- 1 Department of Anesthesiology, Columbia University Medical Center, New York, NY, USA
- 2 Department of Psychology, Indiana University, Bloomington, IN, USA
- 3 Department of Psychology, Villanova University, Villanova, PA, USA
Recent investigations into the neural mechanisms that underlie temporal perception have revealed that the striatum is an important contributor to interval timing processes, and electrophysiological recording studies have shown that the firing rates of striatal neurons are modulated by the time in a trial at which an operant response is made. However, it remains unclear whether striatal firing rate modulations are related to the passage of time alone (i.e., whether temporal information is represented in an “abstract” manner independent of other attributes of biological importance), or whether this temporal information is embedded within striatal activity related to co-occurring contextual information, such as motor behaviors. This study evaluated these two hypotheses by recording from striatal neurons while rats performed a temporal production task. Rats were trained to respond at different nosepoke apertures for food reward under two simultaneously active reinforcement schedules: a variable-interval (VI-15 s) schedule and a fixed-interval (FI-15 s) schedule of reinforcement. Responding during a trial occurred in a sequential manner composing three phases; VI responding, FI responding, VI responding. The vast majority of task-sensitive striatal neurons (95%) varied their firing rates associated with equivalent behaviors (e.g., periods in which their snout was held within the nosepoke) across these behavioral phases, and 96% of cells varied their firing rates for the same behavior within a phase, thereby demonstrating their sensitivity to time. However, in a direct test of the abstract timing hypothesis, 91% of temporally modulated “hold” cells were further modulated by the overt motor behaviors associated with transitioning between nosepokes. As such, these data are inconsistent with the striatum representing time in an “abstract’ manner, but support the hypothesis that temporal information is embedded within contextual and motor functions of the striatum.
Introduction
The ability to adapt to the temporal structure of events in the seconds to minutes range, interval timing, is critical for behaving in an efficient manner with respect to an unstable, but predictable, environment (Gallistel, 1990; Brunner et al., 1996; Buhusi and Meck, 2005). Besides providing the important ability to predict when specific events should occur, interval timing may also be essential for the computational processes underlying associative learning (Gibbon and Balsam, 1981; Miller and Barnet, 1993; Gallistel and Gibbon, 2000; Balsam et al., 2006), adaptive foraging (Kacelnik and Bateson, 1996), and rate estimation (Brunner et al., 1992). In addition, disordered timing may contribute to behavioral deficits in addictive disorders (Bickel and Marsch, 2001; Perry and Carroll, 2008) as well as those seen in a variety of patient populations (Harrington et al., 1998; Malapani et al., 2002; Penney et al., 2005; Beste et al., 2007). However, despite the relevance of interval timing to adaptive behavior, the neural mechanisms underlying this capacity remain unclear. While recent electrophysiological results from rats have shown that primary visual cortex activity is modulated in timing tasks (Shuler and Bear, 2006), thereby opening the possibility that time is computed within single cortical structures (see also Karmarkar and Buonomano, 2007), behavioral work has shown that temporal memories can be transmitted or combined across modalities (Meck and Church, 1982; Roberts and Holder, 1984; Nagarajan et al., 1998; Swanton et al., 2009), thereby suggesting a centralized timing process that operates in an “abstract,” or amodal, fashion (Walsh, 2003; van Wassenhove, 2009). Likewise, functional neuroimaging work in humans (Ferrandez et al., 2003; Nenadic et al., 2003; Coull, 2004; Harrington et al., 2004; Macar et al., 2004; Pouthas et al., 2005; Tregellas et al., 2006; Livesey et al., 2007; Stevens et al., 2007), lesion studies in rodents (Dietrich et al., 1997; Meck, 2006), and electrophysiological recordings from humans (Macar et al., 1999; Pfeuty et al., 2005), non-human primates (Brody et al., 2003; Leon and Shadlen, 2003; Sakurai et al., 2004; Janssen and Shadlen, 2005; Tsujimoto and Sawaguchi, 2005; Mita et al., 2009) and rodents (Matell et al., 2003, 2011), have implicated a broad network of non-sensory structures in interval timing, including parietal and frontal cortices, the basal ganglia, and thalamus. Thus, the available evidence is consistent with the notion of an amodal temporal representation available for behavioral control.
In an attempt to integrate the wide array of neural structures involved in interval timing, Matell and Meck(2000, 2004) proposed the striatal beat frequency (SBF) model of interval timing. In SBF, cortical activity is hypothesized to evolve as a function of time since an event’s occurrence, thereby functioning as a “clock” signal. The striatum is proposed to detect the state of the cortex and respond when the cortical pattern matches previously reinforced states, thereby serving as the memory and decision stages of an information processing model. Output from the striatum is then fed through basal ganglia output channels to the thalamus en route to the motor cortex, thereby engendering a behavioral response.
In order to examine the involvement of the cortex and striatum in interval timing, Matell et al. (2003) tested rats on a mixed 10-s, 40-s probabilistic fixed-interval schedule while recording single cell activity from both anterior cingulate cortex and dorsal anterior striatum. Due to a lower probability of reinforcement at the short duration compared to the long duration, average behavioral response rates ramped up to a maximum around 10 s, and if reinforcement was not delivered, responding ramped back down before ramping back up to an equivalent maximal rate at 40 s. Suggesting a direct relation to motor behavior, many neurons in both structures responded in a similar pattern (i.e., equivalent maximal activity at both 10 and 40 s). However, a subset of neurons showed differential firing rates at one of these times as compared to the other, demonstrating their sensitivity to time. The discrepancy in firing rate between 10 and 40 s was larger in the striatal neurons than the cortical neurons, thereby supporting SBF’s contention that the striatum serves to “recognize” specific trained intervals.
Intriguingly, many of these temporally sensitive neurons showed maximal peak-shaped firing at 10 s, and a secondary, smaller increase in firing at 40 s (see Figure 1A). This activity pattern can be interpreted in two ways: temporally modulated motor coding (Figure 1B) versus “abstract” temporal coding (Figure 1C). In the temporally modulated motor coding scheme, the heightened activity at both 10 and 40 s critically depends upon the co-occurring motor activity of the rat which also peaked at 10 s and ramped to 40 s. In this scheme, the temporal specificity of the neurons is seen as a modulation in the strength of this motor-related firing at one time compared to another. For example, as shown in Figure 1B, a neuron that fires at a greater rate at 10 s than at 40 s could result from an increase in response related firing at 10 s as compared to response related firing at 40 s (the same result could also be due to a decrease in response related activity at 40 s). Support for this type of temporally modulated motor coding scheme comes from classic views of the basal ganglia as a set of motor structures (DeLong, 1983; Marsden, 1984; Schultz and Romo, 1988). In the latter, “abstract,” coding scheme, temporally specific striatal activity would occur without modulation by the motor behaviors of the rat, and the elevated, but differential, firing rates at 10 and 40 s reflect a representation of both of these criterion durations. As shown in Figure 1C, the lower rate of activity at 40 s reflects the scalar variability that is characteristic of interval timing behavior (i.e., the decrease in precision and amplitude seen when subjects estimate longer durations (Gibbon, 1977). In this scheme, the motor activity is a “downstream” behavioral response to the temporal information reflected in the heighted firing rates of the striatal neurons. Support for a motor-independent “abstract” coding scheme comes from recording work in primates in which firing rates fluctuate in a duration-specific manner in the absence of observable behavior (Brody et al., 2003; Leon and Shadlen, 2003).
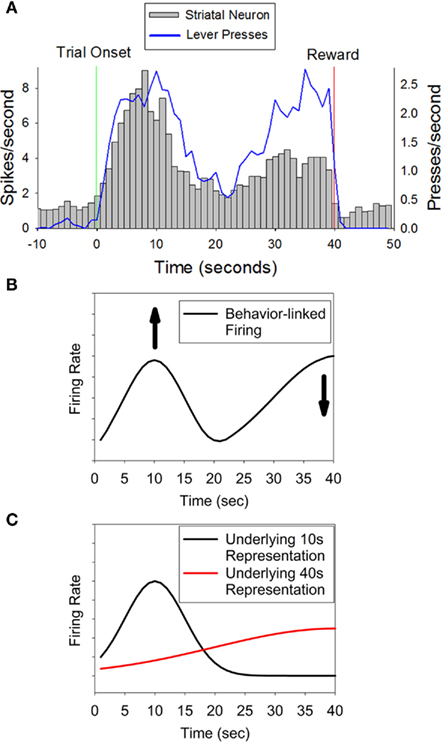
Figure 1. Panel (A) (adapted from Matell et al., 2003) displays the activity pattern of a representative striatal neuron showing differential activity at two times associated with possible reinforcement (10 and 40 s), indicating sensitivity to specific temporal interval(s). Importantly, the behavioral response rate was equivalent at these two times, thereby ruling out a simple motor coding role of the striatum. The peak in firing at 10 s and the secondary increase in firing at 40 s could result from two processes. (B) Represents a temporally modulated motor coding scheme in which the neural activity is directly tied to the motor behavior of the rat, but firing is enhanced at 10 s, and/or diminished at 40 s, thereby demonstrating temporal expectation. (C) Represents an “abstract” temporal coding scheme, such that both 10 and 40 s are represented by the neuron, and the smaller magnitude at 40 s reflects scalar variability. In this latter coding scenario, the motor behavior of the rat is a “downstream” effect and does not contribute to striatal firing rates.
