- 1School of Food and Bioengineering, Xuzhou University of Technology, Jiangsu, Xuzhou, China
- 2School of Biology and Food Engineering, Changshu Institute of Technology, Jiangsu, Suzhou, China
- 3The State Key Laboratory of Pharmaceutical Biotechnology, Medical School, School of Life Sciences, Nanjing University, Nanjing, Jiangsu, China
- 4School of Food Science and Technology, Jiangnan University, Wuxi, Jiangsu, China
- 5Wuxi School of Medicine, Jiangnan University, Wuxi, Jiangsu, China
Background: Achaete-scute homolog 1 transcription factors were important in the differentiation of neuronal-like glioblastoma (GBM) cancer stem cells (CSCs). To gain a better understanding of the role of ASCL1 in GBM, chromatin immunoprecipitation followed by high-throughput sequencing (ChIP-seq) data can be analyzed to construct their gene transcription regulation network.
Methods: GSE87618 was downloaded from the Gene Expression Omnibus, which is a famous database, in the field of biology. The filtered clean reads were mapped to the human genome utilizing the software of bowtie2. Then, differential peak analysis was performed by diffbind. Finally, the annotated gene functions and signaling pathways were investigated by Gene ontology function and kyoto encyclopedia of genes genomes (KEGG) pathway enrichment analysis. Moreover, the protein–protein interaction network (PPI) analysis of genes obtained from ASCL1 was carried out to explore the hub genes influenced by ASCL1.
Results: A total of 516 differential peaks were selected. GO analysis of functions revealed that promoter, untranslated region (UTR), exon, intron, and intergenic genes were mainly enriched in biological pathways such as keratinization, regulation of cAMP metabolic process, blood coagulation, fibrin clot formation, midgut development, and synapse assembly. Genes were mainly enriched in KEGG pathways including pentose phosphate pathway, glycosphingolipid biosynthesis—globo and isoglobo series, ECM–receptor interaction, and adherens junction. In total, 244 nodes and 475 interaction pairs were included in the PPI network with the hub genes including EGFR, CTNNB1, and SPTAN1.
Conclusion: EGFR, SPTAN1, and CTNN1B might be the potential down-stream genes of ASCL1 in GBM development, and CTNN1B might make contributions to GBM progression on regulating the cAMP pathway.
Introduction
Glioblastoma (GBM), as the most common primary malignant brain tumor in adults, is one of the most aggressive and lethal human tumors characterized by a block in cellular differentiation. The median survival can range from 12 to 15 months among patients undergoing the current standard of care treatment involving surgery, chemotherapy, and radiation therapy (Ji et al., 2015; Shabihkhani et al., 2017; Xu et al., 2017; Mortazavi, 2018; Jin et al., 2019). For GBM patients, the disease is hardly diagnosed in the early stage. Meanwhile, recent therapeutic options are limited and prognosis is poor (Berninger et al., 2007). Due to the extremely high malignant grade of GBM, surgical resection combined with radiotherapy and chemotherapy has not changed its malignant progression trend, which is a serious threat to human health. Thus, novel treatment paradigms are urgently needed to improve outcomes. Currently, many efforts are focused on the target therapies, such as traditional small molecule inhibitors, monoclonal antibodies, and immunotherapeutic approaches (Park et al., 2017). These treatment strategies are actively examined in clinical trials and offer an attractive alternation (Shao et al., 2013; Narayanan et al., 2018; Bao et al., 2020). At present, various bioinformatics methods have sprung up, and a large amount of tumor gene expression profile data have become the research direction of tumor precision therapy. Therefore, the study of the glioma gene expression network also has an important theoretical value and practical significance, and its clinical application prospect should not be rested.
ASCL1 is a gene classifier for the pro-neural (PN) transcriptional subgroup of GBM, which plays as a relevant role in the neuronal-like differentiation of glioblastoma stem cells (GSCs). It has been noted that cell-cycle exit and full neuronal specification and differentiation could be induced by ASCL1 over-expression in neural precursor cells (Barrett et al., 2013). Park et al. demonstrated that the transcription factor ASCL1 was required for GSCs to undergo neuronal lineage differentiation, and GSCs with high ASCL1 expression levels were responsive to notch pathway inhibitors and important in driving neuronal fate (Kent et al., 2002; Langmead and Salzberg, 2012; Bolger et al., 2014). Furthermore, Narayanan proposed that ASCL1 might be served as potential subgroup-specific targetable vulnerability in GBM through targeting NDRG1 (Zhang et al., 2008; Stark, 2011; Bao et al., 2018; Ji et al., 2019; Bao et al., 2021). These efforts suggest that ASCL1 plays important roles in neuronal specification. However, the molecular network associated with the roles of ASCL1 in GBM has not yet been researched.
In order to clarify the regulatory mechanisms of the ASCL1 in GBM, the data of ChIP-seq were analyzed by utilizing bioinformatics method. The annotated gene functions and signaling pathways were investigated by Gene ontology (GO) function and kyoto encyclopedia of genes genomes (KEGG) pathway enrichment analysis. Moreover, the protein–protein interaction network (PPI) analysis of genes was constructed to explore the hub genes influenced by ASCL1.
