- 1Department of Psychiatry, Yale University School of Medicine, New Haven, CT, United States
- 2National Center of Post-Traumatic Stress Disorder, VA CT Healthcare, West Haven, CT, United States
- 3Icahn School of Medicine at Mount Sinai, New York City, NY, United States
- 4Department of Genetic Epidemiology in Psychiatry, Central Institute of Mental Health, Medical Faculty Mannheim, Heidelberg University, Mannheim, Germany
- 5Department of Psychiatry and Behavioral Sciences, Texas A&M University, College Station, TX, United States
- 6Facultad de Ciencias, Universidad de los Andes, Bogotá, Colombia
Background: Alcohol use disorder (AUD) is a complex condition associated with adverse health consequences that affect millions of individuals worldwide. Epigenetic modifications, including DNA methylation (5 mC), have been associated with AUD and other alcohol-related traits. Epigenome-wide association studies (EWAS) have identified differentially methylated genes associated with AUD in human peripheral and brain tissue. More recently, epigenetic studies of AUD have also evaluated DNA hydroxymethylation (5 hmC) in the human brain. However, most of the epigenetic work in postmortem brain tissue has examined bulk tissue. In this study, we investigated neuronal-specific 5 mC and 5 hmC alterations at CpG sites associated with AUD in the human orbitofrontal cortex (OFC).
Methods: Neuronal nuclei from the OFC were evaluated in 34 human postmortem brain samples (10 AUD, 24 non-AUD). Reduced representation oxidative bisulfite sequencing was used to assess 5 mC and 5 hmC at the genome-wide level. Differential 5 mC and 5 hmC were evaluated using the methylKit R package and significance was set at false discovery rate < 0.05 and differential methylation > 2. Functional enrichment analyses were performed, and gene-level convergence was evaluated in an independent dataset that assessed 5 mC and 5 hmC of AUD in bulk cortical tissue.
Results: We identified 417 5 mC and 363 5hmC significant differential CpG sites associated with AUD, with 59% in gene promoters. Some of the identified genes have been previously implicated in alcohol consumption, including SYK, DNMT3A for 5 mC, GAD1, DLX1, DLX2, for 5 hmC and GATA4 in both. Convergence with a previous AUD 5 mC and 5 hmC study was observed for 28 genes. We also identified 5 and 35 differential regions for 5 mC and 5 hmC, respectively. Lastly, GWAS enrichment analysis showed an association with AUD for differential 5 mC genes.
Discussion: This study reveals neuronal-specific methylome and hydroxymethylome dysregulation associated with AUD, identifying both previously reported and potentially novel gene associations with AUD. Our findings provide new insights into the epigenomic dysregulation of AUD in the human brain.
1 Introduction
The detrimental effects of alcohol use disorder (AUD) are substantial, resulting in more than 150,000 deaths globally (GBD, 2019 Risk Factors Collaborators, 2020). AUD is characterized by persistent, uncontrollable, and excessive alcohol consumption despite its negative consequences. Although genome-wide association studies (GWAS) have identified genetic risk factors of AUD (Gelernter et al., 2019; Zhou et al., 2020; 2021; 2023), these only account for a portion of the variation observed.
Epigenetic mechanisms, such as DNA methylation (5mC), have been implicated in AUD and alcohol-related traits in human studies evaluating various tissues, including saliva, blood, and brain (Longley et al., 2021; Clark et al., 2022; Montalvo-Ortiz et al., 2022; Zillich et al., 2022). The 5mC mechanism involves the addition of a methyl group to the carbon 5 position of the nucleotide, which is catalyzed by DNA methyltransferases (DNMTs) (Gibney and Nolan, 2010). DNA hydroxymethylation (5hmC) occurs when this methyl group is removed through oxidation catalyzed by a family of ten-eleven translocase proteins (TET1, TET2, and TET3) during the DNA demethylation process. Recent work from our group and others has shown that 5hmC is functionally distinct from 5mC. This epigenetic mark is associated with transcriptional activation and highly prevalent in the brain (Rompala et al., 2022). Several studies have implicated 5hmC in anxiety-related behaviors (Papale et al., 2017), schizophrenia, bipolar disorder (Guidotti et al., 2014; Madrid et al., 2016), autism (Papale et al., 2015), and Alzheimer’s disease (Kuehner et al., 2021). Interestingly, a recent study evaluating 5mC and 5hmC in bulk tissue from the human postmortem brain identified a role for 5hmC in AUD (Clark et al., 2022).
Epigenetic patterns, such as 5mC and 5hmC, are tissue- and cell-type specific, and particularly 5hmC is highly enriched in the brain and abundant in neuronal cells, underscoring the need to investigate this epigenetic mark in brain tissue, particularly in neurons (Kriaucionis and Heintz, 2009; Szulwach et al., 2011; Mellén et al., 2012). However, most epigenetic studies have used bulk brain tissue, which can mask cell-type specific biological signals, highlighting the need for a cell-type-specific approach when evaluating the epigenetic landscape of AUD in the human brain.
The orbitofrontal cortex (OFC) has been implicated in decision making and motivated reward-related behavior (Morisot et al., 2019; Lohoff et al., 2021), and recent neuroimaging studies have associated alterations in this brain region with AUD (Shields and Gremel, 2020; Bracht et al., 2021; Atmaca et al., 2023). Individuals diagnosed with AUD exhibit a reduction in the OFC volume, accompanied by a decrease in gray matter, and an impact on dopaminergic pathways (Volkow et al., 2007; Coleman et al., 2011; Le Berre et al., 2014; Nimitvilai et al., 2017; Moorman, 2018; Morisot et al., 2019; Hernandez and Moorman, 2020). Recent 5mC studies from our group and others have revealed a role of epigenetic mechanisms in OFC in the context of substance use disorders (SUDs) (Kozlenkov et al., 2017; Rompala et al., 2022).
In this study, we examined neuronal-specific 5mC and 5hmC profiles in the OFC of AUD (n = 10) and non-AUD (n = 24) groups to identify epigenetically dysregulated genes and evaluate the differences between 5mC and 5hmC marks in the OFC. We also identify the functional pathways enriched by these epigenetically dysregulated genes, evaluate replication in an independent dataset, and assess its relationship with GWAS studies.
2 Materials and methods
2.1 Study cohort
Our study cohort comprised 34 postmortem brain samples obtained from the National posttraumatic stress disorder (PTSD) Brain Bank51 (NPBB) (Friedman et al., 2017), a brain tissue repository at the U.S. Department of Veterans Affairs (VA). Consisting of European American and African American men with a mean age of 41 (s.d ± 12) (Friedman et al., 2017). The tissue samples were collected after obtaining informed consent from the next-of-kin and processed as described by (Friedman et al., 2017). The clinical diagnosis followed the antemortem assessment protocol (AAP) and postmortem diagnostic assessment protocol (PAP) based on the DSM-IV criteria (Friedman et al., 2017). The samples were categorized into AUD and non-AUD groups. The AUD group included 10 donors with alcohol use disorder (AUD) history, which refers to those diagnosed with alcohol dependence or alcohol abuse. The non-AUD group included 24 donors without an AUD diagnosis. AUD and non-AUD groups were matched by (PTSD), opioid use disorder (OUD), and current smoking. Table 1 presents the demographic and clinical characteristics of the study cohort.
2.2 Neuronal nuclei isolation and DNA extraction
Neuronal nuclei isolation was performed using fluorescence-activated nuclei sorting (FANS), described by Rompala and Nagamatsu et al. (2022) (Rompala et al., 2022), obtaining 0.5–1 M NeuN + nuclei for DNA extraction. Sorted nuclei were centrifuged at 1,500 × g for 15 min at 4°C to obtain a pellet. Next, 500 μL and 50 µL proteinase K (Cat. #69504, Qiagen, Valencia, CA) and 20 mg/mL RNAse A (Cat. #12091021; Thermo-Fischer, Waltham, MA) were used to refloat the pellet. TheDNeasy Blood and Tissue Kit (Cat. #69504, Qiagen) manufacturer’s protocol was used to process the samples. Finally, eluted samples were concentrated to a final volume of 20 µL with the Zymo Genomic DNA Clean and Concentrator-10 kit (Cat. #D4010, Zymo Inc., Irving CA) and stored at −80°C.
