- 1Animal Breeding Biology and Molecular Genetics, Albrecht Daniel Thaer-Institute for Agricultural and Horticultural Sciences, Humboldt-Universität zu Berlin, Berlin, Germany
- 2Department of Applied Sciences, Northumbria University, Newcastle Upon Tyne, United Kingdom
- 3Institute of Animal Breeding and Genetics, Justus-Liebig-Universität, Giessen, Germany
German Black Pied cattle (Deutsches Schwarzbuntes Niederungsrind, DSN) are an endangered dual-purpose cattle breed originating from the North Sea region. The population comprises about 2,500 cattle and is considered one of the ancestral populations of the modern Holstein breed. The current study aimed at defining the breeds closest related to DSN cattle, characterizing their genomic diversity and inbreeding. In addition, the detection of selection signatures between DSN and Holstein was a goal. Relationship analyses using fixation index (FST), phylogenetic, and admixture analyses were performed between DSN and 68 other breeds from the 1000 Bull Genomes Project. Nucleotide diversity, observed heterozygosity, and expected heterozygosity were calculated as metrics for genomic diversity. Inbreeding was measured as excess of homozygosity (FHom) and genomic inbreeding (FRoH) through runs of homozygosity (RoHs). Region-wide FST and cross-population-extended haplotype homozygosity (XP-EHH) between DSN and Holstein were used to detect selection signatures between the two breeds, and RoH islands were used to detect selection signatures within DSN and Holstein. DSN showed a close genetic relationship with breeds from the Netherlands, Belgium, Northern Germany, and Scandinavia, such as Dutch Friesian Red, Dutch Improved Red, Belgian Red White Campine, Red White Dual Purpose, Modern Angler, Modern Danish Red, and Holstein. The nucleotide diversity in DSN (0.151%) was higher than in Holstein (0.147%) and other breeds, e.g., Norwegian Red (0.149%), Red White Dual Purpose (0.149%), Swedish Red (0.149%), Hereford (0.145%), Angus (0.143%), and Jersey (0.136%). The FHom and FRoH values in DSN were among the lowest. Regions with high FST between DSN and Holstein, significant XP-EHH regions, and RoH islands detected in both breeds harbor candidate genes that were previously reported for milk, meat, fertility, production, and health traits, including one QTL detected in DSN for endoparasite infection resistance. The selection signatures between DSN and Holstein provide evidence of regions responsible for the dual-purpose properties of DSN and the milk type of Holstein. Despite the small population size, DSN has a high level of diversity and low inbreeding. FST supports its relatedness to breeds from the same geographic origin and provides information on potential gene pools that could be used to maintain diversity in DSN.
1 Introduction
Autochthonous populations are a crucial source of genetic diversity for the conservation of livestock species harboring important local adaptations (Medugorac et al., 2011). However, local breeds are typically less productive than intensively selected high-performing breeds. Consequently, keeping local breeds is less profitable (Gandini et al., 2007; Hiemstra et al., 2010). Thus, many local populations have been replaced by more profitable breeds which dramatically reduced the herd size of local populations. According to the Food and Agriculture Organization of the United Nations (FAO), 84% of all local breeds in Europe were considered at risk of extinction in 2021 (FAO, 2021).
This trend can also be observed for the German Black Pied cattle population (“Deutsches Schwarzbuntes Niederungsrind”, DSN). DSN is an endangered dual-purpose cattle breed from Germany (GEH e.V., 2020). Its initial farming dates back to the 18th century in the North Sea region of Germany and the Netherlands, where black and white animals were kept (today named DSN in Germany, and Dutch Friesian in the Netherlands). From there, black and white cattle were exported to North America and other parts of Europe. Strong selection on milk yield and dairy character resulted in the high-yielding dairy breed named Holstein Friesian (Holstein). These high-yielding cattle were brought back to Europe in the mid-1960s and rapidly became one of the main dairy cattle breeds worldwide. As a boomerang, DSN cattle were replaced almost entirely by Holstein (Brade, 2011; Brade and Brade, 2013). In 2020, the number of DSN herdbook cows was 2,452 (TGRDEU, 2021). According to the Society for the Conservation of Old and Endangered Livestock Breeds (GEH), populations with an effective population size (Ne) below 200 should be kept as genetic resources (Stier, 2021). For DSN, Ne was estimated as 85 (Jaeger et al., 2018). For that reason, this population became a genetic reserve in 1972, as a resource for the future of livestock breeding in Germany. This decision is in agreement with the Global Plan of Action for Animal Genetic Resources (FAO, 2007), in which it is stated that local breeds represent genetic resources that contain important alleles for the adaptation to local conditions (Hoffmann, 2011; Medugorac et al., 2011; Boettcher et al., 2014; Biscarini et al., 2015).
In this context, the breeding goal in DSN is to conserve the typical and beneficial characteristics of this breed, which are high robustness, fertility, longevity, resistance to multiple diseases, calm temperament, correct positioning of feet and legs, as well as high roughage feed intake capacity, making it suitable for grazing (BRS, 2021). Those characteristics confirm an advantage of DSN to organic farming. Not all those traits, however, are yet fully described in DSN and genetic variants affecting those traits are unknown. So far, studies on milk production (Korkuć et al., 2021), mastitis resistance (Meier et al., 2020), endoparasites infection resistance (May et al., 2019), and fertility traits (Wolf et al., 2021) identified some candidate variants and genes affecting those traits. To improve the identification of DSN-typical DNA variants underlying the phenotypic variance, a customized SNP chip was designed for DSN (Neumann et al., 2021). This DSN-specific SNP chip is currently used to support the characterization of genomic diversity and the identification of association between genetic variants and diverse phenotypes.
For an effective conservation plan, genomic diversity measurements are necessary to evaluate and ensure a minimum pool of genetic variants that provides sufficient adaptation capacity to changing environments and prevents inbreeding depression (Kristensen et al., 2015). Besides, typical measures of heterozygosity (observed and expected) and excess, of homozygosity, genome-wide nucleotide diversity, for example, has been shown to be useful when evaluating the genetic diversity status of a given population (Kardos et al., 2021). The nucleotide diversity is a region-wide metric that is used to quantify the degree of polymorphisms within a population. Additionally, runs of homozygosity (RoH) (McQuillan et al., 2008) have been used to detect inbreeding and signatures of selection on a genome-wide level (Mészáros et al., 2015; Mastrangelo et al., 2016; Gorssen et al., 2021).
Besides the joint historic origin of DSN and Holstein population (Naderi et al., 2020), little is known about which of the breeds that are maintained today are the most closely related to DSN. Due to the small population size of DSN, the risk of increasing inbreeding and losing diversity is high. Taking this into account, the breeds identified to be the most closely related to DSN on the genome level could potentially serve as genomic sources for maintaining and improving the genomic diversity within DSN and vice versa. If we look at the history and customs of people, we expect that breeds from the same region near the North Sea show a high level of genetic proximity (Brade and Brade, 2013; Felius et al., 2014). For that reason, analyses of the fixation index (FST), phylogeny, and admixture could provide important information about the relationship between DSN and other populations from the same or different geographical locations.
Information on the genomic diversity of local breeds such as DSN and its relationship to other breeds can be taken into account in livestock breeding for maintaining the diversity within a breed and improving resilience. In the case of inbreeding depression or the spread of tropical diseases due to climate change, for example, known genomic regions improving disease resistance could be used for genetic rescue programs (Medugorac et al., 2011; Kristensen et al., 2015; Kardos et al., 2021). This would be especially useful for closely related breeds such as DSN and Holstein. Since DSN cattle are maintained under less selection intensity than Holstein, we expect that DSN cattle contain sequence variants that increase phenotypic plasticity and resilience.
The aim of the current work was to characterize the genomic diversity of DSN and define the breeds most closely related to DSN. To obtain a better understanding of the genomic diversity of DSN, we calculated and compared genomic measurements (observed heterozygosity, expected heterozygosity, excess of homozygosity, nucleotide diversity, and genomic inbreeding) within DSN and between breeds. For the analyses, we used whole-genome sequencing data of 302 DSN cattle together with the sequence variants of 68 other taurine breeds obtained from the 1000 Bull Genomes Project (Hayes and Daetwyler, 2019). In order to support the diversity analyses and the definition of the breeds most closely related to DSN, relationship analyses (FST, phylogeny, and admixture) were performed between DSN and the other breeds. To detect regions of high differentiation between DSN and Holstein, we searched for signatures of selection within and between these two breeds.
2 Materials and methods
2.1 Genomic data
Sequence variants from whole-genome sequencing data of 302 DSN animals (Neumann et al., 2021) and 1,388 animals of additional 68 taurine breeds and one Auroch (Bos primigenius) from the 1000 Bull Genomes Project (Run 9) (Hayes and Daetwyler, 2019) were used in this study. Among the sequenced DSN cattle, there were 12 key ancestors of the last 44 to 20 years. Data pre-processing, sequence read mapping, variant discovery and recalibration for DSN have been described previously (Neumann et al., 2021). Basically, we followed the same pipeline guidelines as for the data from the 1000 Bull Genomes Project, using the Bos taurus genome version ARS-UCD1.2 as reference (Rosen et al., 2020). From the 1000 Bull Genomes Project data, only breeds with at least five animals with a minimum average read depth of 8-fold were used (Table 1). The maximum number of animals per breed was restricted to 30, whereas these 30 animals were randomly selected. Exceptions were made for Holstein, where 150 animals were selected (with 30 animals randomly selected per country), and Red and White Dual Purpose, where all 42 available animals were used due to pre-knowledge about their genetic proximity to DSN (Neumann et al., 2021). The sequence variants from the 302 DSN and the other breeds were merged using BCFtools v.1.9 (Narasimhan et al., 2016). A total of 79,019,242 biallelic autosomal variants (72,329,983 SNPs and 6,689,259 indels) occurring in the tranche 99% (from the Variant Recalibration performed by 1000 Bull Genomes Project) and with a call rate ≥ 0.95, were considered in our analyses (Supplementary Figure S1).
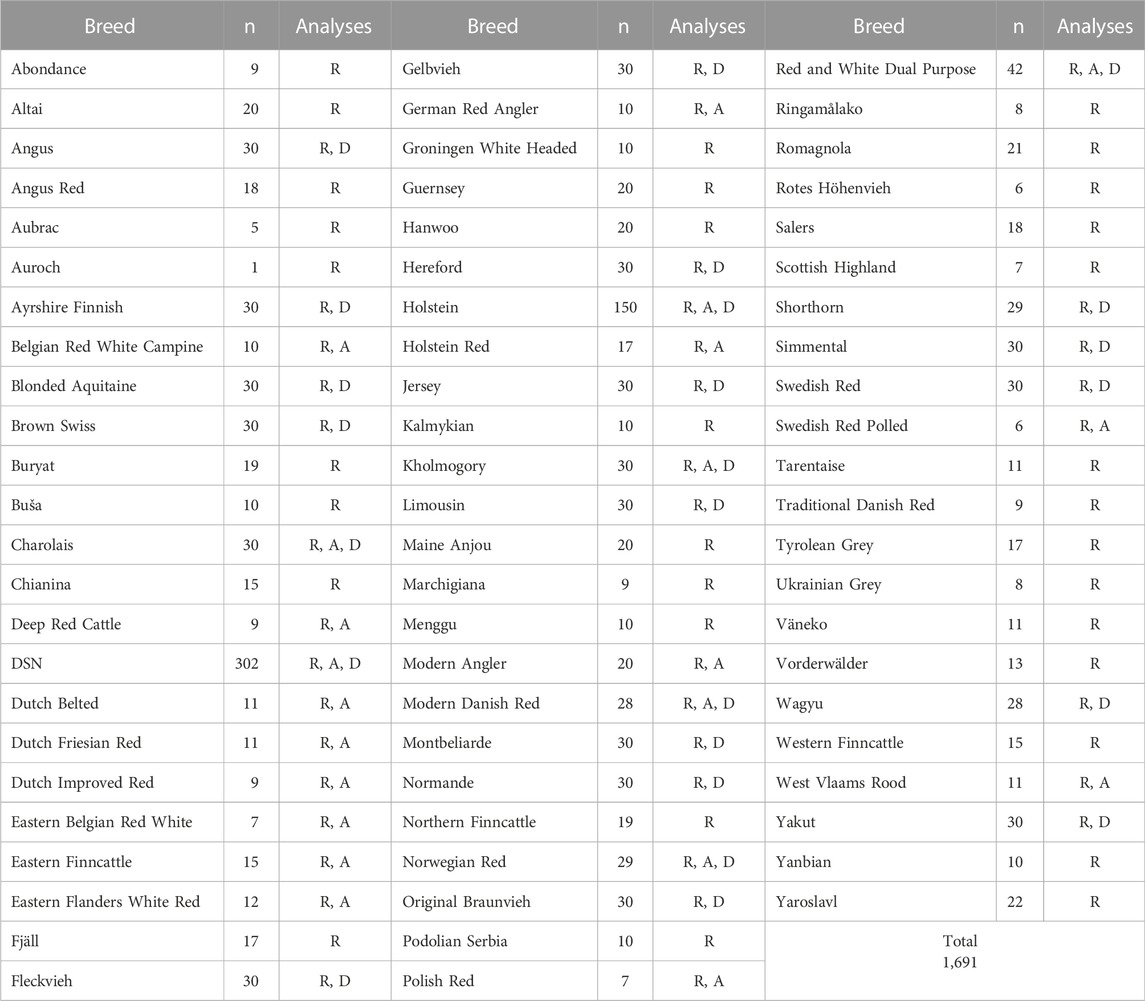
TABLE 1. Number of animals selected per breed and analyses in which they were included. R stands for ‘Relationship’ including phylogeny and FST (all breeds), A stands for ‘Admixture’ (20 breeds with highest FST), and D for ‘Diversity’ (24 breeds with ≥ 25 animals).
For the phylogenetic tree and admixture analyses, the available 79,019,242 sequence variants were pruned (--indep-pairwise) with PLINK v2 (Purcell et al., 2007) using a r2 threshold of 0.6, a window size of 50 SNPs, and a step-size of 5 SNPs to 23,059,286 variants. The same parameters for pruning were also used for other cattle analyses based on WGS (Xia et al., 2021; Zhang et al., 2022), except for a higher, more conservative r2 of 0.6, used at this point to keep variants in medium LD. All 68 breeds were used in the phylogenetic tree and in the FST analyses. Subsequently, the 20 breeds most closely related to DSN according to the estimated FST values were used in the admixture analysis.
Diversity and inbreeding measures were calculated for 24 breeds (Table 1) which had at least 25 animals. According to the FAO recommendation, 25 is the minimum sample count for a precise genetic diversity description of a population (FAO, 2011). For these 24 breeds, the initial 79,019,242 sequence variants were filtered down to 34,856,428 variants segregating among the 1,118 animals of those 24 breeds.
