Identify Maternal Pre-Eclampsia Risk Variants. Sci. Rep. 6, 29085. doi:10.1038/srep29085
Kim, S.-Y., Ryu, H.-M., Yang, J. H., Kim, M.-Y., Ahn, H.-K., Lim, H.-J., et al. (2004). Maternal Serum Levels of VCAM-1, ICAM-1 and E-Selectin in Preeclampsia. J. Korean Med. Sci. 19, 688–692. doi:10.3346/jkms.2004.19.5.688
Kircher, M., Witten, D. M., Jain, P., O'roak, B. J., Cooper, G. M., and Shendure, J. (2014). A General Framework for Estimating the Relative Pathogenicity of Human Genetic Variants. Nat. Genet. 46, 310–315. doi:10.1038/ng.2892
Kobayashi, H. (2015). The Impact of Maternal-Fetal Genetic Conflict Situations on the Pathogenesis of Preeclampsia. Biochem. Genet. 53, 223–234. doi:10.1007/s10528-015-9684-y
Li, Y.-H., Zhang, G.-G., and Wang, N. (2017). Systematic Characterization and Prediction of Human Hypertension Genes. Hypertension 69, 349–355. doi:10.1161/hypertensionaha.116.08573
Li, J., Tong, C., Xu, P., Wang, L., Han, T.-l., Wen, L., et al. (2019). QSOX1 Regulates Trophoblastic Apoptosis in Preeclampsia through Hydrogen Peroxide Production. J. Maternal-Fetal Neonatal Med. 32, 3708–3715. doi:10.1080/14767058.2018.1471459
Loscalzo, J., Kohane, I., and Barabasi, A. L. (2007). Human Disease Classification in the Postgenomic Era: A Complex Systems Approach to Human Pathobiology. Mol. Syst. Biol. 3, 124. doi:10.1038/msb4100163
Melton, P. E., Johnson, M. P., Gokhale-Agashe, D., Rea, A. J., Ariff, A., Cadby, G., et al. (2019). Whole-Exome Sequencing in Multiplex Preeclampsia Families Identifies Novel Candidate Susceptibility Genes. J. Hypertens. 37, 997–1011. doi:10.1097/hjh.0000000000002023
Menche, J., Sharma, A., Kitsak, M., Ghiassian, S. D., Vidal, M., Loscalzo, J., et al. (2015). Uncovering Disease-Disease Relationships through the Incomplete Interactome. Science 347, 1257601. doi:10.1126/science.1257601
Mütze, S., Rudnik-Schöneborn, S., Zerres, K., and Rath, W. (2008). Genes and the Preeclampsia Syndrome. J. Perinat Med. 36, 38–58. doi:10.1515/JPM.2008.004
Neerukonda, S., Shariati, F., Hart, T., Stewart, M., Elkayam, U., and Qamruddin, S. (2020). Cardiovascular Effects of Preeclampsia. Curr. Opin. Cardiol. 35, 357–359. doi:10.1097/hco.0000000000000756
Nejatizadeh, A., Stobdan, T., Malhotra, N., and Pasha, M. A. Q. (2008). The Genetic Aspects of Pre-Eclampsia: Achievements and Limitations. Biochem. Genet. 46, 451–479. doi:10.1007/s10528-008-9163-9
Ng, P. C., and Henikoff, S. (2003). SIFT: Predicting Amino Acid Changes that Affect Protein Function. Nucleic Acids Res. 31, 3812–3814. doi:10.1093/nar/gkg509
Nilsson, E., Salonen Ros, H., Cnattingius, S., and Lichtenstein, P. (2004). The Importance of Genetic and Environmental Effects for Pre-eclampsia and Gestational Hypertension: A Family Study. BJOG: Intern. J. Obs Gyn 111, 200–206. doi:10.1111/j.1471-0528.2004.00042x.x
Poplin, R., Ruano-Rubio, V., Depristo, M. A., Fennell, T. J., Carneiro, M. O., Van Der Auwera, G. A., et al. (2018). Scaling Accurate Genetic Variant Discovery to Tens of Thousands of Samples. bioRxiv, 201178. doi:10.1101/201178
Price, A. L., Patterson, N. J., Plenge, R. M., Weinblatt, M. E., Shadick, N. A., and Reich, D. (2006). Principal Components Analysis Corrects for Stratification in Genome-Wide Association Studies. Nat. Genet. 38, 904–909. doi:10.1038/ng1847