2.3 High-throughput bisulfite sequencing and data processing
Sequencing data were obtained by reduced representation oxidative bisulfite-sequencing (RRoxBS), carried out at the Weill Cornell Epigenomics Core (New York, NY). The library preparations for 5mC and 5hmC were made using Mspl digestion for 400 ng of gDNA with the Ovation RRoxBS Methyl-Seq library preparation kit (TrueMethyl oxBS; Tecan, Switzerland). Bisulfite conversion was followed by a single-end 1 × 50 bp sequencing with the Illumina NovaSeq6000 system (mean depth of 42.7 ± 1.5 (µ ± SEM) million reads per library). The hg38 genome reference was used for adapter trimming, alignment information, and mapping efficiency of the sequencing data using an in-house BiSeq pipeline (Garrett-Bakelman et al., 2015).
2.4 Differential methylation analysis
The MethylKit R package (Akalin et al., 2012) was used to conduct differential methylation (5mC) and hydroxymethylation (5hmC) analyses at CpGs. The samples were filtered using a read coverage above ×10 and under the 99.9th percentile. Normalization was performed using the median method, where the mean was used to calculate the scaling factor to reduce coverage bias in the statistical analysis. Differential 5mC and differential 5hmC analyses were performed using logistic regression with correction for overdispersion and chi-squared significance testing (Akalin et al., 2012). The covariates included in the model were ancestry, age of death, PTSD, OUD, smoking, and postmortem interval (PMI). The sliding linear model (SLIM) was used to fit the p-values to q-values (Wang et al., 2011). The significance level for differential 5mC and 5hmC was defined as q-value < 0.05 and a greater than 2% difference of 5mC and 5hmC between AUD and non-AUD groups. Genome-wide significant (GWS) sites were used for annotation. We used the Genomation R package (Akalin et al., 2015) to annotate CpGs in promoters, introns, exons, and intragenic regions as well as CpG island, CpG islands shores, CpG shelves, and open sea (Akalin et al., 2015). Gene name annotation was performed using Ensembldb (Rainer et al., 2019).
Differentially methylated regions (DMR) and differentially hydroxymethylated regions (DhMR) with a window of 1,000 bases and at least 10 CpG sites covering the region were also examined for both 5mC and 5hmC epigenetic marks, respectively. Significant DMRs and DhMR were defined based on a q value < 0.05.
2.5 Functional enrichment analysis
Genomation R package was used to perform genomic feature annotation (Akalin et al., 2015). Functional enrichment analysis was made using the gene annotation obtained by scan_region.pl perl tool and the UCSC genome browser annotation databases. Genes with a distance greater than 1,500 bp were not considered for the enrichment analysis (Kuhn, Haussler and James Kent, 2013). To conduct functional enrichment analysis we used Metascape (Zhou et al., 2019), Enrichr (Kuleshov et al., 2016), and WebGestalt (Liao et al., 2019), which integrates databases such as NCA TS BioPlanet (Huang et al., 2019), Panther (Mi et al., 2013), Gene Ontology Consortium (Gene Ontology Consortium, 2015), and the Kyoto Encyclopedia of Genes and Genomes (KEGG) (Kanehisa and Goto, 2000). As the enriched terms across all databases may share relationships or exhibit redundancy, we also performed enrichment analysis networks using Metascape to visualize the interaction among the enriched terms (biological pathways). For this, we calculate the Kappa-test score of the enriched terms and hierarchically cluster them into non-redundant groups. This process involves selecting the most significant terms of each cluster and determining connections among them, guided by a Kappa similarity threshold above 0.3. Each node is color-coded for distinction based on the biological pathway they belong to. Protein–protein interaction (PPI) enrichment analysis was also conducted to examine interaction across genes mapping significant 5mC/5hmC CpG sites. The molecular code detection (MCODE) algorithm was used to cluster enrichment ontology terms to identify neighborhoods where proteins are densely connected in the following databases: STRING, BioGrid, and OmniPath (Türei et al., 2016; Oughtred et al., 2019; Szklarczyk et al., 2023).
2.6 GWAS enrichment analysis
The software Multi-marker Analysis of GenoMic Annotation (MAGMA) v1.10 (de Leeuw et al., 2015) was used to conduct gene-level association analysis for 5mC and 5hmC using the GWAS summary statistics of alcohol use disorder (AUD) (Zhou et al., 2020), problematic alcohol use (PAU) (Zhou et al., 2020), cannabis use disorder (CUD) (Johnson et al., 2020), opioid use disorder (OUD) (Polimanti et al., 2020), (PTSD) (Nievergelt et al., 2019), anxiety (Otowa et al., 2016), anorexia nervosa (Anorexia Nervosa Genetics Initiative et al., 2019), bipolar disorder (Mullins et al., 2021), and schizophrenia (Lam et al., 2019). The analysis was based on genetic variants in the 1000 Genomes Project dataset available on the MAGMA website (g1000_eur.bim). Gene annotation for the analysis was performed using the MAGMA NCBI37.3. gene.loc file.
A gene-level overlap between Clark et al. (2022) (Clark et al., 2022) reported genes (p < 1 × 10−5) and our significant findings was assessed. For this, we employed the liftOver tool to convert genome coordinates from hg37 to hg38. Fisher’s exact test was applied using the GeneOverlap 1.38.0 R package (Li Shen and Shenli, 2017) to evaluate whether the gene-level overlap is statistically significant.
3 Results
3.1 AUD-associated 5mC and 5hmC differential CpG sites
After QC, 1908163 and 1707251 CpG sites were included for 5mC and 5hmC differential analyses, respectively. For 5mC, we identified 417 CpG sites after multiple testing corrections with a difference in the percentage of methylation between AUD and non-AUD groups higher than 2. Of these, 137 were hypomethylated and 280 were hypermethylated (Figure 1). For 5hmC, we identified 363 CpG sites after multiple testing corrections, with 213 hypo- and 150 hyper-methylated CpG sites (Figure 2). Tables 2, 3 list the top GWS differential 5mC and 5hmC CpG sites. The 5mC GWS annotation showed that 59% of them were located at the promoter region, 21% at the intragenic region, 14% at introns, and 6% at exons. For the 5hmC GWS, 66% were located at the promoter region, 17% at the intragenic region, 11% at introns, and 6% at exons.
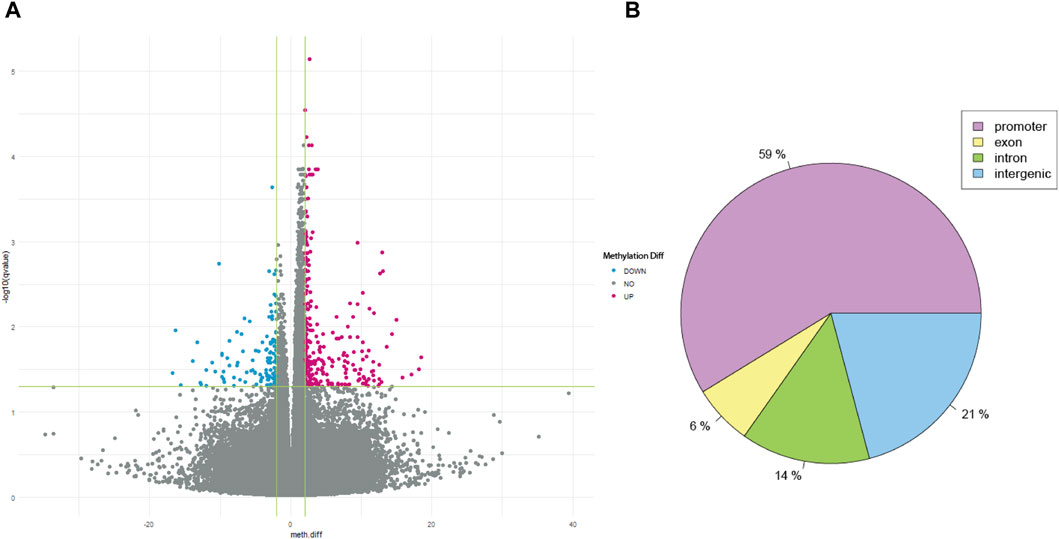
Figure 1. 5mC differential CpG sites associated with AUD. (A) Volcano plot shows the 5mC differential CpG sites associated with AUD. (B) Pie chart depicts the gene location of the GWS CpG sites identified.