2.2 Relationship analyses
2.2.1 Phylogenetic tree
To study the relationship between the available breeds and to detect the most closely related breeds to DSN, a genome-wide phylogenetic tree was built for all Bos taurus autosomes (BTA). Based on 23,059,286 pruned sequence variants, Manhattan distances between animals were calculated and the Unweighted Pair Group Method with Arithmetic mean (UPGMA) algorithm implemented in the biotite library v0.35.0 (Kunzmann and Hamacher, 2018) in Python was used for clustering. The phylogenetic tree was visualized using iTOL v6 (Letunic and Bork, 2019). Individuals of the same breed were collapsed to a branch labelled with the breed’s name. One auroch (Bos primigenius) was added as an outgroup in order to root the tree. Animals of a specific breed clustering outside the expected breed branch were removed. In the case of multiple clusters for a single breed, only the biggest cluster was kept. In addition, to detect migration events, a maximum likelihood tree allowing two migration events with bootstrap in blocks of 500 variants was built using TreeMix v1.13 (Pickrell and Pritchard, 2012). Number of migrations (m) was defined based on the Evanno method (Evanno et al., 2005) as implemented in the R package OptM v0.1.6 (Fitak, 2021). Different m from 2 to 6 were tested and a maximum Δm = 8.66 was estimated when m = 2 edges were selected.
2.2.2 FST calculation
Pairwise FST values between DSN and the other 68 breeds were calculated using variants segregating in either DSN or the other breed from the initial dataset of 79,019,242 sequence variants. FST values were estimated based on Hudson’s method (Hudson et al., 1992) using the scikit-allel v1.3.1 library (Miles et al., 2020) in Python. As discussed by Bhatia et al. (Bhatia et al., 2013), Hudson’s method performs better when the sample size per breed varies largely and demands less computational power for big datasets.
2.2.3 Admixture
The population structure analysis was done with the Admixture v1.3 software (Alexander et al., 2009). For this analysis, we used the segregating sequence variants of the pruned dataset (the same used for the phylogenetic analysis) of the 20 breeds most closely related to DSN according to their FST values. The number of animals for DSN and Holstein was reduced to 50, which were selected based on kinship. The 50 least related animals were selected based on a genomic relationship matrix (Yang et al., 2011) calculated with PLINK v2 (--make-rel) following a greedy approach starting with a randomly selected animal. Unsupervised analyses were performed for K (number of ancestral populations) ranging from 2 to 20 with 5-fold cross-validation (CV), whereof K = 4 was considered for interpretation, with the lowest CV error (CV error = 0.1448). K = 5, 6, and 7, also showed very low CV errors (Supplementary Figure S2). Those results were visualized with the CLUMPAK v1.1 software (Kopelman et al., 2015). Admixture between breeds was confirmed based with f3 statistics calculated on TreeMix v1.13 software using the threepop function over blocks of 10,000 variants.
2.3 Diversity analyses
2.3.1 Genomic diversity
The genomic variation within breeds was assessed as nucleotide diversity (π) (Nei and Li, 1979), and as observed (Ho) and expected (He) heterozygosity. Ho and He were estimated using vcftools v0.1.15 (Danecek et al., 2011), calculated relative to the total number of variants among all 24 breeds (34,856,428). This was done in order to remove bias on the number of segregating variants per breed, and to allow the comparison between breeds. A chi-squared test was performed between Ho and He for each breed using the package statsmodels v0.13.5 (Seabold and Perktold, 2010) in Python. Nucleotide diversity was calculated per window of 10 kb (πwindow) using the library scikit-allel v1.3.1 (Miles et al., 2020) in Python. The nucleotide diversity per chromosome was calculated as the mean (πChrMean) and median (πChrMedian) of all πwindow values of the respective chromosome, and total nucleotide diversity as the mean (πTotMean) and median (πTotMedian) of all πwindow values across the whole genome. Due to the non-parametric nature of the πwindow distributions, we also report median values in the Supplementary Materials (Supplementary Table S4). Since πTotMean and πTotMedian are highly correlated (Pearson correlation coefficient of 0.99), both values allow the same interpretation of the results. We preferred means over medians since means are widely used for nucleotide diversity in the literature, providing opportunities for comparisons. All π measures are shown as percentages, which means that final results were multiplied by 100.
2.3.2 Genomic inbreeding
Genomic inbreeding was assessed using two estimators:
(1) Excess of homozygosity (FHom) which is based on the method of moments (Li and Horvitz, 1953) was calculated as
and
(2) Inbreeding coefficient FRoH which is based on RoHs was estimated using BCFtools v1.9 with an assumed recombination rate of 10–8 per base pair (1 cM/Mb). Inbreeding was calculated for the 24 breeds that were used for diversity analysis. Allele frequency was estimated using vcftools v0.1.15 and used as an input parameter for BCFtools v1.9. RoHs were separated into five groups with the minimal lengths of 50 kb, 100 kb, 1 Mb, 2 Mb, and 4 Mb (McQuillan et al., 2008; Zhang et al., 2015; Forutan et al., 2018; Bhati et al., 2020; Dixit et al., 2020). FRoH was calculated for each group as
where LRoH is the length of a homozygous region and Lgenome the length of the genome covered by SNPs (2,487,849,970 bp for our dataset).
2.4 Signatures of selection
2.4.1 Region-wide FST between DSN and Holstein
FST values between DSN and Holstein were compared across the whole genome in windows of 10 kb. Only windows containing at least five sequence variants were considered. The top 0.01 percentile of the FST values were selected to point to potential differences in selection signatures of those breeds.
2.4.2 Cross-population-extended haplotype homozygosity
The cross-population-extended haplotype homozygosity (XP-EHH) (Sabeti et al., 2007) was calculated between DSN and Holstein using the R package rehh v3.2.2 (Gautier et al., 2017). All segregating variants occurring in DSN or Holstein were considered. Variants were initially phased using Beagle v5.1 (Browning et al., 2018). Positive XP-EHH scores represent variants positively selected in DSN compared to Holstein, and negative scores correspond to variants positively selected in Holstein compared to DSN. p-values were corrected for multiple testing using Bonferroni and variants with a p-value<0.05 were considered as significant. The positions of neighboring significant variants were considered together as significant XP-EHH region for gene annotation.
2.4.3 RoH islands
RoH islands were defined as regions with the highest frequency of SNPs inside RoHs among DSN or Holstein. Frequency of SNP inside RoHs were calculated as the number of animals in which a SNP was reported inside a RoH, divided by the total number of animals in the breed. The threshold to define islands was taken as the top 0.05 percentile of frequencies. Afterwards, the positions of neighboring SNPs satisfying the defined percentile threshold were used to form islands.
2.4.4 Gene annotation
Regions with high difference in FST values between DSN and Holstein, significant XP-EHH regions, and RoH islands ± 250 kb flanking the start and end positions were scanned for protein-coding genes using the Ensembl database release 106 and for QTLs stored in the CattleQTLdb release 47 (Hu et al., 2022). The flanking 250 kb regions were included in our search since linkage groups and haplotype blocks can be quite large in DSN and reach, e.g., in the casein region on BTA 6, even 1 Mb (Korkuć et al., 2021). From the CattleQTLdb, QTLs and associations for “production”, “exterior”, “meat and carcass” (meat), “milk”, “health”, and “reproduction” (fertility) traits of a length <10 kb were considered. The references for the QTLs and associations were retrieved using PubMed IDs through the metapub v0.5.5 Python package. The complete list of publications is shown in Supplementary Tables S7–S9.
3 Results
3.1 Phylogenetic analysis
The phylogenetic analysis of DSN and 68 other cattle breeds including Auroch as an outgroup showed four clear clusters based on geographical origins (Figure 1). The majority of the breeds including DSN and Holstein formed a cluster of Northern European countries. Within this cluster, many Holstein Red cattle were found within the sub-cluster of Holstein cattle and vice versa (Supplementary Table S1), which was expected, since the coat color is the main difference. Also, animals of the breeds Modern Danish Red, Swedish Red, Norwegian Red, Ayrshire Finish, and Modern Angler were mixed with each other, showing wrong assignments or high relationship between those breeds exists (Supplementary Table S1). The cluster of Central Europe comprised all breeds from Austria, Switzerland, Southern Germany and France. Those are mainly dual-purpose breeds kept in mountainous areas. Jersey and Guernsey formed a separate cluster. The remaining cluster was formed by breeds from Eastern European countries, Central Italy, and Asian countries. This last cluster was the closest to Auroch.
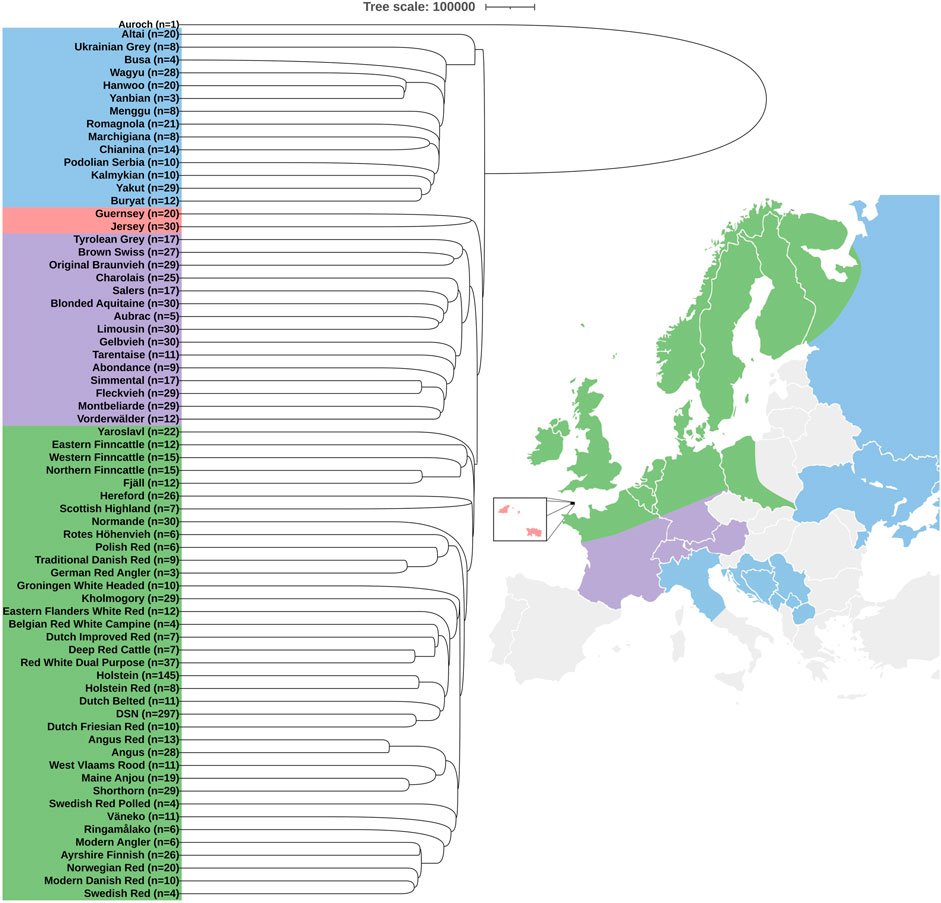
FIGURE 1. Phylogenetic tree and diversity analysis of 69 cattle breeds and Auroch as an outgroup. Colors in the phylogenetic tree represent geographical location of cattle origins: Northern Europe (green), Central Europe (violet), Jersey and Guernsey islands (red), and Eastern Europe together with Central Italy and Asia (blue). In parentheses, n is the number of animals representing the breed. Countries without any genomic breed information are highlighted in gray.
We observed that DSN clustered closely to Dutch Friesian Red, Dutch Belted, Holstein, Holstein Red, Red White Dual Purpose, Deep Red Cattle, Dutch Improved Red, Belgian Red White Campine, Eastern Flanders White Red, Kholmogory, and Groningen White Headed. Those are all breeds originating from Germany, the Netherlands, and Belgium, except for Kholmogory which is from Russia, but crossbreeding with Friesian cattle was reported (Dmitriev and Ernst, 1987).
It is important to mention that the Finncattle breeds, Fjäll, Scottish Highland, Yaroslavl, and Altai clustered outside our reported clusters depending on the pruning parameters (Supplementary Figure S3). In addition, migration events were observed between an ancestor of Shorthorn and Maine Anjou to Charolais and from Traditional Danish Red to an ancestor of Polish Red and Rotes Höhenvieh (Supplementary Figure S4).
3.2 Relatedness to DSN using FST
The same breeds as in the phylogenetic tree analysis showed the closest relationship to DSN in terms of lowest FST values. Those breeds with increasing FST values from 0.032 to 0.075 are Dutch Friesian Red, Dutch Improved Red, Eastern Belgian Red White, Belgian Red White Campine, Deep Red Cattle, Eastern Flanders White Red, Holstein Red, Kholmogory, Red and White Dual Purpose, Holstein, and Dutch Belted. Breeds which have also low FST values ranging between 0.053 and 0.078, but a little bit more distant in the phylogenetic analysis, are Modern Angler, Modern Danish Red, German Red Angler, Polish Red, Norwegian Red, Eastern Finncattle, West Vlaams Rood, and Swedish Red Polled (listed in increasing order). Charolais is the only breed that clustered outside the North Europe cluster in the phylogenetic tree, but had a low FST value of 0.070 to DSN. All FST values are listed in Supplementary Table S2.
3.3 Admixture
The admixture analysis (Figure 2) at K = 4 corroborated the results from the phylogenetic analysis by showing a common ancestral population between DSN, Dutch Friesian Red, Dutch Belted, Kholmogory, Dutch Improved Red, Eastern Flanders White Red, and Eastern Belgian Red White (in blue)—all breeds from the Netherlands and Belgium, except for Kholmogory. Polish Red, Swedish Red Polled, Modern Angler, Norwegian Red, German Red Angler, Modern Danish Red, Charolais, Eastern Finncattle, West Vlaams Rood, and Eastern Flanders White Red appear with a common ancestry (in orange). Holstein and Holstein Red share the same ancestry (in dark blue), with a clear level of introgression in most of the breeds, including DSN. Even though Charolais is the only breed located in a different cluster in the phylogenetic tree, admixture between Charolais, DSN, and breeds closely related to DSN exists. Admixture was detected by f3 statistics, whereof Charolais is significantly admixed from DSN and all the other 19 tested populations (Supplementary Table S3).
