- 1School of Computer Science and School of Cyberspace Science, Xiangtan University, Xiangtan, China
- 2Hunan Institute of Engineering College of textile and clothing, Xiangtan, China
In recent years, many excellent computational models have emerged in microbe-drug association prediction, but their performance still has room for improvement. This paper proposed the OGNNMDA framework, which applied an ordered message-passing mechanism to distinguish the different neighbor information in each message propagation layer, and it achieved a better embedding ability through deeper network layers. Firstly, the method calculates four similarity matrices based on microbe functional similarity, drug chemical structure similarity, and their respective Gaussian interaction profile kernel similarity. After integrating these similarity matrices, it concatenates the integrated similarity matrix with the known association matrix to obtain the microbe-drug heterogeneous matrix. Secondly, it uses a multi-layer ordered message-passing graph neural network encoder to encode the heterogeneous network and the known association information adjacency matrix, thereby obtaining the final embedding features of the microbe-drugs. Finally, it inputs the embedding features into the bilinear decoder to get the final prediction results. The OGNNMDA method performed comparative experiments, ablation experiments, and case studies on the aBiofilm, MDAD and DrugVirus datasets using 5-fold cross-validation. The experimental results showed that OGNNMDA showed the strongest prediction performance on aBiofilm and MDAD and obtained sub-optimal results on DrugVirus. In addition, the case studies on well-known drugs and microbes also support the effectiveness of the OGNNMDA method. Source codes and data are available at: https://github.com/yyzg/OGNNMDA.
1 Introduction
The human microbiome consists of trillions of microbes that reside inside and outside the human body, and these microbes play an essential role in maintaining the overall health of the human body (Ogunrinola et al., 2020). The host-microbe plays a crucial role in several physiological processes in the human body, such as energy collection and storage (Amato et al., 2019), facilitating carbohydrate absorption, and protecting the body from foreign microorganisms and pathogens (Hajiagha et al., 2022). Moreover, the changes in microbiota composition can significantly affect human health Kim et al. (2018); Partula et al. (2019); Catinean et al. (2018). Many studies have shown that the dysbiosis or unbalance of microbiota is closely related to disease, and the microbiota is an important causative factor for many diseases. Therefore, microbes are considered new therapeutic targets for precision medicine (Cullin et al., 2021), and the research on the relationship between microbes and drugs not only aids in drug development but also the diagnosis and treatment of human diseases. However, the popularization and widespread use of antibiotics in modern medicine have led to the emergence of an increasing number of drug-resistant microbes, which seriously threaten human health (Pugazhendhi et al., 2020). Although many researchers have provided extensive evidence on the association between microbes and drugs, traditional biomedical experiments are time-consuming, labor-intensive, and costly (Paul et al., 2010). These reasons hinder the efficiency of drug development and hardly satisfy the massive demands for novel drugs. Therefore, it is necessary to explore the microbe-drug associations at a large-scale level for drug development.
To overcome the above challenges, computational models have emerged as an effective method for identifying microbe-drug associations, and these models are used to predict microbe-drug associations by integrating different genomic information, including genomics, macro genomics, and metabolomics. With the rapid development of high-throughput sequencing technology and advanced genomics techniques, the research on microbe-drug association prediction has developed rapidly, generating a large amount of valuable research data. To further investigate the potential association between microbes and drugs, a series of microbe-drug association databases have been constructed in recent years, such as aBiofilm (Rajput et al., 2018), MDAD (Sun et al., 2018) and DrugVirus (Andersen et al., 2020), which have immensely promoted the development of microbe-drug association prediction models. Over the past few years, many computational models have emerged that utilize the above databases to infer potential associations between microbes and drugs. As an illustration, Zhu et al. proposed a computational method, HMDAKATZ, which applied the KATZ measure to predict inherent associations between microbes and drugs (Zhu et al., 2019b). Long et al. (2020) proposed a computational method called GCNMDA, which combined graph convolutional networks (GCNs) and conditional random fields (CRFs) with an attentional mechanism aiming to identify the hidden associations between microbes and drugs. In 2021, GATMDA was proposed, which utilized inductive matrix completion and graph attention networks (GNNs) to predict associations between microbes and diseases (Long et al., 2021). The Graph2MDA model combined the constructed multimodal attribute graphs and variational graph autoencoder (VGAE) to predict microbe-drug associations accurately (Deng et al., 2022). GSAMDA is likewise a microbe-drug association prediction model, which primarily applies graph attention networks (GATs) and sparse autoencoders (Tan et al., 2022). The computational model NIRBMMDA (Cheng et al., 2022) combines neighborhood-based inference (NI) and restricted Boltzmann machine (RBM) methodologies to predict Microbe-Drug Associations (MDA). By leveraging NI, it extracts proximity information from microbes or drugs, while RBM is used to learn the latent probability distribution inherent in the known association data. This integrative approach harnesses the strengths of both components, resulting in a more robust predictive framework. In the study of Tian et al. (2023), they proposed the SCSMDA model, which was based on GCN and integrated structure-enhanced contrast learning and self-paced negative sampling strategies to improve the accuracy in microbe-drug association prediction. In addition, the GACNNMDA model integrated a GTA-based autoencoder and a CNN-based classifier, which transforms multiple attribute combinations of the microbes and drugs into two feature matrices to predict the associations of the microbes and drugs (Ma et al., 2023). Qu et al. (2023) proposed MHBVDA to predicts virus-drug associations by integrating multiple biological data sources and employing integrating two matrix decomposition-based methods. And it innovatively applies Bounded Nuclear Norm Regularization (BNNR) with regularization terms to mitigate the impact of noisy data and overfitting issues, thereby enhancing prediction accuracy. However, these methods based on graph neural networks still have room for improvement in prediction performance. When multi-layer networks are stacked, there is some confusion between different orders of neighborhood information, the node representations become indistinguishable, and the network performance decreases, which tends to prevent GNN with multiple layers from effectively utilizing the higher-order neighborhood information (Li et al., 2018).
Therefore, to achieve better prediction performance, inspired by the work of Song et al. (2023), this paper proposed an ordered gating mechanism-based ordered message-passing GNN method to infer potential microbe-drug associations, called OGNNMDA. In OGNNMDA, the known association data are preprocessed to compute Gaussian interaction profile kernel similarity and additional biomedical information similarity (microbe functional similarity, drug structural similarity) for drugs and microbes, respectively. Then, the multiple similarity matrices are fused and stitched together to obtain the heterogeneous networks. The heterogeneous network was fed into the encoder consisting of the two-layer fully connected network and the 12-layer ordered message-passing GNN to derive embedding representations of the drugs and microbes, respectively. Finally, the bilinear decoder was adopted to reconstruct the microbe-drug association matrix to infer possible associations between the microbes and drugs. Furthermore, to evaluate the predictive performance of OGNNMDA, in-depth comparative experiments, ablation experiments, and case studies are conducted in this paper. The results demonstrate that OGNNMDA outperforms current representative existing methods and achieves satisfactory results in potential drug-microbe association prediction.
2 Datasets
All the aBiofilm, MDAD and DrugVirus datasets provide important insights into the complex interactions between the drugs and the microbes, providing researchers in the fields of bioinformatics and graphical neural networks with a wealth of information to analyze and utilize to advance their studies and methods. The basic statistical information of the three datasets is presented in Table 1.