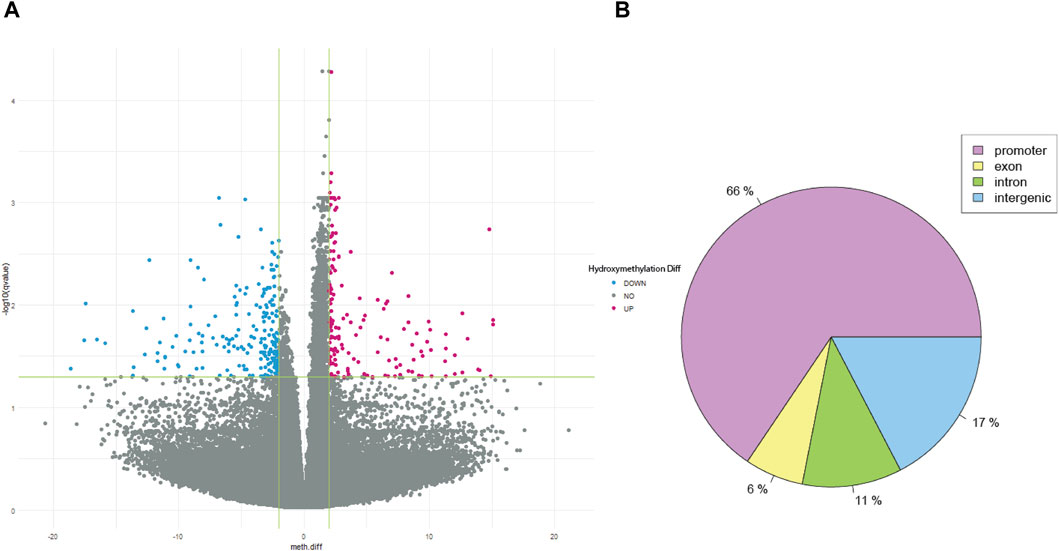
Figure 2. 5hmC differential CpG sites associated with AUD. (A) Volcano plot shows the 5hmC differential CpG sites associated with AUD. (B) Pie chart depicts the gene location of the GWS CpG sites identified.
3.2 Q-Q plot
The quantile–quantile (QQ) plots (Supplementary Figure S1) for the 5mC and 5hmC differential analyses are shown. The lambda values were λ = 1.21 for 5mC and λ = 1.46 for 5hmC. Regarding the DMR and DhMR analyses, lambda values were λ = 0.91 and λ = 1.00, respectively.
3.3 5mC and 5hmC-enriched pathways
For 5mC, we found significant enrichment (using 0bp annotation) for 30 pathways after multiple testing correction (Supplementary Figure S2; Supplementary Table S4). Top-level gene ontology (GO) pathways enriched included cellular process (GO:0009987), developmental process (GO:0032502), regulation of biological process (GO:0050789). The most significantly enriched pathways were cell-cell adhesion (GO:0098609), homophilic cell adhesion via plasma membrane adhesion molecules (GO:0007156), cell-cell adhesion via plasma-membrane adhesion molecules (GO:0098742), positive regulation of nervous system development (GO:0051962), regionalization (GO:0003002), and anterior/posterior pattern specification (GO:0009952). The enrichment of 5mC using the 1500bp annotation included 66 pathways (Supplementary Figure S3; Supplementary Table S5), with top-level GO pathways including cellular process (GO:0009987), developmental process (GO:0032502), and biological regulation (GO:0065007). The most significant enriched pathways were cell-cell adhesion (GO:0098609), homophilic cell adhesion via plasma membrane adhesion molecules (GO:0007156), cell-cell adhesion via plasma-membrane adhesion molecules (GO:0098742), anterior/posterior pattern specification (GO:0009952), regionalization (GO:0003002), pattern specification process (GO:0007389), and regulation of nervous system development (GO:0051960).
For 5hmC, enrichment using the 0bp annotation was found for 260 pathways (Supplementary Figure S4; Supplementary Table S6), including the top-level GO pathways of cellular process (GO:0009987), developmental process (GO:0032502), regulation of biological process (GO:0050789). After multiple testing correction, 158 pathways remained significant, including homophilic cell adhesion via plasma membrane adhesion molecules (GO:0007156), cell-cell adhesion via plasma-membrane adhesion molecules (GO:0098742), pattern specification process (GO:0007389), and cell-cell adhesion (GO:0098609). The enrichment analysis using the 1500bp annotation identified 164 significant pathways (Supplementary Figure S5; Supplementary Table S7), including top-level GO pathways enriched for 5hmC of cellular process (GO:0009987), developmental process (GO:0032502), growth (GO:0040007). The most significant enriched pathways were homophilic cell adhesion via plasma membrane adhesion molecules (GO:0007156), cell-cell adhesion via plasma-membrane adhesion molecules (GO:0098742), pattern specification process (GO:0007389), regionalization (GO:0003002), and cell-cell adhesion (GO:0098609).
3.4 Protein-protein interaction analysis
The PPI network analysis of the differential 5mC genes showed as significant pathways cell-cell adhesion (GO:0098609), homophilic cell adhesion via plasma membrane adhesion molecules (GO:0007156), cell-cell adhesion via plasma-membrane adhesion molecules (GO:0098742). The MCODE cluster algorithm identified GO pathways related to neurogenesis, chromatin organization, and cell adhesion (Supplementary Figures S6, S7; Supplementary Tables S10, S11).
For the 5hmC marks, the significant pathways identified in the PPI network analysis were cell-cell adhesion homophilic cell adhesion via plasma membrane adhesion molecules (GO:0007156), embryonic organ development (GO:0048568), and cell–cell adhesion via plasma-membrane adhesion molecules (GO:0098742). The MCODE cluster algorithm identified GO pathways implicated in neurogenesis, cell adhesion, calcium ion transport, and Wnt signaling (Supplementary Figures S8, S9; Supplementary Tables S12, S13).
3.5 GWAS enrichment analysis
A significant enrichment was identified between genes with differential 5mC and GWAS signals of AUD (p = 0.0022) and PAU (p = 0.019) using the summary stats from Zhou et al., 2020. No significant enrichment was observed for 5mC in CUD (p = 0.96), OUD (p = 0.30), PTSD (p = 0.27), anxiety (p = 0.36), anorexia nervosa (p = 0.86), bipolar disorder (p = 0.69), and schizophrenia (p = 0.69). For 5hmC, no significant enrichment was observed for AUD (p = 0.11), PAU (p = 0.66), CUD (p = 0.75) OUD (p = 0.49), PTSD (p = 0.72), anxiety (p = 0.71), anorexia nervosa (p = 0.55), bipolar disorder (p = 0.11), or schizophrenia (p = 0.06) (Figure 3) (Supplementary Table S3).
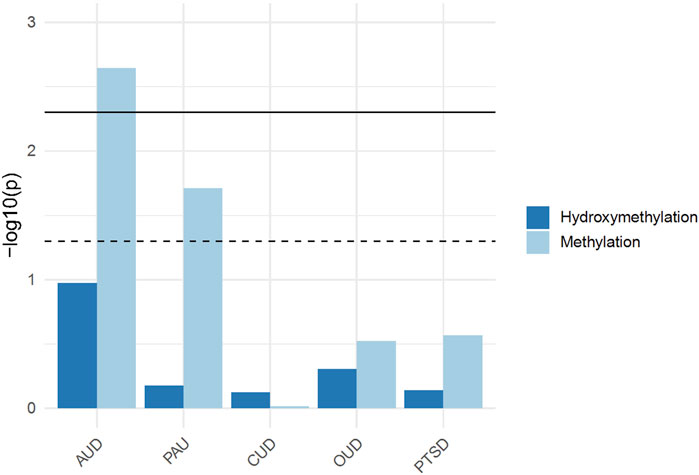
Figure 3. Generalized Gene-Set Analysis of GWAS for 5mC and 5hmC. The bar plot shows the gene-set analysis of GWAS for 5mC and 5hmC, including alcohol use disorder (AUD), problematic alcohol use (PAU), cannabis use disorder (CUD), opioid use disorder (OUD), and post-traumatic stress disorder (PTSD).
3.6 Gene overlap analysis
We compared our findings with those reported by (Clark et al., 2022), which evaluated AUD-associated 5mC and 5hmC in bulk tissue from the human postmortem PFC. For 5mC, we found an overlap of 14 genes with their reported 576 (Figure 4A; Supplementary Table S8). Fisher’s exact test showed that the overlap is significant (p = 8.5e-25, odds ratio = 124.00). Similarly, for 5hmC, we found a significant overlap of 14 genes with their reported 1,023 genes (Figure 4B; p = 3.9e-22, odds ratio = 79.80; Supplementary Table S9).
