- 1Unidad de Genética y Mejora Animal, Departamento de Producción Animal, Facultad de Veterinaria, Universidad de La República, Montevideo, Uruguay
- 2Department of Animal and Dairy Sciences, University of Wisconsin-Madison, Madison, WI, United States
- 3Laboratorio de Endocrinología y Metabolismo Animal, Facultad de Veterinaria, Universidad de La República, Montevideo, Uruguay
- 4Laboratorio de Biología Computacional, Departamento de Desarrollo Biotecnológico, Instituto de Higiene, Facultad de Medicina, Universidad de La República, Montevideo, Uruguay
Several ocular pathologies in cattle, such as ocular squamous cell carcinoma and infectious keratoconjunctivitis, have been associated with low pigmentation of the eyelids. The main objective of this study was to analyze the transcriptome of eyelid skin in Hereford cattle using strand-specific RNA sequencing technology to characterize and identify long noncoding RNAs (lncRNAs). We compared the expression of lncRNAs between pigmented and unpigmented eyelids and analyzed the interaction of lncRNAs and putative target genes to reveal the genetic basis underlying eyelid pigmentation in cattle. We predicted 4,937 putative lncRNAs mapped to the bovine reference genome, enriching the catalog of lncRNAs in Bos taurus. We found 27 differentially expressed lncRNAs between pigmented and unpigmented eyelids, suggesting their involvement in eyelid pigmentation. In addition, we revealed potential links between some significant differentially expressed lncRNAs and target mRNAs involved in the immune response and pigmentation. Overall, this study expands the catalog of lncRNAs in cattle and contributes to a better understanding of the biology of eyelid pigmentation.
1 Introduction
Long noncoding RNAs (lncRNAs) are transcripts longer than 200 bp that do not code for functional proteins (Qureshi et al., 2010). They constitute a highly heterogeneous class of RNAs, including enhancer RNAs, antisense transcripts, and intergenic lncRNAs (Boon et al., 2016). They are transcribed and processed like messenger RNAs (mRNAs): lncRNAs are 3′ polyadenylated, 5′ capped, and multiexonic (Carninci, 2005; Derrien et al., 2012; Li et al., 2019). They have been studied and characterized in various animal species, including mice (Kadakkuzha et al., 2015), humans (Gibb et al., 2011), sheep (Bakhtiarizadeh et al., 2016), goats (Ren et al., 2016; Ling et al., 2017), chickens (You et al., 2019) and cattle (Huang et al., 2012; Weikard et al., 2013; Billerey et al., 2014; Koufariotis et al., 2015; Kern et al., 2018). They are usually expressed at low levels and are under weaker selective constraints than protein-coding genes (Derrien et al., 2012). LncRNAs are poorly conserved among different species compared to mRNAs, and their expression is tissue-specific (Pang et al., 2006; Cabili et al., 2011; Derrien et al., 2012; Kern et al., 2018). Given these characteristics, lncRNAs are regarded as transcriptional “noise” (Chakalova et al., 2005; Ma et al., 2013). In fact, the role of lncRNAs is poorly understood. LncRNAs have been shown to be associated with various critical biological processes, mainly through the regulation of gene expression (Clemson et al., 1996; Gupta et al., 2010; Ma et al., 2013; Weikard et al., 2013; Dempsey and Cui, 2017). LncRNAs can act as signals, decoys, guides and scaffolds to regulate gene expression at the pretranscription, transcription, and post-transcription stages, regulating gene expression in trans and cis (Kornienko et al., 2013; Li et al., 2019). In genome-wide association studies (GWAS), the vast majority of significant single nucleotide polymorphisms (SNPs) are located in non-coding regions (Laurent et al., 2014; Goddard et al., 2016), suggesting that noncoding transcripts could play a more important role than expected, being directly involved in phenotypic variation (Kosinska-Selbi et al., 2020). Notably, the catalog of lncRNAs in livestock animals compared to humans and mice is far from complete (Kern et al., 2018; Kosinska-Selbi et al., 2020).
The study of coat and skin color in cattle has both economic and scientific interest. Several ocular pathologies, such as ocular squamous cell carcinoma, also known as eye cancer, and infectious keratoconjunctivitis, also known as pink eye, have been associated with low pigmentation of the eyelids (Heeney and Valli, 1985; Anderson, 1991). These ocular pathologies have a considerable economic impact since affected animals suffer weight loss and are underpaid at slaughter. Skin pigmentation is a complex, polygenic trait (Cichorek et al., 2013; Ainger et al., 2017). This trait is the result of differences in biochemical processes and the activity of melanocytes (Solano, 2014), which produce two types of melanin, eumelanin (black/brown) and pheomelanin (red/yellow) (Le Pape et al., 2008). The lack of eyelid pigmentation in Hereford cattle is the result of a genetic background that impact melanocyte development, including cell migration. This genetic background may be caused by variations in the expression of gene KIT during embryo development, resulting in impaired migration of melanocyte precursors to the region around the eyes (Grichnik, 2006). The receptor tyrosine kinase KIT and its ligand (KITLG) play an important role in the development of melanocytes, including migration, survival, proliferation, and differentiation (Grichnik, 2006; Mort et al., 2015; Ainger et al., 2017). Gene KITLG has been reported as a candidate gene affecting both eye area pigmentation and eyelid pigmentation in cattle (Pausch et al., 2012; Jara et al., 2022). There are many genes specifically expressed in melanocytes, such as TYR, TYRP1, DCT, PMEL, MITF, and MLANA (D’Mello et al., 2016; Ainger et al., 2017). The regulation of these important genes by lncRNAs has not been studied in relation to eyelid pigmentation in cattle. In recent years, the importance of lncRNAs in the biology of the skin (Wan and Wang, 2014) in different livestock species, including sheep (Yue et al., 2016), goats (Ren et al., 2016), and cattle (Weikard et al., 2013), has been demonstrated. In cattle, 4,848 potential lncRNAs were identified in a study that compared regions of pigmented and non-pigmented skin (body spots) (Weikard et al., 2013). The authors concluded that the transcription pattern of bovine skin is complex and suggested a possible functional relevance of new transcripts, including lncRNAs, in the modulation of pigmentation. The catalog of lncRNAs involved in skin pigmentation in cattle is not very extensive and limited to intergenic lncRNAs (Weikard et al., 2013).
The main objective of this study was to analyze the transcriptome of eyelid skin in Hereford cattle using strand-specific RNA sequencing (ssRNA-seq) to characterize and identify lncRNA possibly involved in eyelid pigmentation. Two contrasting groups were evaluated: steers with completely pigmented eyelids versus steers with no pigmentation in both eyelids (Jara et al., 2020). We evaluated the differential expression of lncRNAs between these two groups and analyzed the interactions between lncRNAs and putative target coding genes to reveal the genetic basis underlying this complex, economically relevant phenotype. Our study provides a valuable resource for the comprehension of lncRNAs, enriches the lncRNA catalog in cattle, and contributes to a better understanding of the molecular mechanisms underlying eyelid pigmentation.
2 Materials and Methods
2.1 Data
These RNA-seq data are available at the NCBI BioProject database with accession number PRJNA627111. The transcriptomes of 11 eyelid skin samples, five samples from 100% pigmented animals, and six samples from 0% pigmented animals were analyzed (Jara et al., 2020). The eyelid transcriptomes were generated using poly-A capture and strand-specific RNA sequencing in an Illumina HiSeq 2500 sequencing system. A total of 542,751,474 (38.5 G) clean reads were obtained. These reads were mapped to the latest cattle (Bos taurus) reference genome ARS-UCD1.2, using the software Hisat2 (v2.1.0) (Kim et al., 2015). The overall mapping rate ranged from 91 to 93%, and only uniquely mapped reads were considered (Supplementary Table S1). Transcriptomes were assembled for each sample using Cufflinks, and then, all the assemblies were merged into one using Cuffmerge.
2.2 Identification of Long NonCoding RNAs
Potential lncRNAs were identified using successive filters starting from all obtained transcripts. First, transcripts that presented the class code “ = “, “e”, “p”, and “c” from the output generated by Cuffcompare were filtered out (You et al., 2019). Transcripts with less than two exons with low expression levels (ten mapped reads per sample in at least five biological replicates were defined as the expression threshold) and shorter than 200 bp were also filtered out. DNA sequences of the transcripts were extracted using gffread (Trapnell et al., 2010). The sequences of known lncRNAs were downloaded from two multispecies lncRNA databases, ALDB (Domestic-Animal LncRNA Database) (Li A. et al., 2015) and NONCODE (Zhao Y. et al., 2016), which contain 8,250 and 23,515 cattle lncRNAs, respectively. BLASTn (version 2.2.31) (Altschul et al., 1990) was used to align the unannotated transcripts to lncRNAs in these two databases using stringent parameters (e-value ≤ 1 × 106, coverage and identity ≥90%). Accurately aligned transcripts against either ALDB or NONCODE (5.0) were regarded as known lncRNAs. On the other hand, the transcripts that did not align with sequences in either ALDB or NONCODE were considered putative novel lncRNAs. This pipeline was adapted from Konsinska-Selbi et al. (2020).