2.1 aBiofilm
In 2018, Rajput et al. introduced the aBiofilm (http://bioinfo.imtech.res.in/manojk/abiofilm/) dataset, which is of great significance for the development of the bioinformatics and graph neural network fields (Rajput et al., 2018). Over the last three decades, many anti-biofilm agents have been experimentally verified to disrupt biofilms. aBiofilm organizes these data, which contain a database, a predictor, and a data visualization module. The database contains biological, chemical, and structural details of 5,027 anti-biofilm agents (1720 different ones) reported from 1988 to 2017. After eliminating redundant associations among them, a total of 2,884 known interaction associations of 1720 drugs and 140 microbes were finally obtained.
2.2 MDAD
MDAD (https://github.com/Sun-Yazhou/MDAD/) is also a valuable microbe-drug association dataset, which was proposed by Sun et al. based on a variety of drug-related databases as well as a large amount of literature (Sun et al., 2018). Specifically, MDAD contains 5,505 associations between 180 microbes and 1,388 drugs collected from 993 documentation. After filtering out redundant information, a total of 2,470 microbe-drug associations were obtained, involving 173 microbes and 1,373 drugs.
2.3 DrugVirus
DrugVirus (https://drugvirus.info/) compiles interactions involving 118 virus-targeting drugs and 83 human viruses, encompassing SARS-CoV-2 (2019-nCoV) (Andersen et al., 2020). Building upon this foundation, Lond et al. systematically extracted and curated 57 drug-virus associations from pertinent drug databases and scholarly publications, which involved 76 unique drugs and 12 distinct viruses. Ultimately, they assembled a dataset comprising 175 drugs and 95 viruses, yielding a total of 933 documented drug-virus interaction records.
3 Preprocessing
In this section, firstly, the definition of the association adjacency matrix is given, secondly, the similarity calculation of drugs and microbe based on the adjacency matrix is given, and finally, the heterogeneous network is obtained based on multiple similarities.
For simplicity, for each dataset, let
That is, for any given
3.1 Constructing drug-drug similarity networks
First, considering that the functions of drugs are determined by their microstructures, and drugs with similar structures have similar chemical properties. So, the SIMCOMP2 tool based on the maximum common substructure between drugs is used in this paper to calculate the drug structure similarity (Hattori et al., 2010). For two drugs di and dj respectively, their structure-based similarity can be expressed as DSS(di, dj). After calculating all the similarities between all drug pairs, an Nd × Nd matrix
Next, for any two given drugs or microbes, the Gaussian interaction profile kernel similarity between them is calculated herein by utilizing a Gaussian kernel function based on known microbe disease associations as shown in Eq. 2:
where A (i, :) and A (j, :) denote the ith and jth rows of the adjacency matrix A, respectively, and γd denotes the drug-normalized kernel bandwidth, which can be calculated by Eq. 3.
3.2 Constructing microbe-microbe similarity networks
Also, this paper measures microbe similarity in two ways. The first one is the functional similarity of microbe proposed by Kamneva (2017). This computational method is mainly based on the microbial gene family information kernel protein-protein interaction association network. The second similarity between microbes is the Gaussian interaction profile kernel similarity MGS. similar to the drug similarity based on the Gaussian interaction profile kernel, for any given microbe pair mi and mj, it is computed using the Gaussian kernel function based on the known microbe drug associations as shown in Eq. 4.
where A (:, i) and A (:, j) denote the ith and jth columns of the adjacency matrix A, respectively, and γm denotes the microbe normalized kernel bandwidth that can be computed according to Eq. 5.
3.3 Constructing the heterogeneous network
Considering that not all drugs have their structures retrieved from databases, it is not possible to obtain all chemical structure similarities between drugs lacking structural information and other drugs. Therefore, in this paper, a comprehensive similarity is constructed to estimate the similarity between drugs and microbes by integrating Gaussian interaction profile nuclear similarity, microbe functional similarity, and drug chemical structure similarity. Specifically, for any two given drugs di and dj, the integrated similarity between them is calculated as shown in Eq. 6:
In addition, for any given microbe pair mi and mj, the combined similarity between them is calculated as shown in Eq. 7:
Then, the heterogeneous network
Next, the model uses above newly constructed heterogeneous network H as an input to the GNN-based encoder to learn the low dimensional embedding representations of the drugs and microbes.
4 Methods
Figure 1 illustrates the framework of OGNNMDA, comprising three primary modules: the input module, encoder module, and decoder module. The input module is responsible for extracting multiple biomedical information features to be utilized as inputs for OGNNMDA. The encoder module focuses on learning the node embedding representation of the microbes and drugs. Lastly, the decoder module employs bilinear decoders to predict new drug-microbe associations.
4.1 Encoder
OGNNMDA is a graph neural network that directly processes the graph as input, effectively utilizing both node information and structural characteristics. Graph neural networks have gained significant popularity in link prediction tasks (Zhang and Chen, 2018), showcasing their widespread adoption. By leveraging the adjacency matrix H obtained earlier, Eq. 9 defines the specific formulation of the GNN.
Here,
The function ϕ calculates the messages transmitted between nodes, where the edge attribute is directly used as the message. The symbol □ represents the message aggregation function, and in this paper, the mean method is employed (Huan et al., 2021). This means that messages received from multiple neighboring nodes are aggregated by taking their average, resulting in message characteristics used for updating node representations. Finally, γ represents the node representation update function, which implements the ordered message-passing mechanism discussed in this paper.
In the message-passing process of a single-level GNN, a node only exchanges messages with its immediate neighbors. This pattern of neighbor message transmission at different orders aligns with the structure of the node root tree in a multi-layer GNN (Liu et al., 2020). As illustrated in Figure 2, for a node v,
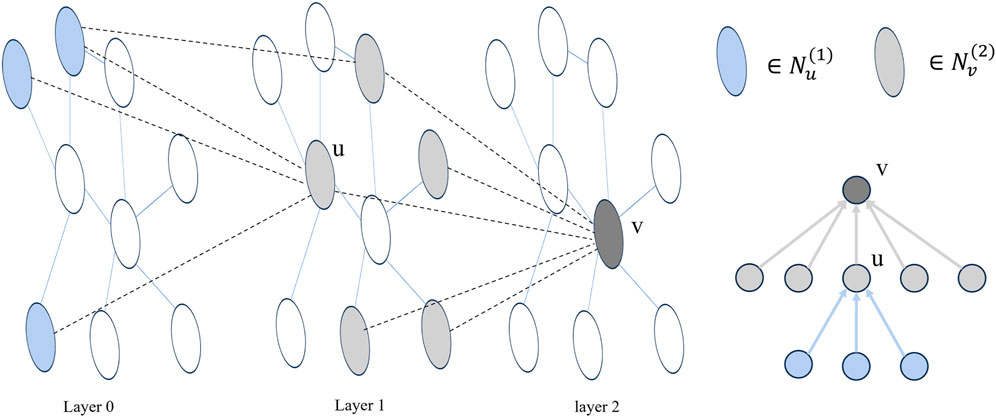
Figure 2. Taking a two-layer GNN as an example, layer 0 represents the initial node embedding, and the adjacency of nodes between layers forms multiple trees. In the figure, u is a neighbor node of v.
In single-layer message passing, direct-neighbor node messages and higher-order neighbor node messages are differentially encoded to ensure orderly message delivery. Specifically, the neuron rows are aligned with the node root tree at each layer, enabling the acquisition of node feature representations with consistent nesting relationships. To implement this alignment encoding method, the neurons can be ordered by linearly arranging the neurons of each layer and considering a segmentation point, denoted as s. The information of the neighbors of the current node v, at order one or higher, can be encoded as
Next, we describe the node feature update function γ, which is exemplified below for a specific node v. The function can be divided into three distinct steps.