Materials and methods
Data sources
GSE87618 was the genome occupancy profiling of differential ASCL1 binding between control and GSC cultures induced to overexpress ASCL1 after 18 h of doxycycline treatment, which were downloaded from the database of Gene Expression Omnibus (GEO, http://www.ncbi.nlm.nih.gov/geo/) (Robinson and Oshlack, 2010). GSE87618 contained data from eight samples, including three ASCL1 ChIP-seq negative controls (nc1, nc2, nc3), one ASCL1 ChIP-seq negative control input (nc_input), three ASCL1 ChIP-seq doxycycline (dox1, dox2, dox3, 18 h of doxycycline treatment), and one ASCL1 ChIP-seq doxycycline input (dox_input, 18 h of doxycycline treatment). Sra format profile data of GSE87618 were downloaded, and the microarray data were then converted into. fastq utilizing fastq-dump (https://trace.ncbi.nlm.nih.gov/Traces/sra/sra.cgi?view=toolkit_doc&f=fastq-dump).
Quality control of sequencing data
In order to filter out the unreliable bases and reads, quality control was performed for the original offline data. Sequencing tape joints were firstly removed. Reads with consecutive masses below 20 at both ends or reads less than 36 nt in length would be removed. Clean reads were obtained by utilizing the tool of Trimmomatic (v3.6) (Yu et al., 2015; Bao et al., 2020).
Sequence alignment
The filtered clean reads were mapped to the human genome (UCSC, hg19), utilizing the software of bowtie 2 (Shannon et al., 2003; Huang et al., 2009; Yu et al., 2012; Szklarczyk et al., 2015). The non-unique mapped reads and low-quality mappings in the results were removed, utilizing default parameters.
Peak calling
Peak signal detection is a key step in the ChIP-seq analysis. MACS2 was used to find the peaks of the enrichment region of trusted sequence (the ASCL1 binding region) from the short sequence alignment results and predict the length of the predicted frag_sizes (Dablander and Hinne, 2019). The default parameters and p-value< 1e-3 were set as the screening threshold.
Differential peak analysis
Based on the obtained alignment result and the peak call result, differential peak analysis was performed, utilizing diffbind software (Wang et al., 2008) to obtain differential peak binding to the chromosome due to the expression level of ASCL1 between the ASCL1 ChIP-seq doxycycline group and negative control group. The downstream analysis was performed on an overlap peak in at least three samples, and the number of reads covered by the peak was calculated to obtain the binding affinity matrix. Then, the differential peaks were calculated by edgeR (Du et al., 2015; Tang et al., 2015). The screening thresholds were designed as false-positive rate (FDR) < 0.05 and |Fold change | > 2.
Peak annotation
The differential peaks obtained in the previous step were annotated, utilizing Chipseeker (Rheinbay et al., 2013). A 3 kb (up: 2500 bp down: 500 bp) sequence near the transcription start site (TSS) was selected as a promoter region.
Gene ontology function and kyoto encyclopedia of genes and genomes pathway enrichment analysis
Enriched GO function and KEGG pathway were analyzed on the annotated genes (Zhang et al., 2008). The genes were divided into five categories based on different positions, including promoter, UTR, exon, intron, and intergenic. KEGG pathways and GO functions were analyzed for genes on different positions, respectively, utilizing the tool of Database for Annotation, Visualization and Integrated Discovery (DAVID) based on hypergeometric test (Azzarelli et al., 2022). The significant threshold was set as p value <0.05.
Protein–protein interaction network
The database of Search Tool for Retrieval of Interacting Genes (STRING) is an online database for predicting PPIs (Wang et al., 2021). Utilizing STRING (version 10.0, http://www.string-db.org/) database, the PPIs of genes were analyzed. The protein pairs with PPI score >0.4 were collected. Then, Cytoscape (version: 3.2.0, http://www.cytoscape.org/) was used to visualize the predicted PPI network (Gorla et al., 2009; Bhinge et al., 2017; Vue et al., 2020).
Three methods were used to evaluate the centrality of the complex network, including degree centrality (Ackermann et al., 2019), betweenness centrality (Nager et al., 2018), and closeness centrality (Chen et al., 1998). CytoNCA was a cytoscape plugin for the calculation of three topology properties (parameter setting: network is without weight) (Mahesparan et al., 1997; Woods et al., 2022). In the CytoNCA output, the node score represented the role of the protein in the network.
Results
Sequencing data quality control and sequence data comparison result
Table 1 shows the quality of sequencing data. The percentages of bases in all eight samples with Phred values greater than 30 were all more than 99.8%. The results of sequencing data comparison are shown in Table 2, and the mapped rates in different samples were all more than 95%.
Peak call based on the expression level of ASCL1
In total, 4792 peaks were obtained in sample DOX_1, 4176 peaks in DOX_2, 4231 peaks in DOX_3, 1211 peaks in NC_1, 1193 peaks in NC_2, and 733 peaks in NC_3. The doxycycline treatment resulted in a higher expression of ASCL1, and then more peaks were obtained.
