- 1 Université de Bordeaux, UMR 5293, Institut des Maladies Neurodégénératives, Bordeaux, France
- 2 CNRS, UMR 5293, Institut des Maladies Neurodégénératives, Bordeaux, France
- 3 Centre Hospitalier Universitaire de Bordeaux, Bordeaux Cedex, France
Decision is a self-generated phenomenon, which is hard to track with standard time averaging methods, such as peri-event time histograms (PETHs), used in behaving animals. Reasons include variability in duration of events within a task and uneven reaction time of animals. We have developed a temporal normalization method where PETHs were juxtaposed all along task events and compared between neurons. We applied this method to neurons recorded in striatum and GPi of behaving monkeys involved in a choice task. We observed a significantly higher homogeneity of neuron activity profile distributions in GPi than in striatum. Focusing on the period of the task during which the decision was taken, we showed that approximately one quarter of all recorded neurons exhibited tuning functions. These so-called coding neurons had average firing rates that varied as a function of the value of both presented cues, a combination here referred to as context, and/or value of the chosen cue. The tuning functions were used to build a simple maximum likelihood estimation model, which revealed that (i) GPi neurons are more efficient at encoding both choice and context than striatal neurons and (ii) context prediction rates were higher than those for choice. Furthermore, the mutual information between choice or context values and decision period average firing rate was higher in GPi than in striatum. Considered together, these results suggest a convergence process of the global information flow between striatum and GPi, preferentially involving context encoding, which could be used by the network to perform decision-making.
Introduction
In a visually guided motor task, decision-making is a distributed neural process that involves the basal ganglia (BG) interacting with the frontal and prefrontal cortical areas as well as with the dopaminergic system (Opris and Bruce, 2005; Schultz, 2006; Daw, 2007; Samejima and Doya, 2007; Kable and Glimcher, 2009). In a recent electrophysiological study in behaving monkeys, using a multiple choice task, we showed that the encoding of the movement direction by the neurons of the striatum (the main input of the BG) and the internal globus pallidus (GPi, the main output of the BG) is modulated by the incentive value of the action (Pasquereau et al., 2007). This could provide a mechanism by which motor program selection could be learned under dopamine control (Samejima and Doya, 2007).
However, the selection process, is only partially accessible using classical electrophysiological analysis methods, such as PETHs. This is because, even when a cue is presented at a known time and the time of the locomotor action to implement the decision is known, the actual moment of decision-making cannot be observed and so its temporal relationship to the cue and other events cannot be precisely known. Moreover, experimental protocols for decision-making assessments (including those used in our own studies) assign a randomly variable duration between task events in order to decorrelate all the steps from one another. This means that the time between events varies for each trial. This, along with the fact that cognitive processing time varies from trial to trial for each animal, prevents the direct comparison of the time course of the neuronal activity profiles. Despite the intrinsic limitation that PETH computation does not by itself provide a framework for statistical inference (Czanner et al., 2008), it remains a widely used tool that provides meaningful insights and whose efficiency has been improved (Endres and Oram, 2010). To solve this conundrum, we developed a simple method to normalize time durations in each trial and thus to build a normalized inter-event time histogram (NIETH) for individual neurons. This normalization method was applied to the whole trial duration because BG activity is notoriously variable and may have dynamic encoding capacities (Arkadir et al., 2004). Using this method, we analyzed data previously recorded in the GPi and the striatum of two monkeys during a reward probability-based, free-choice motor task (Figure 1, see Pasquereau et al., 2007 for details). We then focused our analysis on a possible correlation between the neuronal activity in striatum and GPi and the animal behavior during the crucial period between the appearance of the cue and the go signal, the decision period (DP). To link neuronal activity to behavior, we investigated neuronal coding of behavioral events as a possible basis for a computational predictive model and their mutual information to quantify their interdependence. We thus addressed the questions of how and where information flows were processed in the BG system.
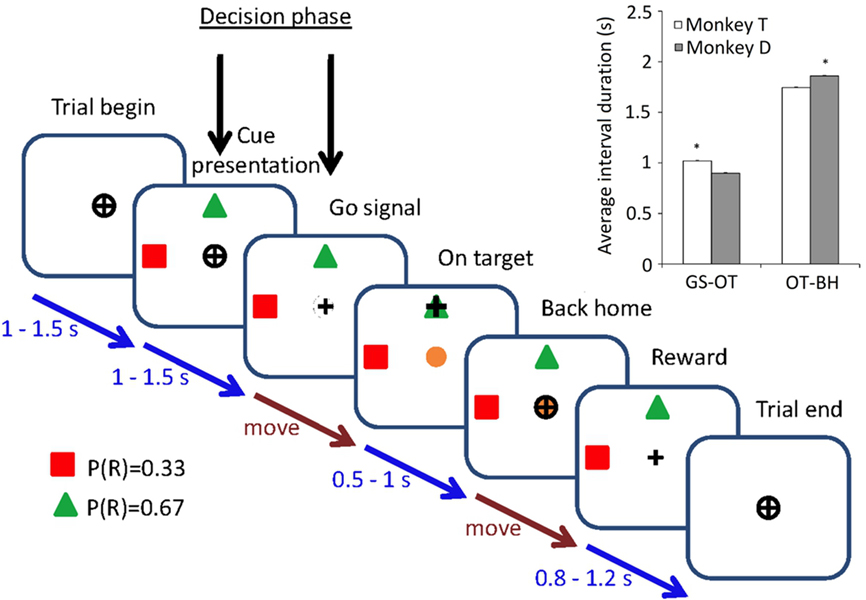
Figure 1. Behavioral paradigm of the reward probability-based free-choice task. Two different targets associated with reward probabilities (here P(R) = 0.33 and 0.67) are displayed simultaneously during each trial in four possible positions in random order (six target combinations) and in random locations (4 × 3 possibilities). The different milestones of a trial sequence are described here with their range of duration. During the “move” phases, the duration is partially under the control of the animal itself. Inset: difference of the average durations of task phases under the control of the monkey, error bars indicate SEM (Student’s t-test, p < 0.01).