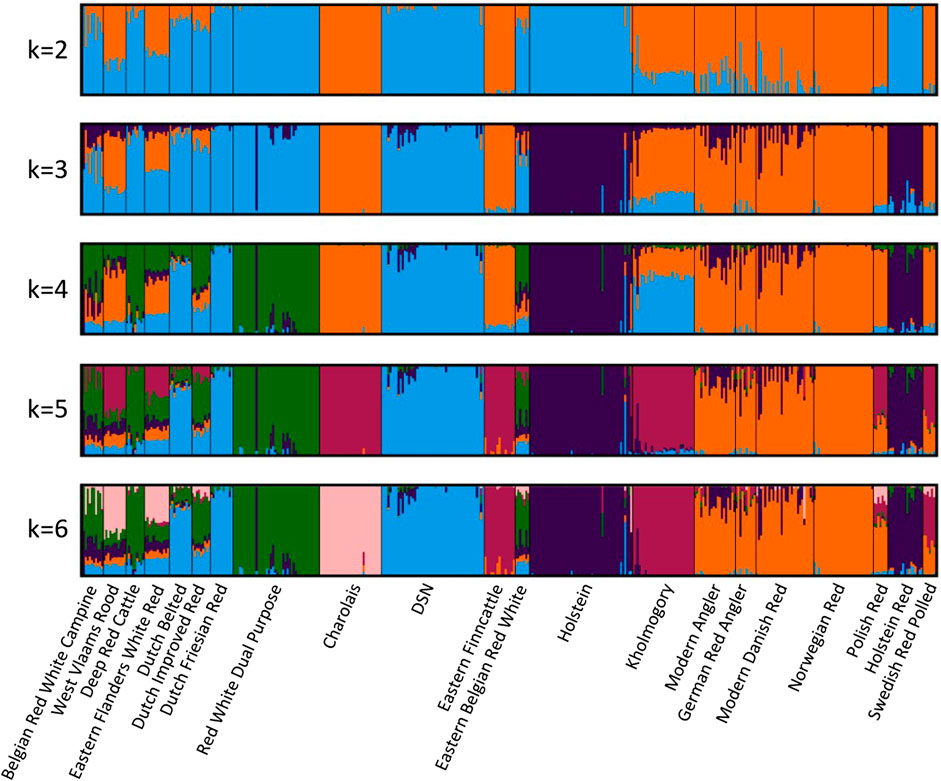
FIGURE 2. Admixture analysis between DSN and the 20 breeds closest to DSN according to the FST values. K = 4 was optimal for the 5-fold cross-validation. Colors indicate the ancestral relationship between breeds.
3.4 Genomic diversity
The average value of the expected heterozygosity per individual (He) ranged between 9.4% in DSN and 11.9% in Yakut and the average observed heterozygosity (Ho) ranged between 9.2% in Jersey and 11.3% in Yakut (Figure 3A). Although the expected and observed heterozygosity did not significantly differ in DSN, absolutely, DSN did not have the lowest observed heterozygosity. With a few exceptions (Holstein, Norwegian Red, Blonded Aquitaine, Normande, Montbeliarde, Shorthorn, and Brown Swiss), most breeds did not show a Ho significantly lower than He (Supplementary Table S4).
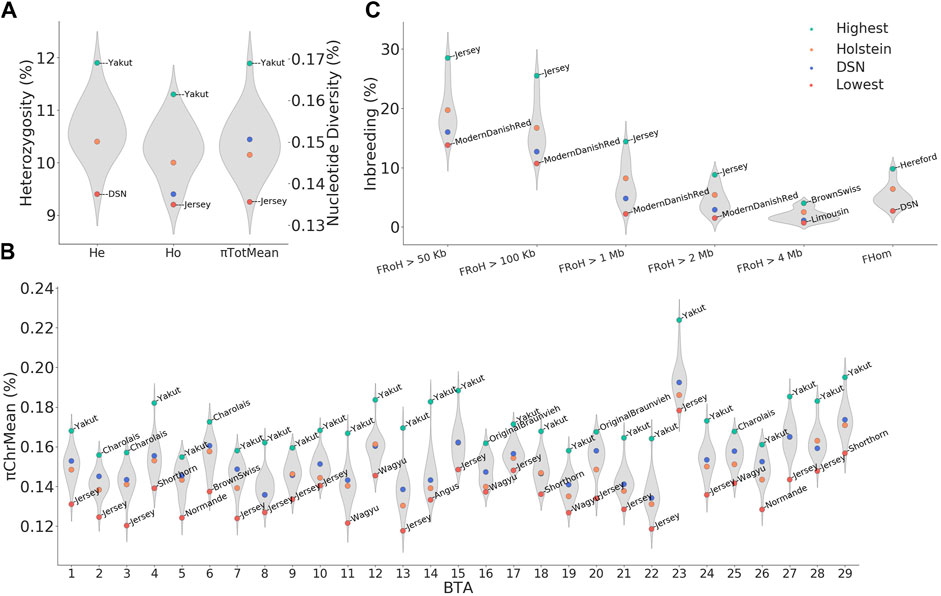
FIGURE 3. Overview of diversity measurements and inbreeding across breeds. (A) Average nucleotide diversity (πTotMean) observed heterozygosity (Ho), andexpected heterozygosity (He) distributions, (B) average nucleotide diversity per chromosome (πTotMean), (C) inbreeding defined based on runs ofhomozygosity (FRoH) and excess of homozygosity (FHom). Values for DSN and Holstein are highlighted in blue and orange, respectively. For each distribution, the highest and lowest values are highlighted in green and red, respectively, including the respective breed names.
The total average genomic diversity per individual πTotMean ranged between 0.136% in Jersey and 0.169% in Yakut (Figure 3A). In DSN, πTotMean was 0.151%. The DSN value was higher than in Holstein (0.147%) and in other breeds such as Red White Dual Purpose, Norwegian Red, Swedish Red, Ayrshire Finnish, Hereford, Angus, Normande, Montbeliarde, Shorthorn, Brown Swiss, Jersey, and Wagyu, but lower than in Modern Danish Red, Kholmogory, Charolais, Gelbvieh, Limousin, Simmental, Original Braunvieh, Fleckvieh, and Yakut (Supplementary Table S3). The values across all breeds for πTotMean and Ho (r = 0.87, p = 4.5 × 10−8) as well as between πTotMean and He (r = 0.88, p = 1.7 × 10−8) correlated highly significantly (Supplementary Table S5).
When looking at the nucleotide diversity per chromosome, πChrMean values in DSN ranged between 0.134% on BTA 22 and 0.192% on BTA 23. The highest nucleotide diversity was evident in all breeds on BTA 23 in the window between 19 and 37 Mb (Figure 3B; Supplementary Table S6). This highly polymorphic region contains many protein-coding genes including the major histocompatibility complex (MHC) and the bovine leukocyte antigen-BoLA. Consistent with the total genomic nucleotide diversity, Jersey and Yakut showed the lowest and highest levels of diversity for most of the chromosomes, respectively (Figure 3B).
3.5 Genomic inbreeding
The inbreeding rate estimated as excess of homozygosity (FHom) ranged from 2.7% in DSN to 9.8% in Hereford (Figure 3C). Hence, FHom in DSN was lower than in Holstein (6.4%) and all the other breeds. As expected, commercial breeds generally showed higher inbreeding rates, as seen for example in Charolais (7.0%), Shorthorn (8.5%), Brown Swiss (9.4%), and Hereford (9.8%).
In total, 1,337,471 RoHs were detected in the 302 sequenced DSN animals with an average length of 102 kb ranging from 526 bp to 14 Mb. On average, 4,428 RoHs were detected per DSN animal. In 150 Holstein animals, in total 507,198 RoHs were found with an average length of 128 kb ranging from 851 bp to 21 Mb. The average number of RoHs per Holstein animal was 4,226. This shows the presence of longer RoHs in Holstein in comparison to DSN.
The inbreeding rate as estimated by FRoH and considering all RoHs longer than 50 kb was on average 16.0% in DSN and 19.7% in Holstein (Figure 3C). From FRoH>50kb to FRoH>2Mb, lowest and highest inbreeding was always observed in Modern Danish Red and Jersey, respectively, while FRoH>4Mb ranged from 0.7% in Limousin to 4.0% in Brown Swiss (Figure 3C). FRoH>1Mb and FRoH>2Mb showed similar results to those detected in FHom (Figure 3C). Significant Pearson’s correlations of 0.60 (p = 1.9 × 10−3) and 0.62 (p = 1.3 × 10−3) were found between FHom and FRoH>1Mb, and FHom and FRoH>2Mb, respectively (Supplementary Table S5).
3.6 Region-wide FST between DSN and Holstein
The analysis of FST values as a measure for the genomic diversity between DSN and Holstein revealed regions of high differentiation between those populations (Figure 4). Peaks of high FST values for windows of 10 kb length were identified on BTA 1, 2, 3, 7, 8, 10, 16, 20, 22 and 24. FST values of 25 10 kb-regions were above the threshold of 0.38 which represents the 0.01 percentile of the FST distribution. Since the average FST between DSN and Holstein was 0.069, most variants showed only low FST values.
The genes encoding 1,4-alpha-glucan branching enzyme 1 (GBE1), HECT, C2 and WW domain containing E3 ubiquitin protein ligase 2 (HECW2), and doublecortin domain containing 2C (DCDC2C) are located inside the 10 kb-regions on BTA 1, 2, and 8, respectively, with FST values between DSN and Holstein above the threshold. Additional 71 genes are located 250 kb up- or downstream of the start and end positions of the 25 detected 10 kb-regions above the threshold (Table 2). Regions on BTA 16 and 24 do not contain protein-coding genes. Annotation on QTLs and associations of all regions from CattleQTLdb are shown in Supplementary Table S7.
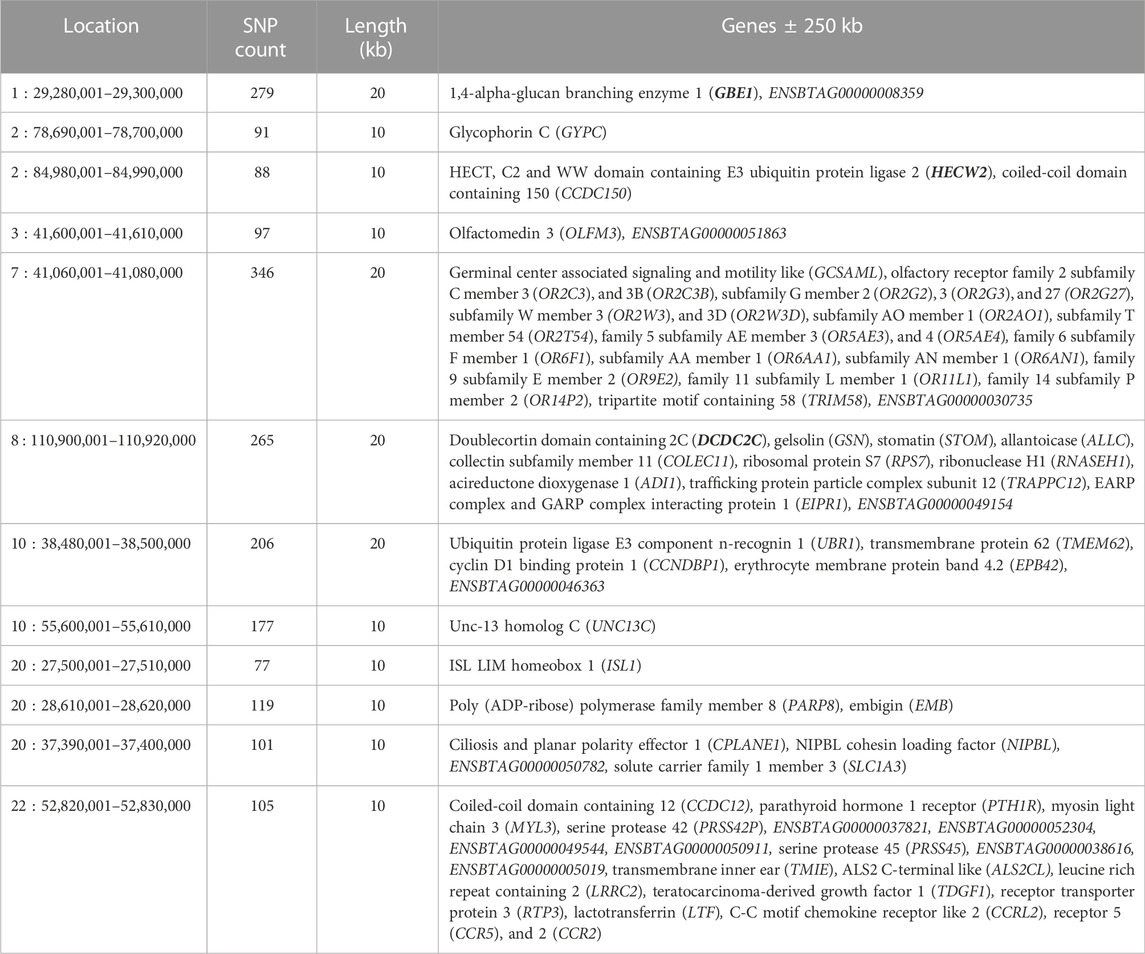
TABLE 2. Positional candidate genes in regions with the highest FST values between DSN and Holstein. Genes in bold are located directly inside the windows with the highest FST values. All other genes are located ± 250 kb from the start and end positions of the windows with the highest FST values. Consecutive windows displaying the same genes are shown together, this is the case for windows in BTA 1, 7, 8, and 10, whereof length is 20 kb.
3.7 Cross-population-extended haplotype homozygosity
The analysis of XP-EHH identified 140 variants positively selected in DSN in comparison to Holstein and 80 variants positively selected in Holstein in comparison to DSN (Figure 5). Those variants are located in DSN in four regions on BTA 5, 12, 18, and 29, and in Holstein in five regions on BTA 2, 8, 10, 18, and 23 (Table 3). The highest number of significant variants was detected on BTA 12 in DSN (124 variants) and on BTA 18 in Holstein (57 variants). 73% of the significant variants were intronic, while 26% were intergenic and 1% downstream of genes.
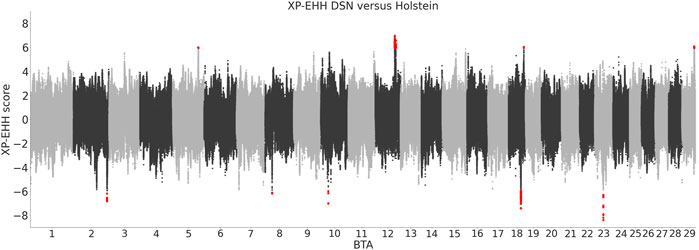
FIGURE 5. XP-EHH scores between DSN and Holstein. Positive scores represent variants positively selected in DSN in comparison to Holstein, and negative scores represent variants positively selected in Holstein in comparison to DSN. Significant scores are shown in red.