Differential peak analysis
The differential peak analysis was performed, and a total of 516 differential peaks were selected. The results of the principal component analysis (PCA) between multiple samples are shown in Figure 1A, and the samples in control and doxycycline treatment group were clustered separately. Differential expression peak hotspot distribution map showed that the profile of differential expression peaks was significantly different in the control and doxycycline treatment group (Figure 1B).
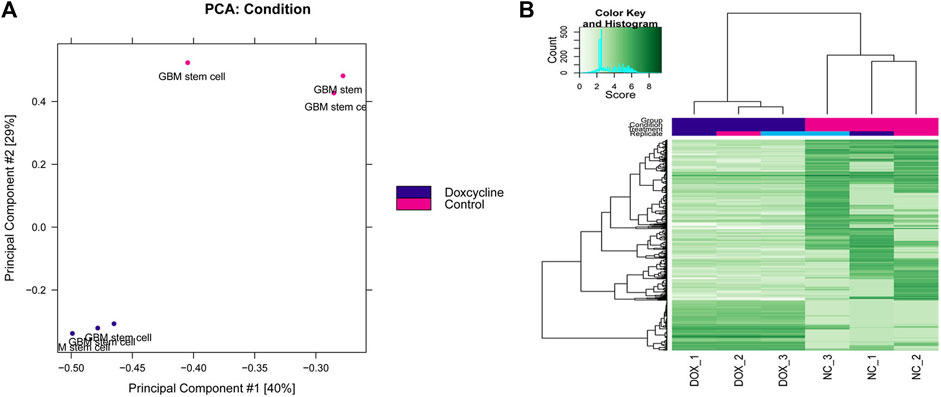
FIGURE 1. Principal component analysis (A) and two-dimensional hotspot clustering map of differential peaks (B).
Differential peak annotation
Peaks were annotated by peakseeker, and results showed that 10% peaks were located near the gene promoter, 33% of the peaks were located in the intergenic region, and 40% of the peaks were located in the intron region of the genes (Figure 2).
GO function and KEGG pathway enrichment of genes involved in differential peak annotation
According to the results of the differential peak annotation, the obtained genes were separately subjected to GO functional and KEGG pathway enrichment analysis. As shown in Figure 3A, genes are mainly enriched in biological pathways, such as “odontogenesis of dentin-containing tooth,” “embryonic digit morphogenesis,” and “negative regulation of cell development.” Figure 3B shows that genes are mainly enriched in KEGG pathways, including “starch and sucrose metabolism,” “small cell lung cancer,” and “relaxin signaling pathway.”
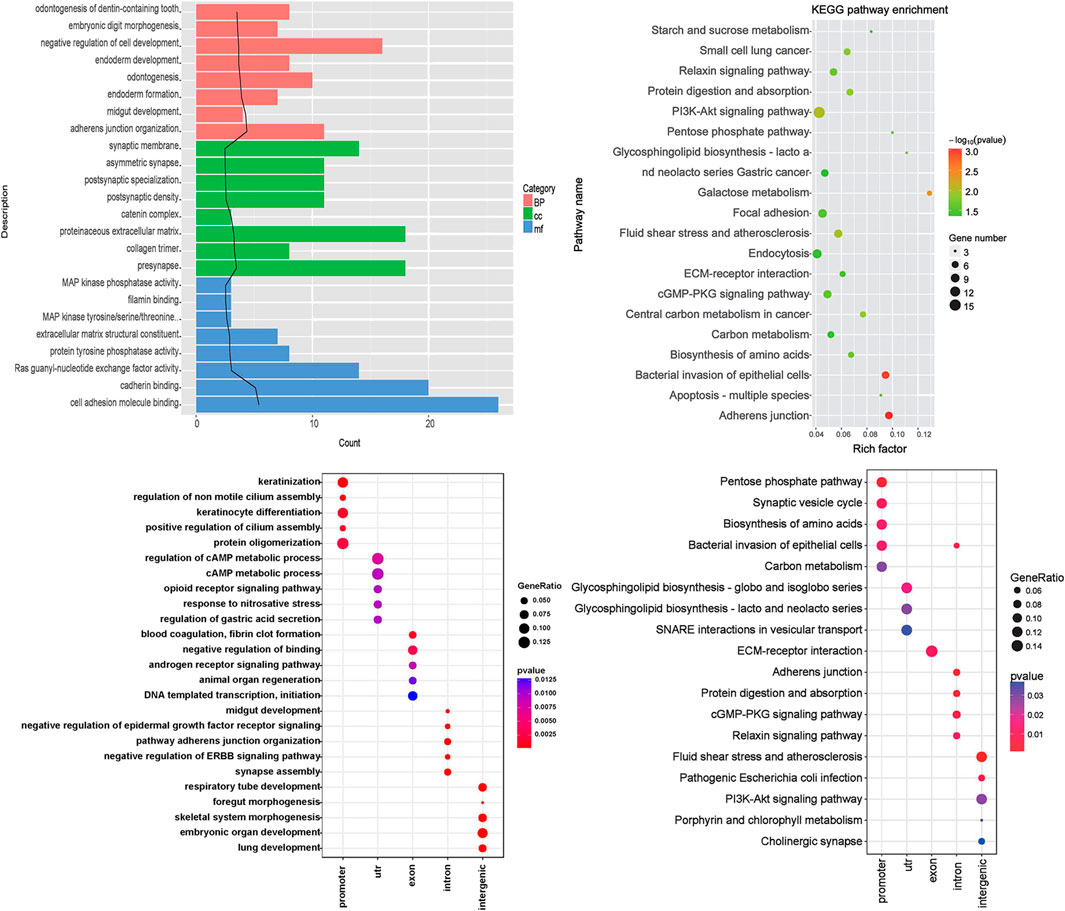
FIGURE 3. Functional enrichment analysis. (A) Gene ontology (GO) enrichment analysis. Category: the category of GO, BP: biological pathway, CC: cell composition, MF: molecular function, term: GO function description information, count: the number of differential genes enriched in the term; the black trend line represents the -log10 (p Value) value. (B) The KEGG pathway enrichment analysis results of differential genes. The rich factor refers to the ratio of the number of differentially expressed genes in the pathway to the total number of genes in the pathway. The size of the rich factor represents the degree of enrichment. (C) Enriched biological pathway of five kinds of genes; (D) KEGG pathway of five kinds of genes. The abscissa represents the group name, and the ordinate represents the enrichment entry name.