To further investigate the role of the striatum in the temporal control of behavior and the temporal expectation of reinforcement, and to assess the form of changes in firing rate that emerge across the trial, we recorded from striatal neurons in rats. Subjects were trained to nosepoke into different nosepoke apertures at different times within a trial, with different reinforcement schedules associated with each aperture. Specifically, rats were trained that nosepoking into a central nosepoke aperture would probabilistically deliver reinforcement after a fixed interval (FI) had elapsed, while poking into a peripheral nosepoke aperture would probabilistically deliver reinforcement at a variable time (VI) in the trial (Matell and Portugal, 2007). No information was provided to the rats to indicate the time or nosepoke aperture at which reinforcement, if any, could be earned on each trial. As such, their behavior during non-reinforced probe trials progressed in a sequential manner; it was directed toward the VI nosepoke aperture early in the trial, transitioned to the FI nosepoke aperture as time approached the criterion fixed-interval duration, and then transitioned back to the VI nosepoke aperture following the passage of the criterion time. Because reinforcement could be earned simply by the rat having its snout in the nosepoke, without making an active response, sustained nosepoke “holding” was generally continuous throughout the trial, except when the rats switched nosepokes, allowing us to address whether striatal neurons showed temporal modulation in their firing rates during matched behaviors by comparing activity across and within nosepoking phases. This design also allowed us to assess whether such temporal modulation was sensitive to the collateral motor behaviors made by the rat during the trial. Specifically, if striatal activity represents the time of predictable reinforcement irrespective of any collateral motor behaviors, neural activity during the behavioral transitions should fall within the range of firing rates occurring during the surrounding nosepoking phases. In contrast, if striatal neurons encode time within a behavioral context, the transition behaviors should produce abrupt changes in firing rate, such that the transition firing rates are outside the range of those associated with the surrounding nosepoke-related activity.
Materials and methods
Subjects
Five male Sprague–Dawley rats (Harlan Labs, Indianapolis, IN, USA) that were approximately 60 days of age at the start of the experiment were used in this study. Rats were housed in pairs with a 12-h light–dark cycle, with lights on from 8 a.m. to 8 p.m. Animals were trained during the light phase of the cycle, and were given continuous access to water throughout the study. All rats were kept on a restricted feeding schedule and their body weights were maintained at 85–90% of their free-feeding weights, adjusted for growth. Following electrode implantation surgeries, rats were housed individually. All procedures were conducted in accordance with the Villanova University’s Institutional Animal Care and Use Committee (IACUC).
Apparatus
All behavioral data were obtained using standard operant-conditioning chambers (30.5 × 25.4 × 30.5 cm – Coulbourn Instruments). The sides of the chamber were ventilated Plexiglas, and the front wall, back wall and ceiling were aluminum. The floor was composed of stainless steel bars. A pellet dispenser attached to the back wall of the operant chamber delivered 45-mg sucrose pellets (Formula F; Noyes Precision, Lancaster, NH, USA) to a food cup. Three nosepoke response apertures with LED cue lights in their interior were placed on the front wall of the chamber. In order to ensure that responding on each nosepoke aperture was broadly similar in terms of body position, two aluminum “hallway” barriers (30.5 cm high × 8.2 cm length) were attached to the front wall so that a rat could not respond on the nosepokes unless its body was perpendicular to the front wall. These barriers also prevented the rat from rapidly switching between nosepokes. The operant chambers were also equipped with a houselight and a seven-tone audio generator. Behavioral data were transmitted to a computer program that recorded all events (Graphic State, Coulbourn Instruments, Whitehall, PA, USA). Following electrode implantation, all neural and behavioral data were recorded with a 40-kHz A-D data acquisition system (Recorder, Plexon Inc., Dallas, Texas).
Behavior
Magazine training (1 session)
In this procedure, a sucrose pellet was delivered once per min for 60 min.
Nosepoke autoshaping (2–3 sessions)
Following magazine training, rats were given sessions of nosepoke autoshaping. In this phase of training, reinforcement was delivered, independently of responding, once per min for the 60-min session. Prior to reinforcement, the center nosepoke cue light was turned on for 1 s. Responses made by the animal on the center nosepoke at any time during the session also resulted in reinforcement. Rats met the autoshaping criterion once they made 60 nosepokes within a session on two consecutive days.
Nosepoke discrimination training (1 session)
Rats were trained to respond on all three nosepokes for reinforcement. Trials began with the activation of a pulsing 1 kHz tone (pulse frequency = 12.5 Hz) and continuous onset of a randomly selected nosepoke light. Reinforcement was delivered for the first poke into the lit nosepoke aperture and the tone and nosepoke light were extinguished, ending the trial. Responses to unlit nosepokes had no consequence. Reinforcement was followed by a random 20–40 s inter-trial interval (ITI) on this and all subsequent procedures. Session duration was 60 min. All rats met the criterion of 20 responses on each active nosepoke during the first session.
Fixed-interval training (∼15 sessions)
Rats were trained on a discrete trials 15 s fixed-interval schedule. Trials again commenced with a 12.5-Hz pulsing 1 kHz tone. In addition to the tone, the cue light of either the left or right nosepoke was activated, and a response made on the illuminated nosepoke initiated an FI trial. At the start of the FI trial, a continuous 4 kHz tone and the center nosepoke cue light were activated, and the side nosepoke light extinguished. Subjects were reinforced for the first response on the FI nosepoke after 15 s had elapsed. Rats were also reinforced if their snout was inside the nosepoke when the criterion duration (15 s) was reached. The tone and cue light were terminated after reinforcement. Sessions lasted 120 min in this and all subsequent procedures. After several sessions, an examination of average response probability as a function of signal duration showed the classic scallop pattern of responding (Ferster and Skinner, 1957). Rats advanced to the next phase of training once the average response probability at the criterion duration (bin from 14–15 s) exceeded 80%.
Peak-interval training (∼10 sessions)
Peak-interval training was identical to fixed-interval training, except that a proportion of trials (50%) were non-reinforced probe trials. On probe trials, the 4-kHz tone and the cue light of the FI nosepoke remained on for 2.5–3.5 times the criterion duration (38–53 s), and all responses had no programmed consequence. No indication was given to the subject as to which trial type (FI or probe) had been selected. As with previous research (Roberts, 1981; Church et al., 1991), an examination of average response probability as a function of time since signal onset revealed a nearly symmetrical, Gaussian shaped function centered around 15 s. In order to quantify the accuracy and precision of temporal responding, this peak function was fit with a Gaussian curve, and the mean and standard deviation of this curve were used as a measure of the expected time of reinforcement (Peak Time), and the precision of this expectation (Peak Spread). The coefficient of variation (Peak Spread/Peak Time) was calculated, and rats were advanced to the next phase of training once this statistic was less than 0.5 for five non-consecutive sessions.
Variable-interval training (∼2–4 sessions)
In this procedure, rats were trained to respond on the left or right nosepoke, randomly selected on each trial, using a variable-interval (15 s) schedule of reinforcement. Trials began in the same manner as during fixed-interval and peak-interval training, with a poke into the illuminated left or right nosepoke. Once the rat self-initiated the trial, the continuous 4 kHz tone commenced and the same nosepoke light which had been flashing to indicate an opportunity to initiate the trial was illuminated continuously. While the rat’s snout was inside of the active VI nosepoke, there was a 2% probability of reinforcement every 300 ms. This low probability of reinforcement resulted in a mean delay to reinforcement of 15 s if the rat occupied the nosepoke continuously. Responses made on the center FI nosepoke or the inactive VI nosepoke had no programmed consequence. Trials ended upon reinforcement. Rats were advanced to the next phase of training once the average response probability during each of the first 5 s of a trial was at least 50%. Following criterion performance, rats were given an additional session of peak-interval training before advancing to the final phase of training.
Peak procedure with variable-interval training/testing (∼ 15 sessions training)
In the final phase of training, rats were trained on a procedure that integrated the peak-interval and variable-interval phases of training, so that three possible trial types could be selected: 15 s FI trials, 38–53 s probe trials, and 15 s VI trials. On all trials, one of the VI nosepoke cue lights (left or right, randomly selected) and the center FI nosepoke cue light were illuminated. Each trial type had a 33% probability of being selected, and no external cues were provided to the animal to indicate which type of trial had been selected. Rats were trained on this procedure until their behavior on probe trials showed a sequential pattern of responding: initially poking on the active VI nosepoke aperture, switching to the FI nosepoke prior to the criterion duration of 15 s, and then returning to the active VI nosepoke after 15 s had passed. Once behavior stabilized, they were implanted with electrodes, given 1 week to recover, and re-trained on this procedure until performance returned to pre-surgical levels. Once criterion responding was restored, electrophysiological recording commenced.
Surgery
Electrodes
Movable electrode ensembles were built using a design described in Bilkey and Muir (1999). Briefly, eight 25 μm HML-coated tungsten microwires were assembled into a bundle. The microwires were cannulated and held in place with epoxy. Through the use of a drive screw, the microdrive allowed implanted electrode bundles to move ventrally through the brain. The total dimension of the bundle of eight electrode tips was approximately 1 mm × 1 mm.
Procedure
Rats were anesthetized with an intramuscular injection of ketamine (100 mg/kg) and xylazine (10 mg/kg), and placed in a stereotaxic frame. The skin and muscle on the skull were retracted, and a small hole was drilled into the skull at the target coordinates (center at AP + 0.5, ML ± 3.0, DV − 3.0). The bundle was implanted into dorsal striatum, and recordings during implantation confirmed that electrodes were operating properly and had been placed in the target structure. In addition to the electrode, four skull screws were implanted to hold the electrode in place. A stainless steel wire was wound around the skull screws to serve as a ground for the electrode. A second electrode bundle was inserted into the frontal cortex (center at AP + 3.0, ML ± 1.5, DV − 0.5), but due to the electrode design, we failed to acquire acceptable recordings from these cortical electrodes. Once the bundles were in place, dental cement was used to secure the array to the skull. The wound was closed, and antibiotic ointment was applied. The animals were given 1 week to recover, during which time they had free access to food and water.
Recording procedure
Following recovery, electrophysiological recordings began once rats re-attained stable performance on the final version of the procedure. Before every session, the electrode assembly was lowered 79 μm (1/4 screw turn) ventrally into the striatum. Headstage cables were plugged into the implants, and the rat was placed in the operant chamber with the sound attenuating cabinet door open in order to differentiate the chamber context during spike thresholding. Subsequently, the door to the operant chamber was closed, and the behavioral session was started. The data acquisition system recorded all neural activity that surpassed a thresholded voltage on each mircowire, as well as all behavioral events.
Single unit discrimination
An off-line computer program (Offline Sorter, Plexon, Dallas, Texas) was used to discriminate and isolate action potentials (“spikes”) from background noise, and from one another. This program allows the user to separate spikes by computing the principal components that maximally explain the variance in waveform shape. Single units were discriminated from noise by clustering in 3-D PCA space. Evaluation of the clustering of these principal components as a function of time in the session was performed to ensure stationarity of the signals. Once single units were discriminated, the timestamps of spikes and behavioral events were examined through a neural analysis program (NeuroExplorer, Nex Technologies, Littleton, MA, USA) and MatLab (The MathWorks, Natick, MA, USA).