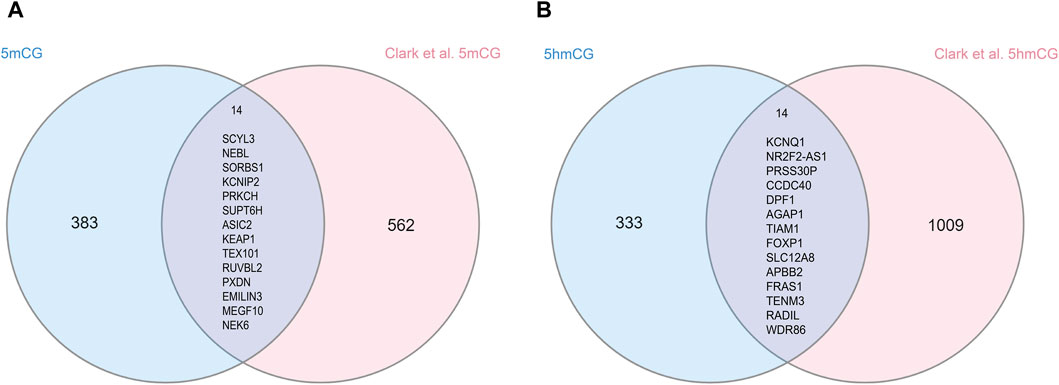
Figure 4. Overlapped differential 5mC and 5hmC genes in human brain tissue. (A) shows the overlap between our 5mC GWS hits and 5mC reported findings from Clark et al. (2022), where 14 genes overlap between both results. (B) shows the overlap between our 5hmC findings from OFC and 5hmC reported findings from Clark et al. (2022), where 14 genes overlap between both results.
3.7 Differentially methylated and hydroxymethylated regions (DMR/DhMR) analysis
After QC, a total of 38757 and 37316 regions were examined in the 5mC and 5hmC analyses, respectively. With a q value < 0.05, a total of 5 DMRs (Supplementary Table S14) and 35 DhMRs (Supplementary Table S15) were significantly associated with AUD. One of the DhMR maps to the TDP1 gene (chr14:89954001-89955000–hg38), a gene also observed with differential 5hmC and nominally associated with AUD in the (Clark et al., 2022).
4 Discussion
This study presents a neuronal-specific epigenomic investigation of AUD in the human brain. We profiled 5mC and 5hmC at the genome-wide scale and revealed differential CpG sites associated with AUD that map genes previously reported with this disorder. Our analysis identified 417 and 363 GWS AUD-associated CpG sites for 5mC (Supplementary Table S1) and 5hmC (Supplementary Table S2), respectively.
Our 5mC findings revealed genes related to AUD or other alcohol traits reported in previous studies. For example, SYK, this gene has been previously associated with alcohol metabolism in the liver and its inhibition is linked to reduced liver inflammation (Qu et al., 2018; Kurniawan et al., 2020). Furthermore, previous studies have found that binge drinking can induce SYK activation, and pharmacological inhibition of SYK significantly decreases alcoholic liver disease in a mouse model of binge drinking (Bukong et al., 2016).
Another differential 5mC gene with prior alcohol-related evidence is the DNMT3A, which encodes for an enzyme involved in de novo DNA methylation (Pino et al., 2017; Fischer et al., 2021). Ethanol exposure can induce a prolonged upregulation of DNMT3A in neuronal precursor cell lines and primary mouse embryonic fibroblasts (Miozzo et al., 2018). Similarly, Dnmt3a has been found upregulated in the nucleus accumbens of alcohol-preferring rats exposed to intermittent ethanol exposure (Niinep et al., 2021).
For 5hmC, one of the identified differential CpG sites previously linked to AUD mapped to GAD1, a gene that encodes for one of the glutamate decarboxylases that catalyze the conversion of glutamate to Gamma-aminobutyric acid (GABA). Chronic alcohol exposure has been linked to a decrease in GABA levels and an increase in GABA receptors (Sytinsky et al., 1975; Tran et al., 1981; Dodd et al., 1996; Behar et al., 1999). GAD1 has also been found to be upregulated in the dorsomedial thalamus of human subjects in individuals with AUD (Hade et al., 2021). However, a recent study did not find a difference in GAD1 mRNA levels in OFC between individuals with AUD and the non-alcoholic group AUD (Edenberg et al., 2010; Underwood et al., 2019). More research is needed to fully elucidate the role of these genes in AUD.
Additional AUD-associated CpG sites with differential 5hmC were those mapped to DLX1 and DLX2, which are also implicated GABA signaling, specifically in interneuron GABA synthesis (Le et al., 2017; Pla et al., 2018) and play a role in interneuron synaptogenesis and dendritogenesis (Pla et al., 2018). Moreover, these genes are suggested to directly promote the expression of Grin2b, a gene previously reported in human stem-cell-derived cortical neurons exposed to chronic alcohol consumption and also reported in the prefrontal cortex and hippocampus of mice treated with chronic alcohol consumption followed by withdrawal (Endele et al., 2010; Xiang et al., 2015; Pla et al., 2018; Myers et al., 2019). In the current study, both DLX1 and DLX2 showed hyper-5hmC and were located in the exon region. DLX2 CpG site was located in the promoter region, suggesting that it could be directly impacting gene expression regulation in individuals with AUD. The effect of 5hmC in the exon region is not well understood; however, in the case of 5mC, it is suggested that the density of 5mC in the exon can enhance gene expression (Li et al., 2018).
GATA4 was identified in both 5mC and 5hmC differential analyses and has been previously associated with alcohol dependence (Treutlein et al., 2009; Edenberg et al., 2010; Karpyak et al., 2014). GATA4 encodes the GATA-motif binding protein type 4, a transcription factor that controls the expression of proteins involved in drug metabolism (Karpyak et al., 2014). In addition, high doses of alcohol increase its expression (Zhong et al., 2010). In our study, this gene was hypomethylated and hypo hydroxymethylated (5mC location at 11697675bp, 5hmC location at 11703255). The differential 5mC CpG site was located in the intronic region, suggesting chromosomal instability. The 5hmC CpG sites were located in the promoter and intronic regions. The presence of 5hmC at the promoter region has been associated with protection of gene transcription in regions where 5mC is present (Ehrlich and Ehrlich, 2014). This suggests an interaction of these epigenetic mechanisms in the modulation of GATA4 expression in the OFC.
In addition to differential analysis at CpG sites, we also assessed differential 5mC and 5hmC regions. We found 5 GWS DMRs, but none of the genes mapping to these regions have been previously linked to AUD or related psychiatric traits. Among the GWS DhMR identified, chr1:65265001-65266000, mapped to intron 1 of DNAJC6, a gene highly expressed in the brain and involved in synaptic vesicle uncoating. When comparing the identified regions with the differential CpG analysis, we observed an overlap between DMR and differential 5mC CpGs with the OSBP2 gene. The EWAS catalog (www.ewascatalog.org) have reported CpG sites in this gene associated with chronic obstructive pulmonary disease and type 2 diabetes based on a blood-based EWAS in the Generation Scotland cohort (n = 18,413). For 5hmC, we found an overlap for RP11-44N22.3, but its functionality is unknown. Lastly, when comparing the identified regions with Clark et al., 2022, we observed an overlap with the LRRC1 and TDP1 gene. CpG sites at the TDP1 were also identified in the differential 5hmC analysis. This gene participates in DNA repair of neuronal cells and disruptions on this gene cause a neurodegenerative disorder known as spinocerebellar ataxia with axonal neuropathy. More research is needed to understand the role of these genes in AUD.
The findings of our enrichment analysis are consistent with previous AUD-related studies identifying development and neurogenesis. For instance, in a previous report examining fetal alcohol syndrome (FAS), these pathways were found to be highly significant (Fischer et al., 2021). Moreover, studies have also reported that AUD impacts cell adhesion and neurogenesis, which involves the development of new neurons and their integration into functional neural networks (Ramanathan, 1996; Arevalo et al., 2008; Pino et al., 2017; Poulose et al., 2017; Lees et al., 2020; Wooden et al., 2021). The effects on developmental processes and neurogenesis may contribute to the cognitive impairment reported in individuals with AUD and may also be linked to brain dysfunction in various regions, including the OFC (De Wilde et al., 2007; Arevalo et al., 2008).