2.3 Coding Potential Analysis
We calculated the coding potential of each transcript using three complementary tools: Coding Potential Calculator 2 (CPC2, version 0.1) (Kang et al., 2017), Coding-Potential Assessment Tool (CPAT, version 2.0.0) (Wang et al., 2013), and Predictor of long noncoding RNAs and messenger RNAs based on an improved k-mer scheme (PLEK, version 1.2) (Li et al., 2014). PLEK and CPC2 are based on the same support vector machine-based (SVM) classification model, while CPAT is based on a logistic regression (Wang et al., 2013; Antonov et al., 2019). All these programs are alignment-free tools and have been proven to be highly effective in discriminating lncRNAs (Han et al., 2016).
The CPAT program estimates coding probability scores. The optimum cutoff value for protein-coding probability is species-specific, and hence, the CPAT was trained using a set of 10,000 known bovine protein-encoding transcripts (Bos_taurus.ARS-UCD1.2.cds.all.fa, version 95) and a set of 10,000 bovine noncoding sequences (larger than 200 bases). The final reference dataset of noncoding RNAs comprised 37,695 sequences (5,930 from Bos_taurus.ARS-UCD1.2.ncrna.fa (version 95), 23,515 from NONCODEv5_cow.fa, and 8,250 from ALDB.cow.lincRNAs.v1.0.fa). Bovine protein-coding transcripts and noncoding sequences were extracted randomly from each annotation, following previously published studies (Billerey et al., 2014; Gupta et al., 2019). In brief, the two training sets were randomly split into ten different parts to perform a 10-fold cross-validation analysis. The cutoff value was selected to maximize specificity and sensitivity. On the other hand, PLEK uses a sliding window-based approach to analyze the transcripts based on a k-mer frequency distribution. PLEK was trained using the same set of sequences that were used for training the CPAT program.
Transcripts that displayed a CPC2 score lower than 0.5, a CPAT score lower than or equal to 0.36, and a PLEK score lower than 0 were considered noncoding genes and were used in subsequent analyses.
2.4 Gene Expression Analysis
Differentially expressed genes were identified using the R package DESeq2 (version 1.18.1) with default parameters (Love et al., 2014). Putative novel lncRNAs, known lncRNAs, and annotated protein-coding genes were all included in this statistical analysis. Only genes with at least ten reads per sample in at least five biological replicates were considered. Genes with an adjusted p-value ≤ 0.05 (Benjamini and Hochberg, 1995) and a |log2FC| ≥ 1.5 were considered differentially expressed (DEs) between pigmented and unpigmented samples.
2.5 Sequence Analysis
The analyses of the different sequence characteristics were performed on 14,361 coding genes (34,447 transcripts), 4,937 putative novel lncRNAs, and 218 known lncRNAs. The GC content and the length of transcripts were obtained using the infoseq function of the EMBOSS package version: 6.6.0.0. The exon number was estimated using custom scripts in bash. The abundance was estimated using Cuffnorm. The minimum free energy (ME) was calculated using the RNAfold program included in the ViennaRNA package version 2.4.9 (Zuker and Stiegler, 1981; Lorenz et al., 2011). To make the ME value of the different RNA sequences comparable, we normalized the ME by the sequences’ length, yielding the MEN. Thus, MEN was calculated by dividing the ME value by the transcript’s size and multiplying it by 100. Thus, the MEN value relates the ME estimation to a segment of 100 nucleotides: MEN = (ME/sequence length)*100 (Zhang et al., 2006).
2.6 Prediction of Target Genes
Two strategies were used to study the association between lncRNAs and target genes acting in cis and trans. In the first case, all “neighbor” protein-coding genes showing differential expression (DEGs, p-value ≤ 0.01, and |log2FC| ≥ 1) were identified in a range of 300 kb upstream and downstream of differentially expressed lncRNAs. Genes that showed significant correlation coefficients at the expression level with neighboring lncRNAs were considered cis target genes (Pearson, r ≥ |0.60|, p-value ≤ 0.05).
For potential associations in trans with differentially expressed protein-coding genes (DEGs, p-value ≤ 0.01 and |log2FC| ≥ 1), sequence complementarity with differentially expressed lncRNAs (p adjust ≤0.05 and |log2FC| ≥ 1.5) was analyzed using LncTar (Li J. et al., 2015). Note that a more stringent threshold was used to detect DE lncRNAs than to detect DE protein-coding genes to obtain a more comprehensive and diverse sample of genes and their functions. The program LncTar identifies potential lncRNA targets by finding the minimum free energy of lncRNA and mRNA pair joint structures. This program was run with a threshold value of ndG −0.08. All predicted interactions of lncRNAs with target mRNAs that showed a significant coexpression correlation (Pearson, r ≥ |0.60|, p-value ≤ 0.05) were kept for further analysis.
The functional roles of the target genes were evaluated using the R package EnrichKit (https://github.com/liulihe954/EnrichKit). Different gene set databases, including GO, MeSH, Reactome, InterPro, and MsigDB, were interrogated in the enrichment analysis. Terms significantly enriched within target genes were detected using Fisher’s exact test, a test of proportions based on the hypergeometric distribution.
2.7 RNA-Seq Data Validation
The results of the RNA-seq analysis were validated using real-time polymerase chain reaction (qRT-PCR). We selected three differentially expressed lncRNAs and three differentially expressed protein-coding genes for validation. Primer sequences and expected product lengths are listed in Supplementary Table S2. Real-time PCRs were performed using 7.5 µL of SYBR® Green master mix (Maxima SYBR Green qPCR Master Mix (2X), with separate ROX vials, Thermo Scientific™, United States of America), equimolar amounts of forward and reverse primers (200 nM, Operon Biotechnologies GmbH, Cologne, Germany), and 20 ng diluted cDNA (1:7.5 in RNase/DNase free water) in a final volume of 15 µL. Samples were analyzed in duplicate in a 72-disk Rotor-GeneTM 6000 (Corbett Life Sciences, Sydney, Australia). Standard amplification conditions were 10 min at 95°C and 40 cycles of 15 s at 95°C, 30 s at 60°C, and 15 s at 72°C. At the end of each run, dissociation curves were analyzed to ensure that the desired amplicon was detected, discarding contaminating DNA or primer dimers. Gene expression was normalized using ACTG1 as a housekeeping gene. Normalized gene expression values (ΔCt) were analyzed using a linear model including the pigmentation group as an independent variable. The association between the normalized gene expression and the pigmentation group was tested using a t-test. The mean and the range of the log2-fold change for each gene were calculated as log2 (2−ΔΔCt) using the estimated ΔΔCt value ±standard error.
3 Results and Discussion
The aim of this study was to identify and analyze lncRNAs associated with eyelid pigmentation in Hereford cattle. Our findings provide further evidence that lncRNAs are actively involved in pigmentation, as suggested by previous studies not only in cows (Weikard et al., 2013) but also in pigs (Zou et al., 2018), goats (Ren et al., 2016), humans (Zhao W. et al., 2016; Tang et al., 2020) and invertebrate organisms such as Crassostrea gigas (Feng et al., 2018).
3.1 Identification of Long NonCoding RNAs
A total of 111,791 transcripts, including 27,806 unannotated transcripts, were obtained after mapping the sequencing reads to the latest bovine genome reference. Among the novel transcripts, 218 were detected in the ALDB and NONCODE databases and were therefore considered known lncRNAs (Supplementary Data Sheet S1). After training, a coding potential cutoff of 0.36 was selected for the CPAT. This value maximizes specificity and sensitivity (98.3%) (see Supplementary Figure S1), similar to other studies (Billerey et al., 2014; Gupta et al., 2019). All transcripts with a score below 0.36 were retained as potential long noncoding RNAs. After integrating the results from CPC2, CPAT, and PLEK, we identified a total of 4,937 unannotated transcripts as putative lncRNAs (Figure 1, Supplementary Figure S2).
Based on RNA-seq analysis of 18 different tissues, Koufariotis et al. (2015) identified 9,778 lncRNAs in cattle. Of special interest, seven lncRNAs were found to be differentially expressed between white and black skin samples (Koufariotis et al., 2015). A recent paper reported a total of 1,535 expressed lncRNAs encoded by 1,183 putative noncoding genes in bovine oocytes (Wang et al., 2020). More specifically, Weikard et al. (2013) identified 4,848 lncRNAs that are associated with pigmentation in cattle, supporting the idea that lncRNAs play an important role in skin pigmentation. Notably, this number of lncRNAs is similar to the 4,937 transcripts that we reported in the present study, although only 314 were in common. The difference between studies could be explained in terms of the analytical pipeline used to identify putative lncRNAs in each case. Note that at the time of writing this manuscript, there is no widely accepted pipeline to identify lncRNAs.