Analysis
The first 38 s of each probe trial and all VI trials with durations greater than 38 s were analyzed and are referred to throughout as probe trials. Our primary goal in this study was to assess whether striatal neurons that varied with time and/or temporal predictability of reward had additional sensitivity to the differential motor behaviors that occurred across the trial. Because nosepoking behavior in each phase was not limited to a single sustained nosepoke, but often consisted of several poke and hold behaviors (see Figure 3B), as well as occasional “checking” movements to the food magazine, we isolated nosepoking periods as the times in which the rats’ snouts were within the nosepoke aperture. These periods were split into poke initiation, poke hold, and poke termination (see Figure 2). Specifically, the poke initiation period was composed of the first 200 ms following onset of a poke. The poke termination period was composed of the last 200 ms prior to termination of a poke. The poke hold period was defined as the remaining time window during which the snout was within the nosepoke aperture. We computed striatal firing rates during these nosepoking periods across the three nosepoking phases [the initial phase of VI nosepoking (VI1), the phase of FI nosepoking (FI), and the second phase of VI nosepoking (VI2)]. On trials with multiple pokes in each phase, the response rates/patterns were determined as the average response rates/patterns within a phase. We also assessed the firing rates during the transitions between nosepoke phases. Transitions were assessed over a 200-ms window immediately following the last nosepoke termination in each of the first two nosepoking phases (T1a and T2a following VI1 and FI withdrawal, respectively) and a 200-ms window immediately preceding the first nosepoke in the latter two nosepoking phases (T1b and T2b preceding FI and VI2 insertion, respectively). We then examined whether firing rates differed between and within behavioral phases to ascertain whether temporal aspects and/or motor behaviors modulated striatal activity. Each analysis is described below and significance was set at p < 0.05 for all analyses. Repeated measures ANOVAs were conducted by passing the data from MatLab to R, an open source statistical package implementing S, by utiziling the packages statconnDCOM (Baier and Neuwirth, 2007), and ezANOVA, using Type III sums of squares, and sum contrasts. Results were identical to those obtained from SPSS. Figure 2 graphically shows these comparisons periods.
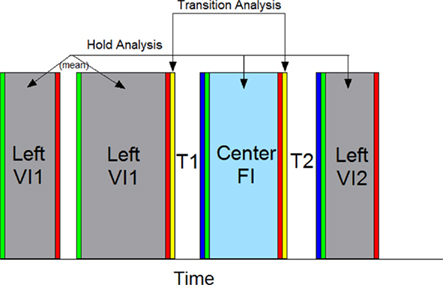
Figure 2. A graphical representation of the behavioral windows used for analysis. The firing rates on each trial were segmented as depicted, splitting the trial into behaviors directed into the VI nosepoke and FI nosepoke. Periods of time in which the rat held its snout within the nosepoke aperture were analyzed, as were brief intervals just following or preceding these nosepoking windows. Responding on the VI nosepoke prior to responding on the FI nosepoke were classified as the VI1 period, and responding on the VI nosepoke following FI responding were classified as the VI2 period. The first 200 ms following the onset of each poke was deemed a poke initiation and is designated in green. The final 200 ms preceding the offset of each poke was deemed a poke termination and is designated in red. The time between poke initiation and poke termination was deemed a nosepoke hold and is colored grey for VI nosepoke holds and light blue for FI nosepoke holds. In order to switch from the VI nosepoke to the FI nosepoke, and vice-versa, the rat had to retreat down a hallway around current VI nosepoke and enter another hallway to advance toward the FI nosepoke. We classified the first and last 200 ms intervals of these switch periods as transitions. The retreat portion is designated yellow, and the procession portion is designated in dark blue. Some, but not all, response periods had multiple pokes/holds (e.g., VI1 here). Therefore, a mean firing rate for each response phase was computed. The response holds lasted varying durations, and the firing rate was simply reported as the total spike count/total duration of the hold. The remaining analytic windows (Poke Initiation, Poke Termination, Transition Retreat, and Transition advance) were of a fixed length, and were further split into ten 20 ms bins to evaluate changes in spike pattern (not shown). To identify whether a single neuron was modulated by the variables in question, repeated measure ANOVAs were conducted by comparing windows of equivalent color, representing equivalent behaviors, with each segment composing a within-subject phase. Individual trials served as “subjects.” Trials were randomly chosen to have the active VI nosepoke on the left or right side of the operant chamber, and the spatial position of the active nosepoke served as a between-subjects factor.
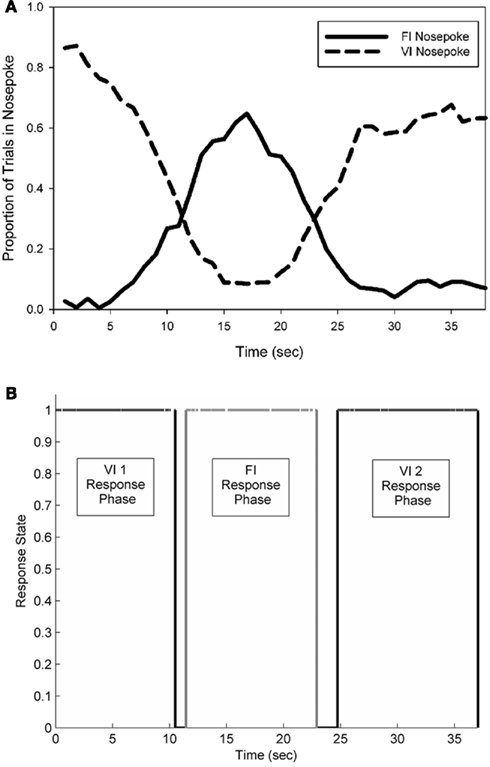
Figure 3. A representative example of the average pattern of responding from a single rat observed during an electrophysiological recording session (A). FI nosepoke responses formed a near-symmetrical peak distribution, centered near 15 s, while two separate periods of responding occurred on the VI nosepoke. (B) Depicts the pattern of responding observed in an individual probe trial. Black lines indicate occupancy in the VI nosepoke, and grey lines indicate occupancy in the FI nosepoke.
Task-sensitivity
Firing rates during each task phase were computed and entered into a repeated measures ANOVA in which behavior (VI1 poke initiation, VI hold, VI1 poke termination, T1a, T1b, FI poke initiation, FI hold, FI poke termination, T2a, T2b, VI2 poke initiation, VI2 hold, VI2 poke termination) served as a within-subject factor, and VI spatial position (left or right VI trial) served as a between-subject factor.
Temporal/schedule modulation across nosepoking phases
Firing rates during the three nosepoking phases were compared with repeated measures ANOVAs examining VI1, FI, and VI2 (initiation, holds, or termination performed separately) as within-subject factors and spatial position of the active VI nosepoke for each trial as a between-subject factor. With the nosepoke holds, because the hold duration varied across pokes and trials, we utilized the mean firing rate during all holds within a phase. For poke initiation and termination, we split the analysis window into ten 20 ms bins, and included time as a within-subject factor. In those neurons that had significant fluctuations in rate during hold behaviors across nosepoking phases, we broadly categorized their response pattern as ramping if firing rates grew in a monotonic manner, and peaking if firing rates were non-monotonic (i.e., low rate – high rate – low rate).
Temporal/schedule modulation across transition phases
Firing rates during the transition behaviors associated with switching nosepokes (200 ms windows as defined above) were compared with a repeated measures ANOVA with transition phase (e.g., T1a versus T2a) as a within-subject factor, and spatial position of the active VI nosepoke as a between-subject factor.
Sensitivity to overt motor behavior
As the majority of cells showed temporal sensitivity during the hold periods across nosepoking phases, we wished to examine whether these cells had firing rates that grew/decayed in a monotonic manner between phases, or whether their activity was influenced by the overt motor behaviors occurring during the transition between nosepokes. To this end, the expected firing rate was computed as a linear function from the mean rate during the last fifth of the preceding hold period to the mean rate during the first fifth of the forthcoming hold period. A repeated measures ANOVA was used to compare the actual firing rate during each transition period to this expected firing rate, with spatial location of the VI nosepoke serving as a between-subjects factor. For example, the firing rate during transition phase T1a was compared to the expected firing rate computed as a linear function from the firing rate during the last 1/5 of the preceding VI1 phase to the firing rate during the first 1/5 of the following FI phase.
Temporal modulation within a behavioral state
In many of the above analyses, the influence of phase/response schedule contingencies was confounded with the influence of elapsed time. Therefore, we analyzed the pattern of firing during nosepoke holding within a single trial. To this end, each phase of nosepoking was split into 5 equal duration sub-phases (Time), and spike rates during nosepoke holds in each of these sub-phases, on each trial, were entered into a repeated measures ANOVA with time as a within-subject factor and spatial position of the VI as a between-subject factor. Neurons with significant modulation within a phase were classified as ramping if the firing rates across their five sub-phases progressed in a monotonic manner, and as peaking if firing rate rose and fell (or vice-versa) in a bi-tonic manner with maximal (minimal) firing rate in the 3rd sub-phase. Because more than 75% of the duration of each phase was composed of nosepoke holding (rather than repeated poking throughout the phase), we performed a similar analysis on firing rates associated with poke initiation and termination, but we split each phase into halves rather than fifths to avoid missing values in the ANOVA.
Histological procedure
The final location of the electrodes was determined once data acquisition was completed. Rats were anesthetized, and a 100-μA current was passed through an electrode for 20 s, creating a small marking lesion. Subsequently, the rats were deeply anesthetized with pentobarbital and perfused intracardially with saline followed by 10% phosphate-buffered formalin. Brains were post-fixed for 1 week, immersed in 30% sucrose-formalin solution, frozen, and sectioned into 50 μm thick slices. Brain slices were stained with cresyl violet, and electrode tracks and placement were verified. Once the final location of each electrode bundle was determined histologically, the approximate location of the electrodes during recordings was computed based upon the number of ventral advancements made with the moveable electrode bundle.