GWAS enrichment analysis showed enrichment of differential 5mC genes with AUD and PAU GWASes (Zhou et al., 2020). No enrichment was identified with GWAS of other SUDs, suggesting a specificity of our differential 5mC marks with alcohol-related traits. When comparing our findings with those reported by Clark et al. (2022), we observed 14 overlapping genes for each epigenetic mark, 5mC and 5hmC. Several overlapping genes with differential 5hmC have been previously linked to SUDs. For instance, KCNQ1, a gene encoding a potassium ion channel, was identified in a GWAS of alcohol dependence (Edenberg et al., 2010; Feng et al., 2022). Similarly, APBB2 has been associated with opioid and amphetamine dependence (Gelernter et al., 2014; Liu et al., 2018). Among the 5mC overlapping genes, ASIC2 has been linked to addiction-related behavior in mice (Kreple et al., 2014). These findings underscore the importance of evaluating both 5mC and 5hmC to fully investigate the role of epigenetic mechanisms on AUD, at least in the brain where 5hmC is highly prevalent and enriched in neurons. Future studies should explore these genes in greater depth to better understand their involvement in AUD.
The limitations of this study are that the donors of the study cohort present heterogeneous comorbidities. All individuals in the OUD group were also diagnosed with PTSD. We controlled this by using PTSD as a covariate in the differential 5mC and 5hmC analyses. In addition, we conducted a GWAS enrichment analysis of our 5mC and 5hmC annotated genes to determine whether the reported genes were enriched for the comorbidity traits, including PTSD and other SUDs. In addition, the cohort size is limited; however, it is comparable to other recently published postmortem brain studies. Another limitation is that all samples are male, limiting to identify the effect of sex in our results. The analyses were only carried out on CpGs sites and it would be important to conduct a study on non-CpGs sites, because of the role of 5mC at non-CpGs on neuropsychiatric diseases from our group and others (Jang et al., 2017; Nagamatsu et al., 2022). Further, since the technologies to assess 5mC and 5hmC in our study and Clark et al., 2022 are different, we were only able to evaluate the convergence between the two studies at the gene-level, not at the CpG level, which limits the interpretation in gene regulatory processes of the differential epigenetic marks identified. To determine if the epigenetic marks in the reported genes of this study are a cause of AUD or an effect, research in model organisms is warranted.
Our study characterized the methylome and hydroxymethylome profiles of AUD in neurons from the human OFC. Our results replicate previous findings in certain genes and highlight new findings for both 5mC and 5hmC. This study reveals new insights into the epigenomic dysregulation of AUD in the human brain and pinpoints potential drug targets for the treatment of individuals suffering from AUD.
Data availability statement
The original contributions presented in the study are included in the article/Supplementary Material, further inquiries can be directed to the corresponding author.
Ethics statement
The studies involving humans were approved by the Yale University Institutional Review Board and the IRB Committee from the Department of Veteran’s Affairs. The studies were conducted in accordance with the local legislation and institutional requirements. Written informed consent was obtained from the participants or the participants’ legal guardians/next of kin.
Author contributions
DA-B: Data curation, Formal Analysis, Investigation, Methodology, Writing–original draft, Writing–review and editing. DN-R: Data curation, Formal Analysis, Methodology, Writing–review and editing. JM-M: Formal Analysis, Writing–review and editing. SN: Writing–review and editing. GR: Data curation, Methodology, Writing–review and editing. LZ: Formal Analysis, Writing–review and editing. SW: Writing–review and editing. SC: Writing–review and editing. ML: Writing–review and editing. JM-O: Conceptualization, Funding acquisition, Methodology, Resources, Supervision, Writing–review and editing.
Members of the Traumatic Stress Brain Research Group
Victor E. Alvarez, David Benedek, Alicia Che, Dianne A. Cruz, David A. Davis, Matthew J. Girgenti, Ellen Hoffman, Paul E. Holtzheimer, Bertrand R. Huber, Alfred Kaye, Terence M. Keane, John H. Krystal, Adam T. Labadorf, Mark W. Logue, Brian Marx, Deborah Mash, Ann McKee, Mark W. Miller, Janitza Montalvo-Ortiz, Crystal Noller, Paula Schnurr, William K. Scott, Thor Stein, Robert Ursano, Douglas E. Williamson, Erika J. Wolf, Keith A. Young.
Funding
The author(s) declare that financial support was received for the research, authorship, and/or publication of this article. This work is supported by the U.S. Department of Veterans Affairs via 1IK2CX002095-01A1 (JM-O) and the National Center of Posttraumatic Stress Disorder, NIDA R21DA050160 (JM-O), and the Kavli Institute for Neuroscience at Yale University Kavli Postdoctoral Award for Academic Diversity (JM-M).
Acknowledgments
We want to thank the donors and their families for making this research possible.
Conflict of interest
The authors declare that the research was conducted in the absence of any commercial or financial relationships that could be construed as a potential conflict of interest.
Publisher’s note
All claims expressed in this article are solely those of the authors and do not necessarily represent those of their affiliated organizations, or those of the publisher, the editors and the reviewers. Any product that may be evaluated in this article, or claim that may be made by its manufacturer, is not guaranteed or endorsed by the publisher.
Supplementary material
The Supplementary Material for this article can be found online at: https://www.frontiersin.org/articles/10.3389/fgene.2024.1345410/full#supplementary-material
References
Akalin, A., Franke, V., Vlahoviček, K., Mason, C. E., and Schübeler, D. (2015). Genomation: a toolkit to summarize, annotate and visualize genomic intervals. Bioinforma. Oxf. Engl. 31, 1127–1129. doi:10.1093/bioinformatics/btu775
Akalin, A., Kormaksson, M., Li, S., Garrett-Bakelman, F. E., Figueroa, M. E., Melnick, A., et al. (2012). methylKit: a comprehensive R package for the analysis of genome-wide DNA methylation profiles. Genome Biol. 13, R87. doi:10.1186/gb-2012-13-10-r87
Anorexia Nervosa Genetics Initiative Watson, H. J., Yilmaz, Z., Thornton, L. M., Hübel, C., Gaspar, H. A., et al. (2019). Genome-wide association study identifies eight risk loci and implicates metabo-psychiatric origins for anorexia nervosa. Nat. Genet. 51, 1207–1214. doi:10.1038/s41588-019-0439-2
Arevalo, E., Shanmugasundararaj, S., Wilkemeyer, M. F., Dou, X., Chen, S., Charness, M. E., et al. (2008). An alcohol binding site on the neural cell adhesion molecule L1. Proc. Natl. Acad. Sci. U.S.A. 105, 371–375. doi:10.1073/pnas.0707815105
Atmaca, M., Tabara, M. F., Koc, M., Gurok, M. G., Baykara, S., Korkmaz, S., et al. (2023). Cortical thickness of the orbitofrontal cortex in patients with alcohol use disorder. Brain Sci. 13, 552. doi:10.3390/brainsci13040552
