- 1 Sobell Department of Motor Neuroscience and Movement Disorders, Institute of Neurology, University College London, London, UK
- 2 Laboratory of Neuropsychology, National Institute of Mental Health, National Institutes of Health, Bethesda, MD, USA
A growing body of evidence suggests that the midbrain dopamine system plays a key role in reinforcement learning and disruption of the midbrain dopamine system in Parkinson’s disease (PD) may lead to deficits on tasks that require learning from feedback. We examined how changes in dopamine levels (“ON” and “OFF” their dopamine medication) affect sequence learning from stochastic positive and negative feedback using Bayesian reinforcement learning models. We found deficits in sequence learning in patients with PD when they were “ON” and “OFF” medication relative to healthy controls, but smaller differences between patients “OFF” and “ON”. The deficits were mainly due to decreased learning from positive feedback, although across all participant groups learning was more strongly associated with positive than negative feedback in our task. The learning in our task is likely mediated by the relatively depleted dorsal striatum and not the relatively intact ventral striatum. Therefore, the changes we see in our task may be due to a strong loss of phasic dopamine signals in the dorsal striatum in PD.
Introduction
It has long been hypothesized that the basal ganglia have a specific role in sequential motor control (Marsden, 1982) and to examine this both explicit and implicit sequence learning have been studied in PD. The majority of studies have focused on implicit learning as this type of learning is thought to depend on intact basal ganglia function (Seidler et al., 2005; Squire, 2009). Many studies have shown impairment in implicit sequence learning in PD (Deroost et al., 2006; Wilkinson and Jahanshahi, 2007; Wilkinson et al., 2010) and some studies have also found impairment in explicit learning in PD (Ghilardi et al., 2003; Carbon et al., 2004; Shohamy et al., 2005; Nagy et al., 2007). However, other studies have shown intact sequence learning in PD (Smith et al., 2001; Shin and Ivry, 2003).
Recently, work has also suggested a role for the basal ganglia and especially dopamine in reinforcement learning (RL), or learning from positive and negative feedback (Schultz et al., 1997; Schultz and Dickinson, 2000; Pessiglione et al., 2006). Consistent with this, disruption of the midbrain dopamine system in Parkinson’s disease (PD) affects performance on RL tasks (Frank et al., 2004; Shohamy et al., 2005; Cools et al., 2006; Wilkinson et al., 2008; Jahanshahi et al., 2009). For example, previous work has shown that patients “ON” medication learn more from positive feedback and less from negative feedback, and patients “OFF” medication have the opposite learning profile (Frank et al., 2004; Cools, 2006; Rutledge et al., 2009).
Thus, work has suggested a role for the striatum in sequence learning and RL. However, the implicit and explicit sequence learning paradigms which have been developed previously do not directly test RL mechanisms. The aim of the present study was to examine sequence learning within a RL framework. Thus, we have developed a task in which subjects had to learn sequences of button presses using positive and negative feedback, where the feedback is stochastic. Specifically, the correct action is not always followed by positive feedback and the incorrect action is not always followed by negative feedback. Thus, subjects have to integrate feedback across trials to infer the correct sequence of actions.
Previous studies of RL in PD have utilized tasks in which participants associated a value to a visual image (Frank et al., 2004; Cools et al., 2007; Bodi et al., 2009; Rutledge et al., 2009), and therefore these tasks have likely engaged ventral striatal mechanisms (Cools et al., 2007). In contrast to this, our task likely engages the dorsal striatum, as it requires subjects to learn sequences of actions, and previous functional imaging (Jueptner et al., 1997; Wachter et al., 2009) and PET (Badgaiyan et al., 2007, 2008) studies have shown that the dorsal striatum is important for motor sequence learning. This functional distinction between the dorsal and ventral striatum is also consistent with the known anatomy, as the ventral striatum receives visual inputs, whereas the dorsal striatum receives motor inputs (Alexander et al., 1986; Haber et al., 2000). In early PD the dorsal striatum is relatively depleted of dopamine whereas the ventral striatum is relatively intact (Kish et al., 1988). Therefore, phasic dopamine may be lower in the dorsal striatum relative to the ventral striatum, whereas tonic dopamine may be less affected, as has been shown with cyclic voltammetry in rats with partial 6-OHDA lesions (Garris et al., 1997). This difference in the level of dopamine innervation suggests that the effects of dopamine and PD on RL performance in our task may differ from the effects seen in previous studies.
Materials and Methods
Participants
Non-demented and non-depressed patients with a clinical diagnosis of Parkinson’s disease according to the UK Brain Bank criteria (Hughes et al., 1992) participated (Table 1).
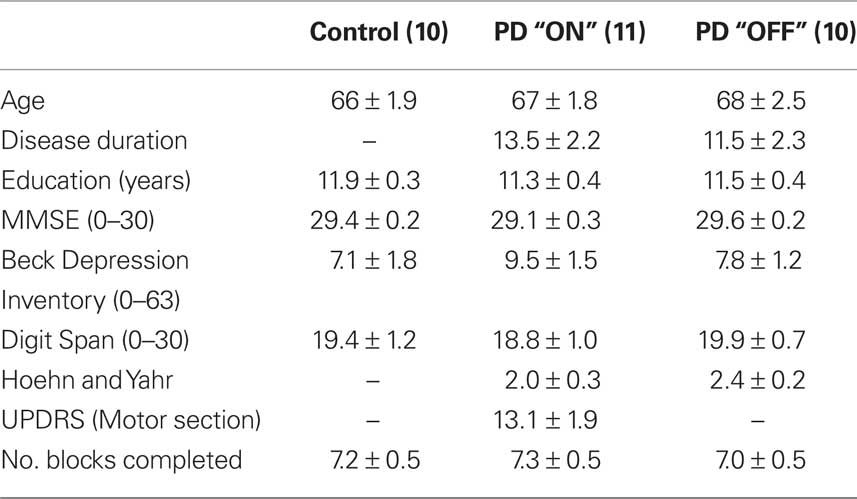
Table 1. Demographic information for all participants and clinical characteristics of patients (mean ± SEM are shown). UPDRS scores were not obtained for the PD “OFF” group.
Fourteen PD patients “ON” (two female) and 10 PD patients “OFF” (no female) their levodopa (L-DOPA) medication were compared to 10 age-matched healthy controls (five female). The study was approved by the Joint Ethics Committee of the Institute of Neurology and the National Hospital for Neurology and Neurosurgery and informed consent was obtained from all participants. Unified Parkinson’s Disease Rating Scale (UPDRS) was used to assess the PD “ON” group and Hoehn and Yahr Scale (Hoehn and Yahr, 1967) was used to assess disease severity and stage of illness in both the PD “ON” and “OFF” groups. Participants in this study were in the mild to moderate stages of the disease. All participants were screened for dementia and clinical depression on the Mini-Mental State Examination (MMSE) (Folstein et al., 1975) and Beck Depression Inventory (BDI) (Beck et al., 1961). All participants were non-demented as indicated by scores >27 and none were suffering from clinical depression as indicated by scores <18. Attention and working memory was assessed using the Digit Span Task (Wechsler, 1997). Three patients had to be excluded in the “ON” medication group: Two of the medicated patients (two male) failed to complete the task (minimum four sets) and another medicated patient (one female) was later found to be on dopamine agonists and was excluded retrospectively (see Table 1 for a summary of demographic information for PD patients and controls). Thus, all included patients were taking only L-DOPA. Patients tested “OFF” refrained from taking their L-DOPA medication overnight and were tested a minimum of 12 h after the cessation of medication (mean duration of medication withdrawal = 14.65 h, SD = 1.59 h, range = 5 h). Six PD patients were assessed first “ON” and then “OFF” their medication. To minimize any potential “carry over” effects, for this subgroup, the average interval between testing “ON” and “OFF” medication was 9 ± 1.7 months. We carried out all statistical analyses using between subjects models as these are the most conservative because they cannot factor out between subject variability.