3.2 Comparison Between mRNAs, Novel Long NonCoding RNAs, and Known Long NonCoding RNAs
We identified a total of 4,937 novels and 218 known lncRNAs in our RNA-seq dataset. The lncRNAs were characterized as novel isoforms (51.5%), sense exon overlap (14.5%), antisense intron overlap (12.3%), intergenic (16.5%), and antisense exon overlap (5.2%) forms (Supplementary Figure S3, Supplementary Data Sheet S1). Of the 51.5% characterized as novel isoforms, 94% were generated from regions that harbor protein-coding genes while 6% were novel isoforms from known lncRNAs. Note that similar results were recently reported by Alexandre et al. (2020) working on lncRNAs associated with feed efficiency in cattle.
Novel and known lncRNAs were evenly distributed across the whole genome, ranging from 1.3% (novel lncRNAs) and 0.45% (known lncRNAs) in BTA20 to 6.6% (novel lncRNAs) and 6.8% (known lncRNAs) in BTA3 (Figure 2).
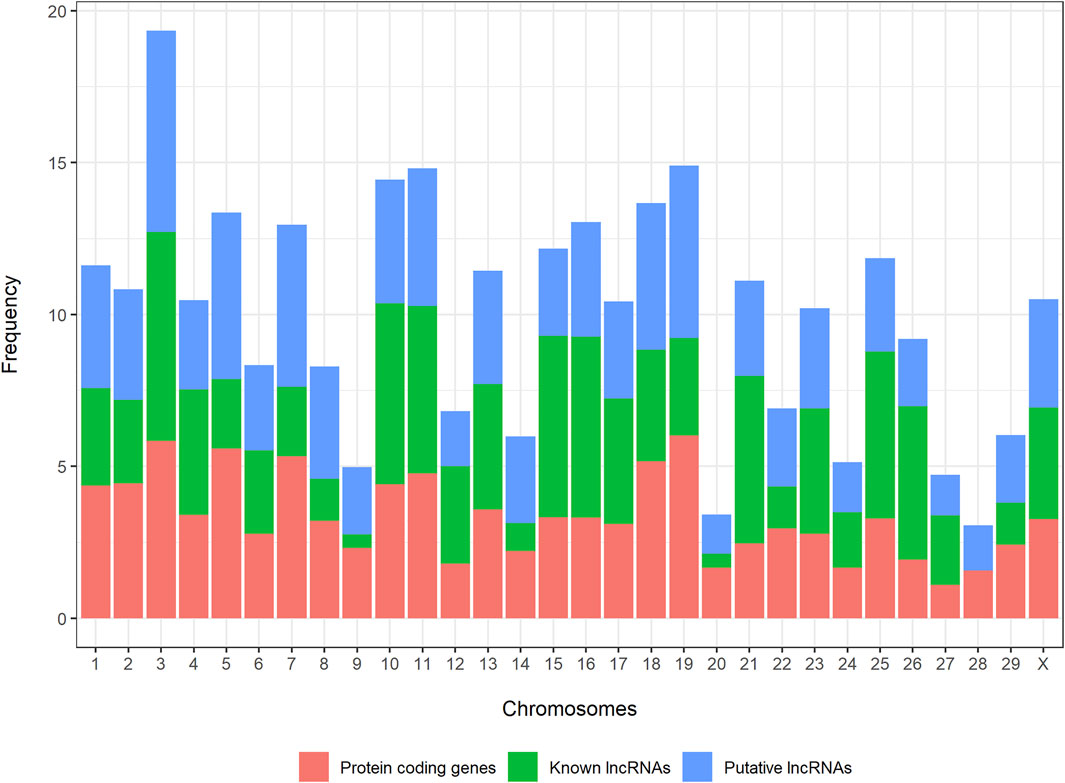
FIGURE 2. Distribution of novel long noncoding RNAs, known long noncoding RNAs, and protein-coding genes across the bovine genome.
We analyzed the guanine-cytosine content (GC), normalized minimum free energy (MEN), transcript length, exon number, and expression level of all putative novel lncRNAs and compared these metrics with those from known lncRNAs and protein-coding genes. Both groups of lncRNAs displayed significantly lower GC content (Figure 3B), shorter length (Figure 3C), and higher MEN (Figure 3D) than protein-coding genes (p-value ≤ 0.05, Wilcoxon test, Table 1). We found no significant differences in the GC content nor in the MEN between the novel and known lncRNAs (p-value = 0.37 and p-value = 0.55, Wilcoxon test, respectively, Table 1). These results agree with previous studies that showed that lncRNAs have some unique sequence features compared to coding genes (Niazi and Valadkhan, 2012).
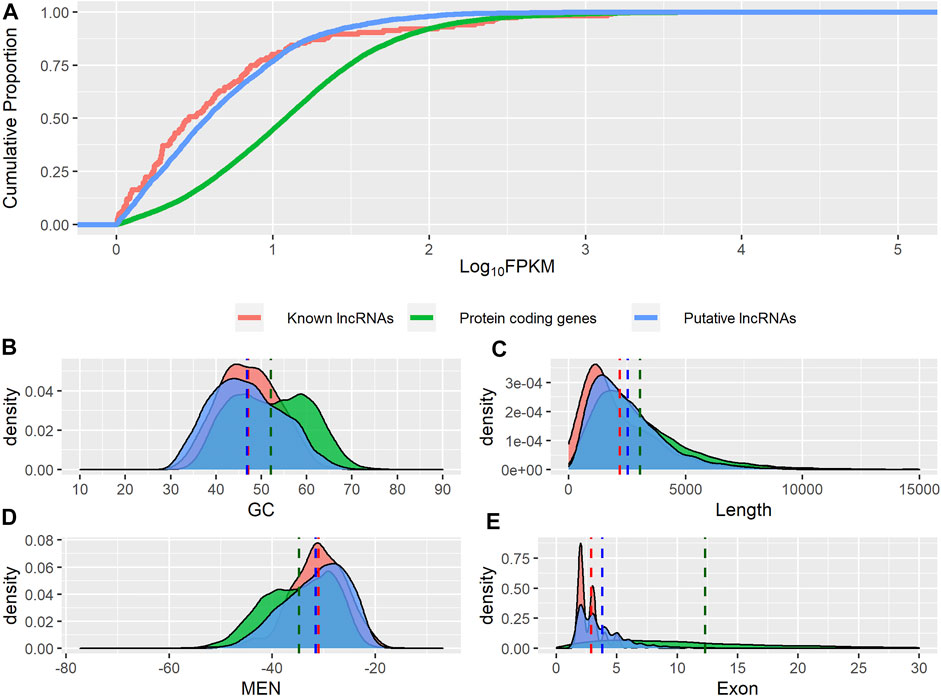
FIGURE 3. Genomic features of putative lncRNAs, known lncRNAs, and protein-coding genes. (A) Comparison of expression level. (B) Distribution of guanine-cytosine content (GC). (C) Distribution of transcripts length. (D) Distribution of normalized minimum free energy (MEN). (E) Distribution of number of exons.
Both novel and putative lncRNAs showed significantly higher MEN values than protein-coding genes (p-value ≤ 0.05, Wilcoxon test, Table 1). The sequence itself seems to contribute considerably to MEN since sequences with the same GC content can potentially present different folding energy values based on their secondary structure (Niazi and Valadkhan, 2012). It can be inferred that lncRNAs have a more flexible structure than mRNAs, which may reflect a higher potential to interact with other molecules. Indeed, it has been shown that lncRNAs have GC and MEN values similar to those of 3′ UTR sequences, suggesting that lncRNAs have regulatory functions (Niazi and Valadkhan, 2012).
We found that the novel lncRNAs were significantly shorter than the known lncRNAs (p-value ≤ 0.05, Wilcoxon test, Table 1). Both groups of noncoding transcripts showed lower expression levels (Figure 3A) and a lower number of exons (Figure 3E) than protein-coding genes (p-value ≤ 0.05, Wilcoxon test).
Overall, our results showed that lncRNAs are characterized by a lower number of exons, lower GC content, lower expression level, and higher normalized minimum free energy and tend to be shorter than protein-coding sequences (Derrien et al., 2012; Harrow et al., 2012; Billerey et al., 2014). Note that these sequence feature analyses support the reliability of the putative novel lncRNAs identified. We believe that the marginal differences observed in the length, expression level, and lower number of exons between the novel and known lncRNAs could be explained by the limited catalog of lncRNAs in cattle (Kosinska-Selbi et al., 2020). However, we could not completely discard the possibility that some of our novel lncRNAs are either pseudogenes or misidentified coding sequences.
3.3 Expression Analysis
We found 65 differentially expressed protein-coding genes (p adjusted ≤0.05 and |log2 FC| ≥1.5) between the pigmented and unpigmented samples (Appendix S2). Among them, MC1R, TYR, PMEL, DCT, MLANA, and KIT showed upregulated expression in pigmented eyelid samples (Jara et al., 2020). These genes are key for the generation, storage, and distribution of melanin (D’Mello et al., 2016). Gene KIT encodes a receptor of tyrosine kinase and is considered a proto-oncogene, involved in the development of melanocytes, including migration, survival, proliferation, and differentiation (Grichnik, 2006). Previous studies showed the important role of KIT in UVB-induced epidermal melanogenesis in humans (Yamada et al., 2013) and in the survival of melanocytes (Mort et al., 2015). Here, KIT showed higher expression in pigmented eyelid samples, suggesting an important role in pigmentation. Interestingly, our analyses suggest that lncRNAs do not interact directly with KIT, and hence, it seems this gene is not directly regulated by these non-coding elements. Moreover, we identified a total of 27 differentially expressed lncRNAs (p adjusted ≤0.05 and |log2FC| ≥ |1.5|); twenty-four were putative novel lncRNAs, and three were classified as known lncRNAs. Eight lncRNAs showed upregulated and 19 lncRNAs showed downregulated expression in the pigmented eyelid samples. The top lncRNA with significantly downregulated expression was TCONS_00073858 (novel) with log2FoldChange = −10.3, while the top lncRNA with upregulated expression was TCONS_00088900 (novel) with log2FoldChange = 7.7.