1. Compute the aggregated message representation
2. For node v, this paper utilizes the gating vector
In Eq. 15, the trainable parameters
3. Equation 17 demonstrates the utilization of the gating vector
In Eq. 17, the symbol ⋅ represents element-by-element multiplication, and LN refers to the layer normalization operation (Chen et al., 2022).
4.2 Decoder
After the previous rounds of the ordered message passing process, the final node embedding representation
To reconstruct the adjacency matrix A′ representing possible microbe-disease associations, the bilinear decoder is employed. It is a structural component employed for predicting the probability of potential edges or links based on node embedding vectors. These decoders commonly integrate the embedding vectors of node pairs within a graph to generate a score function that assesses the likelihood of a link between two nodes. The key characteristic of bilinear decoders lies in their utilization of bilinear transformations to capture the interaction effects among nodes. Specifically, for a drug node and microbe node pair (u, v) with their respective embedding vectors hd(u) and hm(v), a bilinear decoder might compute the score by Eq. 19.
Where W is a learnable weight matrix. This score can be interpreted as the probability of link occurrence after a nonlinear activation function transformation, so that A′ can be obtained by the bilinear decoder as shown in Eq. 20.
In the above formula, where
Algorithm 1.OGNNMDA.
Require: Known associations matrix
Ensure: The constructed drug-microbe associations matrix
1: Construct the heterogeneous network H according to formula (8)
2: Initialize the embedding feature matrix Hinit according to formula (11).
3: Initialize the gate vector = 0
4: for i = 1 → α do
5: calculate h0 according to formula (10)
6: for l = 1 → Lconv do
7: calculate message matrix
8: calculate
9: calculate
10: calculate
11: end for
12: get the embedding feature for drugs and microbes with hd and hm according to formula (18)
13: get the reconstruction matrix A′ by formula (20)
14: end for
4.3 Optimization
During the experiment, positive samples were the drug-microbe pairs with known associations, while negative samples were the drug-microbe pairs without known associations. These sets of positive and negative samples are denoted as Ω+ and Ω−, respectively, for ease of description. It is important to note that the number of pairs with known associations in both the aBiofilm dataset and the MDAD dataset is significantly smaller than the number of pairs without known associations. Therefore, when training OGNNMDA, the loss function incorporates a weighted cross-entropy loss, as defined in Eq. 21.
In the above formula, (i, j) represents a pair of the drug di and microbe mj. λ is introduced as a balancing factor, calculated as the ratio of the number of samples in Ω− to the number of samples in Ω+. This factor helps attenuate the impact of data imbalance and emphasizes the reinforcement of known correlation information.
In this paper, the Xavier initialization method (Duong et al., 2019) is employed to initialize the trainable parameter matrices in various components of the model. These include the 2-layer fully connected layer, the ordered message-passing graph neural network layer, the bilinear decoder, and others, denoted as
To prevent overfitting, the paper introduces node dropout (Piotrowski et al., 2020) and regularized dropout (Berg et al., 2017) schemes in the graph convolution layer. Node dropout can be seen as training multiple models on various sub-nodes, and the combination of these sub-nodes is used to predict unknown microbe-drug pairs (Tan et al., 2020).
5 Results
This paper begins by providing a brief overview of the experimental setup and the analysis and selection of certain hyperparameters. The aim is to validate the predictive performance advantages of OGNNMDA through intensive comparison experiments. These experiments involve 6 representative microbe-drug association prediction models, including state-of-the-art approaches. The evaluation is conducted on three well-known public datasets, namely, aBiofilm, MDAD and DrugVirus, within a 5-fold cross-validation framework. Furthermore, ablation experiments are performed to investigate the effectiveness of the ordered message-passing mechanism employed in OGNNMDA. Finally, a case study is presented to validate OGNNMDA using two commonly used drugs, ciprofloxacin and moxifloxacin, along with two common oral microbes, Actinobacillus aggregatum and Clostridium nucleatum.
5.1 Experimental parameter setting
In this paper, all experimental evaluations are conducted within a five-fold cross-validation setup. To ensure statistical robustness, each method is executed ten independent times for every experiment, thereby enabling the calculation of the mean value for each performance metric across these repetitions. In detail, this involves dividing all known associations in the dataset equally into 5 parts, denoted as
During the i − th (1 ≤ i ≤ 5) cross-validation iteration, the training set is defined as
Based on the previous description of the model structure, OGNNMDA incorporates several hyperparameters, including the dimension size (k) of embedded features, the number of fully-connected layers (Lfc), the number of ordered message-passing GNN layers (Lconv), the initial learning rate (r) of Adam’s optimizer, the total training period (α), the node dropout metrics (β), and the regularized dropout parameter (γ).
To establish initial values for these parameters, we set Lfc = 2, r = 0.008, α = 600, β = 0.6, and γ = 0.4. Subsequently, we examine the effects of different values for parameters k and Lconv through experimental analysis.
To investigate the impact of different hyperparameter values on the model, this paper performed 5-fold cross-validation (5 cv) experiments on the aBiofilm and MDAD datasets. The results for the AUROC were plotted in Figure 3, showcasing the outcomes for various combinations of the parameters Lconv and k.
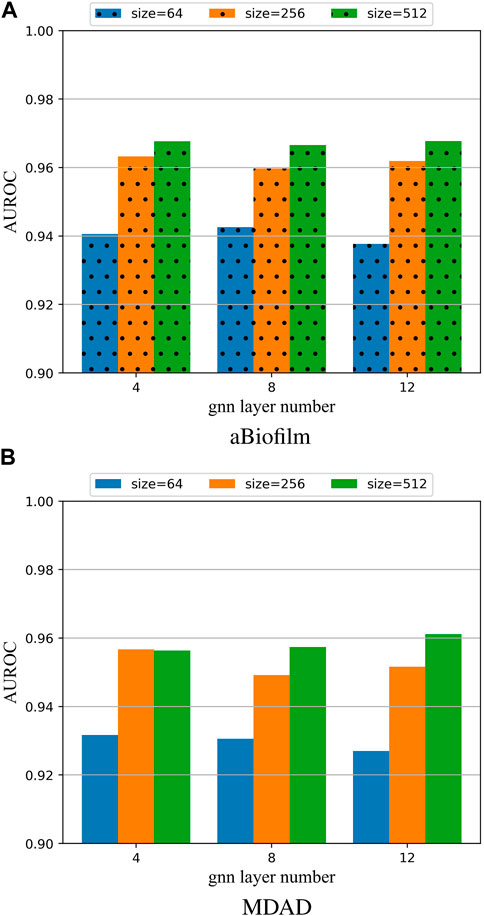
Figure 3. (A) Model hyperparameter analysis on the aBiofilm dataset. (B) Model hyperparameter analysis on the MDAD dataset.
From Figures 3A, B, it is evident that the optimal combination of Lconv and k is Lconv = 12 and k = 512. Therefore, this parameter setting will be utilized for OGNNMDA in subsequent experiments.