Procedure and Task
Participants were given instructions on the task prior to beginning and also one full practice block to familiarize them with the components of the task. During the task they were seated comfortably in front of a computer monitor with their hands resting on a table. A box with two buttons was placed in front of them and they were required to make a sequence of four button presses with their left and right hands, for example: left, right, left, right (LRLR). They did not have to move their arms to execute a button press. Each trial began with the presentation of a green outline circle at the center of the screen which cued the participants to execute a movement (Figure 1A).
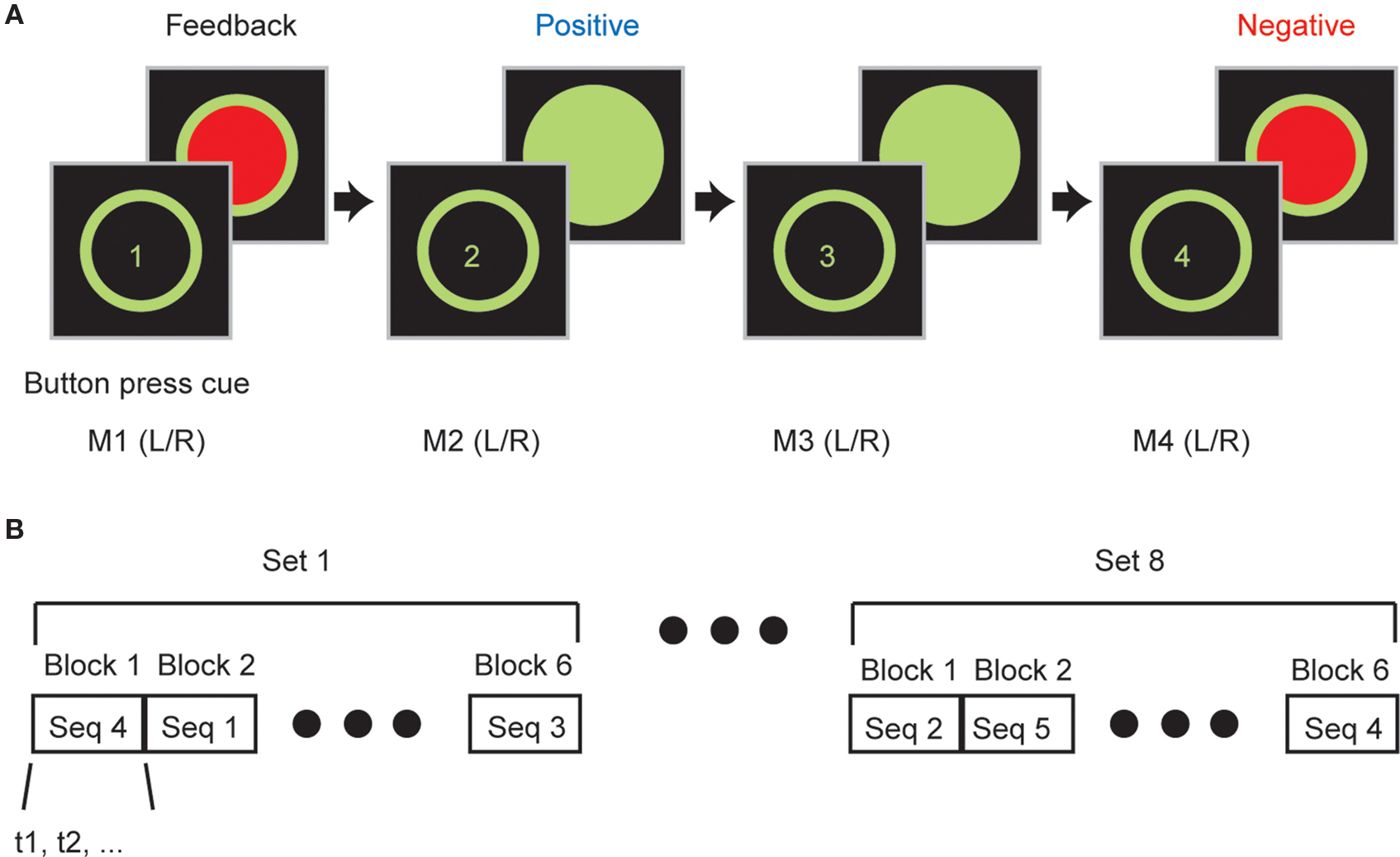
Figure 1. Sequence learning task. (A) Participants executed a sequence of four button presses using combinations of left and right handed button presses. After each button press they were given feedback about whether or not they were correct. (B) Participants completed multiple sets of trials. In each set participants executed all six sequences (S1, S2,…, S6) and sequences were executed in blocks in which participants had to learn and then execute the sequence correctly eight times before advancing to the next sequence in the block. Sequence blocks were presented in pseudo-random order.
They responded by executing a button press with either their left or right index finger. Response time was not constrained, so participants could take as long to react as they liked. After each response, they were given feedback for 750 ms (green or red) about whether or not they had pressed the correct left or right button for that movement in the sequence. This was repeated four times, such that in each trial a sequence was composed of four button presses, with each button press followed by feedback. There was a 1-s inter-trial interval between trials, during which time the screen was blank. Importantly, as the feedback was stochastic, on 15 percent of the button presses the participants were given inconsistent feedback. Specifically, if they had pressed the correct button they were given red feedback and if they had pressed the incorrect button they were given green feedback. Thus, the feedback from any individual button press did not necessarily allow the participants to correct their mistakes but an integration of feedback from multiple trials was required to determine the correct sequence.
We used 6 of the 16 possible sequences (i.e., four button presses with either left or right index fingers gives 16 possible sequences), which were balanced for first order button press probabilities, containing at least two left (LL) and two right (RR) button presses. The sequences used were LLRR, RRLL, LRLR, RLRL, LRRL and RLLR. Each participant executed an average of seven sets of trials (Table 1). In each set all six sequences were executed in blocks presented in a pseudo-random order (Figure 1B). In each block participants were required to learn and then execute one of the sequences eight times correctly (at least four times consecutively) before they advanced to the next sequence (next block) in the set. If they failed to reach criterion by 20 trials they were advanced to the next sequence. Consistent with the learning data presented in results which show that PD “ON” are worse than PD “OFF” which were worse than controls, we found that PD “ON” failed to complete 121/480 total blocks, PD “OFF” failed to complete 55/414 total blocks and controls failed to complete 38/480 total blocks. The control performance is consistent with findings in our previous study (Averbeck et al., 2010), in which healthy, younger participants failed to complete about 9% of blocks. In addition, as mentioned above, participants received one full block of training before the data collection session to familiarize them with the mechanics, the sequences and the stochastic feedback of the task. Thus, it is not likely that differences in performance were due to difficulties understanding the arbitrary mapping between feedback and button presses.