Genes were divided into five categories including promoter, UTR, exon, intron, and intergenic. Functional enrichment analysis was further performed, and the results showed that promoter genes were mainly enriched in biological pathways such as keratinization. UTR genes were enriched in the regulation of the cAMP metabolic process. Exon genes were enriched in blood coagulation and fibrin clot formation, intron genes were enriched in midgut development, and intergenic genes were enriched in synapse assembly (Figure 3C). KEGG pathway analysis showed that the significant pathways included pentose phosphate pathway (promoter genes), glycosphingolipid biosynthesis—globo and isoglobo series (UTR genes), ECM–receptor interaction (exon genes), adherens junction (intron genes), and fluid shear stress and atherosclerosis (intergenic genes) (Figure 3D).
PPI network
The PPI network for genes was constructed (Figure 4), and 244 nodes and 475 interaction pairs were included in the network. The top 10 hub nodes based on the degree centrality, betweenness centrality, and closeness centrality are shown in Table 3, such as epidermal growth factor receptor (EGFR), catenin Beta 1 (CTNNB1), and spectrin alpha, non-erythrocytic 1 (SPTAN1).
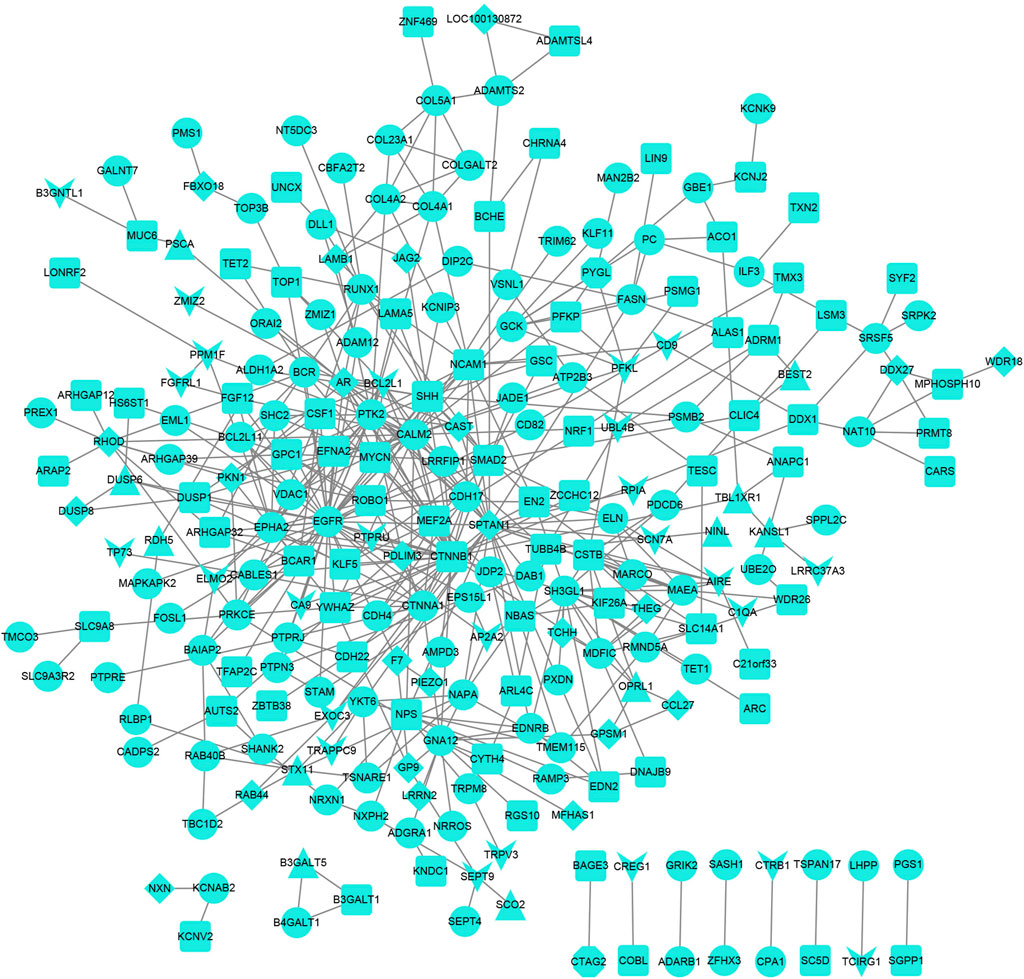
FIGURE 4. Protein–protein interaction network. The V-shaped node represents the promoter, the triangular node represents the UTR, the diamond node represents the exon, the circular node represents the intron, and the square node represents the intergenic region.