Materials and Methods
The reader is invited to refer to the first paper dealing with these data (Pasquereau et al., 2007) for an exhaustive description of materials and methods involved with the data acquisition. Here we provide a summary including only the details necessary to explain the additional analyses and results.
Animal Training and Surgery
The study was conducted on two female rhesus monkeys (Macaca mulatta, weighing 5.6 and 4 kg). The primates were kept under water restriction to increase their motivation during the task training. A veterinarian skilled in the healthcare and maintenance of non-human primates supervised all aspects of animal care. Surgical and experimental procedures were performed in accordance with the Council Directive of 24 November 1986 (86/609/EEC) of the European Community and the National Institute of Health Guide for the Care and Use of Laboratory Animals. In the task, monkeys were trained to move a custom-made manipulandum in a horizontal plane with their right hand. The manipulandum moved a cursor on a computer screen placed 50 cm in front of the monkey. In each trial of a session, two different cue targets (randomly chosen from a set of four targets, each with a different reward probability (P(R) = [0, 0.33, 0.67, and 1])) were displayed simultaneously on the screen. Each cue appeared randomly in one of four possible directions (0°, 90°, 180°, 270°). In order to induce a situation in which there was always an optimal choice, a single trial could not include two identical cues or two targets in the same location. After a random period (1–1.5 s), the “go” signal was given and the monkey had to initiate a movement toward one of the two targets. Once this position was reached the animal had to hold the cursor on the target for a random period (0.5–1 s) after which the cursor had to be moved back to the central position. The reward was then delivered (fruit juice) according to the probability associated with the chosen target. For each successful trial, if the monkey chose the target associated with the highest probability of receiving a reward, their choice was defined as optimal. If not, they would still receive reward with a probability equal to that for the chosen target. A recording chamber was then implanted on the skull of each animal. The surgical procedure for attaching the recording chamber has been extensively described in previous publications (Bezard et al., 2001; Boraud et al., 2001).
For purposes of analysis of the relationship between external sensory cues and the neuronal firing activity, we consider that the context in which the animal was making the decision was the combination of the two targets that were visible during a trial. Thus, with two targets selected from four, there are six possible combinations and therefore six distinct contexts within which the animal makes a decision on which target to choose. Due to the animals being over-trained, they never choose a target associated with a 0 reward probability. Consequently, they are considered to have only three possible choices associated with the remaining 0.33, 0.67, and 1 reward probabilities.
Recording and Data Acquisition
Neuronal recordings were performed in the dorsolateral striatum and the GPi. Data acquisition, spike sorting, and storage are described elsewhere (Pasquereau et al., 2007). The following behavioral events were recorded and stored simultaneously with the electrophysiological recordings: trial begin (TB), cue presentation (CP), go signal (GS), on target (OT), back home (BH), reward/no reward (RW/NRW), and finally trial end (TE). This is described in Figure 1.
Data Analysis
The analyses were performed with custom-made Matlab (MathWorks, Natick, MA, USA) and NeuroExplorer tools and scripts (Nex Technologies, Littleton, MA, USA), and C# libraries (Microsoft, Seattle, WA, USA).
Nieth Analysis
Nieths Extraction and Normalization
To have an overall view of the neuronal dynamics associated with the choice task and to compare both striatal and pallidal activity profiles, we investigated the temporal outline of NIETHs across all the steps of the task. Therefore, we have implemented an algorithm that can automatically identify event sequences of interest within the NIETHs and extract the spike trains related to these events. In a first step, the algorithm, extracted all the recorded sequences where the monkey completed every event through the course of the trial (here the event sequence: TB–CP–GS–OT–BH–RW–TE) and discarded sequences where any event was not completed (e.g., where the monkey failed to return the cursor to home). Because recording continues until and after reward delivery, we were able to note that the firing profile in cases where reward was obtained and those where no reward was obtained were different. Therefore, all trials in which no reward was gained were also discarded. In summary, in the presented results only successfully completed trials where a reward was obtained are shown in order to minimize variation in neuronal activity due to inter-trial variation in behavioral profile. The algorithm then computes the NIETHs using each occurrence of the complete event sequence. Duration of the inter-event intervals (IEI) is either random or behaviorally dependent as shown on Figure 1 and this adds variability to the NIETH length and thus makes inter-neuron activity profile comparisons difficult. In a second step, an additional procedure of time normalization of the NIETH is implemented to solve this problem. The first IEI between TB and CP is always split into the same number of time bins in every trial and for every neuron. This means that the duration of a bin in one trial is not equal to that in another trial but that the number of bins for a given TB–CP interval is the same for every trial. In our study, the first IEI (TB–CP) was always split into 100 bins in order to obtain a bin size close to 10 ms (Zhang and Reid, 2005). Because the duration of this event can vary from 1 to 1.5 s, the length of a bin can thus vary from 10 to 15 ms, but the average length of bin duration can be calculated. This average bin duration is then used for all subsequent IEIs (CP–GS, GS–OT …). Because the average duration of each subsequent event differs, the number of bins allocated for each event also changes. Thus, for example if the average duration of the TB-CP event was 1.2 s and for the CP–GS event was 1.8 s, the CP–GS event would be divided into 100 × 1.8/1.2 = 150 bins. This rescaling prevents time normalization biases by maintaining IEI durations close to the original. Due to the similar IEI average durations between different neurons (most of the random durations are generated by the software itself), this normalization technique finally allows NIETHs alignment in time and thus their comparison. At the same time, amplitude normalization (Burkhardt and Whittle, 1973; Gage et al., 2010) is applied to the NIETH based on the maximum number of spikes observed at any point in time over the whole time course of a trial. This amplitude normalization allows averaging of the profile of activation of many neurons across an event, whatever the maximal firing rate of individual neurons, by considering only their deviation from their baseline level. Without the amplitude normalization, neurons with a high maximal firing rate would have a disproportionate effect on the profile and, assuming that the information within the system is transmitted by population encoding, would distort the representation of the information across the course of an event.