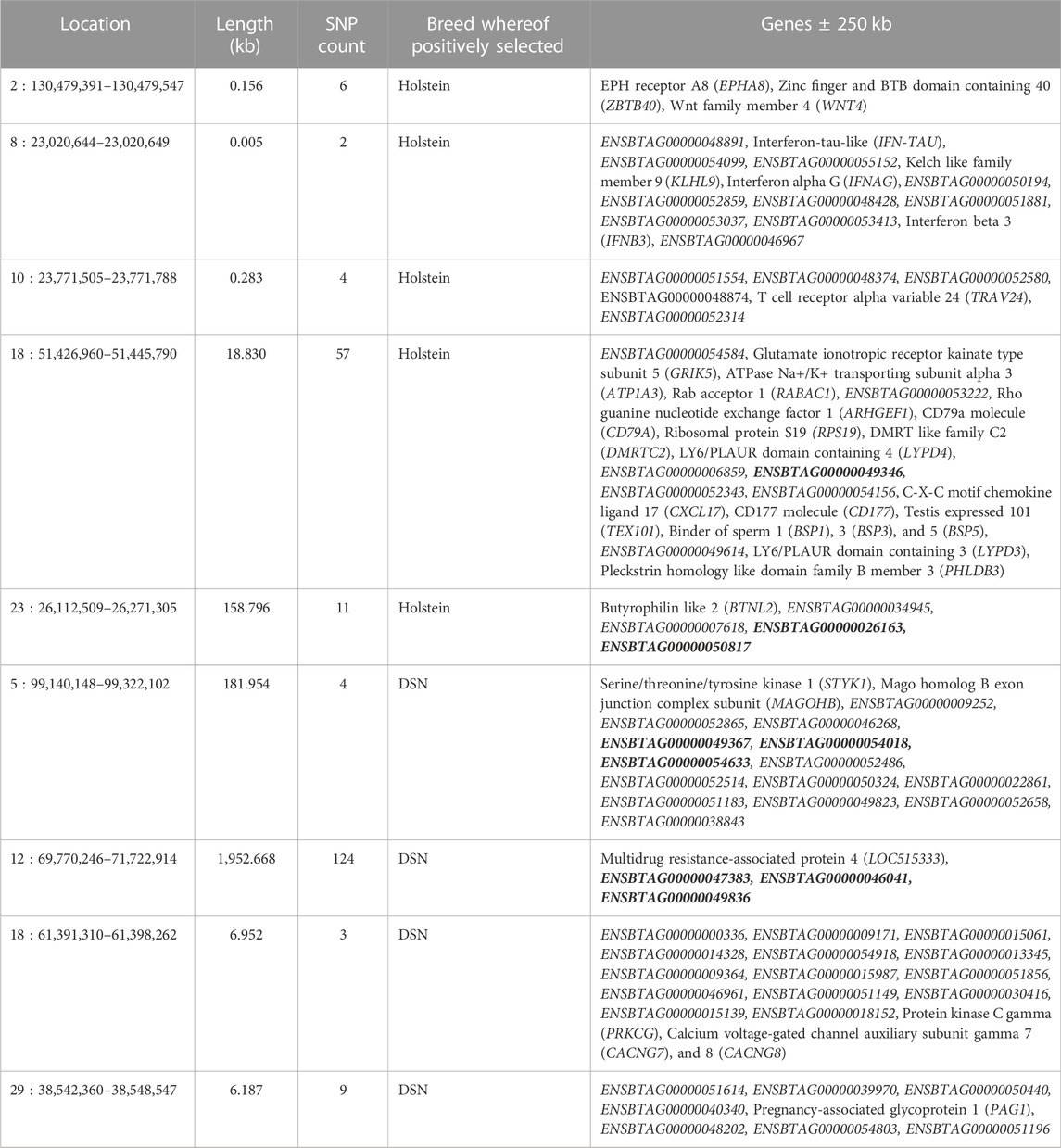
TABLE 3. Positional candidate genes in regions with significant XP-EHH regions between DSN and Holstein. Genes in bold are located directly within the regions. All other genes are located ± 250 kb from the start and end positions of the regions.
Seven genes are located directly within the identified XP-EHH regions, among them four in DSN and three in Holstein. When considering genes located 250 kb up- or downstream of the start and end positions of the regions, 110 genes are detected, 49 in DSN, and 61 in Holstein. Furthermore, no overlaps were detected between regions with high FST and XP-EHH regions. Annotation of genes in QTLs and associations of all regions from CattleQTLdb are shown in Supplementary Table S8.
3.8 RoH islands
Based on the frequency of SNPs inside RoHs, 21 RoH islands were detected in DSN and 19 in Holstein. Supplementary Figure S5 shows the frequency of SNPs inside RoHs for DSN and Holstein, and the threshold used in each case to define islands (0.66 in DSN and 0.61 in Holstein). Regions on BTA 1, 4, 14, and 18, where RoH islands were detected in both breeds in the same location, likely contributed essentially to selection (Table 4).
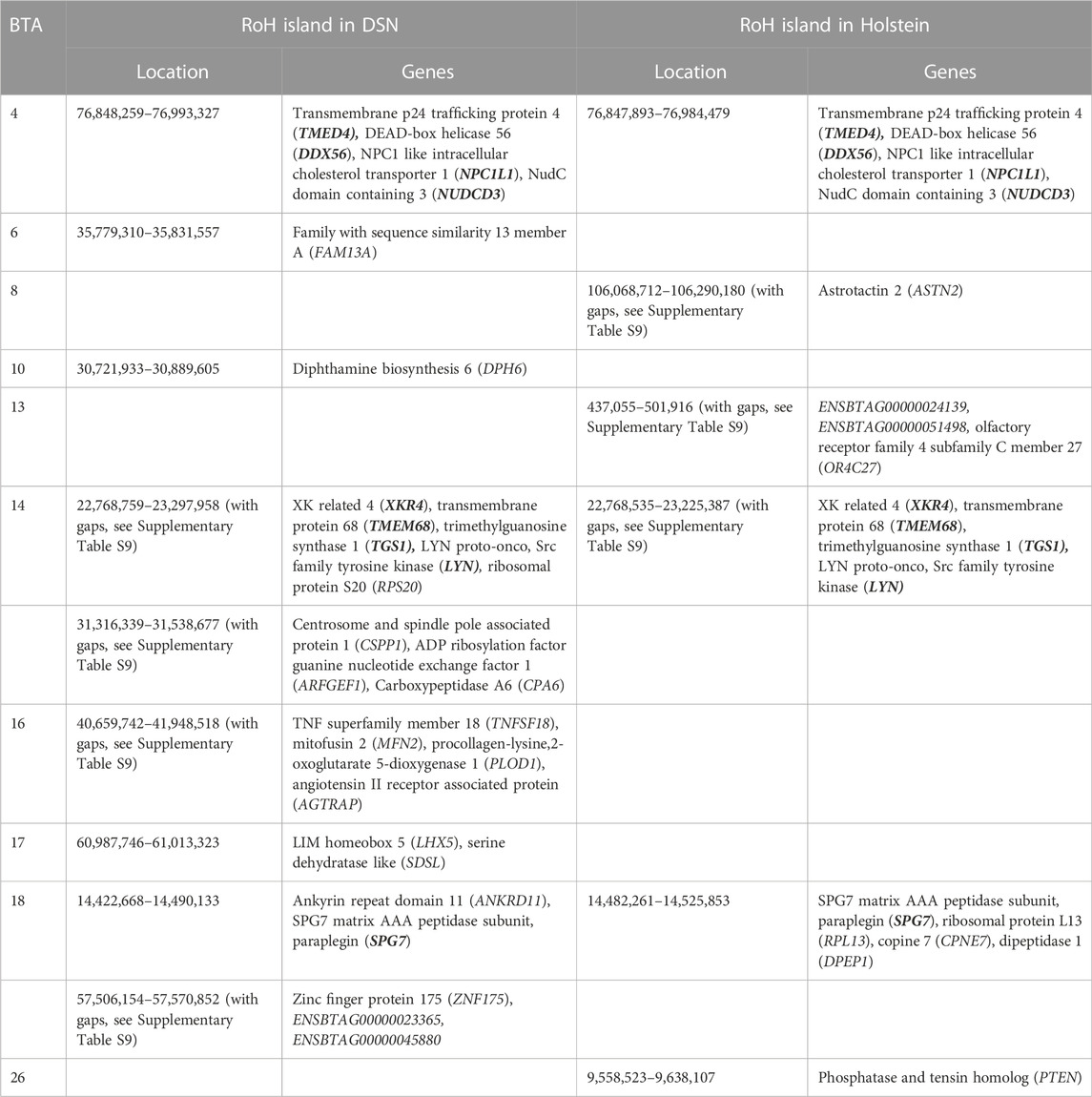
TABLE 4. Location of RoH islands and genes inside RoH islands in DSN and in Holstein. Genes in bold are common between breeds.
In total, 26 genes were found inside all RoH islands in DSN. For Holstein, 17 genes were located inside all RoH islands. Between DSN and Holstein, nine genes overlapped with the RoH islands on BTA 4, 14, and 18. Genes occurring 250 kb up- or downstream from start and end positions of RoH islands are shown in Supplementary Table S9.
No exact overlap was detected between RoH islands and regions with high FST or XP-EHH regions, neither in DSN nor in Holstein. In DSN, the smallest distance of a RoH island to an XP-EHH region was 3.8 Mb on BTA 18 (R2 = 0.001, D’ = 0.12), and to an FST window 7.5 Mb on BTA 10 (R2 = 0.001, D’ = 1). In Holstein, the smallest distance of a RoH island to an XP-EHH region was 3.0 Mb on BTA 20 (R2 = 0.03, D’ = 0.22) and to an FST window 4.6 Mb on BTA 8 (R2 = 0.03, D’ = 0.23).
4 Discussion
4.1 Relationship analyses
Considering the origin of DSN in the North Sea region, our expectation of cattle breeds from the same region such as Dutch Friesian Red, Holstein, and other breeds share a common ancestry with DSN was confirmed. But interestingly, Holstein was not the closest related breed to DSN, neither in FST nor phylogenetic analyses, despite their shared history. Asian and Eastern European countries, and Central Italy showed the closest cluster to Auroch, indicating the most ancient origin. Cattle domestication in Europe is believed to have started in Italy, with further migration of those cattle to Central and Northern Europe (Felius et al., 2014), which is consistent with the clusters of breeds in our phylogenetic tree. In addition, our clusters are also consistent with clusters previously reported by other genetic studies (Felius et al., 2011) that are also based on WGS data of cattle (Dutta et al., 2020). Since pruning parameters slightly affect the tree construction, and considering the complexity of the development of the cattle breeds in Europe (Felius et al., 2014), we cannot entirely explain how much the true relationship between breeds differs from our or reported findings.
We identified an FST value of 0.069 between DSN and Holstein. This value is consistent with the estimate of 0.068 which has been reported using 261 and 4,654 animals from Illumina BovineSNP50 Beadchip data, respectively (Naderi et al., 2020). Generally, FST values support our phylogenetic findings. Nevertheless, small differences were seen in the order of closest related breeds to DSN based on FST values, and breeds inside clusters closely related to DSN in the phylogenetic tree. This may be caused by the fact that the UPGMA algorithm used the average distance between all investigated animals at once to build the phylogenetic tree, while FST values were calculated pairwise between DSN and each breed separately. The latter might slightly bias the analysis towards those two breeds. In addition, the data used for the phylogenetic tree was pruned, while FST values were calculated using only unpruned variants segregating between two investigated breeds.
Although Charolais was not located inside the Northern European cluster in the phylogenetic tree, it showed a low FST value of 0.070 with DSN. Such a low FST value of 0.074 was also reported between Charolais and Holstein (Kelleher et al., 2017). The admixture and f3 analyses provided evidence for some admixture between Charolais and some other breeds from Northern Europe. Additionally, a migration event was observed between an ancestor of two breeds from the Northern European cluster, Shorthorn and Maine Anjou, to Charolais, which might explain the admixture results. An influence of shorthorn on Charolais –or of Durham cattle, ancestor of Shorthorn and Maine Anjou – has been reported before from the breeding history. The two breeds were separated by establishing independent herd books only in 1890 (Felius et al., 2014).
High admixture was observed between Modern Angler, Modern Danish Red, German Red Angler, and Norwegian Red, causing wrong assignments between those breeds in our phylogenetic analysis. This admixture, however, is consistent with recent findings (Schmidtmann et al., 2021).
Finally, we have to mention that every relationship study is dependent on the number of animals per breed, their kinship, and how good the given animals represent the breed. In our study, the number of animals per breed varied from 5 to 302, for diversity analyses from 28 to 302 sticking to FAO guidelines (FAO, 2011). We had no information on the relatedness of individuals and how good the animals represent each breed.
4.2 Genomic diversity
The average total nucleotide diversity (πTotMean) of DSN was similar to other breeds from Northern Europe, but slightly above average indicating good population management. Eastern European and Asian breeds showed higher πTotMean values than Northern and Central European breeds, likely due to less intensive breeding programs, genetic bottlenecks, or founder effects in comparison to the other breeds (Felius et al., 2014). The highest average chromosomal nucleotide diversity (πChrMean), which was found on BTA 23 at 19–37 Mb, can be attributed to the highly polymorphic region of the MHC. This high diversity around the MHC is observed in all mammals (Shiina et al., 2017).
Even though there was a high correlation between πTotMean and Ho and He, DSN showed the lowest He and one of the lowest Ho in comparison to other breeds, differently from its πTotMean result. One reason for this discrepancy might be the large number of 302 DSN animals in comparison to other breeds. However, this hypothesis was discarded since no correlation was detected between the number of animals per breed and πTotMean. Furthermore, πTotMean calculated for either 50 (0.1513%) or 302 DSN animals (0.1506%) which was almost identical. Nevertheless, no statistical difference was detected between Ho and He for DSN. The high nucleotide diversity and low heterozygosity likely results from the small herd size of DSN with 2,452 cows and only 36 breeding bulls (TGRDEU, 2021).
The breeds Jersey and Yakut showed the lowest and highest levels of diversity, respectively. Jersey cattle, which were originally restricted to the Jersey island, suffer from high inbreeding and low genetic diversity rates, which is seen even among animals not kept on the islands anymore (Huson et al., 2020). Lower genetic diversity is expected for insular than for continental populations (Frankham, 1997). Yakut, in contrast, is an ancient breed from Siberia, known for its adaptation to extreme low temperatures. The reported high nucleotide diversity of 0.173% in this breed might be due to lower artificial selection and a higher effective population size of the ancestral Asian taurine in comparison to European cattle (Weldenegodguad et al., 2019).
4.3 Genomic inbreeding
Inbreeding measured by the homozygosity index FHom was generally higher for intensively selected breeds such as Hereford, Brown Swiss, Shorthorn, Charolais, and Holstein. Jersey also showed particular high inbreeding. DSN had the lowest excess of homozygosity index, which demonstrates its good management as a genetic resource. This shows that in a local breed with only about 2,500 animals, diversity can be maintained through a breeding scheme that aims at less intensive selection for production traits (Gutiérrez-Reinoso et al., 2022), which is strongly different from Holstein, for example, where millions of animals are kept. In our analysis, Holstein cattle were available from four countries, the United States, Denmark, Germany, and the Netherlands, which probably led to slightly higher diversity rates than having only Holsteins from one country.