5.2 Comparison experiments
In this study, we replicate the code and data based on publicly accessible resources of these six methodologies, with all competing methods’ parameter configurations set according to their optimal values as reported in their respective publications. The 6 methods we compared OGNNMDA with are HMDAKATZ (Zhu et al., 2019a), GCNNMDA (Long et al., 2020), GSAMDA (Tan et al., 2022), SCSMDA (Tian et al., 2023), LAGCN (Yu et al., 2021), and NTSHMDA (Luo and Long, 2018), which are widely used in linkage prediction problems across various bioinformatics domains. However, due to GSAMDA not having performed experiments on DrugVirus in their paper nor specifying the construction process for the microbe-disease associations and drug-disease associations used to derive disease-based microbial and drug-Hamming similarities, comparative evaluations on DrugVirus are limited to the remaining five competing approaches.
To train and evaluate these methods, a 5-fold cross-validation experimental framework was employed. Performance evaluation was based on metrics such as AUC, AUPR, accuracy, and F1 score, chosen for their effectiveness in assessing performance. The experimental results, including the performance metrics, are presented in Tables 2–4. Additionally, ROC curves (see Figure 4A, 5A, 6A) and PR curves (see Figure 4B, 5B, 6B) were plotted to facilitate comparison among the different methods on the respective datasets.
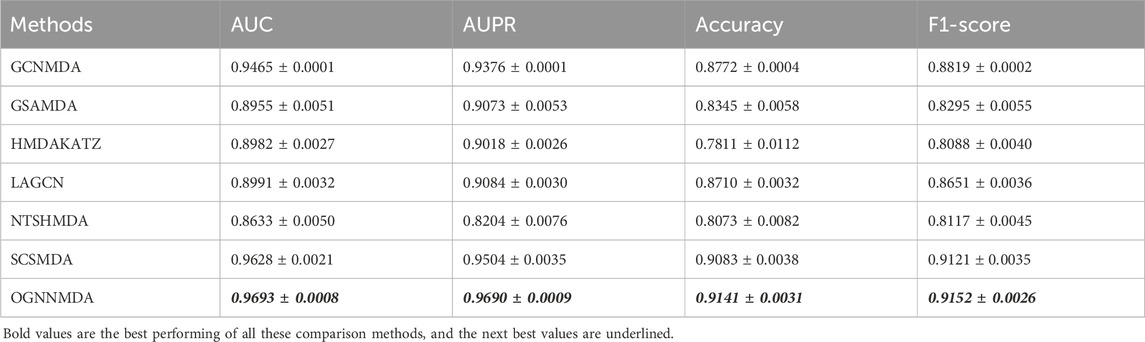
Table 2. Comparison of AUC, AUPR, Acc, and F1-score obtained by each method based on aBiofilm dataset at 5-cv.
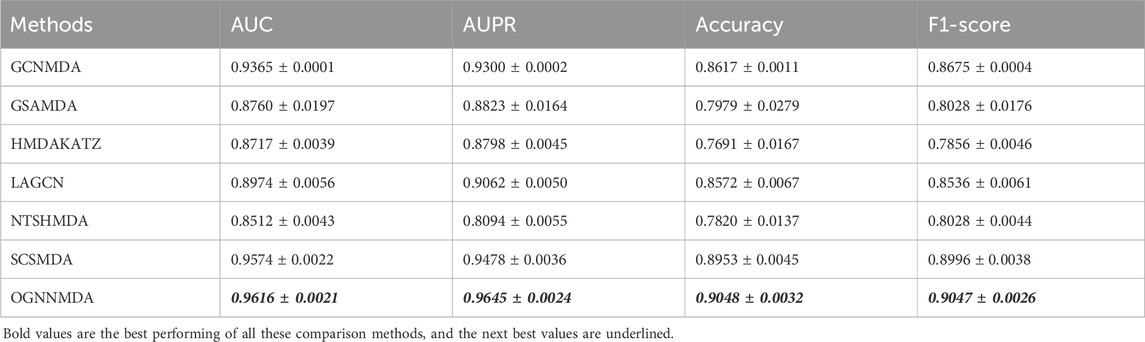
Table 3. Comparison of AUC, AUPR, Acc and F1-score obtained by each method based on MDAD dataset at 5-cv.
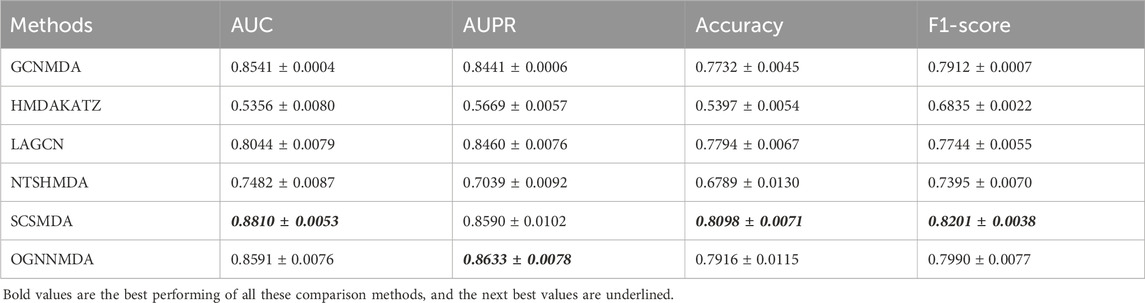
Table 4. Comparison of AUC, AUPR, Acc and F1-score obtained by each method based on DrugVirus dataset at 5-cv.
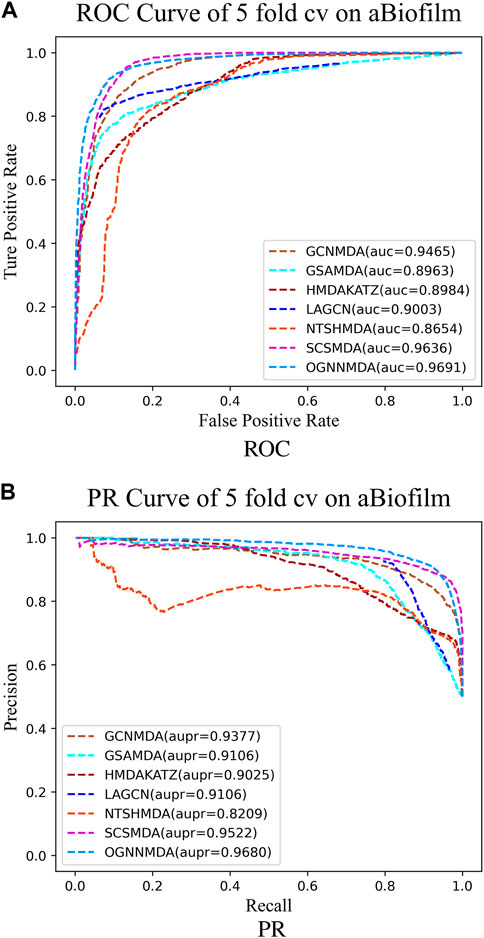
Figure 4. (A) ROC curves for each modeling approach based on the aBiofilm dataset 5-cv. (B) PR curves for each modeling approach based on the aBiofilm dataset 5-cv.