Monkey data have been separately processed and not pooled because of a non-negligible level of variability related to their individual behavior. Indeed, the analysis of the distribution of the two inter-events intervals which are under the control of the animal (GS–OT and OT–BH) exhibited significant differences as shown in Figure 1 inset. Using this method, we have performed new analyses of the data of a previous publication by our team (Pasquereau et al., 2007).
Nieths Study and Comparison
We compared NIETH distributions between striatum and GPi. Our first goal here was to find whether salient activity profile features emerged in the neuronal activity of the two structures. To achieve this, two complementary approaches were explored, applied separately to each monkey. The first approach consisted in computing correlation coefficient matrices between every neuron NIETH. The second relied on the computation of NIETH entropies to estimate the variability within their population. The Shannon (1948) entropy computation (and its derived methods) is now often used as a non-linear analysis tool providing information on neuronal activity temporal organization (Borst and Theunissen, 1999; Lim et al., 2010) or complexity characterization (Haslinger et al., 2010). Here, we used it to quantify the activity variability throughout the task in parallel for every neuron. If the activity profiles are similar, successive entropy values will be low and vice versa. Both approaches were performed on all the NIETHs. The Shannon entropy H (here in bits) of a discrete variable X with n possible values is given by:

where p(xi) is the probability associated with (xi). Due to the high dimensionality of NIETHs, a preliminary discretization procedure is applied before entropy calculation. NIETH amplitudes vary between 0 and 1 and we arbitrarily chose to linearly distribute their values into a 10 interval alphabet ranging from 1 to 10 (i.e., a 0.527 or a 0.596 amplitude value will provide a 5 and a 0.758 will provide a 7). These computations were performed with a dedicated Matlab toolbox (Peng et al., 2005) which was also used for further mutual information calculations. These analyses were performed separately on the two monkeys and on striatum and GPi.
Extraction of “Coding Neurons”
The monkey’s decision is made between the CP and the movement initiation triggered by the GS. This period of time includes the decision process phase itself, but it may also include an amount of time during which the monkey has already made its decision and just waits for the GS. Because it is not easy to differentiate between the decision period and the waiting period (Leblois et al., 2006a), we computed averaged firing rates for each neuron during this decision period (DPAFR) for each different context presented to the monkey and for each of its actual choices. We first extracted the neurons for which significant variations in DPAFR were related to any of the six different context values or to any of the three choice reward probabilities by applying a one-way ANOVA. We thus designated any neuron that had a significant variation in DPAFR to at least one context or one choice as a coding neuron. When the ANOVA was positive we applied post hoc methods based on the Tukey’s least significant difference procedure. We thus obtained tuning functions for each neuron which associated preferential coding context or choice values with a peak in the firing rate. These tuning functions were then applied to basic modeling studies. These analyses were performed with the Matlab Statistical toolbox and applied separately both to the two monkeys and to striatum and GPi.
Firing Rate Carried Information Analysis
We computed mutual information between the DPAFRs and the context value, for coding neurons. The mutual information I between two discrete random variables X and Y is expressed in bits and is given by:

where p(x,y) is the joint probability distribution function of X and Y, p(x), and p(y) are the marginal probability distribution functions of X and Y respectively. The results were used to investigate the respective involvements of the GPi and of the striatum in the processing of information of choice and context encoding in BG.
Tuning Function Model Prediction
The tuning functions derived in the “coding neurons” section were then used as a simple reverse model to assess how good the tuning function was at predicting a direction given the average firing rate in the decision period as an input. For every coding neuron in both structures, the tuning function exhibited a preferential context/choice value encoding (e.g., one neuron can have its highest DPAFR when the animal is presented with a given context or choice number). For each previously extracted coding neuron, our predictive model thus associated the six different reference DPAFRs of the tuning function with each of the six different context values (and respectively the three DPAFRs associated with each of the three choice values). The model was then used as follows: DPAFRs were computed for each trial of a given coding neuron. For every trial, the experimental DPAFR was applied as an input to the tuning function (core of the model) which returned the most likely context or choice theoretical value (i.e., that for which reference DPAFR was closest to the experimental DPAFR). When this theoretical value was the same as the actual, the trial model prediction was considered as successful. Success rates were then computed for context and choice encoding in both monkeys and in both striatum and GPi.
The prediction quality of the model was then compared to random choices based on context and choice chance based rates (respectively 16.67 and 33.33% to obtain the actual value with a random draw). The significance of the model retrieval rates was compared to the chance based rates using a Kolmogorov–Smirnov test. A Wilcoxon rank signed test was then applied on success rates to compare the power of the model concerning context and choice prediction in order to conclude which was most efficiently encoded in the recorded structures.
Results
Nieths Extraction
The software successfully extracted and normalized, both in time and amplitude, the global NIETHs from all recorded neurons and according to the previously defined sequence of events. The present study is based on 111 striatal cells (53 in monkey T and 58 in monkey D) and 107 pallidal cells (51 in monkey T and 56 in monkey D). The normalized NIETH distributions among striatal and pallidal neurons in both monkeys are presented in Figure 2. Several automatic clustering of neuronal subpopulations algorithms, based on principal component analysis of the NIETHs profiles, have been tested here without success. This failure is consistent with the time axis position distribution of the NIETH amplitude peak, as shown in Figure 2. This position is here used as a NIETH sorting parameter and it clearly appears as continuously distributed from one neuron to another all along the time axis.