3.4 Association of Long NonCoding RNAs With Putative Target Genes Acting in cis
We analyzed potential target mRNAs of lncRNAs acting in cis, that is, protein-coding genes within 300 kb upstream and downstream of the location of significant differentially expressed lncRNAs. We found four putative target genes, namely, CXCL13, FABP4, GPR143, and UGT1A1, in the flanking regions of four differentially expressed lncRNAs, TCONS_00094682 (novel), TCONS_00021379 (novel), TCONS_00109545 (novel), and TCONS_00078693 (novel), respectively (Table 2). The lncRNAs TCONS_00094682 and TCONS_00021379 showed downregulated expression in the pigmented samples and acted at the cis level with the CXCL13 and FABP4 genes, respectively. The CXCL13 gene encodes a homeostatic chemokine that traffics B cells and is involved in the immune response (Müller et al., 2002). Fatty acid transporters, such as FABP4, were recently shown to increase their expression during metastatic melanoma development, suggesting a higher uptake of fatty acids and metabolic reprogramming that serves as a signature for this condition (Lee et al., 2020). In contrast, the lncRNAs TCONS_00109545 and TCONS_00078693 showed upregulated expression in the pigmented samples. The lncRNA TCONS_00109545 interacts at the cis level with the GPR143 gene, which plays a critical role in retinal health and development (McKay, 2019). Mutations in GPR143 have been associated with the ocular albinism type 1 (OA1) phenotype (Gao et al., 2020), a genetic disorder characterized by reduced ocular pigmentation. Finally, lncRNA TCONS_00078693 was correlated with the expression of UGT1A1, a gene implicated in retinoic acid binding. Retinoid signaling is affected in early carcinogenesis (Tang and Gudas, 2011), and retinoic acid is often used to prevent photoaging of human skin, preventing melanocytic and keratinocytic atypia (Cho et al., 2005). Note that all these lncRNAs showed a positive correlation with their target genes, and hence, we hypothesize that these lncRNAs interact at the cis level, promoting the expression of these genes through the recruitment of proteins that enable transcription loops (Long et al., 2017; Li et al., 2019; Gil and Ulitsky, 2020).
3.5 Association of Long NonCoding RNAs With Putative Target Genes Acting in Trans
We analyzed the potential target mRNAs of lncRNAs acting in trans from a total of 273 differentially expressed genes that showed sequence complementarity with at least one of the twenty-seven differentially expressed lncRNAs. Of these 273 genes, 193 showed sizable expression correlations with significant lncRNAs (Pearson, r > |0.60|, p-value < 0.05) (Supplementary Data Sheet S3); hence, they were classified as potential trans target genes. We identified target genes involved in melanogenesis, melanosome development (Lin and Fisher, 2007; Kubic et al., 2008; Schmutz and Dreger, 2013), pigmentation processes (Lalueza-Fox et al., 2007; Enriqué Steinberg et al., 2013), tumor pathways (Hoashi et al., 2005), innate immunity and inflammatory signaling (Ito et al., 2015), such as MC1R, PMEL, MLANA, PAX3, IGFBP2, FGF23, and TREM-2. Interestingly, many of these potential trans target genes were reported previously as differentially expressed in pigmented versus non-pigmented samples (Jara et al., 2020) (Supplementary Figure S4, Supplementary Data Sheet S3). The genes MC1R, PMEL, MLANA, and PAX3 showed upregulated expression in the pigmented samples and interacted with lncRNAs with up- and down-regulated expression (Supplementary Data Sheet S2, S3). MC1R is a key pigmentation gene, and its activation in melanocytes stimulates melanogenesis, particularly eumelanogenesis (Beaumont et al., 2007; Cheli et al., 2010; Enriqué Steinberg et al., 2013). The PMEL gene is a key component of mammalian melanosome biogenesis (Clark et al., 2006), and it is required for the generation of cylindrical melanosomes in zebrafish (Watt et al., 2013; Burgoyne et al., 2015). Mutations in PMEL have been shown to regulate hypopigmented phenotypes in vertebrates (Kwon et al., 1995; Kerje et al., 2004). The transcription factor PAX3 interacts with lncRNA TCONS_00088900 and regulates the expression of MITF (Lin and Fisher, 2007), a gene associated with ambilateral circumocular pigmentation in cattle (Pausch et al., 2012). MC1R and MLANA expression levels showed significant positive correlations with the novel lncRNAs TCONS_00088900 and TCONS_00091890, while PMEL also showed a significant positive correlation with TCONS_00091890. MLANA encodes a protein (MART-1) that is localized in melanosomes (De Maziere et al., 2002). Interestingly, MLANA interacts with PMEL and regulates its expression, stability, trafficking, and processing (Hoashi et al., 2005). MLANA showed negative correlations with the lncRNAs ALDBBTAT0000004273 and TCONS_00106295. LncRNAs have different ways of affecting the transcription of target genes in trans, for example, by stabilizing the mRNA (Cao et al., 2017; Long et al., 2017; Li et al., 2019). The participation of lncRNAs in mRNA stabilization and in increasing the expression of specific genes has been recently discussed (Li et al., 2019). From our results, we can infer that ALDBBTAT0000004273 and TCONS_00106295 could be involved in reducing the stability of the mRNA encoded by the MLANA gene and consequently contributing to reduced expression in unpigmented samples. Additionally, the lncRNAs could be involved in the stabilization of the mRNAs of certain genes responsible for pigmentation, such as PEML and MC1R, and thus enhance their expression levels in pigmented eyelids. The genes IGFBP2, FGF23, and TREM-2 showed downregulated expression in the pigmented samples and interacted with lncRNAs with up- and down-regulated expression (Supplementary Data Sheet S2, S3).The FGF23 gene may act as a proinflammatory cytokine (Ito et al., 2015), suggesting a connection between FGF23 and inflammatory processes (Courbebaisse and Lanske, 2018). In fact, high expression of FGF23 is associated with an increased risk of mortality, likely because of its contribution to decreased host defense response to infection, inflammation, and anemia (Courbebaisse and Lanske, 2018). FGF23 showed positive correlations with the ALDBBTAT0000004273 and TCONS_00106295 lncRNAs. The IGFBP2 gene is involved in tumor pathways (Pickard and McCance, 2015) and showed positive correlations with the lncRNAs ALDBBTAT0000001157 and TCONS_00106295. The fatty acid-binding protein 7 (FABP7) gene was also described as a key regulator of cancer metastasis (Cordero et al., 2019), and its expression was upregulated in pigmented samples (Jara et al., 2020). This gene showed significant positive and negative correlations with several lncRNAs. The TREM-2 gene showed a positive correlation with lncRNA TCONS_00073858, which is implicated in innate immunity and inflammatory signaling (Sharif and Knapp, 2008). TREM (TREM-1/TREM-2) gene expression is lower in cutaneous melanoma versus control samples (Nguyen et al., 2015), and these genes could have prognostic and therapeutic value in the treatment of melanoma (Nguyen et al., 2015; Nguyen et al., 2016). From our results, it can be speculated that the lncRNAs described here are involved in stabilizing the mRNAs of genes involved in the immune system and cancer, such as FGF23 (Courbebaisse and Lanske, 2018), IGFBP2 (Pickard and McCance, 2015) and TREM-2.
3.6 Gene Set Enrichment Analysis of Putative Target Genes
A total of 193 genes were identified as putative targets of DE lncRNAs in pigmented samples. To identify enriched functions among them, we performed a gene set enrichment analysis using the annotation retrieved from different databases, including GO, KEGG, MeSH, InterPro, Reactome, and MSigDB. Figure 4 shows the most relevant biological terms and pathways associated with eyelid pigmentation. The most significant functional terms were related to skin pigmentation, such as melanosome (GO:0042470), melanin biosynthetic process (GO:0042438), melanosome organization (GO:0032438), melanocyte differentiation (GO:0030318), melanogenesis (bta04916), skin pigmentation (D012880), pigmentation (D010858) and melanogenesis (M7761) (Figure 4, Supplementary Data Sheet S4). Notably, we found several significant terms related to the inflammatory response and infectious and tumoral pathways: immune response (GO:0006955), defense response to bacterium (GO:0042742), leukocyte chemotaxis (GO:0030595), cytokine-cytokine receptor interaction (bta04060), T cell activation (GO:0042110), cytokine-cytokine receptor interaction (bta04060), immune response (M12401), and immune (humoral) and inflammatory response (M8838) (Figure 4, Supplementary Data Sheet S4). Our findings indicate that target genes of lncRNAs with up- and down-regulated expression in eyelid skin are associated not only with pigmentation or melanogenesis but also with the immune response.