Results
A total of 160 single units were recorded from 5 rats. Electrophysiological recordings occurred over the course of 28 sessions per animal, with approximately 30–40 probe length trials per session. On average, 11 recording sessions (range 3 to 28 sessions) yielded isolatable units. The electrodes passed through the dorsal striatum at the average AP coordinates of +0.44 mm from bregma, and ML coordinates of ± 3.2 mm from bregma. Recordings occurred at DV coordinates that ranged from −2.81 to −6.12, with mean starting depth at −4.01 and mean termination depth at −5.22.
Behavior
Average behavioral response patterns
Subjects primarily engaged in three bouts of sequential responding: an early phase of holding their snouts in the VI nosepoke, a phase of FI nosepoke holding, and a 2nd phase of holding in the VI nosepoke. Responses made at the FI nosepoke formed a near-symmetrical peak distribution, with the mean of this distribution centered near the FI duration of 15 s. During electrophysiological recordings, the average peak time of FI responding was 16.6 (±1.1) s. The mean peak spread of this distribution was 4.9 (±0.5) s, which resulted in a coefficient of variation of 0.30 (±0.04). Figure 3 (top panel) shows the average response pattern of a single representative rat on a single recording session.
Single trial behavioral responding
The nosepoke behavior on single probe trials was well characterized by the same sequential progression of responding as seen in the mean functions. The bottom panel of Figure 3 shows a single trial from the session data shown in the top panel of Figure 3. Across all rats, nosepoking on the VI nosepoke came to an initial end at a mean time of 9.2 (±1.8) s, followed by initiation of poking on the FI nosepoke at a mean time of 11.3 (±1.8) s. The mean termination time of FI nosepoking occurred at 20.5 (±1.8) s, and the mean initiation time of the 2nd bout of VI nosepoking occurred at 24.0 (±2.0) s. These times led to a mean FI response period spread of 9.2 (±2.0) s, and a midpoint of FI responding at 15.9 (±1.5) s.
During these response phases, the rats were predominantly holding their snouts within the nosepoke aperture, but occasionally moved their snouts in and out of the aperture, or backed away from the nosepoke and engaged in other behaviors, such as checking the food cup. The percentage of time during these nosepoking phases in which the rats’ snouts were held within the nosepoke aperture was 78% (±10). Mean poke duration was 1.13 (±0.9) s, with mean maximal poke duration (without snout removal) on each trial of 3.1 (±2.0) s. Our analyses were restricted to periods of time during which the rats snout was within the nosepoke aperture, or brief intervals (200 ms) immediately following or preceding phase transitions.
Neural activity
Trial-wide striatal firing patterns
Individual striatal neurons showed a variety of activity patterns, including peak-shaped profiles (Figure 4A) and ramp-shaped profiles (Figure 4B), or more commonly, complex patterns of activity that showed peak activations around the transitions between nosepoke phases (Figure 4C) or contained both peak-like and ramp-like components (Figure 4D). While these trial wide patterns of neural activity may be meaningful, it is well known that the smooth peak functions obtained in temporal production procedures result from averaging step-like responding (as seen in Figure 3B) across trials (Gibbon and Church, 1990; Cheng and Westwood, 1993; Church et al., 1994; Gallistel et al., 2004; Matell et al., 2006a). In other words, the smooth curves are the direct result of considerable variability in the times of response initiation and termination. Due to this variability, the functions shown in Figure 4 may mask abrupt firing rate changes associated with the behavioral transitions.
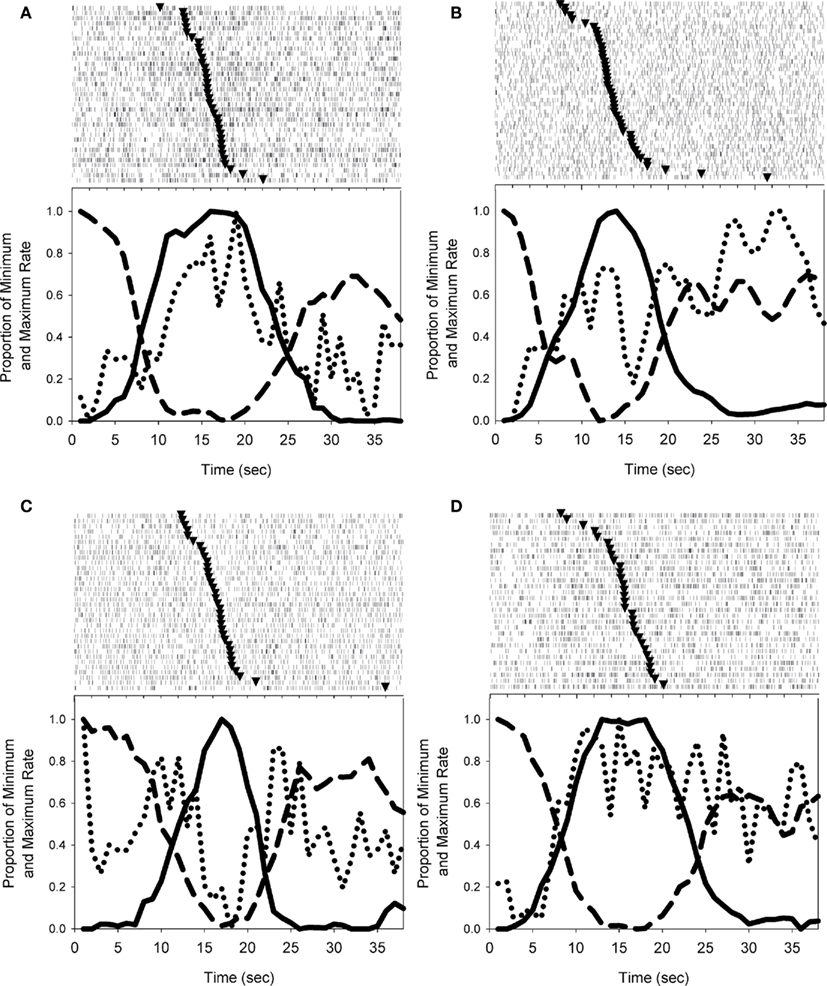
Figure 4. Examples of the firing patterns observed during probe trials. Solid and dashed lines depict nosepoking, dotted lines depict striatal firing rates, and triangles in rasters indicate the midpoint of FI responding on each trial. (A) Depicts a striatal neuron with a peak-shaped activation profile across the trial. (B) Shows a roughly monotonic increase in spike rate as time elapses. In (C), the activity of the cell differs across nosepoking phases (VI versus FI) in an inverted peak shape, but also shows abrupt excitations associated with the transition behaviors. (D) Displays a striatal neuron with both peak-like and ramp-like components. Trials displayed in peri-event rasters were ordered by the midpoint of FI responding.
Task sensitivity
To quantitatively determine whether spike rates were reliably varying in a task-related manner, we computed the mean firing rates on each trial during the periods of time in which a subject initiated a poke (200 ms after poke onset), held its snout within the nosepoke aperture (excluding poke initiation and termination periods), and terminated a poke (200 ms prior to poke offset). In addition, firing rates associated with transitioning between nosepoke apertures were computed using 200 ms windows bordered by the termination or initiation of responding at each phase. Although we did not empirically monitor the rats’ behaviors during these periods, anecdotal observation suggested that these brief 200 ms periods were wholly composed of locomotor behaviors in which the rats were either backing away (transition period A) or approaching (transition period B) the nosepoke. These behavioral periods compose the analytic windows on which all subsequent analyses are based (see Figure 2). Repeated measure ANOVAs with each behavioral period as a within subject factor, and spatial location of active VI nosepoke as a between-subject factor demonstrated that 137/160 (86%) of the neurons showed reliable firing rate fluctuations as a function of these behavioral periods. The remaining analyses were restricted to these task-sensitive neurons.
Temporal modulation across behavioral phases
To ascertain whether neuronal firing rates were modulated by temporal aspects of the task, repeated measures ANOVAs were performed as above, with matched behaviors comprising each phase (e.g., poke hold-related activity). In this way, differences in firing rate can be interpreted as being due to differences in time in the trial and/or the temporal predictability associated with the reinforcement schedule, rather than differences related to overt motor behavior.
First, we compared the mean firing rates that accrued during the periods of time in which the rat’s snout was held within the VI and FI nosepokes (excluding the first and last 200 ms of each poke to minimize the contributions of overt motor behavior). Because these hold periods lasted for different durations of time for each poke, we analyzed the mean firing rate during these hold periods without including elapsed time of the hold as a factor. 64% (88/137) of task-sensitive neurons showed temporal sensitivity in their firing rates. 49/88 cells showed a main effect of Phase, with no effect of spatial position. An additional 39 cells showed an interaction between Phase and Space, indicating phase sensitivity for at least one trial type (i.e., left VI or right VI trials). Of the neurons showing only a main effect of phase, 78% (38) had a peak shape (22 positive, 16 negative), whereas 22% (11) had a monotonic ramping progression across the three phases (7 positive, 4 negative). Of the 39 cells showing an interaction of phase and side, 10 had the same pattern of activity across the two sides (7 peak, 3 ramp), with the interaction resulting from different magnitudes of changes. The remaining 29 cells had different patterns as a function of the spatial position of the active VI nosepoke.
In a similar analysis, we compared firing rates during a 200-ms window either immediately after initiating a nosepoke or immediately before terminating a nosepoke within a response phase. Because these windows were of fixed length, we also examined the pattern of activity by including Time (ten 20 ms bins) as an additional within-subject factor. For poke initiation, 59% of neurons showed phase sensitivity (n = 81), either as a main effect of Phase alone (n = 28), and/or as an interaction of Phase with Side (n = 30), Time (n = 21), and/or the 3-way Phase × Time × Side interaction (n = 9). Similar results were found for poke termination, as 58% of neurons (n = 80) showed phase sensitivity. Of these neurons, 27 had a main effect of Phase alone, whereas the remaining neurons showed an interaction of Phase with Side (n = 32), Time (n = 20), and/or the 3-way interaction (n = 18). A total of 108 neurons showed sensitivity to phase for either poke initiation or poke termination, with 53 of these showing sensitivity to phase for both initiating and terminating a poke. Representative examples of poke-related activity that varied as a function of Phase are shown in Figure 5.