Discussion
GBMs are incurable brain tumors with a high degree of cellular heterogeneity and genetic mutations. For anti-GBM therapies, the loss of proliferation control and unregulated self-renewal would be the most important hard work for clinical treatment. In the context of gliomas, some transcription factors are often expressed and have been shown to function in determining the tumorigenicity and differentiation status of tumor cells. In this study, we focus on ASCL1, a class II basic-helix–loop–helix (bHLH) transcription factor that forms a heterodimer with class I bHLH E-proteins (such as E47/TCF3) to activate specific target genes. Studies have shown that ASCL1 regulates transcriptional targets that are central to the GBM development. The study of Park et al. showed that ASCL1 could control the neuronal fate and suppress tumorigenicity of glioblastoma stem cells by reorganizing chromatin (Park et al., 2017). The study of Rheinbay et al. showed that ASCL1 exerts functions in part by repressing an inhibitor of Wnt signaling, DKK1, resulting in increased signaling through this pathway to maintain the tumorigenicity of glioma cells (Shannon et al., 2003). ASCL1 phosphorylation and ID2 upregulation are roadblocks to glioblastoma stem cell differentiation (Wang et al., 2008; Dablander and Hinne, 2019). The study of Tou et al. showed that the loss of ASCL1 significantly reduces the proliferation of GBMs induced in the brain of a genetically relevant glioma mouse model, resulting in extended survival times, illustrating an important role for ASCL1 in controlling the proliferation of GBM (Du et al., 2015). In our study, a total of 516 differential peaks between ASCL1 overexpressed GSC samples and negative controls were screened. GO analysis of functions revealed that the genes annotated on differential peaks were mainly associated with biological pathways such as keratinization, regulation of cAMP metabolic process, blood coagulation, fibrin clot formation, midgut development, and synapse assembly. Genes were mainly enriched in KEGG pathways including pentose phosphate pathway, glycosphingolipid biosynthesis—globo and isoglobo series, ECM–receptor interaction, adherens junction, and fluid shear stress and atherosclerosis. The PPI network with 244 nodes and 475 interaction pairs was constructed including the hub genes such as EGFR, CTNNB1, and SPTAN1.
The gene expression was mediated by ASCL1 binding to chromatin. In particular, a member of the basic helix–loop–helix (BHLH) family of transcription factors was encoded by ASCL1, and the protein was important in the neuronal differentiation, olfactory, and autonomic neuron generation. In our study, a regulation network associated with ASCL1 was constructed based on ChIP-seq data. In PPI network, EGFR, CTNNB1, and SPTAN1 were hub genes. In lung adenocarcinomas patients, EGFR mediates the activation of RET with neuroendocrine differentiation characterized by ASCL1 expression, implicating that EGFR is a key regulator of RET (Tang et al., 2015). ASCL1 function is an upstream regulator of the Ret Proto-Oncogene, so combined with our findings, we speculate that in GBM, ASCL1 may mediate RET activation through EGFR, thereby affecting tumor progression. The protein encoded by SPTAN1 has been implicated in DNA repair and cell cycle regulation. Meanwhile, this gene was involved with the RET signaling pathway (Rheinbay et al., 2013). Ackermann et al. demonstrated a close relationship between low SPTAN1 expression and tumor progression and metastasis in colorectal cancers (Azzarelli et al., 2022). Although no direct evidence has shown the association between ASCL1 and the genes such as EGFR and SPTAN1 in GBM, the data revealed its potential value as an important downstream gene of ASCL1 in GBM.