Behavioral Model
We fit Bayesian statistical models to the trial-by-trial behavior of individual participants. The models allowed us to quantify trial-by-trial how much the participants had learned about which sequence or which button was correct in the current block. This model is related to reinforcement learning (RL) models, which are often used to model this type of behavior (Sutton and Barto, 1998). However, RL models estimate values, which are not action probabilities. The values in RL models are then passed through logistic or soft-max function to generate action probabilities, and the soft-max functions require an extra temperature parameter to be fit. In this respect, the Bayesian model we use is more direct, as it does not require us to fit a temperature parameter. The Bayesian model allows us to estimate how much positive feedback for a given button press (i.e., a green circle) increases the probability that subjects will press the same button in future trials, as well as how much negative feedback (i.e., a red circle) decreases the probability that subjects will press the same button in future trials. Thus, the model gives us a tool to estimate overall learning rates parametrically, because learning can be equated to the effect of feedback on future button presses. It also gives us the ability to split learning into the effects of positive and negative feedback.
The participants could press either the left or right button at each point in the sequence and therefore they had a binary decision. The model assumed that the participants were trying to learn the sequence, and, therefore, that they were trying to optimize the number of times green feedback was received. Statistically, this can be accomplished by remembering how often green feedback was given for the left (or right) button at each point in the sequence across multiple trials. For example, if green feedback was given more often for the left button for the first movement, then the left button should be pressed. Thus, the model integrates information about red vs. green feedback given for left and right button presses individually for each of the four button presses in the sequence.
The model began with a binomial likelihood function for each movement of the sequence, given by:

where θi,j is the probability that pressing button i (i ∈{left,right}) on movement j (j ∈{1, …, 4}) would be followed by green feedback. The variable ri,j, defined below, is the number of times reward (green feedback) was given when button i was pressed on movement j (or red feedback was given when the other button was pressed), and Nj is the number of trials. The vector DT represents all the data collected up to trial T for the current block, which in this case are the values of r and N. This was the only data relevant to inferring the correct sequence of button presses. Importantly, the model does not contain any information about previous sequences from the current set. Participants were not told that all sequences were given in each set, and therefore it was unlikely that they would be able to infer set boundaries and use this information to improve learning, by estimating which sequences they had not yet executed.
The probability that the left button should be pressed for movement j after T trials (i.e., that it is more likely to be the correct button) is given by:

We have written the posterior here (i.e., p(θright,j | DT)). Button probabilities were equally likely in the experiment so the prior was flat and the posterior is just the normalized likelihood for this estimate.
In order to better predict participant behavior we added two parameters to the basic model that allowed for differential weighting of positive and negative feedback. The differential weighting was implemented by using the following equation for the feedback:

The subscripts positive and negative indicate whether the feedback was positive (green) or negative (red). The total reward (feedback), in Eq. 1 was then given by:

The parameter u(t) is one if green feedback was given and zero if red feedback was given on trial t. Thus, α and β scale the amount that is learned from positive and negative feedback. For an ideal observer both parameters would be 0.5. The parameters, α and β were fit to individual participant decision data by maximizing the likelihood of the participant’s sequence of decisions, given the model parameters. Thus, we maximized:

where Ct is the choice that the participant made for each movement at time t (Ct = 1 for left, Ct = 0 for right) and D* indicates the vector of decision data with elements Ct. Here t iterates over the data without explicitly showing sequence boundaries, as this is how the data is analyzed. This function was maximized using non-linear function maximization techniques in Matlab. We also utilized multiple starting values for the parameters (−0.1, 0, 0.1) to minimize the effects of local minima.
ANOVAs were carried out on the learning parameters to compare groups. We fit a mixed effects ANOVA, with a random effect of subject nested under group (“ON”, “OFF”, control). The two learning parameters (positive (α) and negative (β)) were treated as fixed, repeated measures within subjects. Post hoc comparisons were carried out using Tukey’s HSD test, as indicated. A similar ANOVA was carried out on the fraction correct behavioral performance, except correct block trials was the repeated measure. When separate ANOVAs were carried out on positive and negative feedback terms, we did not correct for multiple comparisons. However, it can be seen that the reported p-values would be significant even under Bonferroni correction.
Models Fit to Evolution of Learning Parameters
We fit parameterized functions to the learning parameters extracted from the model using an increasing number of trials across the block. Visual inspection of the data suggested that the positive parameters would be fit well by a logistic function and the negative parameters by an inverse exponential function. The logistic function is given by:

The free parameters in this model were a, b, c and d. We modeled the logistic curve for the positive feedback parameter across groups with a common amplitude (b), inflection point (c) and slope (d), but different intercepts (a), as the intercept was the term which varied across groups.
The inverse exponential function is given by:

The free parameters in this model were f, g and h. Parameter h was constant across groups, and parameters f and g were common to controls and patients off, but separate for patients on medication, which captured the group differences. Both models were fit using fminsearch in Matlab, such that the sum of the squared error between the predicted parameter values, vi(k) and the actual parameter values were minimized.
Results
We tested PD patients “ON” and “OFF” levodopa and healthy controls and examined whether learning discrepancies related to different feedback processing between the three groups. There was no difference between the three groups (PDON, PDOFF and controls) in age (F2, 28 = 0.35, p = 0.70), education (F2, 28 = 0.78, p = 0.78) or digit span (F2, 28 = 0.35, p = 0.71). There was also no difference in disease duration between the PDON and PDOFF groups (F1, 19 = 0.45, p = 0.51).
When we assessed performance in the sequence learning task we found there were differences in the learning curves between the groups (Figure 2). Overall, we found that PD patients tested “ON” medication performed the worst, followed by the “OFF” medication group, with the healthy controls performing the best. In general, for all three groups, performance reached a plateau on average by three correct trials. An ANOVA showed a main effect of group (F2, 28 = 4.83, p = 0.016), a main effect of block position (F8, 224 = 302.59, p < 0.001) and a group by block position interaction (F16,224 = 2.35, p = 0.003).
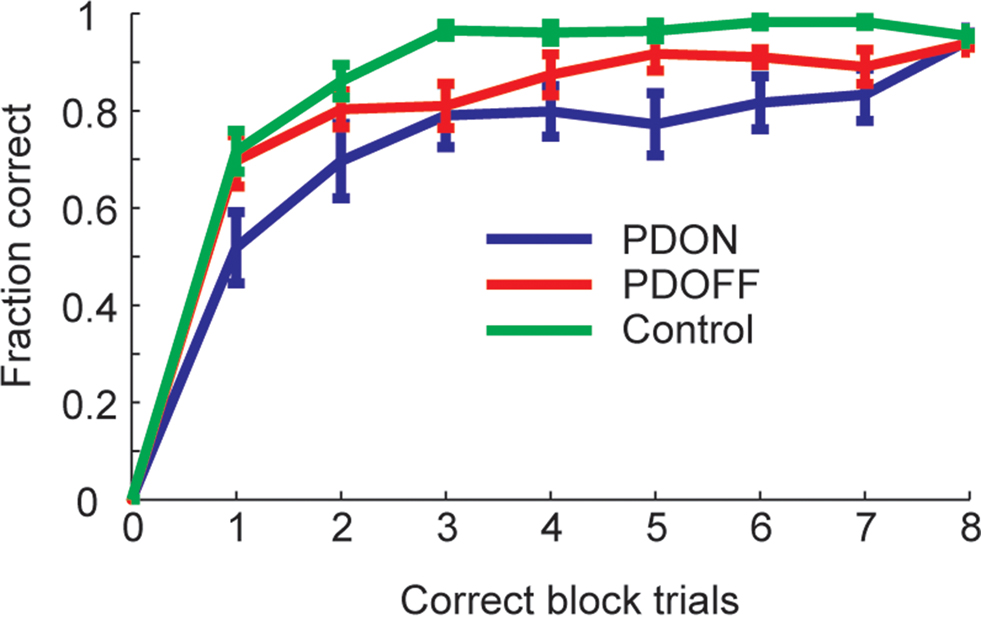
Figure 2. Average learning curves in PD groups and control across the whole block. Error bars are ±1 sem.