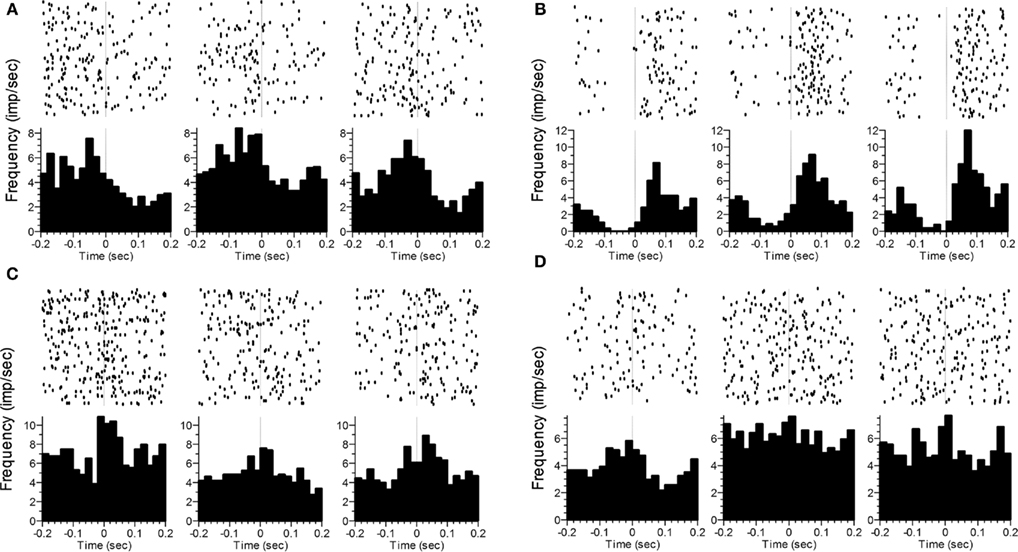
Figure 5. Representative examples of changes in the rate and/or pattern of firing related to poke initiation (A–C) or poke termination (D). The time of photobeam switch breakage (initiation) or completion (termination) is indicated by the vertical dashed line at t = 0. The three columns represent the three phases (VI1 poking, FI poking, VI2 poking). Statistics analyses were performed on the time after poke onset in (A–C), and prior to poke offset in (D). In (A), a significant difference as a function of Phase was seen. In (B), significant effects were found for Phase and Time. In (C), significant effects were seen for Phase, Time and the Phase × Time interaction. In (D), only Phase was significant.
Finally, we compared firing rates during the 200 ms window around the transition period from VI poking to FI poking or vice-versa. Specifically, we compared firing rates while the subject was backing away from the VI nosepoke (at the end of VI1) with those obtained while the subject was backing away from the FI nosepoke. We also compared firing rates when the subject was approaching the FI nosepoke (and the end of VI1) with those from the period of time associated with approaching the VI nosepoke (to begin VI2). As with the poke-related activity, Time (ten 20 ms bins) was included as a factor in order to assess changes in firing pattern. Firing rates while backing away from the nosepoke differed as a function of Phase for 69 neurons (50%), with 19 showing only a main effect of Phase, and the other 50 showing an interaction with Space (33), Time (16) and/or both (16). Firing rates during nosepoke approach differed as a function of Phase in 61 neurons (45%), with 9 showing only a main effect of Phase, and the other 52 showing an interaction with Space (38), Time (15), and/or the 3-way interaction (12). Ninety-three of the neurons showed phase sensitivity to at least one of the transitions, with 37 of these neurons showing sensitivity for both backing away from and approaching the nosepoke. Representative examples of transition-related activity that varied as a function of Phase are shown in Figure 6.
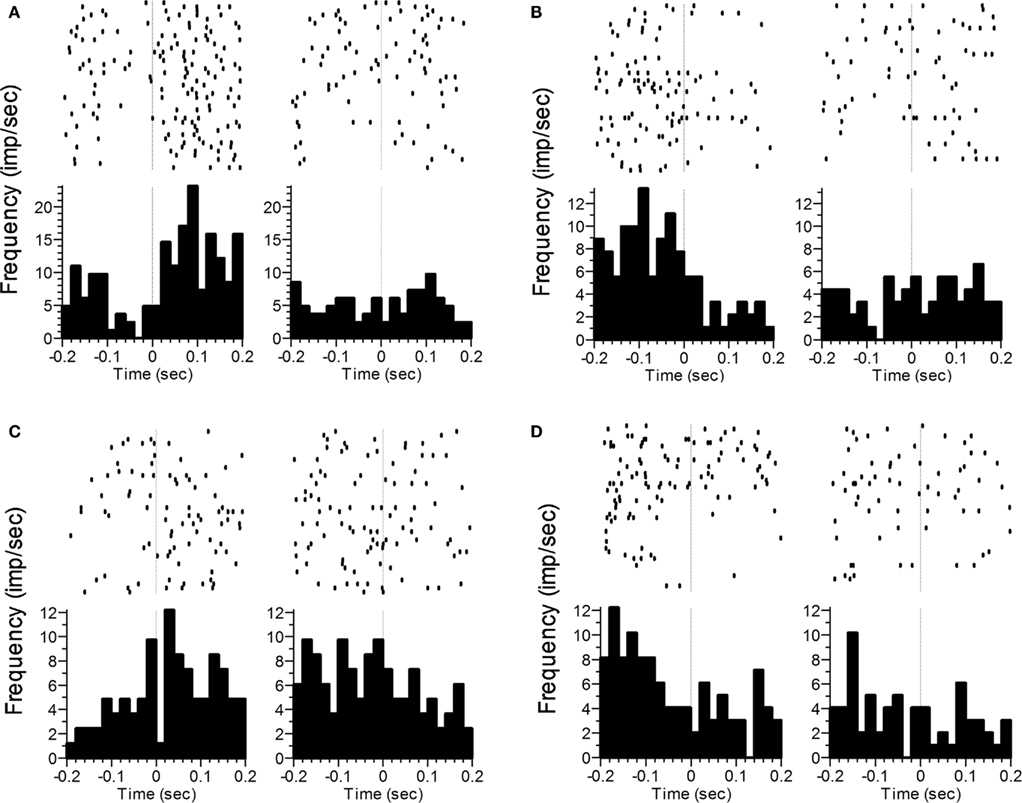
Figure 6. Representative examples of changes in the rate and/or pattern of firing related to transition behaviors. Panels (A) and (B) correspond to the termination of poking and backing away from the nosepoke, whereas panels (C) and (D) correspond to nosepoke approach and initiation of poking. The vertical line at t = 0 indicates offset of the response phase (A,B) or onset of the response phase (C,D). Statistics analyses were performed on the time after poke offset in panels (A,B), and prior to poke onset in panels (C,D). In panels (A,C), a significant difference as a function of Phase was seen. In panels (B,D), significant effects were found for Phase, Time, and the Phase × Time interaction.
Pooling over all of these comparisons between equivalent behaviors displayed at different phases within the task, 95% (131) of the task sensitive neurons showed sensitivity to phase for at least 1 behavior. Of these 131 neurons, 70 showed phase sensitivity to both holding and poking, 61 showed phase sensitivity to both holding and transitioning, and 77 showed phase sensitivity to poking and transitioning. Fifty neurons showed sensitivity to at least one of the poke responses (initiation or termination), one of the transition responses (backing away or approaching the nosepoke) and to holding, with 11 neurons showing sensitivity to phase for all five analyses (holding, poke in, poke out, transition-exiting, transition-entering).
Sensitivity to overt motor behavior
The primary question motivating this experiment was whether the hold-related firing of cells with temporal/phase modulation across the behavioral phases (i.e., holding during the VI and FI periods) had firing rates during one or more of the transition periods that differed from that expected based upon monotonic growth or decay associated with the immediately surrounding response phases. Of the 88 cells showing temporal/phase sensitivity during nosepoke hold behaviors, 80 (91%) had firing rates during one or more of the transition phases that were significantly different from the firing rates expected from the surrounding hold periods. The non-monotonic nature of the transition-related firing is shown in Figure 7. Indeed, all 137 task sensitive cells showed mean activity rates during one or both transition periods that were outside the range of the surrounding nosepoke hold-related firing rates.
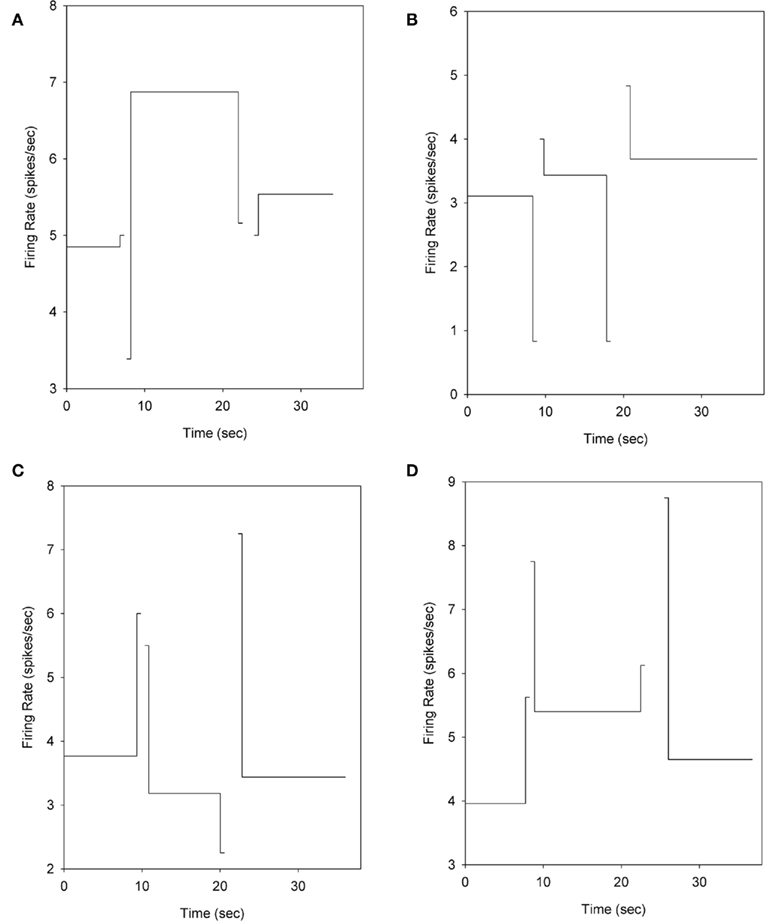
Figure 7. Mean firing rates during the hold windows and transitioning phases. The firing rate of each phase is plotted at a point on the abscissa that corresponds to the mean time at which these phases began and ended. The firing rates are computed by “triggering” at the time of each phase’s onset on a trial-by-trial basis to eliminate the temporal variability of each phase as it relates to trial onset. To facilitate display, the duration of the transition segments is shown over 500 ms, rather than the 200 ms used for analysis. The neurons in panels (A–D) are the same neurons shown in Figures 4A–D.