Behar, K. L., Rothman, D. L., Petersen, K. F., Hooten, M., Delaney, R., Petroff, O. A., et al. (1999). Preliminary evidence of low cortical GABA levels in localized 1H-MR spectra of alcohol-dependent and hepatic encephalopathy patients. Am. J. Psychiatry 156, 952–954. doi:10.1176/ajp.156.6.952
Bracht, T., Soravia, L., Moggi, F., Stein, M., Grieder, M., Federspiel, A., et al. (2021). The role of the orbitofrontal cortex and the nucleus accumbens for craving in alcohol use disorder. Transl. Psychiatry 11, 267. doi:10.1038/s41398-021-01384-w
Bukong, T. N., Iracheta-Vellve, A., Gyongyosi, B., Ambade, A., Catalano, D., Kodys, K., et al. (2016). Therapeutic benefits of spleen tyrosine kinase inhibitor administration on binge drinking-induced alcoholic liver injury, steatosis, and inflammation in mice. Alcohol Clin. Exp. Res. 40, 1524–1530. doi:10.1111/acer.13096
Clark, S. L., Chan, R. F., Zhao, M., Xie, L. Y., Copeland, W. E., Penninx, B. W. J. H., et al. (2022). Dual methylation and hydroxymethylation study of alcohol use disorder. Addict. Biol. 27, e13114. doi:10.1111/adb.13114
Coleman, L. G., He, J., Lee, J., Styner, M., and Crews, F. T. (2011). Adolescent binge drinking alters adult brain neurotransmitter gene expression, behavior, brain regional volumes, and neurochemistry in mice. Alcohol. Clin. Exp. Res. 35, 671–688. doi:10.1111/j.1530-0277.2010.01385.x
de Leeuw, C. A., Mooij, J. M., Heskes, T., and Posthuma, D. (2015). MAGMA: generalized gene-set analysis of GWAS data. PLoS Comput. Biol. 11, e1004219. doi:10.1371/journal.pcbi.1004219
De Wilde, B., Dom, G., Hulstijn, W., and Sabbe, B. (2007). Motor functioning and alcohol dependence. Alcohol. Clin. Exp. Res. 31, 1820–1825. doi:10.1111/j.1530-0277.2007.00484.x
Dodd, P. R., Kril, J. J., Thomas, G. J., Watson, W. E., Johnston, G. A., and Harper, C. G. (1996). Receptor binding sites and uptake activities mediating GABA neurotransmission in chronic alcoholics with Wernicke encephalopathy. Brain Res. 710, 215–228. doi:10.1016/0006-8993(95)01399-7
Edenberg, H. J., Koller, D. L., Xuei, X., Wetherill, L., McClintick, J. N., Almasy, L., et al. (2010). Genome-wide association study of alcohol dependence implicates a region on chromosome 11. Alcohol. Clin. Exp. Res. 34, 840–852. doi:10.1111/j.1530-0277.2010.01156.x
Ehrlich, M., and Ehrlich, K. C. (2014). DNA cytosine methylation and hydroxymethylation at the borders. Epigenomics 6, 563–566. doi:10.2217/epi.14.48
Endele, S., Rosenberger, G., Geider, K., Popp, B., Tamer, C., Stefanova, I., et al. (2010). Mutations in GRIN2A and GRIN2B encoding regulatory subunits of NMDA receptors cause variable neurodevelopmental phenotypes. Nat. Genet. 42, 1021–1026. doi:10.1038/ng.677
Feng, Y.-C. A., Stanaway, I. B., Connolly, J. J., Denny, J. C., Luo, Y., Weng, C., et al. (2022). Psychiatric manifestations of rare variation in medically actionable genes: a PheWAS approach. BMC Genomics 23, 385. doi:10.1186/s12864-022-08600-x
Fischer, M., Chander, P., Kang, H., Mellios, N., and Weick, J. P. (2021). Transcriptomic changes due to early, chronic intermittent alcohol exposure during forebrain development implicate WNT signaling, cell-type specification, and cortical regionalization as primary determinants of fetal alcohol syndrome. Alcohol. Clin. Exp. Res. 45, 979–995. doi:10.1111/acer.14590
Friedman, M. J., Huber, B. R., Brady, C. B., Ursano, R. J., Benedek, D. M., Kowall, N. W., et al. (2017). VA’s national PTSD Brain Bank: a national resource for research. Curr. Psychiatry Rep. 19, 73. doi:10.1007/s11920-017-0822-6
Garrett-Bakelman, F. E., Sheridan, C. K., Kacmarczyk, T. J., Ishii, J., Betel, D., Alonso, A., et al. (2015). Enhanced reduced representation bisulfite sequencing for assessment of DNA methylation at base pair resolution. J. Vis. Exp. JoVE, e52246. doi:10.3791/52246
GBD 2019 Risk Factors Collaborators (2020). Global burden of 87 risk factors in 204 countries and territories, 1990-2019: a systematic analysis for the Global Burden of Disease Study 2019. Lancet lond. Engl. 396, 1223–1249. doi:10.1016/S0140-6736(20)30752-2
Gelernter, J., Kranzler, H. R., Sherva, R., Koesterer, R., Almasy, L., Zhao, H., et al. (2014). Genome-wide association study of opioid dependence: multiple associations mapped to calcium and potassium pathways. Biol. Psychiatry 76, 66–74. doi:10.1016/j.biopsych.2013.08.034
Gelernter, J., Sun, N., Polimanti, R., Pietrzak, R. H., Levey, D. F., Lu, Q., et al. (2019). Genome-wide association study of maximum habitual alcohol intake in >140,000 U.S. European and african American veterans yields novel risk loci. Biol. Psychiatry 86, 365–376. doi:10.1016/j.biopsych.2019.03.984
Gene Ontology Consortium (2015). Gene ontology Consortium: going forward. Nucleic Acids Res. 43, D1049–D1056. doi:10.1093/nar/gku1179
Gibney, E. R., and Nolan, C. M. (2010). Epigenetics and gene expression. Heredity 105, 4–13. doi:10.1038/hdy.2010.54
Guidotti, A., Dong, E., Tueting, P., and Grayson, D. R. (2014). Modeling the molecular epigenetic profile of psychosis in prenatally stressed mice. Prog. Mol. Biol. Transl. Sci. 128, 89–101. doi:10.1016/B978-0-12-800977-2.00004-8
Hade, A.-C., Philips, M.-A., Reimann, E., Jagomäe, T., Eskla, K.-L., Traks, T., et al. (2021). Chronic alcohol use induces molecular genetic changes in the dorsomedial thalamus of people with alcohol-related disorders. Brain Sci. 11, 435. doi:10.3390/brainsci11040435
Hernandez, J. S., and Moorman, D. E. (2020). Orbitofrontal cortex encodes preference for alcohol. eNeuro 7. doi:10.1523/ENEURO.0402-19.2020
Huang, R., Grishagin, I., Wang, Y., Zhao, T., Greene, J., Obenauer, J. C., et al. (2019). The NCATS BioPlanet - an integrated platform for exploring the universe of cellular signaling pathways for toxicology, systems biology, and chemical genomics. Front. Pharmacol. 10, 445. doi:10.3389/fphar.2019.00445
Jang, H. S., Shin, W. J., Lee, J. E., and Do, J. T. (2017). CpG and non-CpG methylation in epigenetic gene regulation and brain function. Genes (Basel) 8, 148. doi:10.3390/genes8060148
Johnson, E. C., Demontis, D., Thorgeirsson, T. E., Walters, R. K., Polimanti, R., Hatoum, A. S., et al. (2020). A large-scale genome-wide association study meta-analysis of cannabis use disorder. Lancet Psychiatry 7, 1032–1045. doi:10.1016/S2215-0366(20)30339-4
Kanehisa, M., and Goto, S. (2000). KEGG: kyoto encyclopedia of genes and genomes. Nucleic Acids Res. 28, 27–30. doi:10.1093/nar/28.1.27
Karpyak, V. M., Winham, S. J., Biernacka, J. M., Cunningham, J. M., Lewis, K. A., Geske, J. R., et al. (2014). Association of GATA4 sequence variation with alcohol dependence. Addict. Biol. 19, 312–315. doi:10.1111/j.1369-1600.2012.00482.x
Kozlenkov, A., Jaffe, A. E., Timashpolsky, A., Apontes, P., Rudchenko, S., Barbu, M., et al. (2017). DNA methylation profiling of human prefrontal cortex neurons in heroin users shows significant difference between genomic contexts of hyper- and hypomethylation and a younger epigenetic age. Genes 8, 152. doi:10.3390/genes8060152
Kreple, C. J., Lu, Y., Taugher, R. J., Schwager-Gutman, A. L., Du, J., Stump, M., et al. (2014). Acid-sensing ion channels contribute to synaptic transmission and inhibit cocaine-evoked plasticity. Nat. Neurosci. 17, 1083–1091. doi:10.1038/nn.3750
Kriaucionis, S., and Heintz, N. (2009). The nuclear DNA base 5-hydroxymethylcytosine is present in Purkinje neurons and the brain. Science 324, 929–930. doi:10.1126/science.1169786