The same pattern for inbreeding was observed when using FRoH as indicated by the high correlation rates between inbreeding metrices. High correlation between FHom and FRoH was also observed in the literature (Mastrangelo et al., 2016). Our FRoH values were consistent with those previously reported, for example, for Original Braunvieh (FRoH>50 kb = 14.58%) (Bhati et al., 2020), Modern Danish Red (FRoH>10 kb = 11.84%), Holstein (FRoH>10 kb = 18.67%), and Jersey (FRoH>10 kb = 24.23%) (Zhang et al., 2015). Another aspect is the length of regions of homozygosity which reflects recent and ancient inbreeding. Longer RoHs indicate more recent inbreeding, while shorter RoHs indicate ancient inbreeding (Makanjuola et al., 2020). Moreover, recent inbreeding shows higher detrimental inbreeding depression effects (Makanjuola et al., 2020). Commercial breeds show more often long RoHs (Marras et al., 2015). This was the case for Hereford and Brown Swiss. Nevertheless, non-commercial breeds such as Jersey, Wagyu, and even Yakut also showed high average RoH lengths and high FRoH>4 Mb (Supplementary Table S4). DSN showed fewer longer RoHs, which were generally shorter, than in other breeds.
4.4 Signatures of selection
Signatures of selection were particularly examined in DSN and Holstein cattle. Considering DSN as a dual-purpose breed for milk and meat production, and Holstein as a high-yielding dairy breed, differentiated regions are expected to contain genes associated with traits influencing meat production, carcass, body conformation, and milk production.
4.4.1 Region-wide FST between DSN and Holstein
In total, we identified 25 high differentiating FST windows likely reflecting signatures of selection in DSN when comparing to Holstein. Two regions on BTA 20 and 10 have been detected previously (Naderi et al., 2020). All other regions were novel. Inside the top high differentiating FST regions, the three genes GBE1, HECW2, DCDC2C were located. GBE1 on BTA 1 was described in literature as responsible for the production of the glycogen branching enzyme, therefore, being involved in the carbohydrate and glycogen metabolisms. Glycogen is an important short-term energy storage molecule in the muscle. GBE1 was also detected in signatures of selection using RoHs in U.S. Holstein cattle (Kim et al., 2013). Its deficiency has also been associated with glycogen storage diseases and stillbirths in humans, cattle, and equines (Ward et al., 2004; Lee et al., 2011; Lorenz et al., 2011; Almodóvar-Payá et al., 2020). Furthermore, the FST region where GBE1 was located overlapped with two QTLs from CattleQTLdb for production traits (Crispim et al., 2015; Hamidi Hay and Roberts, 2017), growth and longevity (Supplementary Table S7). HECW2 on BTA 2 is responsible for protein ubiquitination. The gene was described as a candidate gene for milking speed in French Holstein (Marete A. et al., 2018b), aging and angiogenesis in humans (Rotin and Kumar, 2009; Walter et al., 2011; Choi et al., 2016; Berko et al., 2017). DCDC2C on BTA 8 was suggested to be associated with sperm formation in cattle (Wu et al., 2020), structural defects in cilia in sperm (Jumeau et al., 2017) and in cilia length in sensorial cells in humans (Grati et al., 2015). Moreover, the same region on BTA 8 presented a QTL for meat in the CattleQTLdb (McClure et al., 2012). Those genes point for the differentiation between signatures of selection between DSN and Holstein, including candidates for meat, milk, production, and fertility traits.
In addition to genes located directly inside the topmost differentiated genomic regions between DSN and Holstein, genes within 250 kb up- or downstream were analyzed. GYPC on BTA 2 has been previously reported as a candidate gene for body length (Vanvanhossou et al., 2020) and subclinical ketosis in Holstein (Soares et al., 2021), while CCDC150 was reported as candidate for milk and fat yield in Nordic Holstein cattle (Cai et al., 2020). BTA 7 showed a series of olfactory receptor genes. GCSAML, a germinal center associated signaling and motility like gene of mature B lymphocytes, was reported to be significantly down-regulated in Holstein cows under heat-stress conditions (Kim et al., 2021). Mammalian olfactory receptors are encoded by the largest mammalian multigene family, containing 881 genes on 26 chromosomes. Studies suggest physiological and behavior aspects of variation of olfactory receptor genes, e.g., associated to appetite regulation in livestock (Connor et al., 2018), which influences uptake of nutrients required for milk and meat production. This corroborates the idea of DSN well adapted to grazing with high roughage feed intake. In the BTA 7 region, QTLs for milk and meat production were found in the CattleQTLdb (Daetwyler et al., 2008; McClure et al., 2010; Marete A. et al., 2018b).
Other interesting genes near topmost differentiated genomic regions between DSN and Holstein are CCNDBP1 on BTA 10, a candidate for skeletal myogenesis (Huang et al., 2016); UNC13C on BTA 10 a candidate for feed efficiency (Freua et al., 2016); EMB on BTA 20 a candidate for mammary gland tissue development (Butty et al., 2017); NIPBL on BTA 20 a candidate for growth (de Simoni Gouveia et al., 2017; Wang et al., 2022) and previously detected as positively selected in German Holstein in the study between DSN and German Holstein using Illumina BovineSNP50 Beadchip (Naderi et al., 2020); and ALS2CL, LRRC2, and TDGF1 on BTA 22, which are candidates for milk production (Ibeagha-Awemu et al., 2016) and fertility (Wei et al., 2017; Tríbulo et al., 2018), and previously detected in highly differentiated regions between recent populations of Dutch Frisian and Holstein (Hulsegge et al., 2022). In the region on BTA 22, the gene LTF (lactotransferrin) was found which is a major iron-binding protein in milk and body secretions of bovine (Rejman et al., 1989; Pierce et al., 1991) with an antimicrobial activity (Bellamy et al., 1992). Furthermore, LTF was reported to influence casein yield (Cecchinato et al., 2014). Although the region on BTA 24 did not contain any gene, the same region was associated with endoparasite resistance in DSN (May et al., 2019).
4.4.2 Cross-population-extended haplotype homozygosity
Out of the four regions positively under selection in DSN, two are novel and two had been previously reported (Naderi et al., 2020). The region on BTA 12 has been reported before as positively selected in DSN in a study between DSN and German Holstein using Illumina BovineSNP50 Beadchip (Naderi et al., 2020). In this region, LOC515333 resides, a novel gene that has been so far annotated as coding the multidrug resistance-associated protein 4 (NCBI gene ID 515333). In cattle, this protein was reported to influence fertility traits since it is involved in the transport of prostaglandins and the regulation of oxytocin (Lacroix-Pépin et al., 2011). The XP-EHH region on BTA 18 which is close to the RoH island on the same chromosome also corroborates previous findings (Naderi et al., 2020). This region contains for instance CACNG7, a gene that was reported as a candidate gene for feed efficiency in Nellore cattle (Olivieri et al., 2016).
The regions on BTA 5 and 29 are novel. The region on BTA 5 has been repeatedly associated with milk production (Olsen et al., 2002; Bennewitz et al., 2003; Meredith et al., 2012; Rutten et al., 2013; Jiang et al., 2019). This region contains, for example, the gene STYK1, which has been reported as a candidate gene for heat stress response demonstrated through milk fatty acids alterations in German Holstein (Bohlouli et al., 2022). The region on BTA 29 contains the well described gene PGA1 (Xie et al., 1995; Klisch et al., 2005; López-Gatius et al., 2007), encoding the pregnancy associated glycoprotein-1, which is expressed in the placenta where it is crucial for a healthy gestation in cattle.
For Holstein, regions on 5 chromosomes were found. Only, the region on BTA 2 corroborates the previous study comparing DSN and German Holstein (Naderi et al., 2020). This is likely due to the different Holstein populations used in the different studies. Naderi et al. used Holstein cattle from Germany, while the current study used Holstein cattle from the 1000 Bull Genomes project (Hayes and Daetwyler, 2019) which originate from four different countries. The selection region on BTA 2, for instance, contains the WNT4 gene, which affects ovulation (Tríbulo et al., 2018), neurogenesis and embryogenesis in cattle (Gao et al., 2016).
The four other selection regions detected in Holstein contain genes such as IFNB3 on BTA 8, reported with evidence of inhibition against the bovine herspesvirus type 1 (da Silva et al., 2012), TRAV24 on BTA 10 which is T-cell receptor, BSP1, BSP2, and BSP3 on BTA 18 which bind the sperm proteins 1,3 and 5, respectively (D’Amours et al., 2012), and BTNL2 on BTA 23, which encodes butyrophilin (Afrache et al., 2012), as part of the immunoglobulin superfamily of transmembrane proteins in the MHC.
The absence of an overlap between region-wide FST and XP-EHH analyses is due to differences in the two methods, which are complementary. FST values were calculated based on allele frequencies, while XP-EHH values were calculated based on the decay of haplotype homozygosity, reflecting more recent selection signatures (Sabeti et al., 2007).
4.4.3 RoH islands
When signatures of selection were analyzed within breeds, nine genes were detected by RoH islands in both DSN and Holstein. Those nine genes are located on BTA 4, 14, and 18. The region on BTA 4 has been reported for mastitis resistance (Rupp and Boichard, 2003), growth (Lu et al., 2013), fertility (Grigoletto et al., 2020b), and primarly milk (Ibeagha-Awemu et al., 2016; Sanchez et al., 2017, 2019; Van Den Berg et al., 2020), while the region on BTA 14 was primarly reported for meat (Bolormaa et al., 2011; Neto et al., 2012; Saatchi et al., 2014; Sharma et al., 2014; Ali et al., 2015; Song et al., 2016; Kim et al., 2017; Akanno et al., 2018; Grigoletto et al., 2020a; Srikanth et al., 2020; Wang et al., 2020; Rezende et al., 2021), production (Snelling et al., 2010; Lu et al., 2013; Martínez et al., 2014; Saatchi et al., 2014; Akanno et al., 2018; Zhong et al., 2019; Zhang et al., 2020), and exterior traits (Pryce et al., 2011; Pausch et al., 2016; Wu et al., 2016; Marete A. G. et al., 2018; Bouwman et al., 2018; Tribout et al., 2020). Within this region, for example, we find the gene XKR4 associated with subcutaneous rump fat thickness and growth (Neto et al., 2012; Magalhã et al., 2016; An et al., 2019; Smith et al., 2019). Lastly, the region on BTA 18 was reported as a candidate region for tuberculosis resistance (Ring et al., 2019), milk (Cole et al., 2011; Ibeagha-Awemu et al., 2016; Benedet et al., 2019), and fertility traits (Cole et al., 2011; Gaddis et al., 2016) including spermatogenesis-associated proteins 33 and 2L (SPATA33 and SPATA2L) in the extended regions of 250 kb.
Regions detected in DSN, but not in Holstein, were very often associated with meat and carcass. Among the RoH islands detected in each breed 76.2% in DSN were associated with meat, but only 47.4% in Holstein. For milk, 95.2% of RoH islands in DSN and 78.9% of RoHs islands in Holstein were associated with milk production. Interestingly, the RoH region on BTA 28, which was found in DSN only, previously has been associated with milk production traits in a genome-wide association study in DSN (Korkuć et al., 2021).
Although differences were seen in signatures of selection, many signatures are shared between DSN and Holstein. This is consistent with the genetic relatedness and shared history of DSN and Holstein and the fact that both breeds had been selected for milk yield.
Considering all the results from signatures of selection, traits related to meat and milk showed the largest differences between DSN and Holstein, due to different selection goals. Genes affecting fertility, exterior, production, and health traits were also very frequent. Those findings are consistent with the characteristics of the breed-type and purposes described by the breeding organizations.
5 Conclusion
Despite the small population size of 2,500 animals, the DSN breed does not show any signs of loss of diversity or increased inbreeding compared to other taurine breeds. On the contrary, the inbreeding degree in DSN is even lower and the diversity higher than in Holstein. This is a remarkable result of the breeding strategy used for the maintenance of DSN as a genetic resource and shows the potential of maintaining small local populations while keeping diversity and controlling inbreeding. Our study provides the background for cattle breeds that are closely related to DSN and could, therefore, serve as an external gene pool to keep or even increase the diversity in DSN. Our analyses also provide evidence for high genomic diversity in breeds such as Yakut, Charolais, Kholmogory, and Modern Danish Red, while inbreeding was high in Jersey, Wagyu, Hereford, and Shorthorn, pointing to extra care needed for those breeds.
Moreover, specific genomic regions and positional candidate genes seem to be partially responsible for the DSN-specific characteristics. These include candidate genes previously identified in association studies with DSN, such as one region detected in DSN for endoparasite infection resistance, an important trait for pasture systems. In addition, these regions point to genes associated with traits that have not been studied yet in DSN, but in other breeds or species. Such regions are likely of particular interest for the conservation of DSN and the maintenance of its specific characteristics. Further studies are needed in order to elucidate the function of those regions and underlying causal sequence variants. Besides investigating milk and beef production, the study of new traits for disease resistance and resilience, such as heat stress, methane emissions or feed uptake capacity can further improve our understanding of the importance of DSN as a small local breed and as a genetic resource that contributes to conserve the whole genomic diversity of the species.
Data availability statement
Publicly available datasets were analyzed in this study. This data can be found here: https://www.ebi.ac.uk/ena/browser/view/PRJEB45822. Data from other breeds was provided within the frame of the 1000 Bull Genomes Project Consortium (Run 9).
Ethics statement
Ethical review and approval was not required for the animal study because samples were previously collected based on routine procedures on these farm animals. Ear tags were taken as part of the required registration procedure, blood samples were taken by a trained veterinarian to perform standard health recording. Written informed consent was obtained from the owners for the participation of their animals in this study.
Author contributions
GB, GN, PK, and DA designed the study. GN performed all analyses under PK, DA, and GB supervision. MW helped with the XP-EHH analysis. GN, PK, DA, and GB drafted the manuscript. All authors read and approved the final manuscript.
Funding
This work was financially supported by the German Federal Ministry of Food and Agriculture (BMEL) based on a decision of the Parliament of the Federal Republic of Germany, granted by the Federal Office for Agriculture and Food (BLE), grant number FKZ2818BM090 and 091. The article processing charge was funded by the Deutsche Forschungsgemeinschaft (DFG, German Research Foundation)—491192747 and the Open Access Publication Fund of Humboldt-Universität zu Berlin.
Acknowledgments
We acknowledge the 1000 Bull Genomes Consortium for providing the data, the Rinderproduktion Berlin-Brandenburg GmbH and DSN farms for supporting this project with data, tissue collection and their expertise in animal selection, and Sèyi Fridaïus Ulrich Vanvanhossou for help with the XP-EHH calculations in R.
Conflict of interest
The authors declare that the research was conducted in the absence of any commercial or financial relationships that could be construed as a potential conflict of interest.
Publisher’s note
All claims expressed in this article are solely those of the authors and do not necessarily represent those of their affiliated organizations, or those of the publisher, the editors and the reviewers. Any product that may be evaluated in this article, or claim that may be made by its manufacturer, is not guaranteed or endorsed by the publisher.