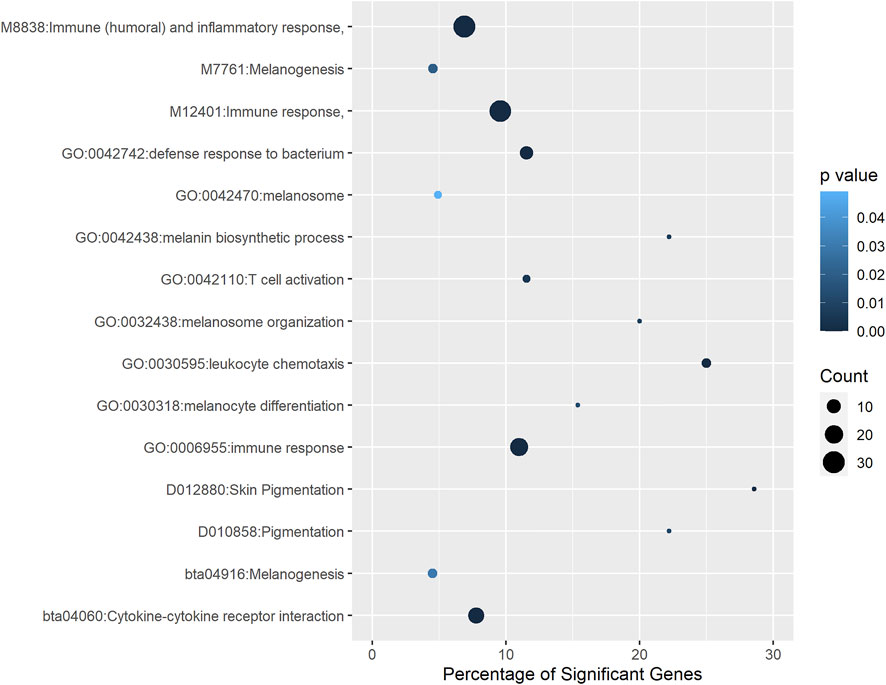
FIGURE 4. Functional terms and pathways significantly enriched with genes associated with eyelid pigmentation. Different annotation databases, including GO, Medical Subject Headings, InterPro, Reactome and MSigDB, were used.
3.7 Validation of Gene Expression Using Quantitative Real-Time Polymerase Chain Reaction
We validated the findings of the RNA-seq experiment using qRT-PCR. Three lncRNAs and three protein-coding genes were evaluated. Figure 5 shows the log2-fold differences in gene expression measured by both RNA-Seq and qRT-PCR, confirming that the six genes showed very similar patterns of abundance with both methods.
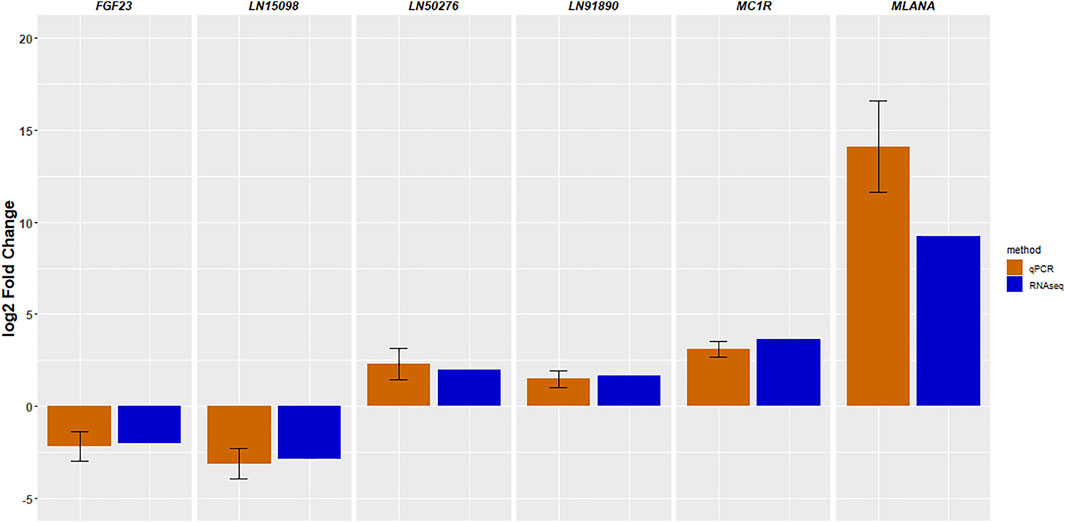
FIGURE 5. Validation of RNA-sequencing results by quantitative RT-PCR. The data are shown as the mean ± standard error.
4 Conclusion
In this work, we described the expression patterns of lncRNAs in the eyelid skin of cattle. We predicted 4,937 putative novel lncRNAs, mapped them to the latest bovine reference genome, and compared their sequence features to those of known lncRNAs and protein-coding genes. A total of 27 lncRNAs were identified as differentially expressed between the pigmented and unpigmented samples. Potential associations were found between specific lncRNAs and putative target genes directly implicated in pigmentation, immune responses, and cancer development. Overall, our study enriches the catalog of lncRNAs in B. taurus, specifically those related to the regulation of eyelid skin pigmentation. Future functional studies should further evaluate the biological functions of these significant lncRNAs.
Data Availability Statement
The original contributions presented in the study are included in the article/Supplementary Material, further inquiries can be directed to the corresponding author.
Author Contributions
EJ, FP, EA, and AI designed the experiments. GR and LT extracted the eyelid samples. EJ and CM analyzed the data. EJ drafted the manuscript. EJ examined the data. FP, EA, and AI reviewed and edited the manuscript. All authors agreed with the manuscript.
Funding
This study was funded by the Comisión Sectorial de Investigación Científica (Universidad de la República, Uruguay) (CSIC INI 2017 #362). The funders had no role in the study design, data collection, analysis, decision to publish, or manuscript preparation. FP, EA, and AI are members of Sistema Nacional de Investigadores, Uruguay. EA, and AI are members of Programa de Desarrollo de las Ciencias Básicas (PEDECIBA), Uruguay.
Conflict of Interest
The authors declare that the research was conducted in the absence of any commercial or financial relationships that could be construed as a potential conflict of interest.
Publisher’s Note
All claims expressed in this article are solely those of the authors and do not necessarily represent those of their affiliated organizations, or those of the publisher, the editors and the reviewers. Any product that may be evaluated in this article, or claim that may be made by its manufacturer, is not guaranteed or endorsed by the publisher.
Supplementary Material
The Supplementary Material for this article can be found online at: https://www.frontiersin.org/articles/10.3389/fgene.2022.864567/full#supplementary-material
Abbreviations
CPC2, Coding Potential Calculator 2; CPAT, Coding-Potential Assessment Tool; PLEK, Predictor of long noncoding RNAs and messenger RNAs based on an improved k-mer scheme; FPKM, fragments per kilobase of transcript per million mapped reads; GO, Gene Ontology; KEGG, Kyoto Encyclopedia of Genes and Genomes; MeSH, Medical Subject Headings; MSigDB, Molecular Signatures Database; lncRNAs, long noncoding RNAs; qRT-PCR, real-time quantitative polymerase chain reaction; NCBI, National Center for Biotechnology Information.