We next fit a model to the data to compare statistically the differential effects of positive and negative feedback. Analyses among the three groups were carried out using the parameter estimates derived from the Bayesian learning model (see Materials and Methods). Larger parameter values indicate that participants made subsequent decisions that more strongly reflected the corresponding feedback. Negative values indicate that participants were not responding appropriately to the corresponding feedback, and values near zero indicate no effect of the feedback on subsequent choices.
The task required participants to learn the sequence and then subsequently execute it several times correctly before a new sequence was introduced. Therefore, it is likely that participants utilized the feedback information differently as the block evolved. We examined this by first fitting the model to the subset of trials before the first correct trial from the block, which would be the early learning trials (Figure 3A). We found a significant main effect of valence (i.e., positive vs. negative feedback which correspond to α and β from Eq. 3; F1,28 = 305.84, p < 0.001) and a significant interaction between valence and group (F2,28 = 4.91, p = 0.015). There was, however, no significant main effect of group. Post hoc comparisons on the interaction term (Figure 3D) showed that there was a significant difference between PDON and controls (Tukey’s HSD; p < 0.05) but not between PDOFF and either PDON or controls. We followed this up with separate one-way ANOVAs on the positive and negative feedback parameters. For positive feedback there was a significant effect of group (F2, 28 = 4.42, p = 0.021) and post hoc comparisons on this one-way ANOVA to examine which groups were significantly different showed a significant difference between PDON and controls (Tukey’s HSD; p < 0.05) but not between PDON and PDOFF (Tukey’s HSD; p > 0.05) or PDOFF and controls (Tukey’s HSD; p > 0.05). For negative feedback, however, there was no significant effect of group on early learning (F2, 28 = 1.45, p = 0.251).
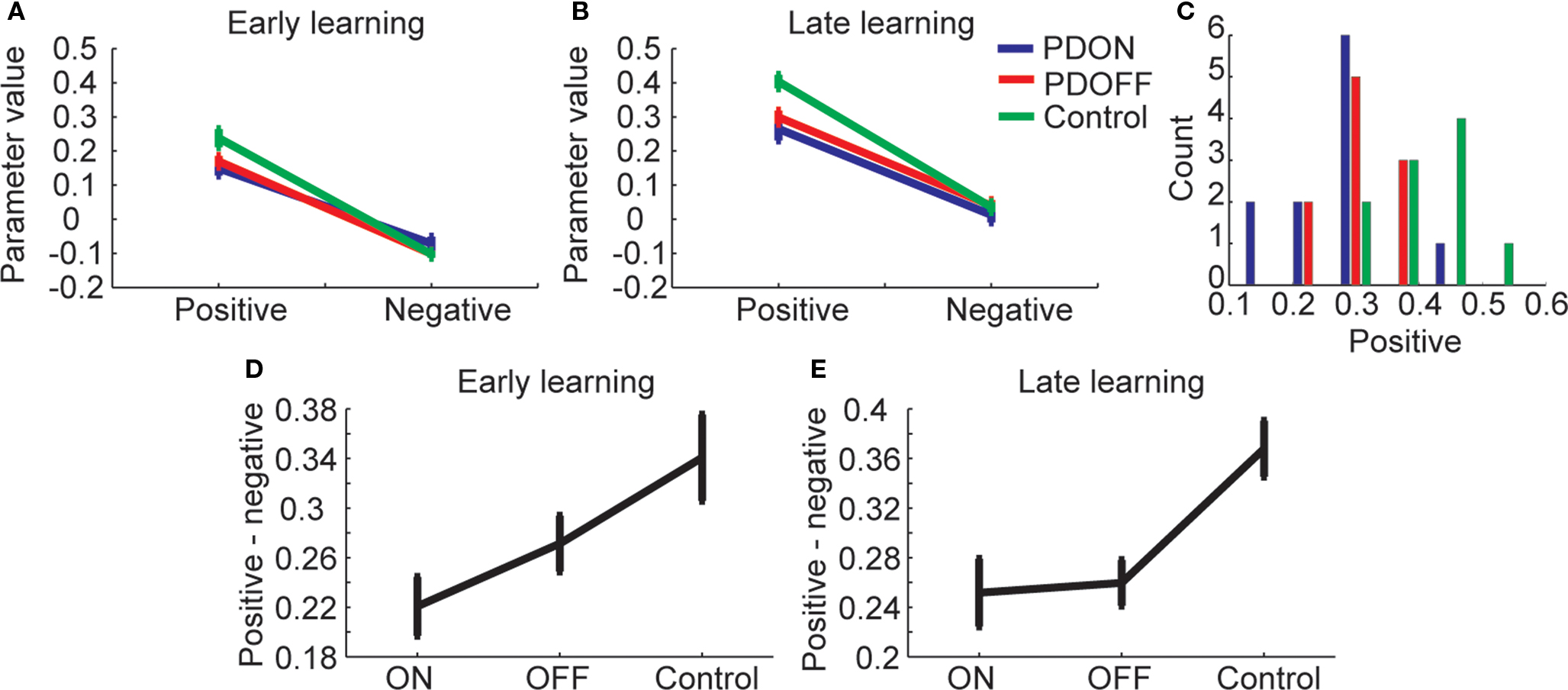
Figure 3. Learning from positive and negative feedback. (A) Parameters from a model fit to the subset of trials before the first correct trial from each block. Positive is parameter α from Eq. 3 and negative is parameter β from Eq. 3. (B). The model fit to the trials from the entire block. (C) Distribution of positive feedback parameter across subjects within each group. (D) Within participant differences in learning from positive and negative feedback for trials before first correct (compare with A). (E) Same as (D) for all data from block (compare with B).
Next, we examined the parameters from the model when it was fit to the data from the entire block (Figure 3B). We found a significant main effect of valence (F1,28 = 467.79, p < 0.001) a significant main effect of group (F2,28 = 4.28, p = 0.024) and a significant interaction between valence and group (F2,28 = 7.61, p = 0.002). The distribution of the positive feedback parameter across groups (Figure 3C) showed that while there was clear separation of the means, there was also overlap between groups. Post hoc comparisons on the interaction between group and valence (Figure 3E) showed that PDON and PDOFF were significantly different than controls (Tukey’s HSD; p < 0.05) but PDON and PDOFF were not significantly different than each other. A follow-up one-way ANOVA run on positive feedback showed a significant effect of group (F2, 28 = 7.49, p = 0.003) and post hoc comparisons showed that the group PDON and PDOFF were significantly different than controls (Tukey’s HSD; p < 0.05), but PDOFF and PDON were not significantly different (Tukey’s HSD; p > 0.05). For negative feedback there was again no significant effect of group (F2, 28 = 0.59, p = 0.562).