Supplementary material
The Supplementary Material for this article can be found online at: https://www.frontiersin.org/articles/10.3389/fgene.2022.993959/full#supplementary-material
References
Afrache, H., Gouret, P., Ainouche, S., Pontarotti, P., and Olive, D. (2012). The butyrophilin (BTN) gene family: From milk fat to the regulation of the immune response. Immunogenetics 64, 781–794. doi:10.1007/s00251-012-0619-z
Akanno, E. C., Chen, L., Abo-Ismail, M. K., Crowley, J. J., Wang, Z., Li, C., et al. (2018). Genome-wide association scan for heterotic quantitative trait loci in multi-breed and crossbred beef cattle. Genet. Sel. Evol. 50, 48. doi:10.1186/s12711-018-0405-y
Alexander, D. H., Novembre, J., and Lange, K. (2009). Fast model-based estimation of ancestry in unrelated individuals. Genome Res. 19, 1655–1664. doi:10.1101/gr.094052.109
Ali, A. A., Khatkar, M. S., Kadarmideen, H. N., and Thomson, P. C. (2015). Additive and epistatic genome-wide association for growth and ultrasound scan measures of carcass-related traits in Brahman cattle. J. Anim. Breed. Genet. 132, 187–197. doi:10.1111/jbg.12147
Almodóvar-Payá, A., Villarreal-Salazar, M., Luna, N., Real-Martínez, A., Andreu, A. L., et al. (2020). Preclinical research in glycogen storage diseases: A comprehensive review of current animal models. Int. J. Mol. Sci. 9621 (21), 9621. doi:10.3390/ijms21249621
An, B., Xia, J., Chang, T., Wang, X., Xu, L., Zhang, L., et al. (2019). Genome-wide association study reveals candidate genes associated with body measurement traits in Chinese Wagyu beef cattle. Anim. Genet. 50, 386–390. doi:10.1111/age.12805
Bellamy, W., Takase, M., Yamauchi, K., Wakabayashi, H., Kawase, K., and Tomita, M. (1992). Identification of the bactericidal domain of lactoferrin. Biochim. Biophys. Acta 1121, 130–136. doi:10.1016/0167-4838(92)90346-f
Benedet, A., Ho, P. N., Xiang, R., Bolormaa, S., De Marchi, M., Goddard, M. E., et al. (2019). The use of mid-infrared spectra to map genes affecting milk composition. J. Dairy Sci. 102, 7189–7203. doi:10.3168/jds.2018-15890
Bennewitz, J., Reinsch, N., Grohs, C., Levéziel, H., Malafosse, A., Thomsen, H., et al. (2003). Combined analysis of data from two granddaughter designs: A simple strategy for QTL confirmation and increasing experimental power in dairy cattle. Genet. Sel. Evol. 353 (35), 319–338. doi:10.1186/1297-9686-35-3-319
Berko, E. R., Cho, M. T., Eng, C., Shao, Y., Sweetser, D. A., Waxler, J., et al. (2017). De novo missense variants in HECW2 are associated with neurodevelopmental delay and hypotonia. J. Med. Genet. 54, 84–86. doi:10.1136/jmedgenet-2016-103943
Bhati, M., Kadri, N. K., Crysnanto, D., and Pausch, H. (2020). Assessing genomic diversity and signatures of selection in Original Braunvieh cattle using whole-genome sequencing data. BMC Genomics 21, 27. doi:10.1186/s12864-020-6446-y
Bhatia, G., Patterson, N., Sankararaman, S., and Price, A. L. (2013). Estimating and interpreting FST: The impact of rare variants. Genome Res. 23, 1514–1521. doi:10.1101/gr.154831.113
Biedermann, G. (2003). Zuchtplanung für die Erhaltung des Alten Schwarzbunten Niederungsrindes. Available at: https://service.ble.de/ptdb/index2.php?detail_id=84928&site_key=141.
Biscarini, F., Nicolazzi, E., Alessandra, S., Boettcher, P., and Gandini, G. (2015). Challenges and opportunities in genetic improvement of local livestock breeds. Front. Genet. 5, 33–16. doi:10.3389/fgene.2015.00033
Boettcher, P. J., Hoffmann, I., Baumung, R., Drucker, A. G., McManus, C., Berg, P., et al. (2014). Genetic resources and genomics for adaptation of livestock to climate change. Front. Genet. 5, 461. doi:10.3389/fgene.2014.00461
Bohlouli, M., Halli, K., Yin, T., Gengler, N., and König, S. (2022). Genome-wide associations for heat stress response suggest potential candidate genes underlying milk fatty acid composition in dairy cattle. J. Dairy Sci. 105, 3323–3340. doi:10.3168/jds.2021-21152
Bolormaa, S., Neto, P., Zhang, Y. D., Bunch, R. J., Harrison, B. E., Goddard, M. E., et al. (2011). A genome-wide association study of meat and carcass traits in Australian cattle. J. Anim. Sci. 89, 2297–2309. doi:10.2527/jas.2010-3138
Bouwman, A. C., Daetwyler, H. D., Chamberlain, A. J., Ponce, C. H., Sargolzaei, M., Schenkel, F. S., et al. (2018). Meta-analysis of genome-wide association studies for cattle stature identifies common genes that regulate body size in mammals. Nat. Genet. 50, 362–367. doi:10.1038/s41588-018-0056-5
Brade, W. (2011). Die Milchrinderzüchtung in der ehemaligen DDR eine retrospektive Bewertung. Berichte uber Landwirtsch. 89, 267.
Brade, W., and Brade, E. (2013). “Breeding history of German Holstein cattle,” in Berichte über Landwirtschaft (Hannover). https://www.cabdirect.org/cabdirect/abstract/20133389715.
Browning, B. L., Zhou, Y., and Browning, S. R. (2018). A one-penny imputed genome from next-generation reference panels. Am. J. Hum. Genet. 103, 338–348. doi:10.1016/j.ajhg.2018.07.015
BRS (2021). Black and White dual purpose. Available at: https://www.rind-schwein.de/brs-cattle/black-and-white-dual-purpose-en.html.
Butty, A. M., Frischknecht, M., Gredler, B., Neuenschwander, S., Moll, J., Bieber, A., et al. (2017). Genetic and genomic analysis of hyperthelia in Brown Swiss cattle. J. Dairy Sci. 100, 402–411. doi:10.3168/jds.2016-11420
Cai, Z., Dusza, M., Guldbrandtsen, B., Lund, M. S., and Sahana, G. (2020). Distinguishing pleiotropy from linked QTL between milk production traits and mastitis resistance in Nordic Holstein cattle. Genet. Sel. Evol. 52, 19. doi:10.1186/s12711-020-00538-6
Cecchinato, A., Ribeca, C., Chessa, S., Cipolat-Gotet, C., Maretto, F., Casellas, J., et al. (2014). Candidate gene association analysis for milk yield, composition, urea nitrogen and somatic cell scores in Brown Swiss cows. Animal 8, 1062–1070. doi:10.1017/S1751731114001098
Choi, K. S., Choi, H. J., Lee, J. K., Im, S., Zhang, H., Jeong, Y., et al. (2016). The endothelial E3 ligase HECW2 promotes endothelial cell junctions by increasing AMOTL1 protein stability via K63-linked ubiquitination. Cell. Signal. 28, 1642–1651. doi:10.1016/j.cellsig.2016.07.015
Cole, J. B., Wiggans, G. R., Ma, L., Sonstegard, T. S., Lawlor, T. J., Crooker, B. A., et al. (2011). Genome-wide association analysis of thirty one production, health, reproduction and body conformation traits in contemporary U.S. Holstein cows. BMC Genomics 12, 408. doi:10.1186/1471-2164-12-408
Connor, E. E., Zhou, Y., and Liu, G. E. (2018). The essence of appetite: Does olfactory receptor variation play a role? J. Anim. Sci. 96, 1551–1558. doi:10.1093/jas/sky068
Crispim, A. C., Kelly, M. J., Guimarães, S. E. F., Silva, E., Fortes, M. R. S., Wenceslau, R. R., et al. (2015). Multi-trait GWAS and new candidate genes annotation for growth curve parameters in brahman cattle. PLoS One 10, e0139906. doi:10.1371/journal.pone.0139906
da Silva, , , Sinani, D., and Jones, C. (2012). ICP27 protein encoded by bovine herpesvirus type 1 (bICP27) interferes with promoter activity of the bovine genes encoding beta interferon 1 (IFN-β1) and IFN-β3. Virus Res. 169, 162–168. doi:10.1016/j.virusres.2012.07.023
Daetwyler, H. D., Schenkel, F. S., Sargolzaei, M., and Robinson, J. A. B. (2008). A genome scan to detect quantitative trait loci for economically important traits in holstein cattle using two methods and a dense single nucleotide polymorphism map. J. Dairy Sci. 91, 3225–3236. doi:10.3168/jds.2007-0333
D’Amours, O., Bordeleau, L. J., Frenette, G., Blondin, P., Leclerc, P., and Sullivan, R. (2012). Binder of sperm 1 and epididymal sperm binding protein 1 are associated with different bull sperm subpopulations. Reproduction 143, 759–771. doi:10.1530/REP-11-0392
Danecek, P., Auton, A., Abecasis, G., Albers, C. A., Banks, E., DePristo, M. A., et al. (2011). The variant call format and VCFtools. Bioinformatics 27, 2156–2158. doi:10.1093/bioinformatics/btr330
de Simoni Gouveia, J. J., Paiva, S. R., McManus, C. M., Caetano, A. R., Kijas, J. W., Facó, O., et al. (2017). Genome-wide search for signatures of selection in three major Brazilian locally adapted sheep breeds. Livest. Sci. 197, 36–45. doi:10.1016/j.livsci.2017.01.006
Dixit, S. P., Singh, S., Ganguly, I., Bhatia, A. K., Sharma, A., Kumar, N. A., et al. (2020). Genome-wide runs of homozygosity revealed selection signatures in Bos indicus. Front. Genet. 11, 92. doi:10.3389/fgene.2020.00092
Dmitriev, N., and Ernst, L. (1987). Animal genetic resources of the USSR. Anim. Prod. Heal. Pap. Publ. by FAO 65, 18
Dutta, P., Talenti, A., Young, R., Jayaraman, S., Callaby, R., Jadhav, S. K., et al. (2020). Whole genome analysis of water buffalo and global cattle breeds highlights convergent signatures of domestication. Nat. Commun. 111 (11), 4739–4813. doi:10.1038/s41467-020-18550-1
Evanno, G., Regnaut, S., and Goudet, J. (2005). Detecting the number of clusters of individuals using the software structure: A simulation study. Mol. Ecol. 14, 2611–2620. doi:10.1111/j.1365-294X.2005.02553.x
FAO (2007). Global plan of action for animal genetic resources and the interlaken declaration. Rome, Italy: FAO. Available at: https://www.fao.org/publications/card/en/c/dafd2e54-21d2-51cc-a79d-457fb447a11b/
FAO (2011). Molecular genetic characterization of animal genetic resources.” in Commission on Genetic resources for Food and Agriculture, Food and Agriculture Organization of the United Nations.
FAO (2021). Risk status of livestock breeds. Available at: https://www.fao.org/sustainable-development-goals/indicators/252/en/.
Felius, M., Beerling, M. L., Buchanan, D. S., Theunissen, B., Koolmees, P. A., and Lenstra, J. A. (2014). On the history of cattle genetic resources. Diversity 6, 705–750. doi:10.3390/d6040705
Felius, M., Koolmees, P. A., Theunissen, B., Lenstra, J. A., Baumung, R., Manatrinon, S., et al. (2011). On the breeds of cattle—historic and current classifications. Divers. (Basel). 3, 660–692. doi:10.3390/d3040660
Fitak, R. R. (2021). OptM: Estimating the optimal number of migration edges on population trees using treemix. Biol. Methods Protoc. 6, bpab017. doi:10.1093/biomethods/bpab017
Forutan, M., Ansari Mahyari, S., Baes, C., Melzer, N., Schenkel, F. S., and Sargolzaei, M. (2018). Inbreeding and runs of homozygosity before and after genomic selection in North American Holstein cattle. BMC Genomics 19, 98. doi:10.1186/s12864-018-4453-z
Frankham, R. (1997). Do island populations have less genetic variation than mainland populations? Heredity 783 (78), 311–327. doi:10.1038/hdy.1997.46
Freua, M. C., Santana, M. H. A., Ventura, R. V., and Ferraz, J. B. S. (2016). Parameters of a dynamic mechanistic model of cattle growth retain enough biological interpretation for genotype-to-phenotype mapping. Genet. Mol. Res. 15, 15048931. doi:10.4238/gmr15048931
Gaddis, P., Null, D. J., and Cole, J. B. (2016). Explorations in genome-wide association studies and network analyses with dairy cattle fertility traits. J. Dairy Sci. 99, 6420–6435. doi:10.3168/jds.2015-10444
Gandini, G., Maltecca, C., Pizzi, F., Bagnato, A., and Rizzi, R. (2007). Comparing local and commercial breeds on functional traits and profitability: The case of reggiana dairy cattle. J. Dairy Sci. 90, 2004–2011. doi:10.3168/jds.2006-204
Gao, Y., Bai, C., Zheng, D., Li, C., Zhang, W., Li, M., et al. (2016). Combination of melatonin and Wnt-4 promotes neural cell differentiation in bovine amniotic epithelial cells and recovery from spinal cord injury. J. Pineal Res. 60, 303–312. doi:10.1111/jpi.12311
Gautier, M., Klassmann, A., and Vitalis, R. (2017). Rehh 2.0: a reimplementation of the R package rehh to detect positive selection from haplotype structure. Mol. Ecol. Resour. 17, 78–90. doi:10.1111/1755-0998.12634
GEH e.V (2020). Die Rote Liste im Überblick. Kassel, Germany: Soc. Conserv. Old Endanger. Livest. Breeds. https://www.g-e-h.de/index.php/rote-liste-menu/rote-liste.