References
Ainger, S. A., Jagirdar, K., Lee, K. J., Soyer, H. P., and Sturm, R. A. (2017). Skin Pigmentation Genetics for the Clinic. Dermatology 233 (1), 1–15. doi:10.1159/000468538
Alexandre, P. A., Reverter, A., Berezin, R. B., Porto-Neto, L. R., Ribeiro, G., Santana, M. H. A., et al. (2020). Exploring the Regulatory Potential of Long Non-coding RNA in Feed Efficiency of Indicine Cattle. GenesGenes 11 (9), 997PMC7565090. doi:10.3390/genes110909975
Altschul, S. F., Gish, W., Miller, W., Myers, E. W., and Lipman, D. J. (1990). Basic Local Alignment Search Tool. J. Mol. Biol. 215 (3), 403–410. doi:10.1016/s0022-2836(05)80360-2
Anderson, D. E. (1991). Genetic Study of Eye Cancer in Cattle. J. Hered. 82 (1), 21–26. doi:10.1093/jhered/82.1.21
Antonov, I. V., Mazurov, E., Borodovsky, M., and Medvedeva, Y. A. (2019). Prediction of lncRNAs and Their Interactions with Nucleic Acids: Benchmarking Bioinformatics Tools. Brief. Bioinform 20 (2), 551–564. doi:10.1093/bib/bby032
Bakhtiarizadeh, M. R., Hosseinpour, B., Arefnezhad, B., Shamabadi, N., and Salami, S. A. (2016). In Silico prediction of Long Intergenic Non-coding RNAs in Sheep. Genome 59 (4), 263–275. doi:10.1139/gen-2015-0141
Beaumont, K. A., Shekar, S. L., Newton, R. A., James, M. R., Stow, J. L., Duffy, D. L., et al. (2007). Receptor Function, Dominant Negative Activity and Phenotype Correlations for MC1R Variant Alleles. Hum. Mol. Genet. 16 (18), 2249–2260. doi:10.1093/hmg/ddm177
Benjamini, Y., and Hochberg, Y. (1995). Controlling the False Discovery Rate: a Practical and Powerful Approach to Multiple Testing. J. R. Stat. Soc. Ser. B Methodol. 57 (1), 289–300. doi:10.1111/j.2517-6161.1995.tb02031.x
Billerey, C., Boussaha, M., Esquerré, D., Rebours, E., Djari, A., Meersseman, C., et al. (2014). Identification of Large Intergenic Non-coding RNAs in Bovine Muscle Using Next-Generation Transcriptomic Sequencing. BMC Genomics 15 (1), 1–10. doi:10.1186/1471-2164-15-499
Boon, R. A., Jaé, N., Holdt, L., and Dimmeler, S. (2016). Long Noncoding RNAs. J. Am. Coll. Cardiol. 67 (10), 1214–1226. doi:10.1016/j.jacc.2015.12.051
Burgoyne, T., O'Connor, M. N., Seabra, M. C., Cutler, D. F., and Futter, C. E. (2015). Regulation of Melanosome Number, Shape and Movement in the Zebrafish Retinal Pigment Epithelium by OA1 and PMEL. J. Cell Sci. 128 (7), 1400–1407. doi:10.1242/jcs.164400
Cabili, M. N., Trapnell, C., Goff, L., Koziol, M., Tazon-Vega, B., Regev, A., et al. (2011). Integrative Annotation of Human Large Intergenic Noncoding RNAs Reveals Global Properties and Specific Subclasses. Genes Dev. 25 (18), 1915–1927. doi:10.1101/gad.17446611
Cao, L., Zhang, P., Li, J., and Wu, M. (2017). LAST, a C-Myc-Inducible Long Noncoding RNA, Cooperates with CNBP to Promote CCND1 mRNA Stability in Human Cells. Elife 6, e30433. doi:10.7554/eLife.30433
Carninci, P. (2005). FANTOM Consortium; RIKEN Genome Exploration Research Group and Genome. Science 309, 1559–1563. doi:10.1126/science.1112014
Chakalova, L., Debrand, E., Mitchell, J. A., Osborne, C. S., and Fraser, P. (2005). Replication and Transcription: Shaping the Landscape of the Genome. Nat. Rev. Genet. 6 (9), 669–677. doi:10.1038/nrg1673
Cheli, Y., Ohanna, M., Ballotti, R., and Bertolotto, C. (2010). Fifteen-year Quest for Microphthalmia-Associated Transcription Factor Target Genes. Pigment Cell & melanoma Res. 23 (1), 27–40. doi:10.1111/j.1755-148X.2009.00653.x
Cho, S., Lowe, L., Hamilton, T. A., Fisher, G. J., Voorhees, J. J., and Kang, S. (2005). Long-term Treatment of Photoaged Human Skin with Topical Retinoic Acid Improves Epidermal Cell Atypia and Thickens the Collagen Band in Papillary Dermis. J. Am. Acad. Dermatology 53 (5), 769–774. doi:10.1016/j.jaad.2005.06.052
Cichorek, M., Wachulska, M., Stasiewicz, A., and Tymińska, A. (2013). Skin Melanocytes: Biology and Development. pdia 1 (1), 30–41. doi:10.5114/pdia.2013.33376
Clark, L. A., Wahl, J. M., Rees, C. A., and Murphy, K. E. (2006). Retrotransposon Insertion in SILV Is Responsible for Merle Patterning of the Domestic Dog. Proc. Natl. Acad. Sci. U.S.A. 103 (5), 1376–1381. doi:10.1073/pnas.0506940103
Clemson, C. M., McNeil, J. A., Willard, H. F., and Lawrence, J. B. (1996). XIST RNA Paints the Inactive X Chromosome at Interphase: Evidence for a Novel RNA Involved in Nuclear/chromosome Structure. J. Cell Biol. 132 (3), 259–275. doi:10.1083/jcb.132.3.259
Cordero, A., Kanojia, D., Miska, J., Panek, W. K., Xiao, A., Han, Y., et al. (2019). FABP7 Is a Key Metabolic Regulator in HER2+ Breast Cancer Brain Metastasis. Oncogene 38 (37), 6445–6460. doi:10.1038/s41388-019-0893-4
Courbebaisse, M., and Lanske, B. (2018). Biology of Fibroblast Growth Factor 23: from Physiology to Pathology. Cold Spring Harb. Perspect. Med. 8 (5), a031260. doi:10.1101/cshperspect.a031260
De Mazière, A. M., Muehlethaler, K., Van Donselaar, E., Salvi, S., Davoust, J., Cerottini, J.-C., et al. (2002). The Melanocytic Protein Melan-A/mart-1 Has a Subcellular Localization Distinct from Typical Melanosomal Proteins. Traffic 3 (9), 678–693. doi:10.1034/j.1600-0854.2002.30909.x
Dempsey, J. L., and Cui, J. Y. (2017). Long Non-coding RNAs: a Novel Paradigm for Toxicology. Toxicol. Sci. 155 (1), 3–21. doi:10.1093/toxsci/kfw203
Derrien, T., Johnson, R., Bussotti, G., Tanzer, A., Djebali, S., Tilgner, H., et al. (2012). The GENCODE V7 Catalog of Human Long Noncoding RNAs: Analysis of Their Gene Structure, Evolution, and Expression. Genome Res. 22 (9), 1775–1789. doi:10.1101/gr.132159.111
D’Mello, S., Finlay, G., Baguley, B., and Askarian-Amiri, M. (2016). Signaling Pathways in Melanogenesis. Ijms 17 (7), 1144. doi:10.3390/ijms17071144
Enriqué Steinberg, J., Baeza, M., and Corva, P. (2013). Detection of Polymorphisms in the PMEL17 Gene in Beef Cattle of Argentina. Rev. Argent. Prod. Anim. 33 (1), 31–41.
Feng, D., Li, Q., Yu, H., Kong, L., and Du, S. (2018). Transcriptional Profiling of Long Non-coding RNAs in Mantle of Crassostrea gigas and Their Association with Shell Pigmentation. Sci. Rep. 8 (1), 1–10. doi:10.1038/s41598-018-19950-6
Gao, X., Liu, T., Cheng, X., Dai, A., Liu, W., Li, R., et al. (2020). A Novel GPR143 Mutation in a Chinese Family with X-linked O-cular A-lbinism T-ype 1. Mol. Med. Rep. 21 (1), 240–248. doi:10.3892/mmr.2019.10813
Gibb, E. A., Brown, C. J., and Lam, W. L. (2011). The Functional Role of Long Non-coding RNA in Human Carcinomas. Mol. Cancer 10 (1), 38–17. doi:10.1186/1476-4598-10-38
Gil, N., and Ulitsky, I. (2020). Regulation of Gene Expression by Cis-Acting Long Non-coding RNAs. Nat. Rev. Genet. 21 (2), 102–117. doi:10.1038/s41576-019-0184-5
Goddard, M. E., Kemper, K. E., MacLeod, I. M., Chamberlain, A. J., and Hayes, B. J. (2016). Genetics of Complex Traits: Prediction of Phenotype, Identification of Causal Polymorphisms and Genetic Architecture. Proc. R. Soc. B 283 (1835), 20160569. doi:10.1098/rspb.2016.0569
Grichnik, J. M. (2006). Kit and Melanocyte Migration. J. Investigative Dermatology 126 (5), 945–947. doi:10.1038/sj.jid.5700164
Gupta, P., Peter, S., Jung, M., Lewin, A., Hemmrich-Stanisak, G., Franke, A., et al. (2019). Analysis of Long Non-coding RNA and mRNA Expression in Bovine Macrophages Brings up Novel Aspects of Mycobacterium avium Subspecies Paratuberculosis Infections. Sci. Rep. 9 (1), 1–14. doi:10.1038/s41598-018-38141-x
Gupta, R. A., Shah, N., Wang, K. C., Kim, J., Horlings, H. M., Wong, D. J., et al. (2010). Long Non-coding RNA HOTAIR Reprograms Chromatin State to Promote Cancer Metastasis. Nature 464 (7291), 1071–1076. doi:10.1038/nature08975
Han, S., Liang, Y., Li, Y., and Du, W. (2016). Long Noncoding RNA Identification: Comparing Machine Learning Based Tools for Long Noncoding Transcripts Discrimination. BioMed Res. Int. 2016, 1–14. doi:10.1155/2016/8496165
Harrow, J., Frankish, A., Gonzalez, J. M., Tapanari, E., Diekhans, M., Kokocinski, F., et al. (2012). GENCODE: the Reference Human Genome Annotation for the ENCODE Project. Genome Res. 22 (9), 1760–1774. doi:10.1101/gr.135350.111
Heeney, J. L., and Valli, V. E. (1985). Bovine Ocular Squamous Cell Carcinoma: an Epidemiological Perspective. Can. J. Comp. Med. 49 (1), 21–26.