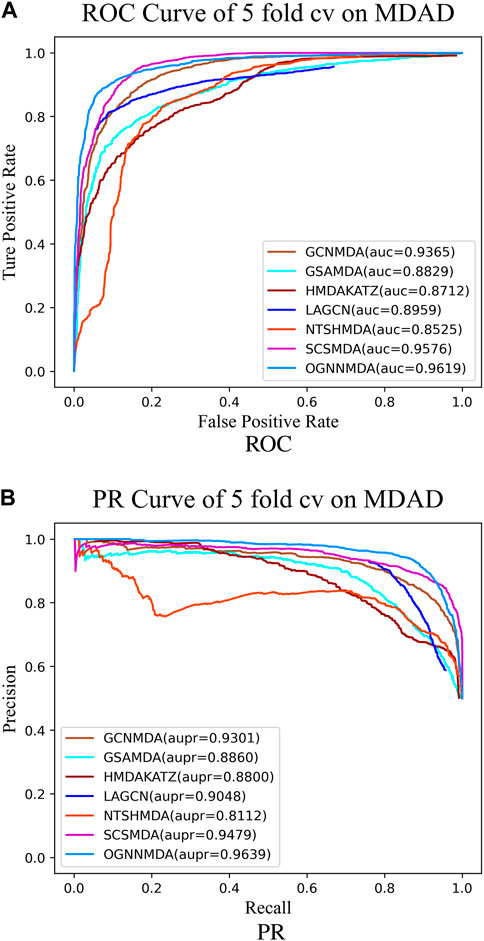
Figure 5. (A) ROC curves for each modeling approach based on the MDAD dataset 5-cv. (B) PR curves for each modeling approach based on the MDAD dataset 5-cv.
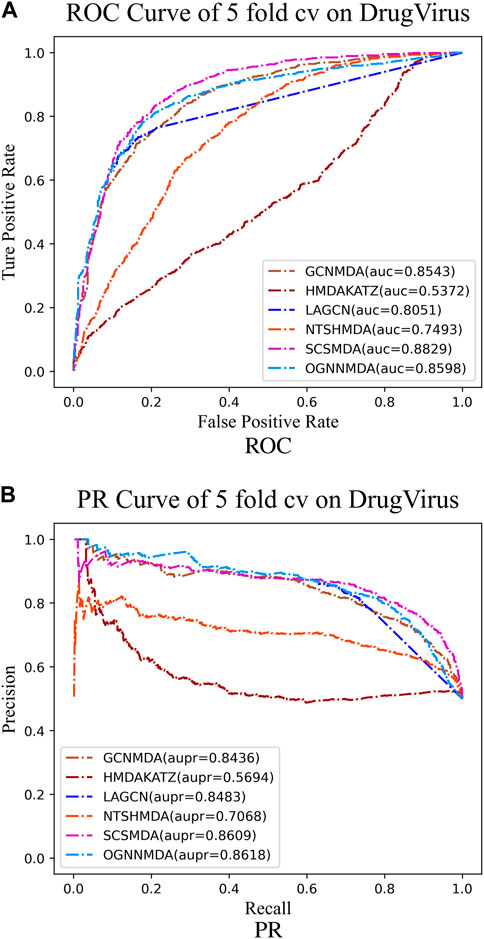
Figure 6. (A) ROC curves for each modeling approach based on the DrugVirus dataset 5-cv. (B) PR curves for each modeling approach based on the DrugVirus dataset 5-cv.
Based on the experimental results from Table 2, it is evident that OGNNMDA achieves the highest AUC values on the aBiofilm dataset, with an average AUC of 0.9693 ± 0.0008. This is 0.65% higher than the next highest AUC value of 0.9628 ± 0.0021 obtained by SCSMDA. OGNNMDA also outperforms other methods in terms of AUPR, Accuracy, and F1-Score, with values of 0.9690 ± 0.0009, 0.9141 ± 0.0031, and 0.9151 ± 0.0026, respectively.
Similarly, in Table 3, which presents the results on the MDAD dataset, OGNNMDA exhibits superior performance across all four evaluation metrics. The comparison between the two tables suggests that OGNNMDA performs better on the aBiofilm dataset compared to MDAD. This disparity can be attributed to the sparser nature of the data in MDAD, resulting in a smaller ratio of positive to negative samples and a more pronounced sample imbalance issue.
Finally, we examine the results from Table 4, which presents the performance of all methods on the DrugVirus dataset. OGNNMDA achieved the highest AUPR score with a mean value of 0.8633 ± 0.0078; however, SCS-MDA outperformed others in terms of the AUC (0.8810 ± 0.0053), Accuracy (0.8098 ± 0.0071), and F1-score (0.8201 ± 0.0038). Notably, OGNNMDA did not maintain its leading position on the DrugVirus dataset as it did on the aBiofilm and MDAD datasets. This relative underperformance may be attributed to the smaller scale of the DrugVirus dataset compared to aBiofilm and MDAD, potentially limiting OGNNMDA’s ability to effectively train its more complex weighting parameters for optimal prediction.
5.3 Ablation experiment
To evaluate the efficacy of the ordered message-passing mechanism, this section presents ablation experiments, the results of which are presented in Table 5. In this context, GNN refers to a simple graph neural network model utilizing a mean aggregator as an encoder, while OGNN represents an enhanced ordered message-passing graph neural network model based on GNN, specifically the model proposed in this paper, OGNNMDA. The evaluation entails 5-fold cross-validation experiments on the aBiofilm and MDAD datasets, with specific parameter settings described in previous sections.
Based on the data presented in Table 5, the underlying GNN encoder exhibits poor performance on both datasets, showing a significant gap in all metrics compared to the OGNNMDA model utilizing OGNN as the encoder. Therefore, it is reasonable to conclude that the ordered message-passing mechanism effectively enhances the embedding performance of GNN, leading to improved prediction results in microbe drug association prediction.
5.4 Case study
To validate the prediction performance of OGNNMDA, case study experiments were conducted using two popular drugs and two microbes as targets. First, OGNNMDA was trained on the complete aBiofilm dataset to obtain the predicted association information neighbor matrix. Then, the top 20 most relevant objects for each target microbe and drug were filtered out. Finally, the relevant published PubMed literature was searched to validate the predicted microbe-drug association pairs against existing references. The first drug selected for the case study was ciprofloxacin, a fluorinated quinolone antibiotic, which has been extensively studied and shown to be associated with a wide range of human microbiome (Yayehrad et al., 2022). For instance, Rehman et al. (2019) demonstrated the effectiveness of amphotericin-B and 5% ciprofloxacin in blocking the growth mechanisms of Pseudomonas aeruginosa and Candida albicans. Ciprofloxacin has also shown susceptibility against Staphylococcus aureus, Staphylococcus epidermidis, Mycobacterium subspecies, Escherichia coli, and Mycobacterium tuberculosis (Smirnova and Oktyabrsky, 2018). The second drug chosen for the case study is moxifloxacin, a fluoroquinolone antibiotic (Rodríguez-López et al., 2020), known to be associated with antibiotic-resistant bacteria (ARB) (Loyola-Rodriguez et al., 2018) and Listeria monocytogenes (Rodríguez-López et al., 2020). The specific experimental results for the two drugs are presented in Tables 6, 7, respectively. These tables provide supporting literature information for the top 20 predicted microbes associated with ciprofloxacin and moxifloxacin. Upon observing Tables 6, 7, it is evident that 20 and 17 out of the top 20 predicted microbes associated with ciprofloxacin and moxifloxacin, respectively, have been validated by the available literature.