Ran, J., Li, H., Fu, J., Liu, L., Xing, Y., Li, X., et al. (2013). Construction and Analysis of the Protein-Protein Interaction Network Related to Essential Hypertension. BMC Syst. Biol. 7, 32. doi:10.1186/1752-0509-7-32
Raymond, D., and Peterson, E. (2011). A Critical Review of Early-Onset and Late-Onset Preeclampsia. Obstet. Gynecol. Surv. 66, 497–506. doi:10.1097/ogx.0b013e3182331028
Roberts, J. M., August, P. A., Bakris, G., Barton, J. R., Bernstein, I. M., Druzin, M., et al. (2013). Hypertension in Pregnancy. Report of the American College of Obstetricians and Gynecologists’ Task Force on Hypertension in Pregnancy. Obstet. Gynecol. 122, 1122–1131. doi:10.1097/01.AOG.0000437382.03963.88
Sado, T., Naruse, K., Noguchi, T., Haruta, S., Yoshida, S., Tanase, Y., et al. (2011). Inflammatory Pattern Recognition Receptors and Their Ligands: Factors Contributing to the Pathogenesis of Preeclampsia. Inflamm. Res. 60, 509–520. doi:10.1007/s00011-011-0319-4
Salonen Ros, H., Lichtenstein, P., Lipworth, L., and Cnattingius, S. (2000). Genetic Effects on the Liability of Developing Pre-eclampsia and Gestational Hypertension. Am. J. Med. Genet. 91, 256–260. doi:10.1002/(sici)1096-8628(20000410)91:4<256:aid-ajmg3>3.0.co;2-t
Serrano, N. C. (2006). Immunology and Genetic of Preeclampsia. Clin. Develop. Immunol. 13, 197–201. doi:10.1080/17402520600876903
Davey Smith, G., and Ebrahim, S. (2003). 'Mendelian Randomization': Can Genetic Epidemiology Contribute to Understanding Environmental Determinants of Disease? Int. J. Epidemiol. 32, 1–22. doi:10.1093/ije/dyg070
Soellner, L., Kopp, K. M., Mütze, S., Meyer, R., Begemann, M., Rudnik, S., et al. (2018). NLRP Genes and Their Role in Preeclampsia and Multi-Locus Imprinting Disorders. J. Perinat Med. 46, 169–173. doi:10.1515/jpm-2016-0405
Steinthorsdottir, V., Mcginnis, R., McGinnis, R., Williams, N. O., Stefansdottir, L., Thorleifsson, G., et al. (2020). Genetic Predisposition to Hypertension Is Associated with Preeclampsia in European and Central Asian Women. Nat. Commun. 11, 5976. doi:10.1038/s41467-020-19733-6
Sutherland, A., Cooper, D. W., Howie, P. W., Liston, W. A., and Macgillivray, I. (1981). The Incidence of Severe Pre-eclampsia Amongst Mothers and Mothers-In-Law of Pre-Eclamptics and Controls. BJOG:An Int. J. O&G 88, 785–791. doi:10.1111/j.1471-0528.1981.tb01304.x
Tan, K. H., Tan, S. S., Sze, S. K., Lee, W. K., Ng, M. J., and Lim, S. K. (2014). Plasma Biomarker Discovery in Preeclampsia Using a Novel Differential Isolation Technology for Circulating Extracellular Vesicles. Am. J. Obstet. Gynecol. 211, 380.e1–380.e13. doi:10.1016/j.ajog.2014.03.038
Than, N. G., Romero, R., Tarca, A. L., Kekesi, K. A., Xu, Y., Xu, Z., et al. (2018). Integrated Systems Biology Approach Identifies Novel Maternal and Placental Pathways of Preeclampsia. Front. Immunol. 9, 1661. doi:10.3389/fimmu.2018.01661
Thomsen, L. C. V., Mccarthy, N. S., Melton, P. E., Cadby, G., Austgulen, R., Nygård, O. K., et al. (2017). The Antihypertensive MTHFR Gene Polymorphism Rs17367504-G Is a Possible Novel Protective Locus for Preeclampsia. J. Hypertens. 35, 132–139. doi:10.1097/hjh.0000000000001131