The parameters from the learning model reflect the learning, which is also reflected in the basic behavior (Figure 2). To tie these together, we correlated, across participants from all three groups, the average number of trials it took them to complete a single sequence block and learning from positive and negative feedback. We first carried this out for early trials, in which we correlated the number of trials it took participants to get one correct with the parameters estimated from the early learning trials (Figure 3A). We found that there was a significant correlation between number of trials with positive (r29 = −0.394, p = 0.028) but not negative (r29 = 0.023, p = 0.902) learning parameters. When we carried out the same analysis for the data from the entire block, we found that there was a significant correlation for both positive (r29 = −0.775, p < 0.001) and negative (r29 = −0.470, p = 0.008) learning parameters. Thus, the learning parameters captured the behavioral performance of the participants and showed that early learning was more strongly correlated with positive feedback and late learning was correlated with both positive and negative feedback.
Both the model parameters and the correlations with primary behavioral performance suggested that participants employed a varying strategy across the block to learn. To examine these strategies in more detail, we fit the model nine times, each time including data up to the next number of correct trials per block. In other words, we first fit the model with all trials before the first correct trial (equivalent to 3A, plotted as 0 correct block trials in Figures 4A,B). Next we fit the model with all trials before the second correct trial (plotted as one correct block trials in Figures 4A,B). We continued this process until we had fit the model to all of the data from each block. We found a smooth evolution of the model parameters as the block evolved. The positive parameter was initially positive and followed a logistic curve as the block evolved (Figure 4A). We found that the intercept was significantly different across groups (F2,272 = 49.4, p < 0.05).
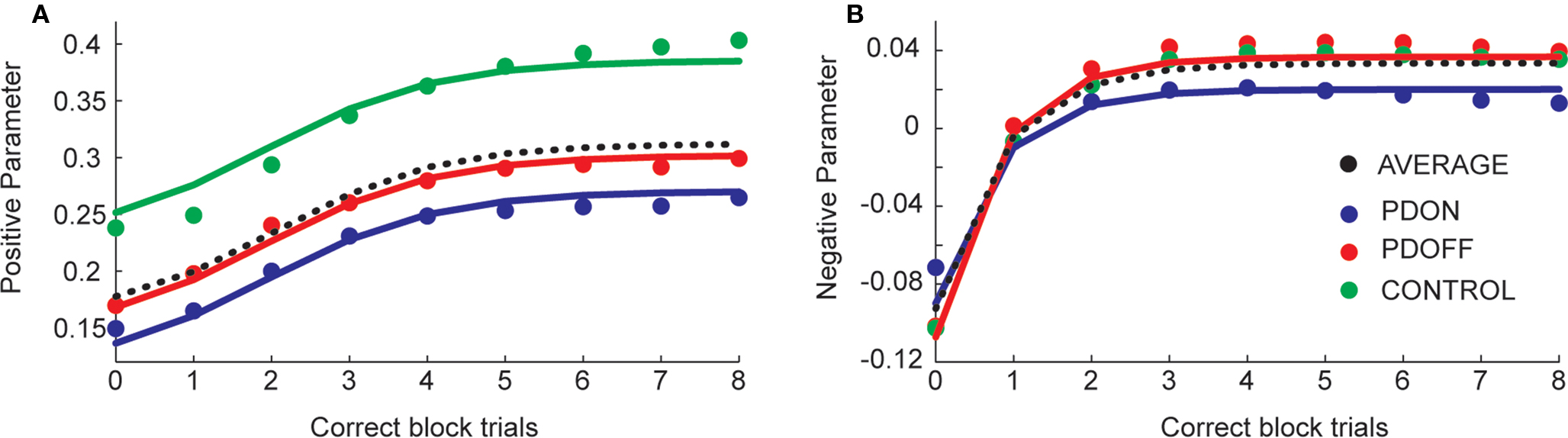
Figure 4. Evolution of positive (A) and negative (B) feedback parameter values as the block evolved. Dotted black lines show the average across the groups. Solid lines are model estimates, large dots are data averaged across participants within each group.
The negative parameter was initially negative, but increased to zero and then to positive values (Figure 4B). Interestingly, it was zero at the first correct trial, which might give some insight into the strategy participants were using. We modeled the evolution using an inverse exponential function (see Materials and Methods). There was an interesting flip in this case, such that the “ON” patients had an initially less negative effect of the parameter which then became less positive (reflecting an overall decrease in their performance similar to that noted above). We examined a model which looked specifically for a difference between “ON” patients and the other two groups, and found that patients “ON” medication had a significantly different intercept and slope (F2,273 = 3.4, p < 0.05).
To examine effects of disease severity and the non-specific factor of working memory performance on learning, we examined correlations between the two model parameters, learning from positive and negative feedback, and the demographic variables disease duration and digit span within the on and off groups and across groups (Table 2). The correlation between learning from positive feedback and disease duration showed a trend towards significance in the PDON group (r9 = −0.59; p = 0.058) and, across PD groups there was a significant correlation between learning from positive feedback and disease duration (r19 = −0.49; p = 0.025). Neither of these would, however, have survived correction for multiple comparisons. There were no other significant or trend correlations.
Discussion
In the present study we examined sequence learning with stochastic feedback in a control group and PD patients “ON” and “OFF” their dopamine replacement therapy. We found that PD patients tested “ON” medication performed significantly worse than controls, whereas PD “OFF” did not differ significantly from PD “ON” or controls. When we parameterized these effects and examined early learning, we found significant differences between PDON and controls, but not between PDON and PDOFF or between PDOFF and controls. When we examined learning across the entire block, however, both PDON and PDOFF were significantly different than controls, but not significantly different than each other. The difference between PDON, PDOFF and controls was due to differences in learning from positive feedback. When we considered how the learning rate parameters evolved across the block, we found a significant difference between PD “ON” and controls and PD “OFF” in the negative feedback term.
Sequence Learning and Reinforcement Learning
The goal of our experiment was to bring together reinforcement learning and sequence learning, both of which have been studied in PD, but not together. An extensive literature has focused on implicit sequence learning in PD, where implicit refers to incidental acquisition of sequential movements by practice without conscious awareness (Cohen and Squire, 1980). The serial reaction time (SRT) task (Nissen and Bullemer, 1987) has been the most frequently used paradigm for testing implicit learning in PD, as implicit learning is thought to depend on an intact basal ganglia (Nissen and Bullemer, 1987). To date most studies have only tested PD patients “ON” medication and found reduction in implicit sequence learning, where PD participants were able to learn sequence-specific knowledge but to a lesser extent than controls (Brown et al., 2003; Shin and Ivry, 2003; Werheid et al., 2003; Frank et al., 2004; Kelly et al., 2004; Smith and McDowall, 2004; Deroost et al., 2006; Seidler et al., 2007; Wilkinson and Jahanshahi, 2007; Wilkinson et al., 2009). There has also been a study that specifically examined the effect of medication on explicit sequential association learning where impairments were found in the “OFF” medication group while “ON” medication patients performed as well as the controls (Shohamy et al., 2005). Additionally, a recent study has shown that patients “ON” learn the least in the SRT whereas patients “OFF” learn better and controls learn best (Kwak et al., 2010). Imaging studies have also shown that during explicit sequence learning in PD there is underactivation in the caudate, putamen and DLPFC when PD patients were compared to controls (Nakamura et al., 2001; Carbon et al., 2003, 2004; Lehericy et al., 2005).
Work on reinforcement learning (RL) or learning from positive and negative feedback, motivated by findings that suggest that dopamine neurons signal reward prediction errors (Schultz et al., 1997) has shown that dopaminergic replacement therapy improves learning from positive feedback but impairs learning from negative feedback, while withdrawal from anti-Parkinsonian medication leads to the reverse profile (Frank et al., 2004; Cools et al., 2006; Bodi et al., 2009; Rutledge et al., 2009; Voon et al., 2010), although these studies do not agree completely on all effects of medication on positive and negative learning.