Furthermore, the first microbe selected for the case study was Aggregate Actinobacteria Accompanying Bacteria, a Gram-negative bacterium belonging to the family Pasteuriaceae (Krueger and Brown, 2020). It is primarily found in the oral cavity and is associated with various oral diseases and systemic infections (Jensen et al., 2019). In terms of its impact on human health, aggregates of Actinobacillus companionis are commonly linked to periodontal diseases, particularly aggressive forms of periodontitis. This bacterium has the ability to invade and colonize periodontal tissues, leading to inflammation, destruction of the periodontal ligament, and bone loss. Consequently, it is often found at a higher rate in individuals with severe periodontal disease. Sol et al. demonstrated that sub-killer concentrations of LL-37, Cathelicidin, and Scrambled LL-37 inhibit the biofilm formation of Actinobacillus actinomycetemcomitans and act as conditioning agents and lectins, greatly enhancing clearance by neutrophils and macrophages (Sol et al., 2013). Basavaraju et al. found that AHL lactonase hydrolyzes the lactone ring in the high serine portion of AHL, without affecting the rest of the signaling molecular structure. This inhibitory effect of AHL lactonase on group sensing of actinomycete aggregates has been observed (Basavaraju et al., 2016). The second microbe chosen for the case study was Clostridium nucleatum, a bacterium known for causing opportunistic infections and recently associated with colorectal cancer (Brennan and Garrett, 2019). In this study, Tables 8, 9 present the top 20 predicted drugs that are most relevant to Aggregate Actinobacteria Accompanying Bacteria and Clostridium nucleatum, respectively. Based on the information in the tables, 17 out of the top 20 predicted drugs for Aggregate Actinobacteria Accompanying Bacteria and 18 out of the top 20 predicted drugs for Clostridium nucleatum have been validated in the existing literature. Therefore, it can be concluded that OGNNMDA achieves satisfactory predictive performance in both microbe and drug case studies.
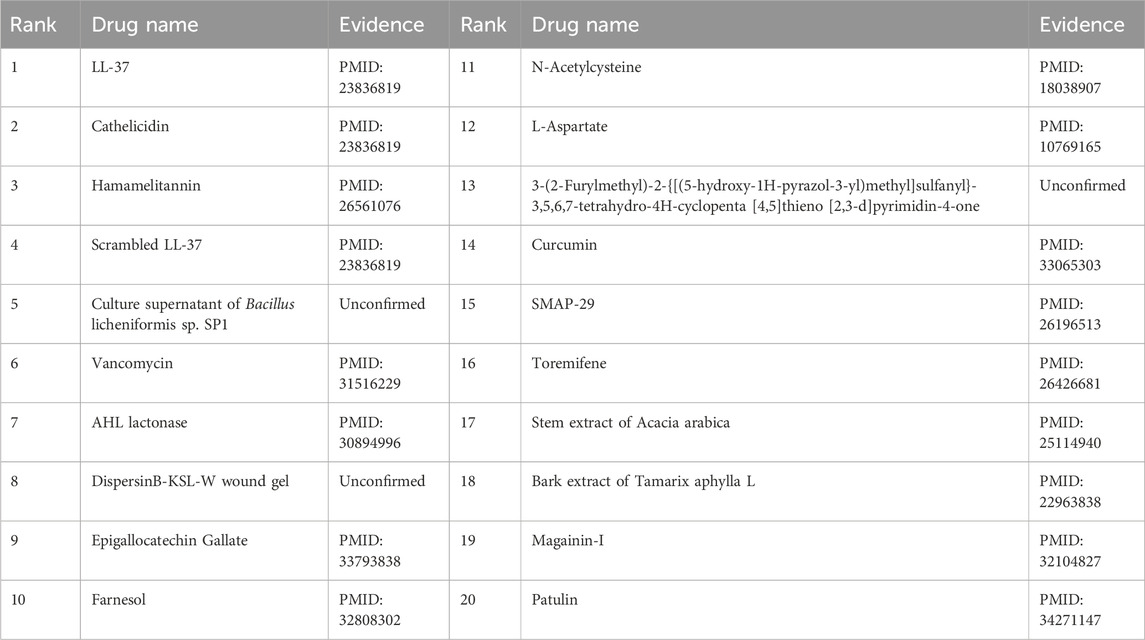
Table 8. Top 20 drugs associated with the microbe Aggregatibacter actinomycetemcomitans predicted by OGNNMDA.
6 Conclusion and discussion
This paper proposes OGNNMDA, a novel deep learning model for predicting potential microbe-drug associations, based on graph neural networks (GNNs) with an ordered message-passing mechanism. OGNNMDA utilizes multiple sources of biological data to construct similarity features for drugs and microbes, which are combined to form a heterogeneous network containing association and similarity information. To obtain drug and microbe embeddings, a multilayer GNN with ordered message passing is employed to differentiate node neighborhood messages during the message passing stage. A bilinear decoder is then used to generate association prediction scores. The OGNNMDA methodology was subjected to a rigorous evaluation regimen, encompassing comparative experiments on the aBiofilm and MDAD datasets as well as the DrugVirus dataset, where it utilized a 5-fold cross-validation scheme. The empirical outcomes revealed that OGNNMDA surpassed the current state-of-the-art performance benchmarks on both the aBiofilm and MDAD datasets. However, in the context of the DrugVirus dataset, OGNNMDA demonstrated a commendable yet second-best performance compared to existing methods. For clarity, while comprehensive experimental evaluations including comparative analyses were conducted for the DrugVirus dataset, the ablation experiments and case studies were confined to the aBiofilm and MDAD datasets alone. Despite this, the overall results affirm OGNNMDA’s robustness and competitive advantage in predicting potential microbe-drug associations across different datasets. The main contributions of this model can be summarized as follows.
1. It fully leverages additional biomedical data, such as microbe functional similarity based on microbial genomic information and drug molecular structural phase-based feature similarity.
2. It introduces an improved GNN model with an ordered message-passing mechanism, which achieves better embedding performance by distinguishing node neighbor messages.
3. The overall model outperforms existing state-of-the-art methods for predicting potential microbe-drug associations.
However, OGNNMDA is not without its limitations. The model’s performance is contingent upon the scale of the accessible dataset; with a relatively modest-sized corpus, the inherent sparsity in the microbial-drug association adjacency matrix can potentially impede the exhaustive exploitation of the graph’s structural information and limit the expressiveness of the learned embeddings. Furthermore, OGNNMDA homogenously handles microbial and drug nodes within the network without explicitly accounting for their distinctive patterns of interaction. In light of these challenges, future research directions can be directed towards:
1. Expanding Feature Representation: Augmenting the existing feature space by integrating supplementary biomedical data such as genomic sequences of microbes (Deng et al., 2022) and pharmacological similarity based on side effect profiles (Zheng et al., 2019). This enrichment could provide deeper insights into the intrinsic properties of both microorganisms and drugs, thereby enhancing the quality of the representations learned.
2. Addressing Sparsity Issues: Investigating innovative techniques to tackle the issue of sparse associations, which might involve adopting advanced link prediction strategies or devising specialized regularization methods that are tailored for sparse graphs. These approaches could ensure more efficient utilization of available relational information.
3. Adaptation of Graph Contrastive Learning: Exploring the potential benefits of incorporating graph contrastive learning (GCL) paradigms to improve the robustness and generalizability of the learned embeddings. GCL has shown promise in other domains by extracting meaningful node or graph representations from limited or unlabeled data, hence it could be a viable avenue to mitigate the impact of small datasets on OGNNMDA’s performance (Cai et al., 2023).
4. Refinement of Message-Passing Mechanisms: Examining alternative graph neural network architectures like Graph Attention Networks (GATs) and Graph Convolutional Networks (GCNs), and refining their message-passing processes to better suit the unique characteristics of the microbial-drug association problem.
By systematically addressing these limitations and venturing into new methodological frontiers, future iterations of OGNNMDA and similar models are poised to achieve heightened accuracy and resilience in predicting microbe-drug associations, thus contributing significantly to this burgeoning research domain.