We also analyzed whether firing rates associated with nosepoke initiation or termination differed from that associated with holding the snout within the nosepoke. To this end, we ran a repeated measures ANVOA with phase (VI1, FI, VI2) and type (holding versus poking) as within subject factors, and spatial position of the active VI nosepoke as a between-subject factor. Of the 88 cells showing temporal/phase sensitivity during holding, 91% (n = 80) showed differential activity between holding and poking, with 64 neurons showing differences between holding and poke initiation, 56 neurons showing differences between holding and poke termination and 40 neurons showing differential activity for both initiation and termination. Together, 99% (87/88) of the neurons showing temporal sensitivity during nosepoke holds showed differential activity during overt motor behaviors (either poking or transitioning). 83% of these cells (73/88) showed differential activity between holding and both poking and transitioning. Such differential activity is incompatible with an abstract representation of time that evolves without sensitivity to the evolving behavior.
Modulation of activity within a response phase
As the above results suggested that striatal activity reflects temporal information as well as motor information, we sought to examine whether firing rates reliably varied as a function of time during nosepoke-holding behavior after controlling for the associated reinforcement schedule. To assess temporal sensitivity within a response phase, we divided the response phase on each trial into five equally spaced bins, and computed the firing rate during nosepoke holds during these bins. 67% (92) of the cells showed temporal sensitivity within at least one phase. Of the 49 cells showing only a main effect of phase in firing rate across the three response phases, without a spatial interaction (as described earlier), 34 (69%) showed significant variation in firing rate within one or more of these nosepoking phases, indicating that time in the trial influences the firing rate of these cells, in addition to any influence of reinforcement schedule. 20% of these cells (10/49) were modulated during the 1st VI phase, 41% of cells (20/49) had significant firing rate modulations during the FI phase, and 27% of these cells (13/49) were modulated during the 2nd VI phase. However, only 4 of these 49 cells showed a peak-shaped (3) or ramp pattern (1) during the FI period, with 7/49 cells showing ramp (3) or peak (4) patterns during one of the VI periods. Thus, while a substantial number of cells had variations in firing rate across time while holding their snouts in the nosepoke under the contingencies of a particular reinforcement schedule, this variation was generally inconsistent with theoretical expectations (i.e., patterns were not peak or ramp shaped).
Similarly, we assessed whether the firing rate and/or pattern associated with nosepoke initiation or termination changed as a function of time within a phase. Due to relatively low rates of poking (as the majority of time within a phase was composed of hold responses), we assessed variation in firing pattern from the first half to second half of each phase. 95% of the cells (131/137) showed changes in their poke response as a function of time within a phase. Seventy-nine cells showed temporal sensitivity within the VI1 period, 95 showed sensitivity during the FI period, and 83 showed sensitivity during the VI2 period. Pooling across both types of behaviors, 133 cells (96%) showed within-phase modulation to either the poke or hold, with 78 cells (56%) showing within phase sensitivity to both poking and holding behaviors.
Maximal change in firing rate
Given the dynamics in firing both across and within phases of the task, we were interested in identifying the behavioral phase that was most effective in modulating the activity of these striatal cells. To this end, we examined whether the nosepoking, holding, transition phases were associated with the maximal change in firing rate compared to the average firing rate associated with these behaviors. Of the 137 task-modulated cells, 3 (2%) showed the maximal rate change during nosepoke holds, 14 (10%) showed the maximal rate during a poke (either initiation or termination), while 120 (88%) had a maximal rate change during one of the transition periods.
Discussion
A number of recent studies have demonstrated that the striatum is a crucial component of the interval timing system (Rao et al., 2001; Malapani et al., 2002; Ferrandez et al., 2003; Matell et al., 2003; Coull, 2004; Harrington et al., 2004; Macdonald and Meck, 2005; Meck, 2006; Drew et al., 2007; Livesey et al., 2007; Stevens et al., 2007). The results of this study add further support to this idea by demonstrating that 95% of the behaviorally sensitive striatal neurons had different firing rates for equivalent behaviors as a function of the phase in the trial at which the behavior was emitted, indicating that either elapsed time and/or the temporal predictability of the reinforcement contingencies modulates firing rates. An equivalently high proportion of striatal cells (96%) showed variation in firing rate as a function of time within a single nosepoking period. As such, these data clearly indicate that the striatum is intimately involved in the temporal control of behavior. Nevertheless, the striatum is a functionally heterogeneous structure (Nisenbaum et al., 1988; Nakano et al., 2000; Chapman et al., 2003), and the large proportion of neurons showing temporal sensitivity should be interpreted with regard to the relatively small number (n = 160) of cells recorded. Still, our results are consistent with previous work showing that neural regions that innervate the striatum (cortex, thalamus, substantia nigra) have all been linked to interval timing (Lejeune et al., 1997; Harrington and Haaland, 1999; Macar et al., 1999, 2004; Komura et al., 2001; Rao et al., 2001; Brody et al., 2003; Ferrandez et al., 2003; Leon and Shadlen, 2003; Nenadic et al., 2003; Coull, 2004; Coull et al., 2004; Sakurai et al., 2004; Janssen and Shadlen, 2005; Tsujimoto and Sawaguchi, 2005; Jahanshahi et al., 2006; Matell et al., 2006a, 2011; Meck, 2006; Shuler and Bear, 2006; Mita et al., 2009). Such data led to the development of the SBF model of interval timing (Matell and Meck, 2000, 2004), which proposed that patterns of neural activity in the cortex evolve as a function of time, and that the striatum learns the cortical activity pattern occurring at the time of reinforcement via a dopaminergic reinforcement signal from the substantia nigra. In subsequent situations requiring the temporal control of behavior, striatal detection of a similar cortical activity pattern initiates responding. While the current data demonstrating striatal involvement in timing behavior provides support for this general interpretation, evaluation of the patterns of activity obtained in this study provide additional clarity regarding the possible roles played by this structure as well as providing some constraints for models of interval timing.
The present study was aimed at assessing whether striatal neurons might represent “abstract” temporal information (Walsh, 2003; van Wassenhove, 2009), divorced from the motor behaviors of the organism. Our results demonstrate that the vast majority of striatal cells cannot be viewed as reflecting the temporal predictability of reinforcement without also demonstrating sensitivity to the motor behaviors involved in obtaining this anticipated reward. In other words, the striatum does not appear to represent time in an “abstract” manner. Specifically, the results of the present study revealed that 99% of those cells showing modulation in hold-related activity as a function of phase had firing rates that were acutely and significantly modified by the overt motor behaviors of the animal in a manner that is inconsistent with a ramp or peak-shaped evolution of activity across the trial. Rather, the striatal activity recorded in the present study showed sensitivity to different behavioral phases and phase transitions, and also showed sensitivity to time within a behavioral phase, but such sensitivity was not reflected by a coherent trial-wide pattern. We note however, that due to trial-by-trial variation in behavioral patterning, neural activity was analyzed in relative rather than absolute time, and it is possible that this analytic approach may have limited our ability to detect coherent temporal patterns. Aside from this concern, given the lack of a coherent trial-wide activity pattern, the response rule(s) utilized for integrating the behavioral and temporal information remains unclear. One possibility is that temporal processing in the striatum is computed in a behavioral state dependent manner, such that the elapsed duration is assessed solely within a single behavioral state. Upon a state transition, the processing of time begins anew. In this scheme, the temporal organization of behavior across the entire trial might occur through striatal and cortical sensitivity to sequential or ordinal components, rather than time, per se (Cromwell and Berridge, 1996; Miyachi et al., 1997; Aldridge and Berridge, 1998; Suri and Schultz, 1998; Tanji, 2001). Indeed, given the interactions between time and sequence (Funahashi et al., 1993; Dominey, 1998; Matell et al., 2006b), studies that compare and contrast these information sources will be necessary to understand their contributions.
Given the striatum’s sensitivity to overt motor behavior, the current results are in conflict with the striatum playing a role as the accumulator in information processing models of interval timing, such as Scalar Timing Theory (SET – Gibbon, 1977) or the Multiple Time Scales model (MTS – Staddon and Higa, 1999). These models specify that the temporal control of the organism is based upon the accumulation or decay of a neural signal that is sequestered from the animal’s behavior. Rather, the behavioral dependence seen here is, in some regards, closer in spirit to the Behavioral Theory of Timing (BET – Killeen and Fetterman, 1988) and a dynamic offshoot, Learning to Time Theory (LET – Machado, 1997), which specify that temporal control is predicated upon transitions in behavioral states. Further, while the present results showing behavioral sensitivity of the recorded neurons are roughly compatible with the proposal that the striatum serves as the decision stage of SET, MTS, and similar behaviorally sequestered models, these neurons cannot be functioning solely as decision stage processors for a single accumulation/decay signal. Rather, monitoring the passage of time appears to be but one of a host of internal and external signals that would be operated upon by the striatum. Similarly, while SBF is generally consistent with the present data in that the cortical activity patterns that are detected by the striatum can include motor cortical patterns that reflect current behavioral states, the finding that temporally modulated neurons are themselves behaviorally sensitive is difficult to reconcile with SBF’s proposal that individual neurons learn a specific cortical activity pattern related to the time of reinforcement (Matell and Meck, 2004).