Our task allowed us to bring together the results showing deficits in sequence learning, which have not been studied in patients by splitting apart responses to positive and negative feedback, and the results which suggest that dopamine replacement therapy affects RL mechanisms. We found an effect of medication status on learning from positive and negative feedback. However, we found that both PD patients “ON” and “OFF” learned less than healthy controls from positive feedback. Overall, participants from all groups in our experiment showed greater learning from positive than negative feedback. When we examined the temporal evolution of the learning, we found that participants initially showed a negative impact of negative feedback, which may be related to an explorative strategy, as participants would be inclined to ignore some negative feedback early on while they were attempting to determine which sequence was correct.
Learning the Value of Actions or Images from Positive and Negative Feedback
We found that subjects learned less from positive feedback, whereas previous studies have shown that patients either learned less from negative feedback and/or more from positive feedback (Frank et al., 2004; Cools et al., 2006; Bodi et al., 2009; Rutledge et al., 2009; Voon et al., 2010). We do not believe the lack of an effect of medication on learning from negative feedback in our task is a result of a lack of power, because we show a substantial effect on learning from positive feedback in our group, and thus it is not an underpowered study. It is always possible that an effect would emerge with a larger group of participants, but the effect would be necessarily of smaller magnitude than the effect we see on learning from positive feedback. Furthermore, our basic behavioral finding, showing deficits in learning which are strongest for patients “ON” medication is consistent with a recent finding in patients “ON” medication in the SRT task (Kwak et al., 2010).
The differences between learning from positive and negative feedback in our task relative to previous studies may be due to differences between the neural substrate that underlies our task compared to the previous studies. Specifically, learning in our task likely engaged the dorsal striatum (Jueptner et al., 1997; Badgaiyan et al., 2007, 2008; Wachter et al., 2009) more heavily than it engaged the ventral striatum, as our task did not require participants to learn to associate a reward with a visual image as in previous experiments (Frank et al., 2004; Cools et al., 2006; Bodi et al., 2009; Rutledge et al., 2009; Voon et al., 2010). Rather, our task required participants to associate positive feedback with a sequence of left/right button presses. Anatomically, visual information flows into the BG either via projections from the temporal lobe or orbital frontal cortex (Pandya et al., 1981; Van Hoesen et al., 1981; Alexander et al., 1986; Saint-Cyr et al., 1990; Haber et al., 2000) and both structures project to the ventral striatum. Motor information, however, flows into the BG from frontal motor areas, including primary and premotor cortices, as well as the supplementary motor areas and area 24 (Flaherty and Graybiel, 1994; Graybiel et al., 1994; Kitano et al., 1998; Haber et al., 2000). The cortical motor areas, unlike the cortical visual areas, project to the dorsal and lateral striatum and not the ventral striatum. Physiological and imaging studies support a role of the dorsal striatum in motor sequence learning (DeLong et al., 1984; Alexander and DeLong, 1985; Jenkins et al., 1994; Jueptner et al., 1997; Boecker et al., 1998; Lehericy et al., 2005), and we have previously shown that our task engages premotor cortex (Averbeck et al., 2010), which projects to the dorsal and not the ventral striatum. Thus, associating visual stimuli with rewards likely engages the ventral striatum, as this is where the visual information enters the BG and associating actions with reward, when they are not contingent on visual stimuli, likely engages the dorsal striatum, as this is where the motor information enters the BG.
In PD dopamine loss is more severe during the early progression of the disease in the dorsal striatum than the ventral striatum (Hornykiewicz and Kish, 1987; Kish et al., 1988). Therefore, medication levels necessary to restore dopamine levels in the dorsal striatum, ameliorating motor pathologies can overdose the ventral striatum, leading to cognitive deficits (Gotham et al., 1988; Kish et al., 1988; Swainson et al., 2000; Cools et al., 2001, 2007). The overdose is often attributed to the inverted U shaped dopamine curve, which hypothesizes that there is an optimal level of dopamine, and dopamine levels above or below this amount lead to behavioral deficits (Arnsten and Goldman-Rakic, 1998; Cools, 2006; Vijayraghavan et al., 2007).
As it is the dorsal and not the ventral striatum that is denervated early in PD, the phasic dopamine signals necessary for learning are likely lost relatively early in the disease progression in the dorsal striatum (Garris et al., 1997), whereas they would be retained in the ventral striatum. It is interesting to note that learning from positive feedback in PD patients on medication in previous studies (Frank et al., 2004; Rutledge et al., 2009) was actually better than control participants. This suggests that dopamine replacement medication is actually boosting dopamine signaling above what is seen in unmedicated control participants, as has been seen in healthy control participants on L-DOPA (Pessiglione et al., 2006). This is in contrast to our study in which PD patients were worse than controls whether “OFF” or “ON” medication, which is consistent with an overall loss of phasic dopamine signaling in the dorsal striatum. Consistent with this, we found a correlation between disease duration and learning from positive feedback, suggesting that the more denervated is the dorsal striatum, the worse the performance on our task. The addition of dopamine replacement medication may raise the tonic level of dopamine in the dorsal striatum, which may further impair any residual phasic dopamine signal. This is due to the fact that tonic dopamine levels are maintained more effectively in the partially denervated striatum than phasic dopamine signals (Garris et al., 1997). The main caveat to this explanation is that it is not yet clear if L-DOPA increases tonic or phasic dopamine, or how much it does so.
Conclusion
We found deficits in sequence learning, within an RL framework, in patients with PD relative to controls. The deficits were mainly due to decreased learning from positive feedback, although across all participant groups learning was more strongly associated with positive than negative feedback in our task. While this is inconsistent with previous findings with other types of probabilistic learning tasks, which suggest that there is increased learning from positive feedback when PD participants are “ON” medication, the learning in our task is likely mediated by the relatively depleted dorsal striatum and not the relatively intact ventral striatum. Therefore, the changes we see in our task are likely due to a strong loss of phasic dopamine signals in the dorsal striatum in PD, and not an overdose of ventral striatum due to excessive dopamine, which likely underlies previous results.
Conflict of Interest Statement
The authors declare that the research was conducted in the absence of any commercial or financial relationships that could be construed as a potential conflict of interest.
Acknowledgments
The authors wish to thank to all the patients and volunteers who participated in this study. This work was supported in part by the Intramural Program of the NIH, National Institute of Mental Health and partly supported by the Wellcome Trust.
References
Alexander, G. E., and DeLong, M. R. (1985). Microstimulation of the primate neostriatum. I. Physiological properties of striatal microexcitable zones. J. Neurophysiol. 53, 1401–1416.
Alexander, G. E., DeLong, M. R., and Strick, P. L. (1986). Parallel organization of functionally segregated circuits linking basal ganglia and cortex. Annu. Rev. Neurosci. 9, 357–381.
Arnsten, A. F., and Goldman-Rakic, P. S. (1998). Noise stress impairs prefrontal cortical cognitive function in monkeys: evidence for a hyperdopaminergic mechanism. Arch. Gen. Psychiatry 55, 362–368.
Averbeck, B. B., Kilner, J., and Frith, C. D. (2010). Neural correlates of sequence learning with stochastic feedback. J. Cogn. Neurosci. (Epub).
Badgaiyan, R. D., Fischman, A. J., and Alpert, N. M. (2008). Explicit motor memory activates the striatal dopamine system. Neuroreport 19, 409–412.