Data availability statement
The original contributions presented in the study are included in the article/Supplementary material, further inquiries can be directed to the corresponding author.
Author contributions
JZ: Data curation, Software, Writing–original draft, Writing–review and editing. LK: Writing–review and editing. AH: Writing–review and editing. QZ: Writing–review and editing. DY: Writing–review and editing. CW: Data curation, Writing–review and editing.
Funding
The author(s) declare financial support was received for the research, authorship, and/or publication of this article. This work was partly sponsored by the National Natural Science Foundation of China (No. 62272064). This work was carried out in part using computing resources at the High Performance Computing Platform of Xiangtan University.
Conflict of interest
The authors declare that the research was conducted in the absence of any commercial or financial relationships that could be construed as a potential conflict of interest.
Publisher’s note
All claims expressed in this article are solely those of the authors and do not necessarily represent those of their affiliated organizations, or those of the publisher, the editors and the reviewers. Any product that may be evaluated in this article, or claim that may be made by its manufacturer, is not guaranteed or endorsed by the publisher.
References
Amato, K. R., Mallott, E. K., McDonald, D., Dominy, N. J., Goldberg, T., Lambert, J. E., et al. (2019). Convergence of human and old world monkey gut microbiomes demonstrates the importance of human ecology over phylogeny. Genome Biol. 20, 201–212. doi:10.1186/s13059-019-1807-z
Andersen, P. I., Ianevski, A., Lysvand, H., Vitkauskiene, A., Oksenych, V., Bjørås, M., et al. (2020). Discovery and development of safe-in-man broad-spectrum antiviral agents. Int. J. Infect. Dis. 93, 268–276. doi:10.1016/j.ijid.2020.02.018
Basavaraju, M., Sisnity, V. S., Palaparthy, R., and Addanki, P. K. (2016). Quorum quenching: signal jamming in dental plaque biofilms. J. Dent. Sci. 11, 349–352. doi:10.1016/j.jds.2016.02.002
Berg, R. V. D., Kipf, T. N., and Welling, M. (2017). Graph convolutional matrix completion. arXiv preprint arXiv:1706.02263.
Brennan, C. A., and Garrett, W. S. (2019). Fusobacterium nucleatum—symbiont, opportunist and oncobacterium. Nat. Rev. Microbiol. 17, 156–166. doi:10.1038/s41579-018-0129-6
Cai, X., Huang, C., Xia, L., and Ren, X. (2023). Lightgcl: simple yet effective graph contrastive learning for recommendation. arXiv preprint arXiv:2302.08191.
Catinean, A., Neag, M. A., Muntean, D. M., Bocsan, I. C., and Buzoianu, A. D. (2018). An overview on the interplay between nutraceuticals and gut microbiota. PeerJ 6, e4465. doi:10.7717/peerj.4465
Chen, Y., Tang, X., Qi, X., Li, C.-G., and Xiao, R. (2022). Learning graph normalization for graph neural networks. Neurocomputing 493, 613–625. doi:10.1016/j.neucom.2022.01.003
Cheng, X., Qu, J., Song, S., and Bian, Z. (2022). Neighborhood-based inference and restricted Boltzmann machine for microbe and drug associations prediction. PeerJ 10, e13848. doi:10.7717/peerj.13848
Cullin, N., Antunes, C. A., Straussman, R., Stein-Thoeringer, C. K., and Elinav, E. (2021). Microbiome and cancer. Cancer Cell 39, 1317–1341. doi:10.1016/j.ccell.2021.08.006
Deng, L., Huang, Y., Liu, X., and Liu, H. (2022). Graph2mda: a multi-modal variational graph embedding model for predicting microbe–drug associations. Bioinformatics 38, 1118–1125. doi:10.1093/bioinformatics/btab792
Duong, C. T., Hoang, T. D., Dang, H. T. H., Nguyen, Q. V. H., and Aberer, K. (2019). On node features for graph neural networks. arXiv preprint arXiv:1911.08795.
Hajiagha, M. N., Taghizadeh, S., Asgharzadeh, M., Dao, S., Ganbarov, K., Köse, Ş., et al. (2022). Gut microbiota and human body interactions; its impact on health: a review. Curr. Pharm. Biotechnol. 23, 4–14. doi:10.2174/1389201022666210104115836
Hattori, M., Tanaka, N., Kanehisa, M., and Goto, S. (2010). Simcomp/subcomp: chemical structure search servers for network analyses. Nucleic acids Res. 38, W652–W656. doi:10.1093/nar/gkq367
Huan, Z., Quanming, Y., and Weiwei, T. (2021). “Search to aggregate neighborhood for graph neural network,” in 2021 IEEE 37th International Conference on Data Engineering (ICDE) (IEEE), 552–563.
Jensen, A. B., Haubek, D., Claesson, R., Johansson, A., and Nørskov-Lauritsen, N. (2019). Comprehensive antimicrobial susceptibility testing of a large collection of clinical strains of aggregatibacter actinomycetemcomitans does not identify resistance to amoxicillin. J. Clin. Periodontology 46, 846–854. doi:10.1111/jcpe.13148
Kamneva, O. K. (2017). Genome composition and phylogeny of microbes predict their co-occurrence in the environment. PLoS Comput. Biol. 13, e1005366. doi:10.1371/journal.pcbi.1005366
Kim, N., Yun, M., Oh, Y. J., and Choi, H.-J. (2018). Mind-altering with the gut: modulation of the gut-brain axis with probiotics. J. Microbiol. 56, 172–182. doi:10.1007/s12275-018-8032-4
Krueger, E., and Brown, A. C. (2020). Aggregatibacter actinomycetemcomitans leukotoxin: from mechanism to targeted anti-toxin therapeutics. Mol. oral Microbiol. 35, 85–105. doi:10.1111/omi.12284
Li, Q., Han, Z., and Wu, X.-M. (2018). Deeper insights into graph convolutional networks for semi-supervised learning. Proc. AAAI Conf. Artif. Intell. 32. doi:10.1609/aaai.v32i1.11604
Liu, M., Gao, H., and Ji, S. (2020). “Towards deeper graph neural networks,” in Proceedings of the 26th ACM SIGKDD international conference on knowledge discovery and data mining, 338–348.