It has been reported that WNT-CTNN1B signaling plays important roles in promoting cancer cell proliferation and stemness, and Nager et al. showed that silencing CTNN1B could decrease cell viability and induce GBM cell apoptosis (Wang et al., 2021). In malignant gliomas, previous evidence showed that protein kinase (PKA) activation was correlated with decreased proliferation, increased differentiation, and apoptosis induction by increasing cAMP levels or directly by cAMP analogues (Vue et al., 2020). Regulation of the cAMP metabolic process was involved in the GO enrichment function in GBM. Together with the data presented herein, there is a growing body of evidence suggesting a role of CTNN1B in GBM progression based on regulating the cAMP pathway. An uncontrolled cell proliferation and infiltrative growth within the brain were the main characteristics in malignant human gliomas. The conjunction with vascular elements has specific interactions between tumor cell surface receptors and specific ECM, which induced an extensive tumor cell movement along blood vessels (Bhinge et al., 2017). Enhancing ASCL1 activity in a neurogenic environment both increases binding at endogenous ASCL1 sites and also results in additional binding to new low affinity sites that favors neuronal differentiation (Gorla et al., 2009). Our data showed that the ECM–receptor interaction and pentose phosphate pathways were dysregulated by ASCL1 overexpression in GSCs.
Our data provide a genome-wide view of gene regulation by ASCL1 signaling in GBM, and we showed important roles of hub genes influenced by ASCL1. However, there are some limitations that should be noted. The data were all analyzed by the method of bioinformatics, and the potential functional enrichment of genes should be further researched by clinical research. Furthermore, only three ASCL1 ChIP-seq negative controls and three ASCL1 ChIP-seq doxycyclines were enrolled in the analysis. It should not be denied that the background of GBM patients varied from each other. Thus, the conclusion should be verified by further systematical analysis.
In summary, we constructed a regulation network for the ASCL1 role involved in neurogenic gene expression program activation in GBM. Our data revealed that EGFR and SPTAN1 were the potential downstream genes of ASCL1 in the GBM development, and CTNN1B might take part in GBM progression based on regulating the cAMP pathway. However, the conclusion should be further verified by experimental data.
Data availability statement
The original contributions presented in the study are included in the article/supplementary material; further inquiries can be directed to the corresponding author.
Author contributions
NZ and JZ designed the experiments of this work. ZL collected the data and dealt with the figures and tables in this work. NZ and TL edited the article.
Funding
This work was supported by the Major Basic Research Project of the Natural Science Foundation of the Jiangsu Higher Education Institutions (Nos. 21KJB360022, 20KJB330002), the Xuzhou Key Research and Development Project (No. KC20147), the General Program of China Postdoctoral Science Foundation (2022M711369), and the Suzhou Science and Technology Council (SNG201907).
Conflict of interest
The authors declare that the research was conducted in the absence of any commercial or financial relationships that could be construed as a potential conflict of interest.
Publisher’s note
All claims expressed in this article are solely those of the authors and do not necessarily represent those of their affiliated organizations, or those of the publisher, the editors, and the reviewers. Any product that may be evaluated in this article, or claim that may be made by its manufacturer, is not guaranteed or endorsed by the publisher.
Abbreviations
ASCL1, achaete-scute homolog 1; BHLH, basic helix–loop–helix; CTNNB1, catenin beta 1; CCHS, congenital central hypoventilation syndrome; CSCs, cancer stem cells; GBMs, glioblastomas; GO, gene ontology; KEGG, kyoto encyclopedia of genes genomes; PPI, protein–protein interaction network; PN, proneural; GSCs, glioblastoma stem cells; FDR, false-positive rate; PCA, principal component analysis; EGFR, epidermal growth factor receptor; SPTAN1, spectrin alpha, non-erythrocytic 1.
References
Ackermann, A., Schrecker, C., Bon, D., Friedrichs, N., Bankov, K., Wild, P., et al. (2019). Downregulation of SPTAN1 is related to MLH1 deficiency and metastasis in colorectal cancer. PLoS One 14 (3), e0213411. doi:10.1371/journal.pone.0213411
Azzarelli, R., McNally, A., Dell'Amico, C., Onorati, M., Simons, B., and Philpott, A. (2022). ASCL1 phosphorylation and ID2 upregulation are roadblocks to glioblastoma stem cell differentiation. Sci. Rep. 12, 2341. doi:10.1038/s41598-022-06248-x
Bao, W., Yang, B., Li, Z., and Zhou, Y. (2018). Laipt: Lysine acetylation site identification with polynomial tree. Int. J. Mol. Sci. 201, 113. doi:10.3390/ijms20010113
Bao, W, Huang, D. S., and Chen, Y. H. (2020). MSIT: malonylation sites identification tree. Curr. Bioinforma. 15, 59–67. doi:10.2174/1574893614666190730110747
Bao, W., Yang, B., and Chen, B. (2021). 2-hydr_ensemble: Lysine 2-hydroxyisobutyrylation identification with ensemble method. Chemom. Intell. Lab. Syst. 215, 104351. doi:10.1016/j.chemolab.2021.104351
Barrett, T., Wilhite, S. E., Ledoux, P., Evangelista, C., Kim, I. F., Tomashevsky, M., et al. (2013). NCBI GEO: archive for functional genomics data sets--update. Nucleic Acids Res. 41, D991–D995. doi:10.1093/nar/gks1193
Berninger, B., Guillemot, F., and Gotz, M. (2007). Directing neurotransmitter identity of neurones derived from expanded adult neural stem cells. Eur. J. Neurosci. 25 (9), 2581–2590. doi:10.1111/j.1460-9568.2007.05509.x
Bhinge, K., Yang, L., Terra, S., Nasir, A., Muppa, P., Aubry, M. C., et al. (2017). EGFR mediates activation of RET in lung adenocarcinoma with neuroendocrine differentiation characterized by ASCL1 expression. Oncotarget 8 (16), 27155–27165. doi:10.18632/oncotarget.15676
Bolger, A. M., Lohse, M., and Usadel, B. (2014). Trimmomatic: a flexible trimmer for illumina sequence data. Bioinformatics 30 (15), 2114–2120. doi:10.1093/bioinformatics/btu170
Chen, T. C., Hinton, D. R., Zidovetzki, R., and Hofman, F. M. (1998). Up-regulation of the cAMP/PKA pathway inhibits proliferation, induces differentiation, and leads to apoptosis in malignant gliomas. Lab. Invest. 78 (2), 165–174.