Hoashi, T., Watabe, H., Muller, J., Yamaguchi, Y., Vieira, W. D., and Hearing, V. J. (2005). MART-1 Is Required for the Function of the Melanosomal Matrix Protein PMEL17/GP100 and the Maturation of Melanosomes. J. Biol. Chem. 280 (14), 14006–14016. doi:10.1074/jbc.M413692200
Huang, W., Long, N., and Khatib, H. (2012). Genome-wide Identification and Initial Characterization of Bovine Long Non-coding RNAs from EST Data. Anim. Genet. 43 (6), 674–682. doi:10.1111/j.1365-2052.2012.02325.x
Ito, N., Wijenayaka, A. R., Prideaux, M., Kogawa, M., Ormsby, R. T., Evdokiou, A., et al. (2015). Regulation of FGF23 Expression in IDG-SW3 Osteocytes and Human Bone by Pro-inflammatory Stimuli. Mol. Cell. Endocrinol. 399, 208–218. doi:10.1016/j.mce.2014.10.007
Jara, E., Peñagaricano, F., Armstrong, E., Ciappesoni, G., Iriarte, A., and Navajas, E. A. (2022). Revealing the Genetic Basis of Eyelid Pigmentation in Hereford Cattle. J. Anim. Sci., skac110 [In press]. doi:10.1093/jas/skac110
Jara, E., Peñagaricano, F., Menezes, C., Tardiz, L., Rodons, G., Iriarte, A., et al. (2020). Transcriptomic Analysis of Eyelid Pigmentation in Hereford Cattle. Anim. Genet. 51 (6), 935–939. doi:10.1111/age.13004
Kadakkuzha, B. M., Liu, X.-A., McCrate, J., Shankar, G., Rizzo, V., Afinogenova, A., et al. (2015). Transcriptome Analyses of Adult Mouse Brain Reveal Enrichment of lncRNAs in Specific Brain Regions and Neuronal Populations. Front. Cell. Neurosci. 9, 63. doi:10.3389/fncel.2015.00063
Kang, Y.-J., Yang, D.-C., Kong, L., Hou, M., Meng, Y.-Q., Wei, L., et al. (2017). CPC2: a Fast and Accurate Coding Potential Calculator Based on Sequence Intrinsic Features. Nucleic Acids Res. 45 (W1), W12–W16. doi:10.1093/nar/gkx428
Kerje, S., Sharma, P., Gunnarsson, U., Kim, H., Bagchi, S., Fredriksson, R., et al. (2004). The Dominant White, Dun and Smoky Color Variants in Chicken Are Associated with Insertion/Deletion Polymorphisms in the PMEL17 GeneSequence Data from This Article Have Been Deposited with the EMBL/GenBank Data Libraries under Accession Nos. AY636124, AY636125, AY636126, AY636127, AY636128, AY636129. Genetics 168 (3), 1507–1518. doi:10.1534/genetics.104.027995
Kern, C., Wang, Y., Chitwood, J., Korf, I., Delany, M., Cheng, H., et al. (2018). Genome-wide Identification of Tissue-specific Long Non-coding RNA in Three Farm Animal Species. BMC Genomics 19 (1), 1–14. doi:10.1186/s12864-018-5037-7
Kim, D., Langmead, B., and Salzberg, S. L. (2015). HISAT: a Fast Spliced Aligner with Low Memory Requirements. Nat. Methods 12 (4), 357–360. doi:10.1038/nmeth.3317
Kornienko, A. E., Guenzl, P. M., Barlow, D. P., and Pauler, F. M. (2013). Gene Regulation by the Act of Long Non-coding RNA Transcription. BMC Biol. 11 (1), 1–14. doi:10.1186/1741-7007-11-59
Kosinska-Selbi, B., Mielczarek, M., and Szyda, J. (2020). Review: Long Non-coding RNA in Livestock. animal 14 (10), 2003–2013. doi:10.1017/S1751731120000841
Koufariotis, L. T., Chen, Y.-P. P., Chamberlain, A., Vander Jagt, C., and Hayes, B. J. (2015). A Catalogue of Novel Bovine Long Noncoding RNA across 18 Tissues. PloS one 10 (10), e0141225. doi:10.1371/journal.pone.0141225
Kubic, J. D., Young, K. P., Plummer, R. S., Ludvik, A. E., and Lang, D. (2008). Pigmentation PAX-Ways: the Role of Pax3 in Melanogenesis, Melanocyte Stem Cell Maintenance, and Disease. Pigment Cell & melanoma Res. 21 (6), 627–645. doi:10.1111/j.1755-148X.2008.00514.x
Kwon, B. S., Halaban, R., Ponnazhagan, S., Kim, K., Chintamaneni, C., Bennett, D., et al. (1995). Mousesilver.mutation Is Caused by a Single Base Insertion in the Putative Cytoplasmic Domain of Pmel 17. Nucl. Acids Res. 23 (1), 154–158. doi:10.1093/nar/23.1.154
Lalueza-Fox, C., Römpler, H., Caramelli, D., Stäubert, C., Catalano, G., Hughes, D., et al. (2007). A Melanocortin 1 Receptor Allele Suggests Varying Pigmentation Among Neanderthals. Science 318 (5855), 1453–1455. doi:10.1126/science.1147417
Le Pape, E., Wakamatsu, K., Ito, S., Wolber, R., and Hearing, V. J. (2008). Regulation of Eumelaninpheomelanin Synthesis and Visible Pigmentation in Melanocytes by Ligands of the Melanocortin 1 Receptor. Pigment Cell & melanoma Res. 21 (4), 477–486. doi:10.1111/j.1755-148X.2008.00479.x
Lee, H. J., Chen, Z., Collard, M., Chen, J. G., Wu, M., Alani, R. M., et al. (2020). FADS2-mediated Fatty Acid Desaturation and Cholesterol Esterification Are Signatures of Metabolic Reprogramming during Melanoma Progression. bioRxiv [Epub ahead of print]. doi:10.1101/2020.07.12.198903
Li, A., Zhang, J., and Zhou, Z. (2014). PLEK: a Tool for Predicting Long Non-coding RNAs and Messenger RNAs Based on an Improved K-Mer Scheme. BMC Bioinforma. 15 (1), 1–10. doi:10.1186/1471-2105-15-311
Li, A., Zhang, J., Zhou, Z., Wang, L., Liu, Y., and Liu, Y. (2015a). ALDB: a Domestic-Animal Long Noncoding RNA Database. PloS one 10 (4), e0124003. doi:10.1371/journal.pone.0124003
Li, J., Ma, W., Zeng, P., Wang, J., Geng, B., Yang, J., et al. (2015b). LncTar: a Tool for Predicting the RNA Targets of Long Noncoding RNAs. Brief. Bioinform 16 (5), 806–812. doi:10.1093/bib/bbu048
Li, Z., Zhao, W., Wang, M., and Zhou, X. (2019). The Role of Long Noncoding RNAs in Gene Expression Regulation. Gene Expr. Profiling Cancer 1, 17. doi:10.5772/intechopen.81773
Lin, J. Y., and Fisher, D. E. (2007). Melanocyte Biology and Skin Pigmentation. Nature 445 (7130), 843–850. doi:10.1038/nature05660
Ling, Y., Xu, L., Zhu, L., Sui, M., Zheng, Q., Li, W., et al. (2017). Identification and Analysis of Differentially Expressed Long Non-coding RNAs between Multiparous and Uniparous Goat (Capra hircus) Ovaries. PloS one 12 (9), e0183163. doi:10.1371/journal.pone.0183163
Long, Y., Wang, X., Youmans, D. T., and Cech, T. R. (2017). How Do lncRNAs Regulate Transcription? Sci. Adv. 3 (9), eaao2110. doi:10.1126/sciadv.aao2110
Lorenz, R., Bernhart, S. H., Höner zu Siederdissen, C., Tafer, H., Flamm, C., Stadler, P. F., et al. (2011). ViennaRNA Package 2.0. Algorithms Mol. Biol. 6 (1), 1–14. doi:10.1186/1748-7188-6-26
Love, M., Anders, S., and Huber, W. (2014). Differential Analysis of Count Data–The DESeq2 Package. Genome Biol. 15 (550), 10–1186. doi:10.1186/s13059-014-0550-8
Ma, L., Bajic, V. B., and Zhang, Z. (2013). On the Classification of Long Non-coding RNAs. RNA Biol. 10 (6), 924–933. doi:10.4161/rna.24604
McKay, B. S. (2019). Pigmentation and Vision: Is GPR143 in Control? J. Neuro Res. 97 (1), 77–87. doi:10.1002/jnr.24246
Mort, R. L., Jackson, I. J., and Patton, E. E. (2015). The Melanocyte Lineage in Development and Disease. Development 142 (4), 620–632. doi:10.1242/dev.106567
Müller, G., Höpken, U. E., Stein, H., and Lipp, M. (2002). Systemic Immunoregulatory and Pathogenic Functions of Homeostatic Chemokine Receptors. J. Leukoc. Biol. 72 (1), 1–8. doi:10.1189/jlb.72.1.1
Nguyen, A. H., Lim, V. M., Fleegel, J. P., Hunter, W. J., and Agrawal, D. K. (2016). Cutaneous Expression of TREM, Vitamin D Receptor and HMGB1 in Vitamin D Deficiency. Int. J. Clin. Exp. Pathol. 9 (8), 8506–8512.