Triche, E. W., Uzun, A., Dewan, A. T., Kurihara, I., Liu, J., Occhiogrosso, R., et al. (2014). Bioinformatic Approach to the Genetics of Preeclampsia. Obstet. Gynecol. 123, 1155–1161. doi:10.1097/aog.0000000000000293
Valenzuela, F. J., Pérez-Sepúlveda, A., Torres, M. J., Correa, P., Repetto, G. M., and Illanes, S. E. (2012). Pathogenesis of Preeclampsia: The Genetic Component. J. Pregnancy 2012, 632732. doi:10.1155/2012/632732
Van Der Auwera, G. A., Carneiro, M. O., Hartl, C., Poplin, R., Del Angel, G., Levy-Moonshine, A., et al. (2013). From FastQ Data to High Confidence Variant Calls: the Genome Analysis Toolkit Best Practices Pipeline. Curr. Protoc. Bioinformatics 43, 11–33. doi:10.1002/0471250953.bi1110s43
Wall, D. P., Esteban, F. J., Deluca, T. F., Huyck, M., Monaghan, T., Velez De Mendizabal, N., et al. (2009). Comparative Analysis of Neurological Disorders Focuses Genome-Wide Search for Autism Genes. Genomics 93, 120–129. doi:10.1016/j.ygeno.2008.09.015
Wang, K., Li, M., and Hakonarson, H. (2010). ANNOVAR: Functional Annotation of Genetic Variants from High-Throughput Sequencing Data. Nucleic Acids Res. 38, e164. doi:10.1093/nar/gkq603
Ward, K. (2008). Genetic Factors in Common Obstetric Disorders. Clin. Obstet. Gynecol. 51, 74–83. doi:10.1097/grf.0b013e3181616545
Zhang, A., and Huang, S. (2012). Progress in Pathogenesis of Proteinuria. Int. J. Nephrol. 2012, 314251. doi:10.1155/2012/314251
Zhang, L., Cao, Z., Feng, F., Xu, Y.-N., Li, L., and Gao, H. (2020). A Maternal GOT1 Novel Variant Associated with Early-Onset Severe Preeclampsia Identified by Whole-Exome Sequencing. BMC Med. Genet. 21, 49. doi:10.1186/s12881-020-0989-2
Zhao, M., Li, L., Yang, X., Cui, J., and Li, H. (2017). FN1, FOS, and ITGA5 Induce Preeclampsia: Abnormal Expression and Methylation. Hypertens. Pregnancy 36, 302–309. doi:10.1080/10641955.2017.1385795
Keywords: preeclampsia, complex diseases, network biology, protein protein interaction (PPI), computational biology
Citation: Schuster J, Tollefson GA, Zarate V, Agudelo A, Stabila J, Ragavendran A, Padbury J and Uzun A (2022) Protein Network Analysis of Whole Exome Sequencing of Severe Preeclampsia. Front. Genet. 12:765985. doi: 10.3389/fgene.2021.765985
Received: 28 August 2021; Accepted: 03 November 2021;
Published: 02 June 2022.
Edited by:
Ka-Chun Wong, City University of Hong Kong, Hong Kong SAR, ChinaReviewed by:
Neetika Nath, Universitätsmedizin Greifswald, GermanyHaoxiang Cheng, Icahn School of Medicine at Mount Sinai, United States
Copyright © 2022 Schuster, Tollefson, Zarate, Agudelo, Stabila, Ragavendran, Padbury and Uzun. This is an open-access article distributed under the terms of the Creative Commons Attribution License (CC BY). The use, distribution or reproduction in other forums is permitted, provided the original author(s) and the copyright owner(s) are credited and that the original publication in this journal is cited, in accordance with accepted academic practice. No use, distribution or reproduction is permitted which does not comply with these terms.
*Correspondence: Alper Uzun, alper_uzun@brown.edu