Dablander, F., and Hinne, M. (2019). Node centrality measures are a poor substitute for causal inference. Sci. Rep. 9 (1), 6846. doi:10.1038/s41598-019-43033-9
Du, Y., Gao, C., Chen, X., Hu, Y., Sadiq, R., Deng, Y., et al. (2015). A new closeness centrality measure via effective distance in complex networks. Chaos 25 (3), 033112. doi:10.1063/1.4916215
Gorla, L., Mondellini, P., Cuccuru, G., MiccicheF., , Cassinelli, G., CreMonaM., , et al. (2009). Proteomics study of medullary thyroid carcinomas expressing RET germ-line mutations: Identification of new signaling elements. Mol. Carcinog. 48 (3), 220–231. doi:10.1002/mc.20474
Huang, da W., Sherman, B. T., and Lempicki, R. A. (2009). Systematic and integrative analysis of large gene lists using DAVID bioinformatics resources. Nat. Protoc. 4 (1), 44–57. doi:10.1038/nprot.2008.211
Ji, Z., Wu, D., Zhao, W., Peng, H., Zhao, S., Huang, D., et al. (2015). Systemic modeling myeloma-osteoclast interactions under normoxic/hypoxic condition using a novel computational approach. Sci. Rep. 5, 13291. doi:10.1038/srep13291
Ji, Z., Zhao, W., Lin, H. K., and Zhou, X. (2019). Systematically understanding the immunity leading to CRPC progression. PLoS Comput. Biol. 159, e1007344. doi:10.1371/journal.pcbi.1007344
Jin, M. C., Wu, A., Xiang, M., Azad, T. D., Soltys, S. G., Li, G., et al. (2019). Prognostic factors and treatment patterns in the management of giant cell glioblastoma. World Neurosurg. 128, e217–e224. doi:10.1016/j.wneu.2019.04.103
Kent, W. J., Sugnet, C. W., Furey, T. S., Roskin, K. M., Pringle, T. H., Zahler, A. M., et al. (2002). The human genome browser at UCSC. Genome Res. 12 (6), 996–1006. doi:10.1101/gr.229102
Langmead, B., and Salzberg, S. L. (2012). Fast gapped-read alignment with Bowtie 2. Nat. Methods 9 (4), 357–359. doi:10.1038/nmeth.1923
Mahesparan, R., Tysnes, B. B., Edvardsen, K., Haugeland, H. K., Cabrera, I. G., Lund-JohansenM., , et al. (1997). Role of high molecular weight extracellular matrix proteins in glioma cell migration. Neuropathol. Appl. Neurobiol. 23 (2), 102–112. doi:10.1111/j.1365-2990.1997.tb01192.x
Mortazavi, S. M. J. (2018). Commentary: geographic variations in the incidence of glioblastoma and prognostic factors predictive of overall survival in US adults from 2004-2013. Front. Aging Neurosci. 10, 105. doi:10.3389/fnagi.2018.00105
Nager, M., Sallan, M. C., Visa, A., Pushparaj, C., Santacana, M., Macia, A., et al. (2018). Inhibition of WNT-CTNNB1 signaling upregulates SQSTM1 and sensitizes glioblastoma cells to autophagy blockers. Autophagy 14 (4), 619–636. doi:10.1080/15548627.2017.1423439
Narayanan, A., Gagliardi, F., Gallotti, A. L., Mazzoleni, S., Cominelli, M., Fagnocchi, L., et al. (2018). The proneural gene ASCL1 governs the transcriptional subgroup affiliation in glioblastoma stem cells by directly repressing the mesenchymal gene NDRG1. Cell Death Differ. 26, 1813–1831. doi:10.1038/s41418-018-0248-7
Park, N. I., Guilhamon, P., Desai, K., McAdam, R. F., Langille, E., O'Connor, M., et al. (2017). ASCL1 reorganizes chromatin to direct neuronal fate and suppress tumorigenicity of glioblastoma stem cells. Cell Stem Cell 21 (3), 411. doi:10.1016/j.stem.2017.08.008
Rheinbay, E., Suvà, M. L., Gillespie, S. M., Wakimoto, H., Patel, A. P., Shahid, M., et al. (2013). An aberrant transcription factor network essential for Wnt signaling and stem cell maintenance in glioblastoma. Cell Rep. 3 (5), 1567–1579. doi:10.1016/j.celrep.2013.04.021
Robinson, M. D., and Oshlack, A. (2010). A scaling normalization method for differential expression analysis of RNA-seq data. Genome Biol. 11 (3), R25. doi:10.1186/gb-2010-11-3-r25
Shabihkhani, M., Telesca, D., Movassaghi, M., Naeini, Y. B., Naeini, K. M., Hojat, S. A., et al. (2017). Incidence, survival, pathology, and genetics of adult Latino Americans with glioblastoma. J. Neurooncol. 132 (2), 351–358. doi:10.1007/s11060-017-2377-0
Shannon, P., Markiel, A., Ozier, O., Baliga, N. S., Wang, J. T., Ramage, D., et al. (2003). Cytoscape: a software environment for integrated models of biomolecular interaction networks. Genome Res. 13 (11), 2498–2504. doi:10.1101/gr.1239303
Shao, H., Peng, T., Ji, Z., Su, J., and Zhou, X. (2013). Systematically studying kinase inhibitor induced signaling network signatures by integrating both therapeutic and side effects. PLoS One 8, 12e80832. doi:10.1371/journal.pone.0080832
Stark, R. B. G. (2011). DiffBind: Differential binding analysis of ChIP-seq peak data. Bioconductor. Available at: http://bioconductor.org/packages/release/bioc/vignettes/DiffBind/inst/doc/DiffBind.pdf.