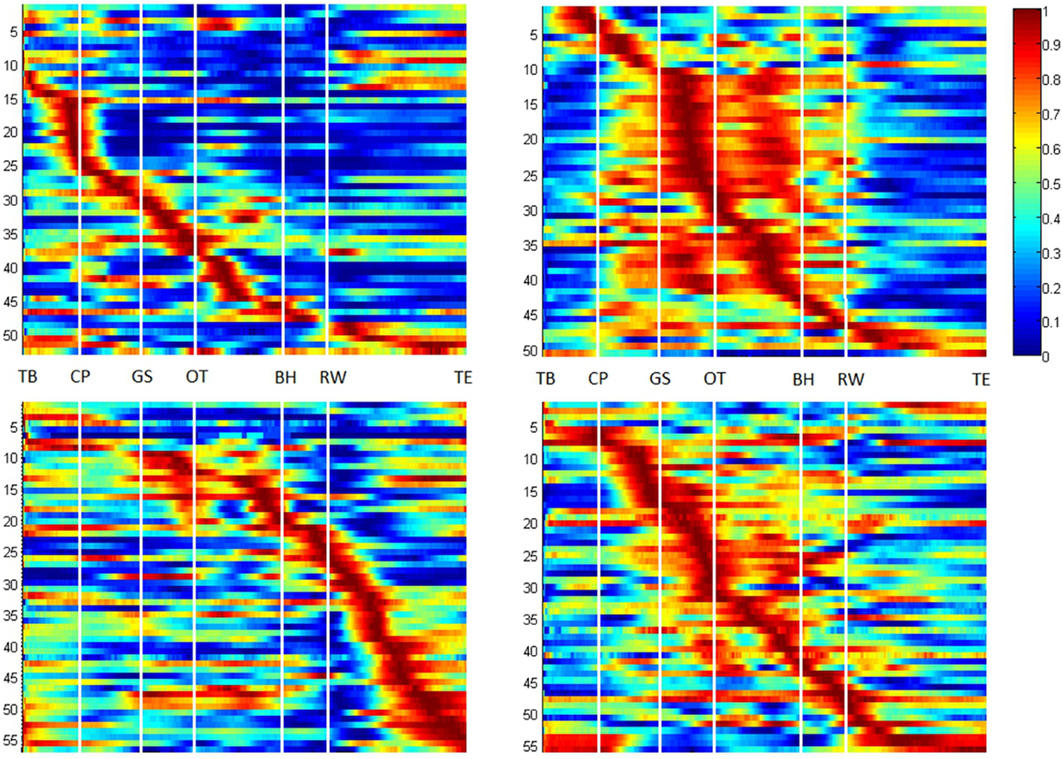
Figure 2. NIETH plots from the striatum (left) and GPi (right) of monkey T (top) and monkey D (bottom), aligned on each event. The neurons were sorted using the curve peak time value. The color bar indicates the normalized amplitude value for each neuron.
The Population Activity Synchronization Differs between Striatal and Pallidal Neurons
A two-way ANOVA was applied to the average correlation coefficient (ACC) values to investigate possible monkey and structure combination (GPi–striatum, GPi–GPi, and striatum–striatum) effects. The resulting p-values were significant (p < 0.01) and provide evidence for interactions between monkey, structure combination and ACC. NIETH correlation coefficient matrices were then processed and compared separately for both monkeys and for striatal and GPi neurons. As was expected after a visual control of Figure 2, differences were revealed between the two regions regarding the neuronal dynamics, as shown in Figures 3A,B. The NIETHs correlation coefficient values were higher between GPi neurons than striatum neurons in both monkeys. This demonstrates that there is less dynamic variability between GPi neurons and this was confirmed by the estimate of their ACC values according to structure. As shown in Figure 3C, these latter differed significantly between the two regions. This emphasizes a higher temporal synchronization of GPi neuronal spike trains compared to striatum. Moreover, the lowest absolute value of correlation coefficient occurred in both monkeys when computing the ACC value between GPi and striatum, which is another argument in favor of a possible functional dissociation between the two structures. These results were similar in both monkeys.
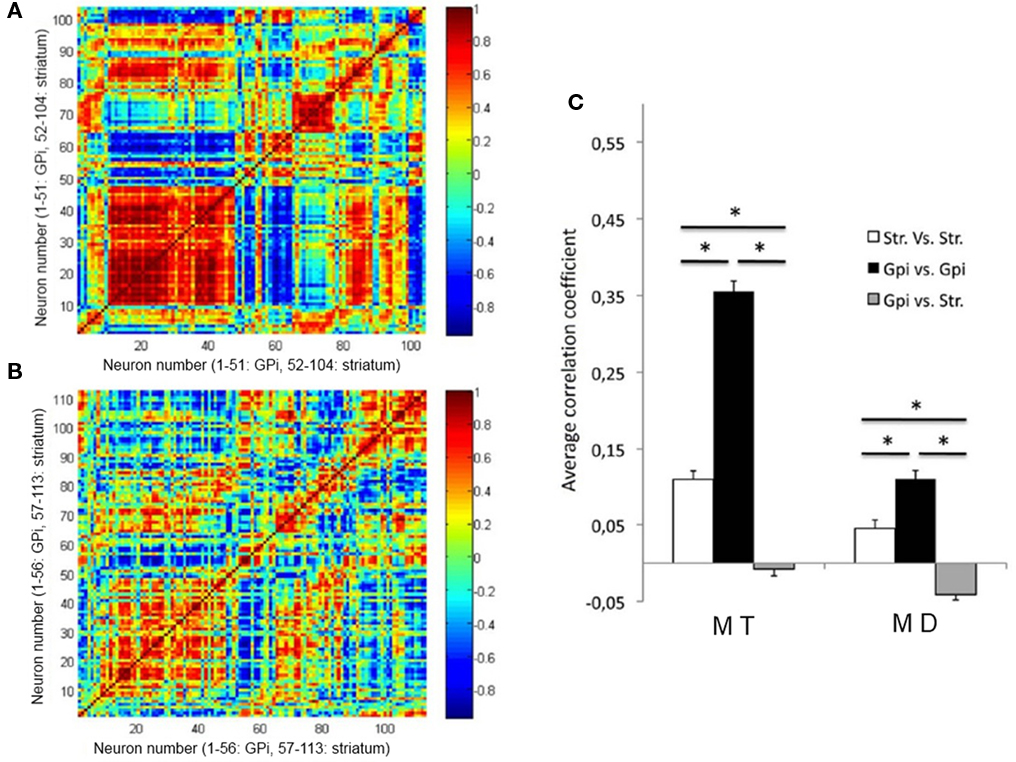
Figure 3. Cross-correlation matrices of all the neuron NIETHs for monkey T (A) and monkey D (B). (C) Histograms of average correlation coefficient values of the neuron NIETHs. Color bar: correlation coefficient value, error bars indicate SEM (one-way ANOVA, p < 0.01, post hoc: Tukey’s least significant difference procedure).