Kuehner, J. N., Chen, J., Bruggeman, E. C., Wang, F., Li, Y., Xu, C., et al. (2021). 5-hydroxymethylcytosine is dynamically regulated during forebrain organoid development and aberrantly altered in Alzheimer’s disease. Cell Rep. 35, 109042. doi:10.1016/j.celrep.2021.109042
Kuhn, R. M., Haussler, D., and Kent, W. J. (2013). The UCSC genome browser and associated tools. Brief. Bioinform 14, 144–161. doi:10.1093/bib/bbs038
Kuleshov, M. V., Jones, M. R., Rouillard, A. D., Fernandez, N. F., Duan, Q., Wang, Z., et al. (2016). Enrichr: a comprehensive gene set enrichment analysis web server 2016 update. Nucleic Acids Res. 44, W90–W97. doi:10.1093/nar/gkw377
Kurniawan, D. W., Storm, G., Prakash, J., and Bansal, R. (2020). Role of spleen tyrosine kinase in liver diseases. World J. Gastroenterol. 26, 1005–1019. doi:10.3748/wjg.v26.i10.1005
Lam, M., Chen, C.-Y., Li, Z., Martin, A. R., Bryois, J., Ma, X., et al. (2019). Comparative genetic architectures of schizophrenia in East Asian and European populations. Nat. Genet. 51, 1670–1678. doi:10.1038/s41588-019-0512-x
Le, T. N., Zhou, Q.-P., Cobos, I., Zhang, S., Zagozewski, J., Japoni, S., et al. (2017). GABAergic interneuron differentiation in the basal forebrain is mediated through direct regulation of glutamic acid decarboxylase isoforms by dlx homeobox transcription factors. J. Neurosci. Off. J. Soc. Neurosci. 37, 8816–8829. doi:10.1523/JNEUROSCI.2125-16.2017
Le Berre, A.-P., Rauchs, G., La Joie, R., Mézenge, F., Boudehent, C., Vabret, F., et al. (2014). Impaired decision-making and brain shrinkage in alcoholism. Eur. Psychiatry J. Assoc. Eur. Psychiatr. 29, 125–133. doi:10.1016/j.eurpsy.2012.10.002
Lees, B., Meredith, L. R., Kirkland, A. E., Bryant, B. E., and Squeglia, L. M. (2020). Effect of alcohol use on the adolescent brain and,behavior. Pharmacol. Biochem. Behav. 192, 172906. doi:10.1016/j.pbb.2020.172906
Li, S., Zhang, J., Huang, S., and He, X. (2018). Genome-wide analysis reveals that exon methylation facilitates its selective usage in the human transcriptome. Brief. Bioinform. 19, 754–764. doi:10.1093/bib/bbx019
Liao, Y., Wang, J., Jaehnig, E. J., Shi, Z., and Zhang, B. (2019). WebGestalt 2019: gene set analysis toolkit with revamped UIs and APIs. Nucleic Acids Res. 47, W199–W205. doi:10.1093/nar/gkz401
Li Shen, M. S., and Shenli, S. C. (2017). GeneOverlap: test and visualize gene overlaps. doi:10.18129/B9.BIOC.GENEOVERLAP
Liu, C.-C., Fang, C.-P., Liu, T.-H., Kuo, H.-W., Liu, S. C., Wang, S.-C., et al. (2018). APBB2 is associated with amphetamine use and plasma beta-amyloids in patients receiving methadone maintenance treatment. Prog. Neuropsychopharmacol. Biol. Psychiatry 83, 92–98. doi:10.1016/j.pnpbp.2018.01.008
Lohoff, F. W., Roy, A., Jung, J., Longley, M., Rosoff, D. B., Luo, A., et al. (2021). Epigenome-wide association study and multi-tissue replication of individuals with alcohol use disorder: evidence for abnormal glucocorticoid signaling pathway gene regulation. Mol. Psychiatry 26, 2224–2237. doi:10.1038/s41380-020-0734-4
Longley, M. J., Lee, J., Jung, J., and Lohoff, F. W. (2021). Epigenetics of alcohol use disorder-A review of recent advances in DNA methylation profiling. Addict. Biol. 26, e13006. doi:10.1111/adb.13006
Madrid, A., Papale, L. A., and Alisch, R. S. (2016). New hope: the emerging role of 5-hydroxymethylcytosine in mental health and disease. Epigenomics 8, 981–991. doi:10.2217/epi-2016-0020
Mellén, M., Ayata, P., Dewell, S., Kriaucionis, S., and Heintz, N. (2012). MeCP2 binds to 5hmC enriched within active genes and accessible chromatin in the nervous system. Cell 151, 1417–1430. doi:10.1016/j.cell.2012.11.022
Mi, H., Muruganujan, A., Casagrande, J. T., and Thomas, P. D. (2013). Large-scale gene function analysis with the PANTHER classification system. Nat. Protoc. 8, 1551–1566. doi:10.1038/nprot.2013.092
Miozzo, F., Arnould, H., de Thonel, A., Schang, A.-L., Sabéran-Djoneidi, D., Baudry, A., et al. (2018). Alcohol exposure promotes DNA methyltransferase DNMT3A upregulation through reactive oxygen species-dependent mechanisms. Cell Stress Chaperones 23, 115–126. doi:10.1007/s12192-017-0829-2
Montalvo-Ortiz, J. L., Gelernter, J., Cheng, Z., Girgenti, M. J., Xu, K., Zhang, X., et al. (2022). Epigenome-wide association study of posttraumatic stress disorder identifies novel loci in U.S. military veterans. Transl. Psychiatry 12, 65. doi:10.1038/s41398-022-01822-3
Moorman, D. E. (2018). The role of the orbitofrontal cortex in alcohol use, abuse, and dependence. Prog. Neuropsychopharmacol. Biol. Psychiatry 87, 85–107. doi:10.1016/j.pnpbp.2018.01.010
Morisot, N., Phamluong, K., Ehinger, Y., Berger, A. L., Moffat, J. J., and Ron, D. (2019). mTORC1 in the orbitofrontal cortex promotes habitual alcohol seeking. eLife 8, e51333. doi:10.7554/eLife.51333
Mullins, N., Forstner, A. J., O’Connell, K. S., Coombes, B., Coleman, J. R. I., Qiao, Z., et al. (2021). Genome-wide association study of more than 40,000 bipolar disorder cases provides new insights into the underlying biology. Nat. Genet. 53, 817–829. doi:10.1038/s41588-021-00857-4
Myers, S. J., Yuan, H., Kang, J.-Q., Tan, F. C. K., Traynelis, S. F., and Low, C.-M. (2019). Distinct roles of GRIN2A and GRIN2B variants in neurological conditions. F1000Research 8, F1000. doi:10.12688/f1000research.18949.1
Nagamatsu, S. T., Rompala, G., Hurd, Y. L., Núñez-Rios, D. L., and Montalvo-Ortiz, J. L.Traumatic Stress Brain Research Group (2022). CpH methylome analysis in human cortical neurons identifies novel gene pathways and drug targets for opioid use disorder. Front. Psychiatry 13, 1078894. doi:10.3389/fpsyt.2022.1078894
Nievergelt, C. M., Maihofer, A. X., Klengel, T., Atkinson, E. G., Chen, C.-Y., Choi, K. W., et al. (2019). International meta-analysis of PTSD genome-wide association studies identifies sex- and ancestry-specific genetic risk loci. Nat. Commun. 10, 4558. doi:10.1038/s41467-019-12576-w
Niinep, K., Anier, K., Eteläinen, T., Piepponen, P., and Kalda, A. (2021). Repeated ethanol exposure alters DNA methylation status and dynorphin/kappa-opioid receptor expression in nucleus accumbens of alcohol-preferring AA rats. Front. Genet. 12, 750142. doi:10.3389/fgene.2021.750142
Nimitvilai, S., Lopez, M. F., Mulholland, P. J., and Woodward, J. J. (2017). Ethanol dependence abolishes monoamine and GIRK (Kir3) channel inhibition of orbitofrontal cortex excitability. Neuropsychopharmacol. Off. Publ. Am. Coll. Neuropsychopharmacol. 42, 1800–1812. doi:10.1038/npp.2017.22
Otowa, T., Hek, K., Lee, M., Byrne, E. M., Mirza, S. S., Nivard, M. G., et al. (2016). Meta-analysis of genome-wide association studies of anxiety disorders. Mol. Psychiatry 21, 1485–1399. doi:10.1038/mp.2016.11
Oughtred, R., Stark, C., Breitkreutz, B.-J., Rust, J., Boucher, L., Chang, C., et al. (2019). The BioGRID interaction database: 2019 update. Nucleic Acids Res. 47, D529–D541. doi:10.1093/nar/gky1079
Papale, L. A., Madrid, A., Li, S., and Alisch, R. S. (2017). Early-life stress links 5-hydroxymethylcytosine to anxiety-related behaviors. Epigenetics 12, 264–276. doi:10.1080/15592294.2017.1285986
Papale, L. A., Zhang, Q., Li, S., Chen, K., Keleş, S., and Alisch, R. S. (2015). Genome-wide disruption of 5-hydroxymethylcytosine in a mouse model of autism. Hum. Mol. Genet. 24, 7121–7131. doi:10.1093/hmg/ddv411
Pino, A., Fumagalli, G., Bifari, F., and Decimo, I. (2017). New neurons in adult brain: distribution, molecular mechanisms and therapies. Biochem. Pharmacol. 141, 4–22. doi:10.1016/j.bcp.2017.07.003