Gorssen, W., Meyermans, R., Janssens, S., and Buys, N. (2021). A publicly available repository of ROH islands reveals signatures of selection in different livestock and pet species. Genet. Sel. Evol. 531 (53), 2–10. doi:10.1186/s12711-020-00599-7
Grati, M., Chakchouk, I., Ma, Q., Bensaid, M., Desmidt, A., Turki, N., et al. (2015). A missense mutation in DCDC2 causes human recessive deafness DFNB66, likely by interfering with sensory hair cell and supporting cell cilia length regulation. Hum. Mol. Genet. 24, 2482–2491. doi:10.1093/hmg/ddv009
Grigoletto, L., Ferraz, J. B. S., Oliveira, H. R., Eler, J. P., Bussiman, F. O., Abreu Silva, B. C., et al. (2020a). Genetic architecture of carcass and meat quality traits in Montana Tropical® composite beef cattle. Front. Genet. 11, 123. doi:10.3389/fgene.2020.00123
Grigoletto, L., Santana, M. H. A., Bressan, F. F., Eler, J. P., Nogueira, M. F. G., Kadarmideen, H. N., et al. (2020b). Genetic parameters and genome-wide association studies for anti-müllerian hormone levels and antral follicle populations measured after estrus synchronization in Nellore cattle. Animals. 10, 1185–1215. doi:10.3390/ani10071185
Gutiérrez-Reinoso, M. A., Aponte, P. M., and García-Herreros, M. (2022). A review of inbreeding depression in dairy cattle: Current status, emerging control strategies, and future prospects. J. Dairy Res. 89, 3–12. doi:10.1017/S0022029922000188
Hamidi Hay, E., and Roberts, A. (2017). Genomic prediction and genome-wide association analysis of female longevity in a composite beef cattle breed. J. Anim. Sci. 95, 1467–1471. doi:10.2527/jas.2016.1355
Hayes, B. J., and Daetwyler, H. D. (2019). 1000 bull genomes project to map simple and complex genetic traits in cattle: Applications and outcomes. Annu. Rev. Anim. Biosci. 7, 89–102. doi:10.1146/annurev-animal-020518-115024
Hiemstra, S. J., Haas, Y., and Gandini, G. (2010). Local cattle breeds in Europe. 1st ed. Wageningen, The Netherlands: Wageningen Academic Publishers. doi:10.3920/978-90-8686-697-7
Hoffmann, I. (2011). Livestock biodiversity and sustainability. Livest. Sci. 139, 69–79. doi:10.1016/j.livsci.2011.03.016
Hu, Z.-L., Park, C. A., and Reecy, J. M. (2022). Bringing the animal QTLdb and CorrDB into the future: Meeting new challenges and providing updated services. Nucleic Acids Res. 50, D956–D961. doi:10.1093/nar/gkab1116
Huang, Y., Chen, B., Ye, M., Liang, P., Zhangfang, Y., Huang, J., et al. (2016). Ccndbp1 is a new positive regulator of skeletal myogenesis. J. Cell. Sci. 129, 2767–2777. doi:10.1242/jcs.184234
Hudson, R. R., Slatkint, M., and Maddison, W. P. (1992). Estimation of levels of gene flow from DNA sequence data. Genetics 132, 583–589. doi:10.1093/genetics/132.2.583
Hulsegge, I., Oldenbroek, K., Bouwman, A., Veerkamp, R., and Windig, J. (2022). Selection and drift: A comparison between historic and recent Dutch friesian cattle and recent holstein friesian using WGS data. Animals. 329 (12), 329. doi:10.3390/ani12030329
Huson, H. J., Sonstegard, T. S., Godfrey, J., Hambrook, D., Wolfe, C., Wiggans, G., et al. (2020). A genetic investigation of island Jersey cattle, the foundation of the Jersey breed: Comparing population structure and selection to Guernsey, holstein, and United States Jersey cattle. Front. Genet. 11, 366. doi:10.3389/fgene.2020.00366
Ibeagha-Awemu, E. M., Peters, S. O., Akwanji, K. A., Imumorin, I. G., and Zhao, X. (2016). High density genome wide genotyping-by-sequencing and association identifies common and low frequency SNPs, and novel candidate genes influencing cow milk traits. Sci. Rep. 6, 31109. doi:10.1038/srep31109
Jaeger, M., Scheper, C., König, S., and Brügemann, K. (2018). Inbreeding and genetic relationships of the endangered dual-purpose black and white cattle breed (DSN) based on own genetic breed percentage calculations. Züchtungskd. 90, 262.
Jiang, J., Ma, L., Prakapenka, D., VanRaden, P. M., Cole, J. B., and Da, Y. (2019). A large-scale genome-wide association study in U.S. Holstein cattle. Front. Genet. 10, 412. doi:10.3389/fgene.2019.00412
Jumeau, F., Chalmel, F., Fernandez-Gomez, F. J., Carpentier, C., Obriot, H., Tardivel, M., et al. (2017). Defining the human sperm microtubulome: An integrated genomics approach. Biol. Reprod. 96, 93–106. doi:10.1095/biolreprod.116.143479
Kardos, M., Armstrong, E. E., Fitzpatrick, S. W., Hauser, S., Hedrick, P. W., Miller, J. M., et al. (2021). The crucial role of genome-wide genetic variation in conservation. Proc. Natl. Acad. Sci. U. S. A. 118, e2104642118. doi:10.1073/pnas.2104642118
Kelleher, M. M., Berry, D. P., Kearney, J. F., McParland, S., Buckley, F., and Purfield, D. C. (2017). Inference of population structure of purebred dairy and beef cattle using high-density genotype data. Animal 11, 15–23. doi:10.1017/S1751731116001099
Kim, E. S., Cole, J. B., Huson, H., Wiggans, G. R., Tassel, Van, Crooker, B. A., et al. (2013). Effect of artificial selection on runs of homozygosity in U.S. Holstein cattle. PLoS One 8, 813. doi:10.1371/journal.pone.0080813
Kim, E. T., Joo, S. S., Kim, D. H., Gu, B. H., Park, D. S., Atikur, R. M., et al. (2021). Common and differential dynamics of the function of peripheral blood mononuclear cells between holstein and Jersey cows in heat-stress environment. Animals. 11, 19–20. doi:10.3390/ani11010019
Kim, H. J., Sharma, A., Lee, S. H., Lee, D. H., Lim, D. J., Cho, Y. M., et al. (2017). Genetic association of PLAG1, SCD, CYP7B1 and FASN SNPs and their effects on carcass weight, intramuscular fat and fatty acid composition in Hanwoo steers (Korean cattle). Anim. Genet. 48, 251–252. doi:10.1111/age.12523
Klisch, K., Sousa, De, Beckers, J. F., Leiser, R., and Pich, A. (2005). Pregnancy associated glycoprotein-1, -6, -7, and -17 are major products of bovine binucleate trophoblast giant cells at midpregnancy. Mol. Reprod. Dev. 71, 453–460. doi:10.1002/mrd.20296
Kopelman, N. M., Mayzel, J., Jakobsson, M., Rosenberg, N. A., and Mayrose, I. (2015). Clumpak: A program for identifying clustering modes and packaging population structure inferences across K. Mol. Ecol. Resour. 15, 1179–1191. doi:10.1111/1755-0998.12387
Korkuć, P., Arends, D., May, K., König, S., and Brockmann, G. A. (2021). Genomic loci affecting milk production in German black pied cattle (DSN). Front. Genet. 12, 640039. doi:10.3389/fgene.2021.640039
Kristensen, T. N., Hoffmann, A. A., Pertoldi, C., and Stronen, A. V. (2015). What can livestock breeders learn from conservation genetics and vice versa? Front. Genet. 6, 38. doi:10.3389/fgene.2015.00038
Kunzmann, P., and Hamacher, K. (2018). Biotite: A unifying open source computational biology framework in Python. BMC Bioinforma. 2018 191 (19), 1–8. doi:10.1186/S12859-018-2367-Z
Lacroix-Pépin, N., Danyod, G., Krishnaswamy, N., Mondal, S., Rong, P. M., Chapdelaine, P., et al. (2011). The multidrug resistance-associated protein 4 (MRP4) appears as a functional carrier of prostaglandins regulated by oxytocin in the bovine endometrium. Endocrinology 152, 4993–5004. doi:10.1210/en.2011-1406
Lee, Y. C., Chang, C. J., Bali, D., Chen, Y. T., and Yan, Y. T. (2011). Glycogen-branching enzyme deficiency leads to abnormal cardiac development: Novel insights into glycogen storage disease IV. Hum. Mol. Genet. 20, 455–465. doi:10.1093/hmg/ddq492
Letunic, I., and Bork, P. (2019). Interactive tree of life (iTOL) v4: Recent updates and new developments. Web Serv. issue Publ. doi:10.1093/nar/gkz239
Li, C. C., and Horvitz, D. G. (1953). Some methods of estimating the inbreeding coefficient. Am. J. Hum. Genet. 5, 107.
López-Gatius, F., Hunter, R. H. F., Garbayo, J. M., Santolaria, P., Yániz, J., Serrano, B., et al. (2007). Plasma concentrations of pregnancy-associated glycoprotein-1 (PAG-1) in high producing dairy cows suffering early fetal loss during the warm season. Theriogenology 67, 1324–1330. doi:10.1016/j.theriogenology.2007.02.004
Lorenz, M. D., Coates, J. R., and Kent, M. (2011). Tetraparesis, hemiparesis, and ataxia. Handb. Vet. Neurol., 162–249. doi:10.1016/B978-1-4377-0651-2.10007-4
Lu, D., Miller, S., Sargolzaei, M., Kelly, M., Vander Voort, G., Caldwell, T., et al. (2013). Genome-wide association analyses for growth and feed efficiency traits in beef cattle. J. Anim. Sci. 91, 3612–3633. doi:10.2527/jas.2012-5716
Magalhã, A. F. B., De Camargo, G. M. F., Fernandes, J., Gordo, D. G. M., Tonussi, R. L., Costa, R. B., et al. (2016). Genome-wide association study of meat quality traits in Nellore cattle. PLoS One 11, e0157845. doi:10.1371/journal.pone.0157845
Makanjuola, B. O., Maltecca, C., Miglior, F., Schenkel, F. S., and Baes, C. F. (2020). Effect of recent and ancient inbreeding on production and fertility traits in Canadian Holsteins. BMC Genomics 21, 605–615. doi:10.1186/s12864-020-07031-w
Marete, A. G., Guldbrandtsen, B., Lund, M. S., Fritz, S., Sahana, G., and Boichard, D. (2018a). A meta-analysis including pre-selected sequence variants associated with seven traits in three French dairy cattle populations. Front. Genet. 9, 522. doi:10.3389/fgene.2018.00522
Marete, A., Sahana, G., Fritz, S., Lefebvre, R., Barbat, A., Lund, M. S., et al. (2018b). Genome-wide association study for milking speed in French Holstein cows. J. Dairy Sci. 101, 6205–6219. doi:10.3168/jds.2017-14067
Marras, G., Gaspa, G., Sorbolini, S., Dimauro, C., Ajmone-Marsan, P., Valentini, A., et al. (2015). Analysis of runs of homozygosity and their relationship with inbreeding in five cattle breeds farmed in Italy. Anim. Genet. 46, 110–121. doi:10.1111/age.12259
Martínez, R., Gómez, Y., and Martínez-Roch, J. F. (2014). Genome-wide association study on growth traits in Colombian creole breeds and crossbreeds with Zebu cattle. Genet. Mol. Res. 13, 6420–6432. doi:10.4238/2014.August.25.5
Mastrangelo, S., Tolone, M., Di Gerlando, R., Fontanesi, L., Sardina, M. T., and Portolano, B. (2016). Genomic inbreeding estimation in small populations: Evaluation of runs of homozygosity in three local dairy cattle breeds. Animal 10, 746–754. doi:10.1017/S1751731115002943
May, K., Scheper, C., Brügemann, K., Yin, T., Strube, C., Korkuć, P., et al. (2019). Genome-wide associations and functional gene analyses for endoparasite resistance in an endangered population of native German Black Pied cattle. BMC Genomics 20, 277. doi:10.1186/s12864-019-5659-4
McClure, M. C., Morsci, N. S., Schnabel, R. D., Kim, J. W., Yao, P., Rolf, M. M., et al. (2010). A genome scan for quantitative trait loci influencing carcass, post-natal growth and reproductive traits in commercial Angus cattle. Anim. Genet. 41, 597–607. doi:10.1111/j.1365-2052.2010.02063.x
McClure, M. C., Ramey, H. R., Rolf, M. M., McKay, S. D., Decker, J. E., Chapple, R. H., et al. (2012). Genome-wide association analysis for quantitative trait lociinfluencing Warner–Bratzler shear force in five taurine cattlebreeds. Anim. Genet. 43, 662–673. doi:10.1111/j.1365-2052.2012.02323.x
McQuillan, R., Leutenegger, A. L., Abdel-Rahman, R., Franklin, C. S., Pericic, M., Barac-Lauc, L., et al. (2008). Runs of homozygosity in European populations. Am. J. Hum. Genet. 83, 359–372. doi:10.1016/j.ajhg.2008.08.007
Medugorac, I., Veit-Kensch, C. E., Ramljak, J., Brka, M., Marković, B., Stojanović, S., et al. (2011). Conservation priorities of genetic diversity in domesticated metapopulations: A study in taurine cattle breeds. Ecol. Evol. 1, 408–420. doi:10.1002/ece3.39
Meier, S., Arends, D., Korkuć, P., Neumann, G. B., and Brockmann, G. A. (2020). A genome-wide association study for clinical mastitis in the dual-purpose German Black Pied cattle breed. J. Dairy Sci. 103, 10289–10298. doi:10.3168/jds.2020-18209
Meredith, B. K., Kearney, F. J., Finlay, E. K., Bradley, D. G., Fahey, A. G., Berry, D. P., et al. (2012). Genome-wide associations for milk production and somatic cell score in Holstein-Friesian cattle in Ireland. BMC Genet. 13, 21. doi:10.1186/1471-2156-13-21
Mészáros, G., Boison, S. A., O’Brien, Pérez, Ferencakovic, M., Curik, I., Da Silva, M. V. B., et al. (2015). Genomic analysis for managing small and endangered populations: A case study in tyrol grey cattle. Front. Genet. 6, 173. doi:10.3389/fgene.2015.00173
Miles, A., Ralph, P., Harding, N., Pisupati, R., et al. (2020). cggh/scikit-allel. doi:10.5281/ZENODO.3935797
Naderi, S., Moradi, M. H., Farhadian, M., Yin, T., Jaeger, M., Scheper, C., et al. (2020). Assessing selection signatures within and between selected lines of dual-purpose black and white and German Holstein cattle. Anim. Genet. 51, 391–408. doi:10.1111/age.12925
Narasimhan, V., Danecek, P., Scally, A., Xue, Y., Tyler-Smith, C., and Durbin, R. (2016). BCFtools/RoH: A hidden markov model approach for detecting autozygosity from next-generation sequencing data. Bioinformatics 32, 1749–1751. doi:10.1093/bioinformatics/btw044
Nei, M., and Li, W. H. (1979). Mathematical model for studying genetic variation in terms of restriction endonucleases. Proc. Natl. Acad. Sci. U. S. A. 76, 5269–5273. doi:10.1073/pnas.76.10.5269
Neto, P., Bunch, R. J., Harrison, B. E., and Barendse, W. (2012). Variation in the XKR4 gene was significantly associated with subcutaneous rump fat thickness in indicine and composite cattle. Anim. Genet. 43, 785–789. doi:10.1111/j.1365-2052.2012.02330.x
Neumann, G. B., Korkuć, P., Arends, D., Wolf, M. J., May, K., Reißmann, M., et al. (2021). Design and performance of a bovine 200 k SNP chip developed for endangered German Black Pied cattle (DSN). BMC Genomics 221 (22), 905–913. doi:10.1186/s12864-021-08237-2