The measure of the Shannon entropy between striatum and GPi added consistent results to this first outcome (respectively 3.11 and 1.25 for monkey T and 2.79 and 1.19 for monkey D). Indeed, Shannon entropy can be considered as a measure of the variability of the signals emitted by a source (here the NIETHs shapes) and we noticed that, in both monkeys, the value was lower in GPi than in striatum, as shown in Figure 4.
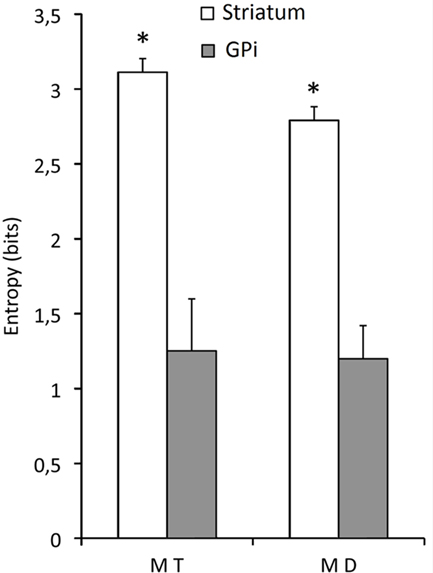
Figure 4. NIETH entropies computed for neurons in monkey T and monkey D. Error bars indicate SEM (Student’s t-test, p < 0.01).
These two results suggest that, considering a given behavioral event expected value such as a choice or a context encoding, the information carried by striatum seems condensed in GPi.
Coding Neurons Activity Analysis
Only neurons that showed an average DPAFR that was dependent on the context or choice were used for analysis of the coding (one-way ANOVA, p < 0.01). For monkey T, 33.96% of the striatal (n = 53) and 21.57% of the GPi neurons (n = 51) and for monkey D, respectively 17.24% (n = 58) and 14.29% (n = 56) displayed such a property (Figure 5A). Tuning function curves were extracted for each coding neuron by computing the DPAFR for every context and choice for that neuron. Some tuning function samples are shown here that exhibit either preferential context (Figure 5B) or choice coding values (Figure 5C).
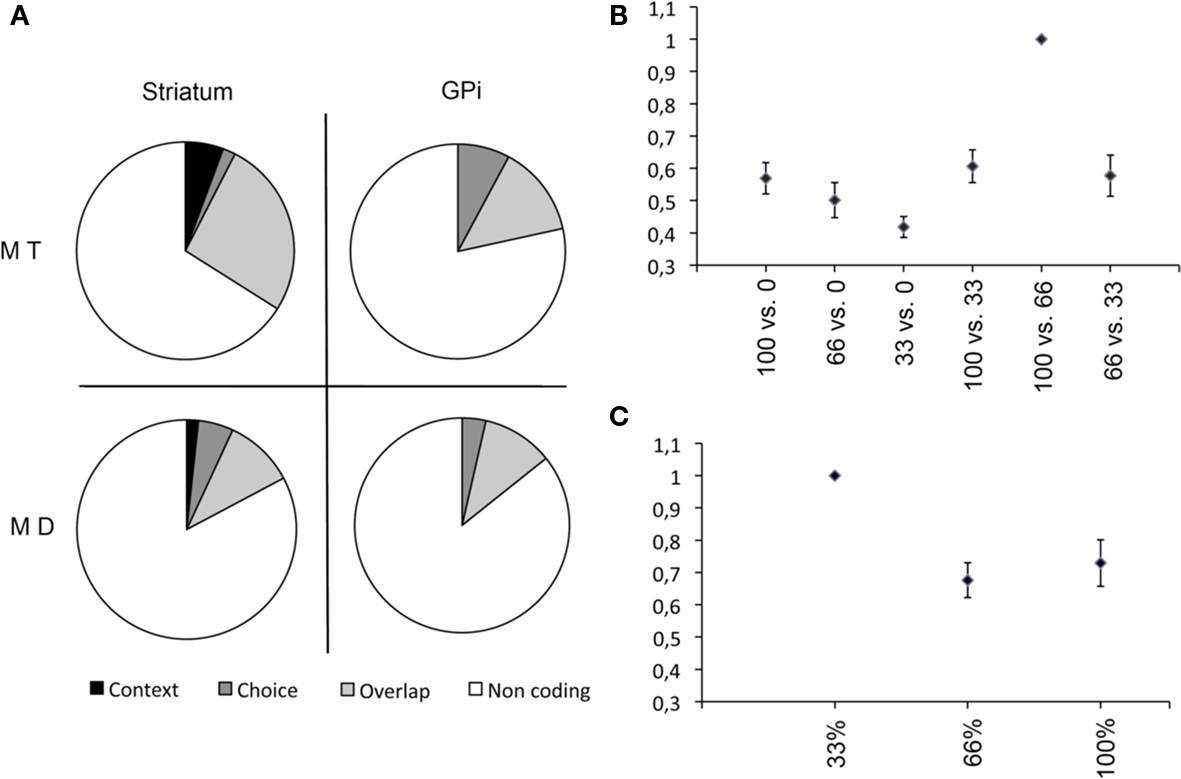
Figure 5. Results of the coding neurons extraction. (A) Coding distributions regarding context or choice values for both monkey T (N = 53 for striatum and 51 for GPi) and monkey D (respectively N = 58 and 56). Overlap indicates neurons coding for both context and choice. (B) Three tuning functions mean and ± SEM plot of context encoding (abscissa: context, ordinate: normalized firing rate) and (C) of three tuning functions of choice encoding samples (abscissa: choice value, ordinate: normalized firing rate).