A related finding from the present work is that the transition periods were more effective at driving activity during the task compared to the nosepoke periods (88% of task-modulated cells had maximally modulated activity during one of the transition phases vs. 12% during either nosepoking or holding the snout within the nosepoke). These data suggest that the behavioral transitions, rather than the nosepoking behaviors which are the most proximately reinforced behaviors, are particularly relevant for driving striatal activity. This finding is at odds with most information-processing based timing models which view the interval between trial onset and the delivery of reinforcement to be the primary piece of temporal information learned by the organism (Gibbon, 1977; Gibbon and Church, 1984; Church and Broadbent, 1991; Staddon and Higa, 1999; Matell and Meck, 2004). As such, neural activity that represents biologically relevant times might be expected to peak at the criterion duration, much as the animal’s responses do (see Figure 3), and several reports have identified maximal neural activity at this time point (Leon and Shadlen, 2003; Matell et al., 2003; Janssen and Shadlen, 2005; Shuler and Bear, 2006; Mita et al., 2009). In contrast, the present data suggest that the striatum may specifically encode the optimal time to begin and end responding, without representing a specific expectation of when reinforcement should arrive. Such transition related activity may be viewed as being consistent with the decision stage of interval timing models (i.e., detecting the times at which a currently elapsed interval is “similar enough” to previously reinforced times to generate responding (e.g., Ivry and Spencer, 2004; Lo and Wang, 2006). However, a simple instantiation of these models would predict that such decision stage activity would continue throughout the FI response phase, rather than being maximal at the onset or offset of this phase. Rather, the present results suggest that the striatum may serve to represent the appropriate time of action to effectively interface with the environment, rather than represent the time of external events which then demand action. These finding again are consistent with aspects of both BET and LET in that these models construe temporal control to result from a sequence of behavioral states, as the “action” in these models is the transition between behavioral states (Killeen and Fetterman, 1988).
While the current findings showed that the large majority of striatal cells had intra-state firing rate modulations, few of these firing patterns were of a theoretically predicted pattern (i.e., peaks or ramps) and none were consistent across all behavioral states (e.g., the few neurons that ramped during the 1st VI period did not continue to ramp or peak during the FI and 2nd VI periods). As with the sensitivity to the overt motor behavior associated with switching between nosepokes, this finding is difficult to reconcile with interval timing models that base temporal control on monotonic- or peak-shaped evolution of an internal clock signal that is sequestered from behavior. While previous investigators have noted ramp- and peak-shaped patterns of cortical activity in interval timing tasks (Kojima and Goldman-Rakic, 1982; Fuster, 1997; Brody et al., 2003; Leon and Shadlen, 2003; Sakurai et al., 2004; Janssen and Shadlen, 2005; Tsujimoto and Sawaguchi, 2005; Mita et al., 2009), these prior results have come from primates required to remain motionless across the entire period in which such activity patterns have been assessed. Thus, while typical interpretations of such data have been that these activity profiles are related to anticipated reinforcement, the current data suggest that they may be related to impending behavioral state changes. Indeed, recent work from our lab has shown a variety of peak and ramp patterns in a premotor cortical region during a steady state response period, but as in the present work, these patterns were not maintained throughout the entire trial (Matell et al., 2011). Conversely, while the behavioral timing models (BET and LET) do not view behavioral states as having meaningful temporal dynamics, which conflicts with the intra-state modulations seen in the present recordings, these models do not require that individual neural states directly map onto discrete behavioral states, but rather propose that some neural states and state transitions might be covert (Killeen and Fetterman, 1988). As such, the current data are consistent with these models. While not modeled as part of SBF, Matell and Meck (2004) suggested that, given the loop-circuitry of the cortico-striatal-thalamic network, it was likely that temporal information arises in a dynamic manner as activity propagates repeatedly through the circuit. In this manner, neural and behavioral state changes could be incorporated into the general framework of SBF.
Conflict of Interest Statement
The authors declare that the research was conducted in the absence of any commercial or financial relationships that could be construed as a potential conflict of interest.
Acknowledgments
This work was supported by NIH grants R03DA18789 and R15DA029809 to Matthew S. Matell.
References
Aldridge, J. W., and Berridge, K. C. (1998). Coding of serial order by neostriatal neurons: a “natural action” approach to movement sequence. J. Neurosci. 18, 2777–2787.
Balsam, P. D., Fairhurst, S., and Gallistel, C. R. (2006). Pavlovian contingencies and temporal information. J. Exp. Psychol. Anim. Behav. Process. 32, 284–294.
Beste, C., Saft, C., Andrich, J., Müller, T., Gold, R., and Falkenstein, M. (2007). Time processing in Huntington’s disease: a group-control study. PLoS ONE 2, e1263. doi: 10.1371/journal.pone.0001263
Bickel, W. K., and Marsch, L. A. (2001). Toward a behavioral economic understanding of drug dependence: delay discounting processes. Addiction 96, 73–86.
Bilkey, D. K., and Muir, G. M. (1999). A low cost, high precision subminiature microdrive for extracellular unit recording in behaving animals. J. Neurosci. Methods 92, 87–90.
Brody, C. D., Hernandez, A., Zainos, A., and Romo, R. (2003). Timing and neural encoding of somatosensory parametric working memory in macaque prefrontal cortex. Cereb. Cortex 13, 1196–1207.
Brunner, D., Kacelnik, A., and Gibbon, J. (1992). Optimal foraging and timing processes in the starling Sturnus vulgaris: effect of intercapture interval. Anim. Behav. 44, 597–613.
Brunner, D., Kacelnik, A., and Gibbon, J. (1996). Memory for inter-reinforcement interval variability and patch departure decisions in the starling, Sturnis vulgaris. Anim. Behav. 51, 1025–1045.
Buhusi, C. V., and Meck, W. H. (2005). What makes us tick? Functional and neural mechanisms of interval timing. Nat. Rev. Neurosci. 6, 755–765.
Chapman, D. E., Keefe, K. A., and Wilcox, K. S. (2003). Evidence for functionally distinct synaptic NMDA receptors in ventromedial versus dorsolateral striatum. J. Neurophysiol. 89, 69–80.
Cheng, K., and Westwood, R. (1993). Analysis of single trials in pigeons timing performance. J. Exp. Psychol. Anim. Behav. Process. 19, 56–67.
Church, R. M., and Broadbent, H. A. (1991). “A connectionist model of timing,” in Neural Network Models of Conditioning and Action eds M. L. Commons, S. Grossberg, and J. E. R. Staddon (New Jersey, NJ: Lawrence Erlbaum Associates), 225–240.
Church, R. M., Meck, W. H., and Gibbon, J. (1994). Application of scalar timing theory to individual trials. J. Exp. Psychol. Anim. Behav. Process. 20, 135–155.
Church, R. M., Miller, K. D., Meck, W. H., and Gibbon, J. (1991). Symmetrical and asymmetrical sources of variance in temporal generalization. Anim. Learn. Behav. 19, 207–214.
Coull, J. T. (2004). fMRI studies of temporal attention: allocating attention within, or towards, time. Cogn. Brain Res. 21, 216–226.
Coull, J. T., Vidal, F., Nazarian, B., and Macar, F. (2004). Functional anatomy of the attentional modulation of time estimation. Science 303, 1506–1508.
Cromwell, H. C., and Berridge, K. C. (1996). Implementation of action sequences by a neostriatal site: a lesion mapping study of grooming syntax. J. Neurosci. 16, 3444–3458.
DeLong, M. R. (1983). The neurophysiologic basis of abnormal movements in basal ganglia disorders. Neurobehav. Toxicol. Teratol. 5, 611–616.
Dietrich, A., Frederick, D. L., and Alien, J. D. (1997). The effects of total and subtotal prefrontal cortex lesions on the timing ability of the rat. Psychobiology 25, 191–201.
Dominey, P. F. (1998). A shared system for learning serial and temporal structure of sensori-motor sequences? Evidence from simulation and human experiments. Cogn. Brain Res. 6, 163–172.
Drew, M. R., Simpson, E. H., Kellendonk, C., Herzberg, W. G., Lipatova, O., Fairhurst, S., Kandel, E. R., Malapani, C., and Balsam, P. D. (2007). Transient overexpression of striatal D2 receptors impairs operant motivation and interval timing. J. Neurosci. 27, 7731–7739.
Ferrandez, A. M., Hugueville, L., Lehéricy, S., Poline, J. B., Marsault, C., and Pouthas, V. (2003). Basal ganglia and supplementary motor area subtend duration perception: an fMRI study. Neuroimage 19, 1532–1544.
Ferster, C. B., and Skinner, B. F. (1957). Schedules of Reinforcement. East Norwalk, CT: Appleton-Century-Crofts.
Funahashi, S., Inoue, M., and Kubota, K. (1993). Delay-related activity in the primate prefrontal cortex during sequential reaching tasks with delay. Neurosci. Res. 18, 171–175.
Fuster, J. M. (1997). The Prefrontal Cortex: Anatomy, Physiology, and Neuropsychology of the Frontal Lobe. Philadelphia: Lippincott-Raven.
Gallistel, C. R., King, A., and McDonald, R. (2004). Sources of variability and systematic error in mouse timing behavior. J. Exp. Psychol. Anim. Behav. Process. 30, 3–16.
Gibbon, J. (1977). Scalar expectancy theory and Weber’s Law in animal timing. Psychol. Rev. 84, 279–325.
Gibbon, J., and Balsam, P. (1981). “Spreading associations in time,” in Autoshaping and Conditioning Theory eds C. M. Locurto, H. S. Terrace, and J. Gibbon (New York, NY: Academic Press), 219–253.
Gibbon, J., and Church, R. M. (1984). “Sources of variance in an information processing theory of timing,” in Animal Cognition, eds H. L. Roitblat, T. G. Bever, and H. S. Terrace (Hillsdale, NJ: Erlbaum), 465–488.
Harrington, D. L., Boyd, L. A., Mayer, A. R., Sheltraw, D. M., Lee, R. R., Huang, M., and Rao, S. M. (2004). Neural representation of interval encoding and decision making. Cogn. Brain Res. 21, 193–205.
Harrington, D. L., and Haaland, K. Y. (1999). Neural underpinnings of temporal processing: a review of focal lesion, pharmacological, and functional imaging research. Rev. Neurosci. 10, 91–116.
Harrington, D. L., Haaland, K. Y., and Hermanowicz, N. (1998). Temporal processing in the basal ganglia. Neuropsychology 12, 3–12.
Ivry, R. B., and Spencer, R. M. (2004). The neural representation of time. Curr. Opin. Neurobiol. 14, 225–232.