Badgaiyan, R. D., Fischman, A. J., and Alpert, N. M. (2007). Striatal dopamine release in sequential learning. Neuroimage 38, 549–556.
Beck, A. T., Erbaugh, J., Ward, C. H., Mock, J., and Mendelsohn, M. (1961). An inventory for measuring depression. Arch. Gen. Psychiatry 4, 561–571.
Bodi, N., Keri, S., Nagy, H., Moustafa, A., Myers, C. E., Daw, N., Dibo, G., Takats, A., Bereczki, D., and Gluck, M. A. (2009). Reward-learning and the novelty-seeking personality: a between- and within-subjects study of the effects of dopamine agonists on young Parkinsons patients. Brain 132, 2385–2395.
Boecker, H., Dagher, A., Ceballos-Baumann, A. O., Passingham, R. E., Samuel, M., Friston, K. J., Poline, J., Dettmers, C., Conrad, B., and Brooks, D. J. (1998). Role of the human rostral supplementary motor area and the basal ganglia in motor sequence control: investigations with H2 15O PET. J. Neurophysiol. 79, 1070–1080.
Brown, R. G., Jahanshahi, M., Limousin-Dowsey, P., Thomas, D., Quinn, N. P., and Rothwell, J. C. (2003). Pallidotomy and incidental sequence learning in Parkinson’s disease. Neuroreport 14, 21–24.
Carbon, M., Ghilardi, M. F., Feigin, A., Fukuda, M., Silvestri, G., Mentis, M. J., Ghez, C., Moeller, J. R., and Eidelberg, D. (2003). Learning networks in health and Parkinson’s disease: reproducibility and treatment effects. Hum. Brain Mapp. 19, 197–211.
Carbon, M., Ma, Y., Barnes, A., Dhawan, V., Chaly, T., Ghilardi, M. F., and Eidelberg, D. (2004). Caudate nucleus: influence of dopaminergic input on sequence learning and brain activation in Parkinsonism. Neuroimage 21, 1497–1507.
Cohen, N. J., and Squire, L. R. (1980). Preserved learning and retention of pattern-analyzing skill in amnesia: dissociation of knowing how and knowing that. Science 210, 207–210.
Cools, R. (2006). Dopaminergic modulation of cognitive function-implications for L-DOPA treatment in Parkinson’s disease. Neurosci. Biobehav. Rev. 30, 1–23.
Cools, R., Altamirano, L., and D’Esposito, M. (2006). Reversal learning in Parkinson’s disease depends on medication status and outcome valence. Neuropsychologia 44, 1663–1673.
Cools, R., Barker, R. A., Sahakian, B. J., and Robbins, T. W. (2001). Enhanced or impaired cognitive function in Parkinson’s disease as a function of dopaminergic medication and task demands. Cereb. Cortex 11, 1136–1143.
Cools, R., Lewis, S. J. G., Clark, L., Barker, R. A., and Robbins, T. W. (2007). L-DOPA disrupts activity in the nucleus accumbens during reversal learning in Parkinson’s disease. Neuropsychopharmacology 32, 180–189.
DeLong, M. R., Georgopoulos, A. P., Crutcher, M. D., Mitchell, S. J., Richardson, R. T., and Alexander, G. E. (1984). Functional organization of the basal ganglia: contributions of single-cell recording studies. Ciba Found. Symp. 107, 64–82.
Deroost, N., Kerckhofs, E., Coene, M., Wijnants, G., and Soetens, E. (2006). Learning sequence movements in a homogenous sample of patients with Parkinson’s disease. Neuropsychologia 44, 1653–1662.
Flaherty, A. W., and Graybiel, A. M. (1994). Input-output organization of the sensorimotor striatum in the squirrel monkey. J. Neurosci. 14, 599–610.
Folstein, M. F., Folstein, S. E., and McHugh, P. R. (1975). “Mini-mental state”. A practical method for grading the cognitive state of patients for the clinician. J. Psychiatr. Res. 12, 189–198.
Frank, M. J., Seeberger, L. C., and O’reilly, R. C. (2004). By carrot or by stick: cognitive reinforcement learning in parkinsonism. Science 306, 1940–1943.
Garris, P. A., Walker, Q. D., and Wightman, R. M. (1997). Dopamine release and uptake rates both decrease in the partially denervated striatum in proportion to the loss of dopamine terminals. Brain Res. 753, 225–234.
Ghilardi, M. F., Eidelberg, D., Silvestri, G., and Ghez, C. (2003). The differential effect of PD and normal aging on early explicit sequence learning. Neurology 60, 1313–1319.
Gotham, A. M., Brown, R. G., and Marsden, C. D. (1988). ‘Frontal’ cognitive function in patients with Parkinson’s disease ‘on’ and ‘off’ levodopa. Brain 111(Pt 2), 299–321.
Graybiel, A. M., Aosaki, T., Flaherty, A. W., and Kimura, M. (1994). The basal ganglia and adaptive motor control. Science 265, 1826–1831.
Haber, S. N., Fudge, J. L., and McFarland, N. R. (2000). Striatonigrostriatal pathways in primates form an ascending spiral from the shell to the dorsolateral striatum. J. Neurosci. 20, 2369–2382.
Hoehn, M. M., and Yahr, M. D. (1967). Parkinsonism: onset, progression and mortality. Neurology 17, 427–442.
Hornykiewicz, O., and Kish, S. J. (1987). Biochemical pathophysiology of Parkinson’s disease. Adv. Neurol. 45, 19–34.
Hughes, A. J., Daniel, S. E., Kilford, L., and Lees, A. J. (1992). Accuracy of clinical-diagnosis of idiopathic parkinsons-disease – a clinicopathological study of 100 cases. J. Neurol. Neurosurg. Psychiatr. 55, 181–184.
Jahanshahi, M., Wilkinson, L., Gahir, H., Dharminda, A., and Lagnado, D. A. (2009). Medication impairs probabilistic classification learning in Parkinson’s disease. Neuropsychologia (Epub).
Jenkins, I. H., Brooks, D. J., Nixon, P. D., Frackowiak, R. S., and Passingham, R. E. (1994). Motor sequence learning: a study with positron emission tomography. J. Neurosci. 14, 3775–3790.
Jueptner, M., Frith, C. D., Brooks, D. J., Frackowiak, R. S., and Passingham, R. E. (1997). Anatomy of motor learning. II. Subcortical structures and learning by trial and error. J. Neurophysiol. 77, 1325–1337.
Kelly, S. W., Jahanshahi, M., and Dirnberger, G. (2004). Learning of ambiguous versus hybrid sequences by patients with Parkinson’s disease. Neuropsychologia 42, 1350–1357.
Kish, S. J., Shannak, K., and Hornykiewicz, O. (1988). Uneven pattern of dopamine loss in the striatum of patients with idiopathic Parkinson’s disease. Pathophysiologic and clinical implications. N. Engl. J. Med. 318, 876–880.
Kitano, H., Tanibuchi, I., and Jinnai, K. (1998). The distribution of neurons in the substantia nigra pars reticulata with input from the motor, premotor and prefrontal areas of the cerebral cortex in monkeys. Brain Res. 784, 228–238.
Kwak, Y., Muller, M. L., Bohnen, N. I., Dayalu, P., and Seidler, R. D. (2010). Effect of dopaminergic medications on the time course of explicit motor sequence learning in Parkinson’s disease. J. Neurophysiol. 103, 942–949.