Mutual information between DPAFRs and both context and choice values were computed and the results expressed in bits. The resulting amount of mutual information carried by striatal neurons was less than that carried by GPi neurons both in context (0.20 vs. 0.73 for monkey T and 0.28 vs. 0.72 for monkey D) and in choice encoding (0.11 vs. 0.29 for monkey T and 0.10 vs. 0.34 for monkey D, Mann–Whitney test, p < 0.01) as shown in Figures 6A,B. GPi neurons appear as more reliable encoders because the DPAFRs of GPi neurons yielded more information on both the context and the choice values than those of striatal neurons in both monkeys,. This implies that the context and/or the choice information are refined between the striatum and the pallidal processing stages and therefore suggests an information convergence mechanism from one structure to another in the sense of a dimensionality reduction (Bar-Gad et al., 2003). On average, one GPi neuron provides as much information as 2.9 striatum neurons in context encoding (and respectively 2.5 in choice encoding). In other words, sampling of fewer neurons in GPi is required to obtain a similar amount of information about both context and choice.
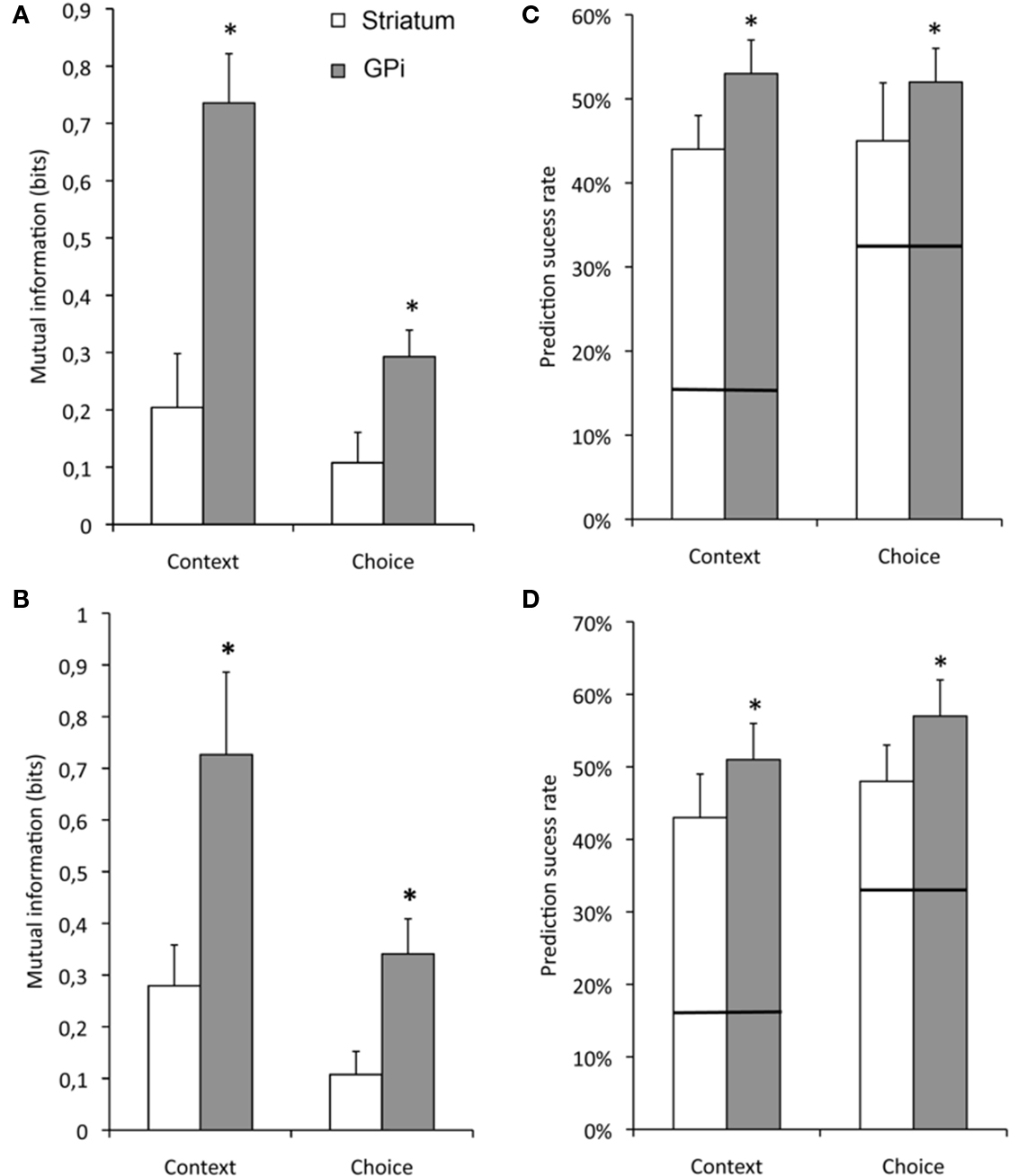
Figure 6. Mutual information between DPAFR and context and choice values (A) for monkey T and (B) for monkey D. Prediction model success rates: (C) for monkey T and (D) for monkey D. Horizontal bars on (C,D) histograms indicate the respective success base chance to predict the correct values (respectively 1/6 for context and 1/3 for choice). Error bars indicate SEM (Mann–Whitney test, p < 0.01).
Performance of Reverse Tuning Curve Model
The previously constructed tuning curves were then used as a model to assess their capability of predicting a context or choice given a DPAFR as input. When the output of the model was the same context or choice that had generated the DPAFR, the model was considered to have made a successful selection. This allowed us to estimate the efficiency of the model in reconstructing the original choice and context values. Figures 6C,D summarize these computations. Our empirical method provided information on the ability of the model to reconstruct a significant part of the original data and thus on its retrieval capability. This allowed us to compare the predictive power of the two neuronal subpopulations. Considering the context or choice prediction rates, we observed a significant higher success rate for GPi neurons compared to striatal neurons. This corroborates the previous mutual information outcomes and confirms a greater involvement of the GPi compared to striatum in both context value and choice encoding and thus an information convergence process. The second result was obtained by comparing the averaged level of context and choice encoding success rates, relative to chance, giving an unbiased respective retrieval success rate. As shown in Figure 6C,D, the success rate profiles of context and choice encoding were similar for the two monkeys. In a first step we compared the actual success rates of the model to the success rates due to chance, which can be described by a binomial distribution with a success base probability value of 16.67% for context and 33.33% for choice. Kolmogorov–Smirnov tests applied for both monkeys for both striatum and GPi vs. chance give p-values of p < 1% for both context and choice. This confirmed that the model predicted both context and choice at a level far greater than chance. In the second step we subtracted the success base chance rate from the actual model results to remove bias and compared the predictive power of the model in context and choice encoding (Bernard and Lapointe, 1987). A Mann–Whitney test was then applied to the unbiased data using an alternative hypothesis of “less” for the choice prediction. For both monkeys and both anatomical structures p < 0.01 were obtained. This suggests that, during the decision period, the average value of the firing rates of the GPi and striatal neurons preferentially encodes the context rather than the choice value.