Szklarczyk, D., Franceschini, A., Wyder, S., Forslund, K., Heller, D., Huerta-Cepas, J., et al. (2015). STRING v10: protein-protein interaction networks, integrated over the tree of life. Nucleic Acids Res. 43, D447–D452. doi:10.1093/nar/gku1003
Tang, Y., Li, M., Wang, J., Pan, Y., and Wu, F. X. (2015). CytoNCA: a cytoscape plugin for centrality analysis and evaluation of protein interaction networks. Biosystems. 127, 67–72. doi:10.1016/j.biosystems.2014.11.005
Vue, T. Y., Kollipara, R. K., Borromeo, M. D., Smith, T., Mashimo, T., Burns, D. K., et al. (2020). ASCL1 regulates neurodevelopmental transcription factors and cell cycle genes in brain tumors of glioma mouse models. Glia 68 (12), 2613–2630. doi:10.1002/glia.23873
Wang, H., Hernandez, J. M., and Van Mieghem, P. (2008). Betweenness centrality in a weighted network. Phys. Rev. E Stat. Nonlin. Soft Matter Phys. 77, 046105. doi:10.1103/PhysRevE.77.046105
Wang, X., Pei, Z., Hossain, A., Bai, Y., and Chen, G. (2021). Transcription factor-based gene therapy to treat glioblastoma through direct neuronal conversion. Cancer Biol. Med. 18 (3), 860–874. doi:10.20892/j.issn.2095-3941.2020.0499
Woods, L. M., Ali, F. R., Gomez, R., Chernukhin, I., Marcos, D., Parkinson, L. M., et al. (2022). Elevated ASCL1 activity creates de novo regulatory elements associated with neuronal differentiation. BMC Genomics 23 (1), 255. doi:10.1186/s12864-022-08495-8
Xu, H., Chen, J., and Qin, Z. (2017). Geographic variations in the incidence of glioblastoma and prognostic factors predictive of overall survival in US adults from 2004-2013. Front. Aging Neurosci. 9, 352. doi:10.3389/fnagi.2017.00352
Yu, G., Wang, L. G., Han, Y., and He, Q. Y. (2012). clusterProfiler: an R package for comparing biological themes among gene clusters. OMICS 16 (5), 284–287. doi:10.1089/omi.2011.0118
Yu, G., Wang, L. G., and He, Q. Y. (2015). ChIPseeker: an R/bioconductor package for ChIP peak annotation, comparison and visualization. Bioinformatics 31 (14), 2382–2383. doi:10.1093/bioinformatics/btv145
Keywords: achaete-scute homolog 1 gene, glioblastomas, bioinformatics, ChIP-seq, signaling pathways
Citation: Zhang N, Zhang J, Liu Z and Li T (2022) Identification of signaling pathways associated with achaete-scute homolog 1 in glioblastomas through ChIP-seq data bioinformatics. Front. Genet. 13:938712. doi: 10.3389/fgene.2022.938712
Received: 07 May 2022; Accepted: 06 July 2022;
Published: 06 September 2022.
Edited by:
Zhiwei Ji, Nanjing Agricultural University, ChinaCopyright © 2022 Zhang, Zhang, Liu and Li. This is an open-access article distributed under the terms of the Creative Commons Attribution License (CC BY). The use, distribution or reproduction in other forums is permitted, provided the original author(s) and the copyright owner(s) are credited and that the original publication in this journal is cited, in accordance with accepted academic practice. No use, distribution or reproduction is permitted which does not comply with these terms.
*Correspondence: Tushuai Li, tushuaili@jiangnan.edu.cn