Pla, R., Stanco, A., Howard, M. A., Rubin, A. N., Vogt, D., Mortimer, N., et al. (2018). Dlx1 and Dlx2 promote interneuron GABA synthesis, synaptogenesis, and dendritogenesis. Cereb. Cortex N. Y. N. 1991 28, 3797–3815. doi:10.1093/cercor/bhx241
Polimanti, R., Walters, R. K., Johnson, E. C., McClintick, J. N., Adkins, A. E., Adkins, D. E., et al. (2020). Leveraging genome-wide data to investigate differences between opioid use vs. opioid dependence in 41,176 individuals from the Psychiatric Genomics Consortium. Mol. Psychiatry 25, 1673–1687. doi:10.1038/s41380-020-0677-9
Poulose, S. M., Miller, M. G., Scott, T., and Shukitt-Hale, B. (2017). Nutritional factors affecting adult neurogenesis and cognitive function. Adv. Nutr. Bethesda Md 8, 804–811. doi:10.3945/an.117.016261
Qu, C., Zheng, D., Li, S., Liu, Y., Lidofsky, A., Holmes, J. A., et al. (2018). Tyrosine kinase SYK is a potential therapeutic target for liver fibrosis. Hepatol. Balt. Md 68, 1125–1139. doi:10.1002/hep.29881
Rainer, J., Gatto, L., and Weichenberger, C. X. (2019). ensembldb: an R package to create and use Ensembl-based annotation resources. Bioinformatics 35, 3151–3153. doi:10.1093/bioinformatics/btz031
Ramanathan, R., Wilkemeyer, M. F., Mittal, B., Perides, G., and Charness, M. E. (1996). Alcohol inhibits cell-cell adhesion mediated by human L1 [published erratum appears in J Cell Biol 1996 Jun;133(5):1139-40]. J. Cell Biol. 133, 381–390. doi:10.1083/jcb.133.2.381
Rompala, G., Nagamatsu, S. T., Martínez-Magaña, J. J., Wang, J., Girgenti, M. J., Krystal, J. H., et al. (2022). Profiling neuronal methylome and hydroxymethylome of opioid use disorder in the human orbitofrontal cortex. Psychiatry Clin. Psychol. doi:10.1101/2022.09.09.22279769
Shields, C. N., and Gremel, C. M. (2020). Review of orbitofrontal cortex in alcohol dependence: a disrupted cognitive map? Alcohol. Clin Exp Res 44, 1952–1964. doi:10.1111/acer.14441
Sytinsky, I. A., Guzikov, B. M., Gomanko, M. V., Eremin, V. P., and Konovalova, N. N. (1975). The gamma-aminobutyric acid (GABA) system in brain during acute and chronic ethanol intoxication. J. Neurochem. 25, 43–48. doi:10.1111/j.1471-4159.1975.tb07691.x
Szklarczyk, D., Kirsch, R., Koutrouli, M., Nastou, K., Mehryary, F., Hachilif, R., et al. (2023). The STRING database in 2023: protein-protein association networks and functional enrichment analyses for any sequenced genome of interest. Nucleic Acids Res. 51, D638–D646. doi:10.1093/nar/gkac1000
Szulwach, K. E., Li, X., Li, Y., Song, C.-X., Wu, H., Dai, Q., et al. (2011). 5-hmC–mediated epigenetic dynamics during postnatal neurodevelopment and aging. Nat. Neurosci. 14, 1607–1616. doi:10.1038/nn.2959
Tran, V. T., Snyder, S. H., Major, L. F., and Hawley, R. J. (1981). GABA receptors are increased in brains of alcoholics. Ann. Neurol. 9, 289–292. doi:10.1002/ana.410090312
Treutlein, J., Cichon, S., Ridinger, M., Wodarz, N., Soyka, M., Zill, P., et al. (2009). Genome-wide association study of alcohol dependence. Arch. Gen. Psychiatry 66, 773–784. doi:10.1001/archgenpsychiatry.2009.83
Türei, D., Korcsmáros, T., and Saez-Rodriguez, J. (2016). OmniPath: guidelines and gateway for literature-curated signaling pathway resources. Nat. Methods 13, 966–967. doi:10.1038/nmeth.4077
Underwood, M. D., Bakalian, M. J., Dwork, A. J., Min, E., Mann, J. J., and Arango, V. (2019). GAD mRNA in orbital prefrontal cortex and anterior cingulate cortex in alcoholics compared with nonpsychiatric controls: a negative postmortem study. J. Psychiatry Brain Sci. 4, e190007. doi:10.20900/jpbs.20190007
Volkow, N. D., Wang, G.-J., Telang, F., Fowler, J. S., Logan, J., Jayne, M., et al. (2007). Profound decreases in dopamine release in striatum in detoxified alcoholics: possible orbitofrontal involvement. J. Neurosci. 27, 12700–12706. doi:10.1523/JNEUROSCI.3371-07.2007
Wang, H.-Q., Tuominen, L. K., and Tsai, C.-J. (2011). SLIM: a sliding linear model for estimating the proportion of true null hypotheses in datasets with dependence structures. Bioinformatics 27, 225–231. doi:10.1093/bioinformatics/btq650
Wooden, J. I., Thompson, K. R., Guerin, S. P., Nawarawong, N. N., and Nixon, K. (2021). Consequences of adolescent alcohol use on adult hippocampal neurogenesis and hippocampal integrity. Int. Rev. Neurobiol. 160, 281–304. doi:10.1016/bs.irn.2021.08.005
Xiang, Y., Kim, K.-Y., Gelernter, J., Park, I.-H., and Zhang, H. (2015). Ethanol upregulates NMDA receptor subunit gene expression in human embryonic stem cell-derived cortical neurons. PLOS ONE 10, e0134907. doi:10.1371/journal.pone.0134907
Zhong, L., Zhu, J., Lv, T., Chen, G., Sun, H., Yang, X., et al. (2010). Ethanol and its metabolites induce histone lysine 9 acetylation and an alteration of the expression of heart development-related genes in cardiac progenitor cells. Cardiovasc. Toxicol. 10, 268–274. doi:10.1007/s12012-010-9081-z
Zhou, H., Kalayasiri, R., Sun, Y., Nuñez, Y. Z., Deng, H.-W., Chen, X.-D., et al. (2021). Genome-wide meta-analysis of alcohol use disorder in east asians. Addict. Med. doi:10.1101/2021.09.17.21263732
Zhou, H., Kember, R. L., Deak, J. D., Xu, H., Toikumo, S., Yuan, K., et al. (2023). Multi-ancestry study of the genetics of problematic alcohol use in >1 million individuals. Psychiatry Clin. Psychol., 2023.01.24.23284960. doi:10.1101/2023.01.24.23284960
Zhou, H., Sealock, J. M., Sanchez-Roige, S., Clarke, T.-K., Levey, D. F., Cheng, Z., et al. (2020). Genome-wide meta-analysis of problematic alcohol use in 435,563 individuals yields insights into biology and relationships with other traits. Nat. Neurosci. 23, 809–818. doi:10.1038/s41593-020-0643-5
Zhou, Y., Zhou, B., Pache, L., Chang, M., Khodabakhshi, A. H., Tanaseichuk, O., et al. (2019). Metascape provides a biologist-oriented resource for the analysis of systems-level datasets. Nat. Commun. 10, 1523. doi:10.1038/s41467-019-09234-6
Keywords: methylation, hydroxymethylation, epigenetics, alcohol use disorder, postmortem brain
Citation: Andrade-Brito DE, Núñez-Ríos DL, Martínez-Magaña JJ, Nagamatsu ST, Rompala G, Zillich L, Witt SH, Clark SL, Lattig MC and Montalvo-Ortiz JL (2024) Neuronal-specific methylome and hydroxymethylome analysis reveal significant loci associated with alcohol use disorder. Front. Genet. 15:1345410. doi: 10.3389/fgene.2024.1345410
Received: 27 November 2023; Accepted: 14 March 2024;
Published: 03 April 2024.
Edited by:
Lea Davis, Vanderbilt University Medical Center, United StatesReviewed by:
Biju Viswanath, National Institute of Mental Health and Neurosciences (NIMHANS), IndiaMark Z. Kos, The University of Texas Rio Grande Valley, United States
Copyright © 2024 Andrade-Brito, Núñez-Ríos, Martínez-Magaña, Nagamatsu, Rompala, Zillich, Witt, Clark, Lattig and Montalvo-Ortiz. This is an open-access article distributed under the terms of the Creative Commons Attribution License (CC BY). The use, distribution or reproduction in other forums is permitted, provided the original author(s) and the copyright owner(s) are credited and that the original publication in this journal is cited, in accordance with accepted academic practice. No use, distribution or reproduction is permitted which does not comply with these terms.
*Correspondence: Janitza L. Montalvo-Ortiz, janitza.montalvo-ortiz@yale.edu