Long, Y., Luo, J., Zhang, Y., and Xia, Y. (2021). Predicting human microbe–disease associations via graph attention networks with inductive matrix completion. Briefings Bioinforma. 22, bbaa146. doi:10.1093/bib/bbaa146
Long, Y., Wu, M., Kwoh, C. K., Luo, J., and Li, X. (2020). Predicting human microbe–drug associations via graph convolutional network with conditional random field. Bioinformatics 36, 4918–4927. doi:10.1093/bioinformatics/btaa598
Loyola-Rodriguez, J. P., Ponce-Diaz, M. E., Loyola-Leyva, A., Garcia-Cortes, J. O., Medina-Solis, C. E., Contreras-Ramire, A. A., et al. (2018). Determination and identification of antibiotic-resistant oral streptococci isolated from active dental infections in adults. Acta Odontol. Scand. 76, 229–235. doi:10.1080/00016357.2017.1405463
Luo, J., and Long, Y. (2018). Ntshmda: prediction of human microbe-disease association based on random walk by integrating network topological similarity. IEEE/ACM Trans. Comput. Biol. Bioinforma. 17, 1341–1351. doi:10.1109/TCBB.2018.2883041
Ma, Q., Tan, Y., and Wang, L. (2023). Gacnnmda: a computational model for predicting potential human microbe-drug associations based on graph attention network and cnn-based classifier. BMC Bioinforma. 24, 35. doi:10.1186/s12859-023-05158-7
Ogunrinola, G. A., Oyewale, J. O., Oshamika, O. O., and Olasehinde, G. I. (2020). The human microbiome and its impacts on health. Int. J. Microbiol. 2020, 8045646. doi:10.1155/2020/8045646
Partula, V., Mondot, S., Torres, M. J., Kesse-Guyot, E., Deschasaux, M., Assmann, K., et al. (2019). Associations between usual diet and gut microbiota composition: results from the milieu intérieur cross-sectional study. Am. J. Clin. Nutr. 109, 1472–1483. doi:10.1093/ajcn/nqz029
Paul, S. M., Mytelka, D. S., Dunwiddie, C. T., Persinger, C. C., Munos, B. H., Lindborg, S. R., et al. (2010). How to improve R&D productivity: the pharmaceutical industry's grand challenge. Nat. Rev. Drug Discov. 9, 203–214. doi:10.1038/nrd3078
Piotrowski, A. P., Napiorkowski, J. J., and Piotrowska, A. E. (2020). Impact of deep learning-based dropout on shallow neural networks applied to stream temperature modelling. Earth-Science Rev. 201, 103076. doi:10.1016/j.earscirev.2019.103076
Pugazhendhi, A., Michael, D., Prakash, D., Krishnamaurthy, P. P., Shanmuganathan, R., Al-Dhabi, N. A., et al. (2020). Antibiogram and plasmid profiling of beta-lactamase producing multi drug resistant staphylococcus aureus isolated from poultry litter. J. King Saud University-Science 32, 2723–2727. doi:10.1016/j.jksus.2020.06.007
Qu, J., Song, Z., Cheng, X., Jiang, Z., and Zhou, J. (2023). A new integrated framework for the identification of potential virus–drug associations. Front. Microbiol. 14, 1179414. doi:10.3389/fmicb.2023.1179414
Rajput, A., Thakur, A., Sharma, S., and Kumar, M. (2018). abiofilm: a resource of anti-biofilm agents and their potential implications in targeting antibiotic drug resistance. Nucleic acids Res. 46, D894–D900. doi:10.1093/nar/gkx1157
Rehman, A., Patrick, W. M., and Lamont, I. L. (2019). Mechanisms of ciprofloxacin resistance in pseudomonas aeruginosa: new approaches to an old problem. J. Med. Microbiol. 68, 1–10. doi:10.1099/jmm.0.000873
Rodríguez-López, P., Rodríguez-Herrera, J. J., and Cabo, M. L. (2020). Tracking bacteriome variation over time in listeria monocytogenes-positive foci in food industry. Int. J. food Microbiol. 315, 108439. doi:10.1016/j.ijfoodmicro.2019.108439
Smirnova, G. V., and Oktyabrsky, O. N. (2018). Relationship between escherichia coli growth rate and bacterial susceptibility to ciprofloxacin. FEMS Microbiol. Lett. 365, fnx254. doi:10.1093/femsle/fnx254
Sol, A., Ginesin, O., Chaushu, S., Karra, L., Coppenhagen-Glazer, S., Ginsburg, I., et al. (2013). Ll-37 opsonizes and inhibits biofilm formation of aggregatibacter actinomycetemcomitans at subbactericidal concentrations. Infect. Immun. 81, 3577–3585. doi:10.1128/IAI.01288-12
Song, Y., Zhou, C., Wang, X., and Lin, Z. (2023). Ordered gnn: ordering message passing to deal with heterophily and over-smoothing. arXiv preprint arXiv:2302.01524.
Sun, Y.-Z., Zhang, D.-H., Cai, S.-B., Ming, Z., Li, J.-Q., and Chen, X. (2018). Mdad: a special resource for microbe-drug associations. Front. Cell. Infect. Microbiol. 8, 424. doi:10.3389/fcimb.2018.00424
Tan, S. Z. K., Du, R., Perucho, J. A. U., Chopra, S. S., Vardhanabhuti, V., and Lim, L. W. (2020). Dropout in neural networks simulates the paradoxical effects of deep brain stimulation on memory. Front. Aging Neurosci. 12, 273. doi:10.3389/fnagi.2020.00273
Tan, Y., Zou, J., Kuang, L., Wang, X., Zeng, B., Zhang, Z., et al. (2022). Gsamda: a computational model for predicting potential microbe–drug associations based on graph attention network and sparse autoencoder. BMC Bioinforma. 23, 492. doi:10.1186/s12859-022-05053-7
Tian, Z., Yu, Y., Fang, H., Xie, W., and Guo, M. (2023). Predicting microbe–drug associations with structure-enhanced contrastive learning and self-paced negative sampling strategy. Briefings Bioinforma. 24, bbac634. doi:10.1093/bib/bbac634
Wang, L., Yang, X., Kuang, L., Zhang, Z., Zeng, B., and Chen, Z. (2023). Graph convolutional neural network with multi-layer attention mechanism for predicting potential microbe-disease associations. Curr. Bioinforma. 18, 497–508. doi:10.2174/1574893618666230316113621
Yayehrad, A. T., Wondie, G. B., and Marew, T. (2022). Different nanotechnology approaches for ciprofloxacin delivery against multidrug-resistant microbes. Infect. Drug Resist. 15, 413–426. doi:10.2147/IDR.S348643
Yu, Z., Huang, F., Zhao, X., Xiao, W., and Zhang, W. (2021). Predicting drug–disease associations through layer attention graph convolutional network. Briefings Bioinforma. 22, bbaa243. doi:10.1093/bib/bbaa243
Zhang, M., and Chen, Y. (2018). Link prediction based on graph neural networks. Adv. neural Inf. Process. Syst. 31. doi:10.48550/arXiv.1802.09691
Zheng, Y., Peng, H., Ghosh, S., Lan, C., and Li, J. (2019). Inverse similarity and reliable negative samples for drug side-effect prediction. BMC Bioinforma. 19, 554–104. doi:10.1186/s12859-018-2563-x
Zhu, L., Duan, G., Yan, C., and Wang, J. (2019a). “Prediction of microbe-drug associations based on katz measure,” in 2019 IEEE international conference on bioinformatics and biomedicine (BIBM) (IEEE), 183–187.
Keywords: graph neural network, ordered message-passing mechanism, microbe-drug association, multi-similarities, prediction model
Citation: Zhao J, Kuang L, Hu A, Zhang Q, Yang D and Wang C (2024) OGNNMDA: a computational model for microbe-drug association prediction based on ordered message-passing graph neural networks. Front. Genet. 15:1370013. doi: 10.3389/fgene.2024.1370013
Received: 13 January 2024; Accepted: 14 March 2024;
Published: 16 April 2024.
Edited by:
Wen Zhang, Huazhong Agricultural University, ChinaReviewed by:
Jia Qu, Changzhou University, ChinaAdvait Balaji, Occidental Petroleum Corporation, United States
Copyright © 2024 Zhao, Kuang, Hu, Zhang, Yang and Wang. This is an open-access article distributed under the terms of the Creative Commons Attribution License (CC BY). The use, distribution or reproduction in other forums is permitted, provided the original author(s) and the copyright owner(s) are credited and that the original publication in this journal is cited, in accordance with accepted academic practice. No use, distribution or reproduction is permitted which does not comply with these terms.
*Correspondence: Linai Kuang, kla@xtu.edu.cn