Nguyen, A. H., Koenck, C., Quirk, S. K., Lim, V. M., Mitkov, M. V., Trowbridge, R. M., et al. (2015). Triggering Receptor Expressed on Myeloid Cells in Cutaneous Melanoma. Clin. Transl. Sci. 8 (5), 441–444. doi:10.1111/cts.12308
Niazi, F., and Valadkhan, S. (2012). Computational Analysis of Functional Long Noncoding RNAs Reveals Lack of Peptide-Coding Capacity and Parallels with 3′ UTRs. RNA 18 (4), 825–843. doi:10.1261/rna.029520.111
Pang, K. C., Frith, M. C., and Mattick, J. S. (2006). Rapid Evolution of Noncoding RNAs: Lack of Conservation Does Not Mean Lack of Function. Trends Genet. 22 (1), 1–5. doi:10.1016/j.tig.2005.10.003
Pausch, H., Wang, X., Jung, S., Krogmeier, D., Edel, C., Emmerling, R., et al. (2012). Identification of QTL for UV-Protective Eye Area Pigmentation in Cattle by Progeny Phenotyping and Genome-wide Association Analysis. PloS one 7 (5), e36346. doi:10.1371/journal.pone.0036346
Pickard, A., and McCance, D. J. (2015). IGF-binding Protein 2 €" Oncogene or Tumor Suppressor? Front. Endocrinol. 6, 25. doi:10.3389/fendo.2015.00025
Qureshi, I. A., Mattick, J. S., and Mehler, M. F. (2010). Long Non-coding RNAs in Nervous System Function and Disease. Brain Res. 1338, 20–35. doi:10.1016/j.brainres.2010.03.110
Ren, H., Wang, G., Chen, L., Jiang, J., Liu, L., Li, N., et al. (2016). Genome-wide Analysis of Long Non-coding RNAs at Early Stage of Skin Pigmentation in Goats (Capra hircus). BMC Genomics 17 (1), 1–12. doi:10.1186/s12864-016-2365-3
Schmutz, S. M., and Dreger, D. L. (2013). Interaction ofMC1RandPMELalleles on Solid Coat Colors in Highland Cattle. Anim. Genet. 44 (1), 9–13. doi:10.1111/j.1365-2052.2012.02361.x
Sharif, O., and Knapp, S. (2008). From Expression to Signaling: Roles of TREM-1 and TREM-2 in Innate Immunity and Bacterial Infection. Immunobiology 213 (9-10), 701–713. doi:10.1016/j.imbio.2008.07.008
Solano, F. (2014). Melanins: Skin Pigments and Much More—Types, Structural Models, Biological Functions, and Formation Routes. New J. Sci. 2014, 498276. doi:10.1155/2014/498276
St. Laurent, G., Vyatkin, Y., and Kapranov, P. (2014). Dark Matter RNA Illuminates the Puzzle of Genome-wide Association Studies. BMC Med. 12 (1), 1–8. doi:10.1186/1741-7015-12-97
Tang, L., Liang, Y., Xie, H., Yang, X., and Zheng, G. (2020). Long Non‐coding RNAs in Cutaneous Biology and Proliferative Skin Diseases: Advances and Perspectives. Cell Prolif. 53 (1), e12698. doi:10.1111/cpr.12698
Tang, X.-H., and Gudas, L. J. (2011). Retinoids, Retinoic Acid Receptors, and Cancer. Annu. Rev. Pathol. Mech. Dis. 6, 345–364. doi:10.1146/annurev-pathol-011110-130303
Trapnell, C., Williams, B. A., Pertea, G., Mortazavi, A., Kwan, G., Van Baren, M. J., et al. (2010). Transcript Assembly and Quantification by RNA-Seq Reveals Unannotated Transcripts and Isoform Switching during Cell Differentiation. Nat. Biotechnol. 28 (5), 511–515. doi:10.1038/nbt.1621
Wan, D. C., and Wang, K. C. (2014). Long Noncoding RNA: Significance and Potential in Skin Biology. Cold Spring Harb. Perspect. Med. 4 (5), a015404. doi:10.1101/cshperspect.a015404
Wang, J., Koganti, P. P., and Yao, J. (2020). Systematic Identification of Long Intergenic Non-coding RNAs Expressed in Bovine Oocytes. Reprod. Biol. Endocrinol. 18 (1), 1–9. doi:10.1186/s12958-020-00573-4
Wang, L., Park, H. J., Dasari, S., Wang, S., Kocher, J.-P., and Li, W. (2013). CPAT: Coding-Potential Assessment Tool Using an Alignment-free Logistic Regression Model. Nucleic Acids Res. 41 (6), e74. doi:10.1093/nar/gkt006
Watt, B., van Niel, G., Raposo, G., and Marks, M. S. (2013). PMEL: a Pigment Cell-specific Model for Functional Amyloid Formation. Pigment. Cell Melanoma Res. 26 (3), 300–315. doi:10.1111/pcmr.12067
Weikard, R., Hadlich, F., and Kuehn, C. (2013). Identification of Novel Transcripts and Noncoding RNAs in Bovine Skin by Deep Next Generation Sequencing. BMC Genomics 14 (1), 789–815. doi:10.1186/1471-2164-14-789
Yamada, T., Hasegawa, S., Inoue, Y., Date, Y., Yamamoto, N., Mizutani, H., et al. (2013). Wnt/β-Catenin and Kit Signaling Sequentially Regulate Melanocyte Stem Cell Differentiation in UVB-Induced Epidermal Pigmentation. J. Investigative Dermatology 133 (12), 2753–2762. doi:10.1038/jid.2013.235
You, Z., Zhang, Q., Liu, C., Song, J., Yang, N., and Lian, L. (2019). Integrated Analysis of lncRNA and mRNA Repertoires in Marek's Disease Infected Spleens Identifies Genes Relevant to Resistance. BMC Genomics 20 (1), 1–15. doi:10.1186/s12864-019-5625-1
Yue, Y., Guo, T., Yuan, C., Liu, J., Guo, J., Feng, R., et al. (2016). Integrated Analysis of the Roles of Long Noncoding RNA and Coding RNA Expression in Sheep (Ovis aries) Skin during Initiation of Secondary Hair Follicle. PLoS One 11 (6), e0156890. doi:10.1371/journal.pone.0156890
Zhang, B. H., Pan, X. P., Cox, S. B., Cobb, G. P., and Anderson, T. A. (2006). Evidence that miRNAs Are Different from Other RNAs. Cell. Mol. Life Sci. 63 (2), 246–254. doi:10.1007/s00018-005-5467-7
Zhao, W., Mazar, J., Lee, B., Sawada, J., Li, J.-L., Shelley, J., et al. (2016a). The Long Noncoding RNA SPRIGHTLY Regulates Cell Proliferation in Primary Human Melanocytes. J. Investigative Dermatology 136 (4), 819–828. doi:10.1016/j.jid.2016.01.018
Zhao, Y., Li, H., Fang, S., Kang, Y., Wu, W., Hao, Y., et al. (2016b). NONCODE 2016: an Informative and Valuable Data Source of Long Non-coding RNAs. Nucleic Acids Res. 44 (D1), D203–D208. doi:10.1093/nar/gkv1252
Zou, C., Li, L., Cheng, X., Li, C., Fu, Y., Fang, C., et al. (2018). Identification and Functional Analysis of Long Intergenic Non-coding RNAs Underlying Intramuscular Fat Content in Pigs. Front. Genet. 9, 102. doi:10.3389/fgene.2018.00102
Keywords: eye cancer, lncRNAs, noncoding genetic elements, strand-specific RNAseq, beef cattle
Citation: Jara E, Peñagaricano F, Armstrong E, Menezes C, Tardiz L, Rodons G and Iriarte A (2022) Identification of Long Noncoding RNAs Involved in Eyelid Pigmentation of Hereford Cattle. Front. Genet. 13:864567. doi: 10.3389/fgene.2022.864567
Received: 28 January 2022; Accepted: 20 April 2022;
Published: 04 May 2022.
Edited by:
Tad Stewart Sonstegard, Acceligen, United StatesReviewed by:
Xianyong Lan, Northwest A&F University, ChinaAroa Suarez Vega, Universidad de León, Spain
Copyright © 2022 Jara, Peñagaricano, Armstrong, Menezes, Tardiz, Rodons and Iriarte. This is an open-access article distributed under the terms of the Creative Commons Attribution License (CC BY). The use, distribution or reproduction in other forums is permitted, provided the original author(s) and the copyright owner(s) are credited and that the original publication in this journal is cited, in accordance with accepted academic practice. No use, distribution or reproduction is permitted which does not comply with these terms.
*Correspondence: Andrés Iriarte, airiarte@higiene.edu.uy