Discussion
This study presents a novel attempt to shed light on the correlation between BG neuron spike train dynamics and behavioral decision-making tasks. It provides evidence that encoding neurons show at least two remarkable properties: (i) the firing activity of GPi neurons during the DP carries more information on the context and on the choice values than the striatal neurons and (ii) both structures preferentially encode the context rather than the choice.
The BG Encoded Information as a Continuum during the Task
We have presented in this paper, several original approaches to improve analysis of time-dynamic neuronal activity as well as of the information flows in the striatum and the GPi of an animal involved in a sensory-motor probabilistic decision-task. These approaches rely on normalized NIETH profiles (PETH computed on all the events of the task) analysis. This approach clearly showed that neurons of both structures cannot be classified into different clusters. Instead, they encoded the various parameters of the task as a continuum of responses (Figure 2). It also brings out that NIETH profile variability was higher in the striatum than in the GPi.
Different Levels of Synchronization in the Striatum and the GPi
The higher level of synchronization inside the GPi than in the striatum was then analyzed using two different methods: correlation coefficient analysis (Figure 3) and the computation of entropy (Figure 4). This confirmed our previous work (Pasquereau et al., 2007) where we showed that, during the executive part of a choice task, the GPi activity is strongly related to the action performed (encoding mainly movement parameters and action value), while the striatum stays more variable, encoding different parameters (chosen target value, non-chosen target value, motor parameters, action value, etc) in roughly equal proportions of neurons. This study shows that this focus on the action to perform in the output structure of the BG is associated with a high correlation level between GPi neurons. Experiments have shown that only 10–15% of GPi neurons responded to a specific task (Pasquereau et al., 2007) and moreover it makes sense that such simple behavior does not recruit the whole BG system. These data may seem at variance with another study showing decorrelation between GP neurons in a discrimination task (Joshua et al., 2009). However, this study used a non-instrumental task (the animal had no action to perform in response to the cues) while, in our task, the action consequent upon the choice between two options is an essential aspect of the task. Considering different populations of neurons coding different tuning functions, their differential activation related to one specific trial will allow the selection of one specific action as a result of competitive mechanisms (Mink, 1996; Gurney et al., 2001; Nambu, 2004; Leblois et al., 2006b). Comparing these two studies reinforces the hypothesis that the very significant and transient synchronized response in the GPi neural population reflects the decision-making and action selection processes occurring in the cortico-BG loop.
Basal Ganglia Encode Contexts and Choices
In our experiments monkeys were over-trained and maximized their payoff by choosing the target with the higher reward value (for details see Pasquereau et al., 2007). This implies that the encoding strategies for the BG may vary between two boundaries: either it may solely encode the chosen target or its activity may be related to the context dependent choice (Mink and Thach, 1991). We have therefore focused our analysis on the decision period and used two methods to assess the relationship between the neural activity of the BG and the choices performed by the animals (Figure 6). Our model study reveals a better correlation for the encoding of the context than for the encoding of the choice and both (model and mutual information measure) show that the GPi is a better predictor than the striatum for both parameters. These data imply that, during the critical phase, when the animal decides which action to perform, the BG are deeply involved in the computation process which leads to the decision. The fact that there is a robust transformation (as shown by the higher correlation between GPi neurons) of the cortical input information as it passes from the input structure (the striatum) to the output stage (the GPi) is a further confirmation of the importance of the BG in the decision-making process. Two hypotheses can explain why the correlation is higher for the context than for the choice: (i) the BG preferentially encoded the context or (ii) the BG takes into account the context in order to perform a choice. The latter hypothesis has already been proposed by other teams (Morris et al., 2006; Niv et al., 2006) and supports the hypothesis that the cortex BG loop acts as a SARSA learning system and encodes the combination of choice made and context within which the choice is made. Unfortunately, as the monkeys optimized their behavior in our task, thus maximizing their gains, it is impossible to rule out either of these hypotheses.
Conclusion
This work is a first attempt to analyze comprehensively the process of neural computation occurring in the BG during the full duration of a trial of a behavioral task. The high variability of BG neural population firing rate and the impossibility to define clear cut categories of neurons, especially in the output stage, makes this approach more appropriate than the classical PETH which reduces the richness of the time course of the neural responses. The normalization approach we adopted allowed us to visualize and analyze the decision period and allowed us to demonstrate the crucial role played by this structure on the decision-making process. Our model based approach to the coding neuron tuning functions led us to deduce that the context was comparatively better encoded than the choice. The fact that the GPi encodes the context more than the choice itself can also be related to the fact that the different aspects of the context converge from the striatum to the GPi (Mink, 1996). Taken together with our previous data (Pasquereau et al., 2007) and theoretical approach (Leblois et al., 2006a) we infer that this contextual information is used to shape the tuning functions allowing decision to occur by competition mechanisms in the cortex BG loop.
Conflict of Interest Statement
The authors declare that the research was conducted in the absence of any commercial or financial relationships that could be construed as a potential conflict of interest.
References
Arkadir, D., Morris, G., Vaadia, E., and Bergman, H. (2004). Independent coding of movement direction and reward prediction by single pallidal neurons. J. Neurosci. 24, 10047–10056.
Bar-Gad, I., Morris, G., and Bergman, H. (2003). Information processing, dimensionality reduction and reinforcement learning in the basal ganglia. Prog. Neurobiol. 71, 439–473.