Lehericy, S., Benali, H., Van de Moortele, P. F., Pelegrini-Issac, M., Waechter, T., Ugurbil, K., and Doyon, J. (2005). Distinct basal ganglia territories are engaged in early and advanced motor sequence learning. Proc. Natl. Acad. Sci. U.S.A. 102, 12566–12571.
Marsden, C. D. (1982). The mysterious motor function of the basal ganglia – the Robert Wartenberg lecture. Neurology 32, 514–539.
Nagy, H., Keri, S., Myers, C. E., Benedek, G., Shohamy, D., and Gluck, M. A. (2007). Cognitive sequence learning in Parkinson’s disease and amnestic mild cognitive impairment: Dissociation between sequential and non-sequential learning of associations. Neuropsychologia 45, 1386–1392.
Nakamura, T., Ghilardi, M. F., Mentis, M., Dhawan, V., Fukuda, M., Hacking, A., Moeller, J. R., Ghez, C., and Eidelberg, D. (2001). Functional networks in motor sequence learning: abnormal topographies in Parkinson’s disease. Hum. Brain Mapp. 12, 42–60.
Nissen, M. J., and Bullemer, P. (1987). Attentional requirements of learning – evidence from performance-measures. Cogn. Psychol. 19, 1–32.
Pandya, D. N., Van Hoesen, G. W., and Mesulam, M. M. (1981). Efferent connections of the cingulate gyrus in the rhesus monkey. Exp. Brain Res. 42, 319–330.
Pessiglione, M., Seymour, B., Flandin, G., Dolan, R. J., and Frith, C. D. (2006). Dopamine-dependent prediction errors underpin reward-seeking behaviour in humans. Nature 442, 1042–1045.
Rutledge, R. B., Lazzaro, S. C., Lau, B., Myers, C. E., Gluck, M. A., and Glimcher, P. W. (2009). Dopaminergic drugs modulate learning rates and perseveration in parkinson’s patients in a dynamic foraging task. J. Neurosci. 29, 15104–15114.
Saint-Cyr, J. A., Ungerleider, L. G., and Desimone, R. (1990). Organization of visual cortical inputs to the striatum and subsequent outputs to the pallido-nigral complex in the monkey. J. Comp. Neurol. 298, 129–156.
Schultz, W., Dayan, P., and Montague, P. R. (1997). A neural substrate of prediction and reward. Science 275, 1593–1599.
Schultz, W., and Dickinson, A. (2000). Neuronal coding of prediction errors. Annu. Rev. Neurosci. 23, 473–500.
Seidler, R. D., Purushotham, A., Kim, S. G., Ugurbil, K., Willingham, D., and Ashe, J. (2005). Neural correlates of encoding and expression in implicit sequence learning. Exp. Brain Res. 165, 114–124.
Seidler, R. D., Tuite, P., and Ashe, J. (2007). Selective impairments in implicit learning in Parkinson’s disease. Brain Res. 1137, 104–110.
Shin, J. C., and Ivry, R. B. (2003). Spatial and temporal sequence learning in patients with Parkinson’s disease or cerebellar lesions. J. Cogn. Neurosci. 15, 1232–1243.
Shohamy, D., Myers, C. E., Grossman, S., Sage, J., and Gluck, M. A. (2005). The role of dopamine in cognitive sequence learning: evidence from Parkinson’s disease. Behav. Brain Res. 156, 191–199.
Smith, J., Siegert, R. J., McDowall, J., and Abernethy, D. (2001). Preserved implicit learning on both the serial reaction time task and artificial grammar in patients with Parkinson’s disease. Brain Cogn. 45, 378–391.
Smith, J. G., and McDowall, J. (2004). Impaired higher order implicit sequence learning on the verbal version of the serial reaction time task in patients with Parkinson’s disease. Neuropsychology 18, 679–691.
Sutton, R. S., and Barto, A. G. (1998). Reinforcement learning an introduction (Cambridge, Mass: MIT Press).
Swainson, R., Rogers, R. D., Sahakian, B. J., Summers, B. A., Polkey, C. E., and Robbins, T. W. (2000). Probabilistic learning and reversal deficits in patients with Parkinson’s disease or frontal or temporal lobe lesions: possible adverse effects of dopaminergic medication. Neuropsychologia 38, 596–612.
Van Hoesen, G. W., Yeterian, E. H., and Lavizzo-Mourey, R. (1981). Widespread corticostriate projections from temporal cortex of the rhesus monkey. J. Comp. Neurol. 199, 205–219.
Vijayraghavan, S., Wang, M., Birnbaum, S. G., Williams, G. V., and Arnsten, A. F. (2007). Inverted-U dopamine D1 receptor actions on prefrontal neurons engaged in working memory. Nat. Neurosci. 10, 376–384.
Voon, V., Pessiglione, M., Brezing, C., Gallea, C., Fernandez, H. H., Dolan, R. J., and Hallett, M. (2010). Mechanisms underlying dopamine-mediated reward bias in compulsive behaviors. Neuron 65, 135–142.
Wachter, T., Lungu, O. V., Liu, T., Willingham, D. T., and Ashe, J. (2009). Differential effect of reward and punishment on procedural learning. J. Neurosci. 29, 436–443.
Wechsler, A. S. (1997). Wechsler Adult Intelligence Scale-3rd Edition (WAIS-3®) New York The Psychological Corporation.
Werheid, K., Ziessler, M., Nattkemper, D., and Yves von, C. D. (2003). Sequence learning in Parkinson’s disease: the effect of spatial stimulus-response compatibility. Brain Cogn. 52, 239–249.
Wilkinson, L., and Jahanshahi, M. (2007). The striatum and probabilistic implicit sequence learning. Brain Res. 1137, 117–130.
Wilkinson, L., Khan, Z., and Jahanshahi, M. (2009). The role of the basal ganglia and its cortical connections in sequence learning: evidence from implicit and explicit sequence learning in Parkinson’s disease. Neuropsychologia 47, 2564–2573.
Wilkinson, L., Lagnado, D. A., Quallo, M., and Jahanshahi, M. (2008). The effect of feedback on non-motor probabilistic classification learning in Parkinson’s disease. Neuropsychologia 46, 2683–2695.
Keywords: Parkinson’s disease, dopamine, sequence learning, reinforcement learning
Citation: Seo M, Beigi M, Jahanshahi M and Averbeck BB (2010) Effects of dopamine medication on sequence learning with stochastic feedback in Parkinson’s disease. Front. Syst. Neurosci. 4:36. doi: 10.3389/fnsys.2010.00036
Received: 18 May 2010;
Paper pending published: 22 June 2010;
Accepted: 02 July 2010;
Published online: 12 August 2010
Edited by:
Ranulfo Romo, Universidad Nacional Autónoma de México, MexicoReviewed by:
Jose Bargas, Universidad Nacional Autónoma de México, MexicoHugo Merchant, Universidad Nacional Autónoma de México, Mexico
Copyright: © 2010 Seo, Beigi, Jahanshahi and Averbeck. This is an open-access article subject to an exclusive license agreement between the authors and the Frontiers Research Foundation, which permits unrestricted use, distribution, and reproduction in any medium, provided the original authors and source are credited.
*Correspondence: Bruno B. Averbeck, Laboratory of Neuropsychology, National Institute of Mental Health, National Institutes of Health, Building 49 Room 1B80, 49 Convent Drive MSC 4415, Bethesda, MD 20892-4415, USA. e-mail:YnJ1bm8uYXZlcmJlY2tAbmloLmdvdg==