Olivieri, B. F., Mercadante, M. E. Z., Cyrillo, J. N. D. S. G., Branco, R. H., Bonilha, S. F. M., De Albuquerque, L. G., et al. (2016). Genomic regions associated with feed efficiency indicator traits in an experimental Nellore cattle population. PLoS One 11, e0164390. doi:10.1371/journal.pone.0164390
Olsen, H. G., Gomez-Raya, L., Våge, D. I., Olsaker, I., Klungland, H., Svendsen, M., et al. (2002). A genome scan for quantitative trait loci affecting milk production in Norwegian dairy cattle. J. Dairy Sci. 85, 3124–3130. doi:10.3168/jds.S0022-0302(02)74400-7
Pausch, H., Emmerling, R., Schwarzenbacher, H., and Fries, R. (2016). A multi-trait meta-analysis with imputed sequence variants reveals twelve QTL for mammary gland morphology in Fleckvieh cattle. Genet. Sel. Evol. 48, 14. doi:10.1186/s12711-016-0190-4
Pickrell, J. K., and Pritchard, J. K. (2012). Inference of population splits and mixtures from genome-wide allele frequency data. PLOS Genet. 8, e1002967. doi:10.1371/journal.pgen.1002967
Pierce, A., Colavizza, D., Benaissa, M., Maes, P., Tartar, A., Montreuil, J., et al. (1991). Molecular cloning and sequence analysis of bovine lactotransferrin. Eur. J. Biochem. 196, 177–184. doi:10.1111/j.1432-1033.1991.tb15801.x
Pryce, J. E., Hayes, B. J., Bolormaa, S., and Goddard, M. E. (2011). Polymorphic regions affecting human height also control stature in cattle. Genetics 187, 981–984. doi:10.1534/genetics.110.123943
Purcell, S., Neale, B., Todd-Brown, K., Thomas, L., Ferreira, M. A. R., Bender, D., et al. (2007). Plink: A tool set for whole-genome association and population-based linkage analyses. Am. J. Hum. Genet. 81, 559–575. doi:10.1086/519795
Rejman, J. J., Hegarty, H. M., and Hurley, W. L. (1989). Purification and characterization of bovine lactoferrin from secretions of the involuting mammary gland: Identification of multiple molecular weight forms. Comp. Biochem. Physiol. B 93, 929–934. doi:10.1016/0305-0491(89)90068-0
Rezende, F. M., Rodriguez, E., Leal-Gutiérrez, J. D., Elzo, M. A., Johnson, D. D., Carr, C., et al. (2021). Genomic approaches reveal pleiotropic effects in crossbred beef cattle. Front. Genet. 12. doi:10.3389/fgene.2021.627055
Ring, S. C., Purfield, D. C., Good, M., Breslin, P., Ryan, E., Blom, A., et al. (2019). Variance components for bovine tuberculosis infection and multi-breed genome-wide association analysis using imputed whole genome sequence data. PLoS One 14, e0212067. doi:10.1371/journal.pone.0212067
Rosen, B. D., Bickhart, D. M., Schnabel, R. D., Koren, S., Elsik, C. G., Tseng, E., et al. (2020). De novo assembly of the cattle reference genome with single-molecule sequencing. GigaScience 9, 1–9. doi:10.1093/gigascience/giaa021
Rotin, D., and Kumar, S. (2009). Physiological functions of the HECT family of ubiquitin ligases. Nat. Rev. Mol. Cell. Biol. 106 (10), 398–409. doi:10.1038/nrm2690
Rupp, R., and Boichard, D. (2003). Genetics of resistance to mastitis in dairy cattle. Vet. Res. 34, 671–688. doi:10.1051/vetres:2003020
Rutten, M. J. M., Bouwman, A. C., Sprong, R. C., van Arendonk, J. A. M., and Visker, M. H. P. W. (2013). Genetic variation in vitamin B-12 content of bovine milk and its association with SNP along the bovine genome. PLoS One 8, e62382. doi:10.1371/journal.pone.0062382
Saatchi, M., Schnabel, R. D., Taylor, J. F., and Garrick, D. J. (2014). Large-effect pleiotropic or closely linked QTL segregate within and across ten US cattle breeds. BMC Genomics 15, 442. doi:10.1186/1471-2164-15-442
Sabeti, P. C., Varilly, P., Fry, B., Lohmueller, J., Hostetter, E., Cotsapas, C., et al. (2007). Genome-wide detection and characterization of positive selection in human populations. Nature 4497164 (449), 913–918. doi:10.1038/nature06250
Sanchez, M. P., Govignon-Gion, A., Croiseau, P., Fritz, S., Hozé, C., Miranda, G., et al. (2017). Within-breed and multi-breed GWAS on imputed whole-genome sequence variants reveal candidate mutations affecting milk protein composition in dairy cattle. Genet. Sel. Evol. 49, 68. doi:10.1186/s12711-017-0344-z
Sanchez, M. P., Ramayo-Caldas, Y., Wolf, V., Laithier, C., El Jabri, M., Michenet, A., et al. (2019). Sequence-based GWAS, network and pathway analyses reveal genes co-associated with milk cheese-making properties and milk composition in Montbéliarde cows. Genet. Sel. Evol. 51, 34. doi:10.1186/s12711-019-0473-7
Schmidtmann, C., Schönherz, A., Guldbrandtsen, B., Marjanovic, J., Calus, M., Hinrichs, D., et al. (2021). Assessing the genetic background and genomic relatedness of red cattle populations originating from Northern Europe. Genet. Sel. Evol. 531 (53), 23–18. doi:10.1186/s12711-021-00613-6
Seabold, S., and Perktold, J. (2010). statsmodels: Econometric and statistical modeling with python.” in Proceedings of the 9th Python in Science Conference
Sharma, A., Dang, C. G., Kim, K. S., Kim, J. J., Lee, H. K., Kim, H. C., et al. (2014). Validation of genetic polymorphisms on BTA14 associated with carcass trait in a commercial Hanwoo population. Anim. Genet. 45, 863–867. doi:10.1111/age.12204
Shiina, T., Blancher, A., Inoko, H., and Kulski, J. K. (2017). Comparative genomics of the human, macaque and mouse major histocompatibility complex. Immunology 150, 127–138. doi:10.1111/imm.12624
Smith, J. L., Wilson, M. L., Nilson, S. M., Rowan, T. N., Oldeschulte, D. L., Schnabel, R. D., et al. (2019). Genome-wide association and genotype by environment interactions for growth traits in U.S. Gelbvieh cattle. BMC Genomics 20, 926–1013. doi:10.1186/s12864-019-6231-y
Snelling, W. M., Allan, M. F., Keele, J. W., Kuehn, L. A., McDaneld, T., Smith, T. P. L., et al. (2010). Genome-wide association study of growth in crossbred beef cattle. J. Anim. Sci. 88, 837–848. doi:10.2527/jas.2009-2257
Soares, R. A. N., Vargas, G., Duffield, T., Schenkel, F., and Squires, E. J. (2021). Genome-wide association study and functional analyses for clinical and subclinical ketosis in Holstein cattle. J. Dairy Sci. 104, 10076–10089. doi:10.3168/jds.2020-20101
Song, Y., Xu, L., Chen, Y., Zhang, L., Gao, H., Zhu, B., et al. (2016). Genome-wide association study reveals the PLAG1 gene for knuckle, biceps and shank weight in simmental beef cattle. PLoS One 11, e0168316. doi:10.1371/journal.pone.0168316
Srikanth, K., Lee, S. H., Chung, K. Y., Park, J. E., Jang, G. W., Park, M. R., et al. (2020). A gene-set enrichment and protein–protein interaction network-based GWAS with regulatory SNPs identifies candidate genes and pathways associated with carcass traits in hanwoo cattle. Genes. 11, 316. doi:10.3390/genes11030316
Stier, K. (20212022). Die Rote Liste der gefährdeten Nutztierrassen der GEH. GEH. Available at: https://www.g-e-h.de/index.php/nachrichten/330-neueroteliste.
TGRDEU (2021). Rind: Deutsches Schwarzbuntes Niederungsrind. Available at: https://tgrdeu.genres.de/nutztiere/suche-nutztiere/genetik-detaildarstellung/?tx_sttgrdeu_nutztier%5Baction%5D=genetikDetail&tx_sttgrdeu_nutztier%5Bcontroller%5D=Nutztier&tx_sttgrdeu_nutztier%5Bg_id%5D=654&cHash=961248c5168bd52ab4538b0d7506f9e8.
Tribout, T., Croiseau, P., Lefebvre, R., Barbat, A., Boussaha, M., Fritz, S., et al. (2020). Confirmed effects of candidate variants for milk production, udder health, and udder morphology in dairy cattle. Genet. Sel. Evol. 52, 55. doi:10.1186/s12711-020-00575-1
Tríbulo, P., Siqueira, L. G. B., Oliveira, L. J., Scheffler, T., and Hansen, P. J. (2018). Identification of potential embryokines in the bovine reproductive tract. J. Dairy Sci. 101, 690–704. doi:10.3168/jds.2017-13221
Van Den Berg, I., Xiang, R., Jenko, J., Pausch, H., Boussaha, M., Schrooten, C., et al. (2020). Meta-analysis for milk fat and protein percentage using imputed sequence variant genotypes in 94, 321 cattle from eight cattle breeds. Genet. Sel. Evol. 52, 37. doi:10.1186/s12711-020-00556-4
Vanvanhossou, S. F. U., Scheper, C., Dossa, L. H., Yin, T., Brügemann, K., and König, S. (2020). A multi-breed GWAS for morphometric traits in four Beninese indigenous cattle breeds reveals loci associated with conformation, carcass and adaptive traits. BMC Genomics 211 (21), 783–816. doi:10.1186/s12864-020-07170-0
Walter, S., Atzmon, G., Demerath, E. W., Garcia, M. E., Kaplan, R. C., Kumari, M., et al. (2011). A genome-wide association study of aging. Neurobiol. Aging 32, e15–e28. doi:10.1016/j.neurobiolaging.2011.05.026
Wang, S., Raza, S. H. A., Zhang, K., Mei, C., Alamoudi, M. O., Aloufi, B. H., et al. (2022). Selection signatures of Qinchuan cattle based on whole-genome sequences. Anim. Biotechnol. 1, 3252. doi:10.1080/10495398.2022.2033252
Wang, Y., Zhang, F., Mukiibi, R., Chen, L., Vinsky, M., Plastow, G., et al. (2020). Genetic architecture of quantitative traits in beef cattle revealed by genome wide association studies of imputed whole genome sequence variants: II: Carcass merit traits. BMC Genomics 21, 6273. doi:10.1186/S12864-019-6273-1
Ward, T. L., Valberg, S. J., Adelson, D. L., Abbey, C. A., Binns, M. M., and Mickelson, J. R. (2004). Glycogen branching enzyme (GBE1) mutation causing equine glycogen storage disease IV. Mamm. Genome 15, 570–577. doi:10.1007/s00335-004-2369-1
Wei, Q., Zhong, L., Zhang, S., Mu, H., Xiang, J., Yue, L., et al. (2017). Bovine lineage specification revealed by single-cell gene expression analysis from zygote to blastocyst. Biol. Reprod. 97, 5–17. doi:10.1093/biolre/iox071
Weldenegodguad, M., Popov, R., Pokharel, K., Ammosov, I., Ming, Y., Ivanova, Z., et al. (2019). Whole-genome sequencing of three native cattle breeds originating from the northernmost cattle farming regions. Front. Genet. 10, 728. doi:10.3389/fgene.2018.00728
Wolf, M. J., Yin, T., Neumann, G. B., Korkuć, P., Brockmann, G. A., König, S., et al. (2021). Genome-wide association study using whole-genome sequence data for fertility, health indicator, and endoparasite infection traits in German black pied cattle. Genes. 1163 (12), 1163. doi:10.3390/genes12081163
Wu, S., Mipam, T. D., Xu, C., Zhao, W., Shah, M. A., Yi, C., et al. (2020). Testis transcriptome profiling identified genes involved in spermatogenic arrest of cattleyak. PLoS One 15, e0229503. doi:10.1371/journal.pone.0229503
Wu, X., Guldbrandtsen, B., Lund, M. S., and Sahana, G. (2016). Association analysis for feet and legs disorders with whole-genome sequence variants in 3 dairy cattle breeds. J. Dairy Sci. 99, 7221–7231. doi:10.3168/jds.2015-10705
Xia, X., Zhang, S., Zhang, H., Zhang, Z., Chen, N., Li, Z., et al. (2021). Assessing genomic diversity and signatures of selection in Jiaxian Red cattle using whole-genome sequencing data. BMC Genomics 22, 43–11. doi:10.1186/s12864-020-07340-0
Xie, S., Green, J., Beckers, J. F., and Roberts, R. M. (1995). The gene encoding bovine pregnancy-associated glycoprotein-1, an inactive member of the aspartic proteinase family. Gene 159, 193–197. doi:10.1016/0378-1119(94)00928-l
Yang, J., Lee, S. H., Goddard, M. E., and Visscher, P. M. (2011). Gcta: A tool for genome-wide complex trait analysis. Am. J. Hum. Genet. 88, 76–82. doi:10.1016/j.ajhg.2010.11.011
Zhang, F., Wang, Y., Mukiibi, R., Chen, L., Vinsky, M., Plastow, G., et al. (2020). Genetic architecture of quantitative traits in beef cattle revealed by genome wide association studies of imputed whole genome sequence variants: I: Feed efficiency and component traits. BMC Genomics 21, 6362. doi:10.1186/S12864-019-6362-1
Zhang, Q., Guldbrandtsen, B., Bosse, M., Lund, M. S., and Sahana, G. (2015). Runs of homozygosity and distribution of functional variants in the cattle genome. BMC Genomics 16, 542. doi:10.1186/s12864-015-1715-x
Zhang, S., Yao, Z., Li, X., Zhang, Z., Liu, X., Yang, P., et al. (2022). Assessing genomic diversity and signatures of selection in Pinan cattle using whole-genome sequencing data. BMC Genomics 23, 460–510. doi:10.1186/s12864-022-08645-y
Keywords: Bos taurus, Holstein, whole-genome sequencing, inbreeding, phylogenetic analysis, runs of homozygosity, selection signatures, 1000 Bull Genomes project
Citation: Neumann GB, Korkuć P, Arends D, Wolf MJ, May K, König S and Brockmann GA (2023) Genomic diversity and relationship analyses of endangered German Black Pied cattle (DSN) to 68 other taurine breeds based on whole-genome sequencing. Front. Genet. 13:993959. doi: 10.3389/fgene.2022.993959
Received: 14 July 2022; Accepted: 28 November 2022;
Published: 04 January 2023.
Edited by:
Stéphane Joost, Swiss Federal Institute of Technology Lausanne, SwitzerlandReviewed by:
Mehmet Gültas, South Westphalia University of Applied Sciences, GermanyHugo Oswaldo Toledo-Alvarado, National Autonomous University of Mexico, Mexico
Mario Barbato, Catholic University of the Sacred Heart, Italy
Copyright © 2023 Neumann, Korkuć, Arends, Wolf, May, König and Brockmann. This is an open-access article distributed under the terms of the Creative Commons Attribution License (CC BY). The use, distribution or reproduction in other forums is permitted, provided the original author(s) and the copyright owner(s) are credited and that the original publication in this journal is cited, in accordance with accepted academic practice. No use, distribution or reproduction is permitted which does not comply with these terms.
*Correspondence: Gudrun A. Brockmann, gudrun.brockmann@hu-berlin.de