Bernard, P.-M., and Lapointe, C. (1987). Mesures statistiques en épidémiologie. Québec: Presses de l’Université du Québec.
Bezard, E., Boraud, T., Chalon, S., Brotchie, J. M., Guilloteau, D., and Gross, C. E. (2001). Pallidal border cells: an anatomical and electrophysiological study in the MPTP-treated monkey. Neuroscience 103, 119–125.
Boraud, T., Bezard, E., Bioulac, B., and Gross, C. (2001). Dopamine agonist-induced dyskinesias are correlated to both firing pattern and frequency alteration of pallidal neurons in the MPTP-treated monkey. Brain 124, 546–557.
Borst, A., and Theunissen, F. E. (1999). Information theory and neural coding. Nat. Neurosci. 2, 947–957.
Burkhardt, D. A., and Whittle, P. (1973). Intensity coding in the frog retina. Quantitative relations between impulse and graded activity. J. Gen. Physiol. 61, 305–322.
Czanner, G., Eden, U. T., Wirth, S., Yanike, M., Suzuki, W. A., and Brown, E. N. (2008). Analysis of between-trial and within-trial neural spiking dynamics. J. Neurophysiol. 99, 2672–2693.
Daw, N. D. (2007). Dopamine: at the intersection of reward and action. Nat. Neurosci. 10, 1505–1507.
Endres, D., and Oram, M. (2010). Feature extraction from spike trains with Bayesian binning: ‘latency is where the signal starts’. J. Comput. Neurosci. 29, 149–169.
Gage, G. J., Stoetzner, C. R., Wiltschko, A. B., and Berke, J. D. (2010). Selective activation of striatal fast-spiking interneurons during choice execution. Neuron 67, 466–479.
Gurney, K., Prescott, T. J., and Redgrave, P. (2001). A computational model of action selection in the basal ganglia. I. A new functional anatomy. Biol. Cybern. 84, 401–410.
Haslinger, R., Klinkner, K. L., and Shalizi, C. R. (2010). The computational structure of spike trains. Neural Comput. 22, 121–157.
Joshua, M., Adler, A., Rosin, B., Vaadia, E., and Bergman, H. (2009). Encoding of probabilistic rewarding and aversive events by pallidal and nigral neurons. J. Neurophysiol. 101, 758–772.
Kable, J. W., and Glimcher, P. W. (2009). The neurobiology of decision: consensus and controversy. Neuron 63, 733–745.
Leblois, A., Boraud, T., Meissner, W., Bergman, H., and Hansel, D. (2006a). Competition between feedback loops underlies normal and pathological dynamics in the basal ganglia. J. Neurosci. 26, 3567–3583.
Leblois, A., Meissner, W., Bezard, E., Bioulac, B., Gross, C. E., and Boraud, T. (2006b). Temporal and spatial alterations in GPi neuronal encoding might contribute to slow down movement in Parkinsonian monkeys. Eur. J. Neurosci. 24, 1201–1208.
Lim, J., Sanghera, M. K., Darbin, O., Stewart, R. M., Jankovic, J., and Simpson, R. (2010). Nonlinear temporal organization of neuronal discharge in the basal ganglia of Parkinson’s disease patients. Exp. Neurol. 224, 542–544.
Mink, J. W. (1996). The basal ganglia: focused selection and inhibition of competing motor programs. Prog. Neurobiol. 50, 381–425.
Mink, J. W., and Thach, W. T. (1991). Basal ganglia motor control. II. Late pallidal timing relative to movement onset and inconsistent pallidal coding of movement parameters. J. Neurophysiol. 65, 301–329.
Morris, G., Nevet, A., Arkadir, D., Vaadia, E., and Bergman, H. (2006). Midbrain dopamine neurons encode decisions for future action. Nat. Neurosci. 9, 1057–1063.
Nambu, A. (2004). A new dynamic model of the cortico-basal ganglia loop. Prog. Brain Res. 143, 461–466.
Opris, I., and Bruce, C. J. (2005). Neural circuitry of judgment and decision mechanisms. Brain Res. Brain Res. Rev. 48, 509–526.
Pasquereau, B., Nadjar, A., Arkadir, D., Bezard, E., Goillandeau, M., Bioulac, B., Gross, C. E., and Boraud, T. (2007). Shaping of motor responses by incentive values through the basal ganglia. J. Neurosci. 27, 1176–1183.
Peng, H., Long, F., and Ding, C. (2005). Feature selection based on mutual information: criteria of max-dependency, max-relevance, and min-redundancy. IEEE Trans. Pattern Anal. Mach. Intell. 27, 1226–1238.
Samejima, K., and Doya, K. (2007). Multiple representations of belief states and action values in corticobasal ganglia loops. Ann. N. Y. Acad. Sci. 1104, 213–228.
Schultz, W. (2006). Behavioral theories and the neurophysiology of reward. Annu. Rev. Psychol. 57, 87–115.
Keywords: decision making, electrophysiology, striatum, globus pallidus, primate
Citation: Garenne A, Pasquereau B, Guthrie M, Bioulac B and Boraud T (2011) Basal ganglia preferentially encode context dependent choice in a two-armed bandit task. Front. Syst. Neurosci. 5:23. doi: 10.3389/fnsys.2011.00023
Received: 01 February 2011; Paper pending published: 25 February 2011;
Accepted: 20 April 2011; Published online: 09 May 2011.
Edited by:
James M. Tepper, Rutgers, The State University of New Jersey, USAReviewed by:
Izhar Bar-Gad, Bar-Ilan University, IsraelBrian Hyland, University of Otago, New Zealand
Copyright: © 2011 Garenne, Pasquereau, Guthrie, Bioulac and Boraud. This is an open-access article subject to a non-exclusive license between the authors and Frontiers Media SA, which permits use, distribution and reproduction in other forums, provided the original authors and source are credited and other Frontiers conditions are complied with.
*Correspondence: Thomas Boraud, UMR Centre National de la Recherche Scientifique 5293, 146, rue Leo Saignat, 33 076 Bordeaux Cedex, France. e-mail:dGJvcmF1ZEB1LWJvcmRlYXV4Mi5mcg==