Jahanshahi, M., Jones, C. R., Dirnberger, G., and Frith, C. D. (2006). The substantia nigra pars compacta and temporal processing. J. Neurosci. 26, 12266–12273.
Janssen, P., and Shadlen, M. N. (2005). A representation of the hazard rate of elapsed time in macaque area LIP. Nat. Neurosci. 8, 234–241.
Kacelnik, A., and Bateson, M. (1996). Risky theories: the effects of variance on foraging decisions. Am. Zool. 36, 402–434.
Karmarkar, U. R., and Buonomano, D. V. (. (2007) ). Timing in the absence of clocks: encoding time in neural network states. Neuron 53, 427–438.
Killeen, P. R., and Fetterman, J. G. (1988). A behavioral theory of timing. Psychol. Rev. 95, 274–295.
Kojima, S., and Goldman-Rakic, P. S. (1982). Delay-related activity of prefrontal neurons in rhesus monkeys performing delayed response. Brain Res. 248, 43–49.
Komura, Y., Tamura, R., Uwano, T., Nishijo, H., Kaga, K., and Ono, T. (2001). Retrospective and prospective coding for predicted reward in the sensory thalamus. Nature 412, 546–549.
Lejeune, H., Maquet, P., Bonnet, M., Casini, L., Ferrara, A., Macar, F., Pouthasd, V., Timsit-Berthier, M., and Vidal, F. (1997). The basic pattern of activation in motor and sensory temporal tasks: positronemission tomography data. Neurosci. Lett. 235, 21–24.
Leon, M. I., and Shadlen, M. N. (2003). Representation of time by neurons in the posterior parietal cortex of the macaque. Neuron 38, 317–327.
Livesey, A. C., Wall, M. B., and Smith, A. T. (2007). Time perception: manipulation of task difficulty dissociates clock functions from other cognitive demands. Neuropsychologia 45, 321–331.
Lo, C. C., and Wang, X. J. (2006). Cortico-basal ganglia circuit mechanism for a decision threshold in reaction time tasks. Nat. Neurosci. 9, 956–963.
Macar, F., Anton, J. L., Bonnet, M., and Vidal, F. (2004). Timing functions of the supplementary motor area: an event-related fMRI study. Cogn. Brain Res. 21, 206–215.
Macar, F., Vidal, F., and Casini, L. (1999). The supplementary motor area in motor and sensory timing: evidence from slow brain potential changes. Exp. Brain Res. 125, 271–280.
Macdonald, C. J., and Meck, W. H. (2005). Differential effects of clozapine and haloperidol on interval timing in the supraseconds range. Psychopharmacology (Berl.) 1–13.
Malapani, C., Deweer, B., and Gibbon, J. (2002). Separating storage from retrieval dysfunction of temporal memory in Parkinson’s disease. J. Cogn. Neurosci. 14, 311–322.
Matell, M., and Portugal, G. (2007). Impulsive responding on the peak-interval procedure. Behav. Processes 74, 198–208.
Matell, M. S., Bateson, M., and Meck, W. H. (2006a). Single-trials analyses demonstrate that increases in clock speed contribute to the methamphetamine-induced horizontal shifts in peak-interval timing functions. Psychopharmacology (Berl.) 188, 201–212.
Matell, M. S., Berridge, K. C., and Wayne Aldridge, J. (2006b). Dopamine D1 activation shortens the duration of phases in stereotyped grooming sequences. Behav. Processes 71, 241–249.
Matell, M. S., and Meck, W. H. (2000). Neuropsychological mechanisms of interval timing behavior. Bioessays 22, 94–103.
Matell, M. S., and Meck, W. H. (2004). Cortico-striatal circuits and interval timing: coincidence detection of oscillatory processes. Cogn. Brain Res. 21, 139–170.
Matell, M. S., Meck, W. H., and Nicolelis, M. A. (2003). Interval timing and the encoding of signal duration by ensembles of cortical and striatal neurons. Behav. Neurosci. 117, 760–773.
Matell, M. S., Shea-Brown, E., Gooch, C., Wilson, A. G., and Rinzel, J. (2011). A heterogeneous population code for elapsed time in rat medial agranular cortex. Behav. Neurosci. 125, 54–73.
Meck, W. H. (2006). Neuroanatomical localization of an internal clock: a functional link between mesolimbic, nigrostriatal, and mesocortical dopaminergic systems. Brain Res. 1109, 93–107.
Meck, W. H., and Church, R. M. (1982). Discrimination of intertrial intervals in cross-modal transfer of duration. Bull. Psychon. Soc. 19, 234–236.
Miller, R. R., and Barnet, R. C. (1993). The role of time in elementary associations. Curr. Dir. Psychol. Sci. 2, 106–111.
Mita, A., Mushiake, H., Shima, K., Matsuzaka, Y., and Tanji, J. (2009). Interval time coding by neurons in the presupplementary and supplementary motor areas. Nat. Neurosci. 12, 502–507.
Miyachi, S., Hikosaka, O., Miyashita, K., Kárádi, Z., and Rand, M. K. (1997). Differential roles of monkey striatum in learning of sequential hand movement. Exp. Brain Res. 115, 1–5.
Nagarajan, S. S., Blake, D. T., Wright, B. A., Nancy, B., and Merzenich, M. M. (1998). Practice-related improvements in somatosensory interval discrimination are temporally specific but generalize across skin location, hemisphere, and modality. J. Neurosci. 18, 1559–1570.
Nakano, K., Kayahara, T., Tsutsumi, T., and Ushiro, H. (2000). Neural circuits and functional organization of the striatum. J. Neurol. 247(Suppl. 5), V1–V15.
Nenadic, I., Gaser, C., Volz, H. P., Rammsayer, T., Häger, F., and Sauer, H. (2003). Processing of temporal information and the basal ganglia: new evidence from fMRI. Exp. Brain Res. 148, 238–246.
Nisenbaum, E. S., Orr, W. B., and Berger, T. W. (1988). Evidence for two functionally distinct subpopulations of neurons within the rat striatum. J. Neurosci. 8, 4138–4150.
Penney, T. B., Meck, W. H., Roberts, S. A., Gibbon, J., and Erlenmeyer-Kimling, L. (2005). Interval-timing deficits in individuals at high risk for schizophrenia. Brain Cogn. 58, 109–118.
Perry, J. L., and Carroll, M. E. (2008). The role of impulsive behavior in drug abuse. Psychopharmacology (Berl.) 200, 1–26.
Pfeuty, M., Ragot, R., and Pouthas, V. (2005). Relationship between CNV and timing of an upcoming event. Neurosci. Lett. 382, 106–111.
Pouthas, V., George, N., Poline, J. B., Pfeuty, M., Vandemoorteele, P. F., Hugueville, L., Ferrandez, A. M., Lehéricy, S., Lebihan, D., and Renault, B. (2005). Neural network involved in time perception: an fMRI study comparing long and short interval estimation. Hum. Brain Mapp. 25, 433–441.
Rao, S. M., Mayer, A. R., and Harrington, D. L. (2001). The evolution of brain activation during temporal processing. Nat. Neurosci. 4, 317–323.
Roberts, S. (1981). Isolation of an internal clock. J. Exp. Psychol. Anim. Behav. Process. 7, 242–268.
Roberts, S., and Holder, M. D. (1984). What starts an internal clock? J. Exp. Psychol. Anim. Behav. Process. 10, 273–296.
Sakurai, Y., Takahashi, S., and Inoue, M. (2004). Stimulus duration in working memory is represented by neuronal activity in the monkey prefrontal cortex. Eur. J. Neurosci. 20, 1069–1080.
Schultz, W., and Romo, R. (1988). Neuronal activity in the monkey striatum during the initiation of movements. Exp. Brain Res. 71, 431–436.
Shuler, M. G., and Bear, M. F. (2006). Reward timing in the primary visual cortex. Science 311, 1606–1609.
Staddon, J. E. R., and Higa, J. J. (1999). Time and memory: towards a pacemaker-free theory of interval timing. J. Exp. Anal. Behav. 71, 215–251.
Stevens, M. C., Kiehl, K. A., Pearlson, G., and Calhoun, V. D. (2007). Functional neural circuits for mental timekeeping. Hum. Brain Mapp. 28, 394–408.
Suri, R. E., and Schultz, W. (1998). Learning of sequential movements by neural network model with dopamine-like reinforcement signal. Exp. Brain Res. 121, 350–354.
Swanton, D. N., Gooch, C. M., and Matell, M. S. (2009). Averaging of temporal memories by rats. J. Exp. Psychol. Anim. Behav. Process. 35, 434–439.
Tanji, J. (2001). Sequential organization of multiple movements: involvement of cortical motor areas. Annu. Rev. Neurosci. 24, 631–651.
Tregellas, J. R., Davalos, D. B., and Rojas, D. C. (2006). Effect of task difficulty on the functional anatomy of temporal processing. Neuroimage 32, 307–315.
Tsujimoto, S., and Sawaguchi, T. (2005). Neuronal activity representing temporal prediction of reward in the primate prefrontal cortex. J. Neurophysiol. 93, 3687–3692.
van Wassenhove, V. (2009). Minding time in an amodal representational space. Philos. Trans. R. Soc. Lond., B, Biol. Sci. 364, 1815–1830.
Keywords: interval timing, striatum, electrophysiology, time perception, rats
Citation: Portugal GS, Wilson AG and Matell MS (2011) Behavioral sensitivity of temporally modulated striatal neurons. Front. Integr. Neurosci. 5:30. doi: 10.3389/fnint.2011.00030
Received: 25 February 2011;
Paper pending published: 16 March 2011;
Accepted: 27 June 2011;
Published online: 12 July 2011.
Edited by:
Warren H. Meck, Duke University, USAReviewed by:
Hugo Merchant, Universidad Nacional Autónoma de México, MexicoMark Laubach, The John B. Pierce Laboratory, USA
Copyright: © 2011 Portugal, Wilson and Matell. This is an open-access article subject to a non-exclusive license between the authors and Frontiers Media SA, which permits use, distribution and reproduction in other forums, provided the original authors and source are credited and other Frontiers conditions are complied with.
*Correspondence: Matthew S. Matell, Department of Psychology, Villanova University, 800 Lancaster Ave, Villanova, PA 19085, USA. e-mail: matthew.matell@villanova.edu