- 1 Department of Computational Biology, KTH Royal Institute of Technology, Stockholm, Sweden
- 2 Stockholm Brain Institute, Stockholm, Sweden
- 3 Intelligent Signal Processing Group, Department of Electronics, University of Barcelona, Barcelona, Spain
- 4 Artificial Olfaction Group, Institute for Bioengineering of Catalonia, Barcelona, Spain
In sensory processing of odors, the olfactory bulb is an important relay station, where odor representations are noise-filtered, sharpened, and possibly re-organized. An organization by perceptual qualities has been found previously in the piriform cortex, however several recent studies indicate that the olfactory bulb code reflects behaviorally relevant dimensions spatially as well as at the population level. We apply a statistical analysis on 2-deoxyglucose images, taken over the entire bulb of glomerular layer of the rat, in order to see how the recognition of odors in the nose is translated into a map of odor quality in the brain. We first confirm previous studies that the first principal component could be related to pleasantness, however the next higher principal components are not directly clear. We then find mostly continuous spatial representations for perceptual categories. We compare the space spanned by spatial and population codes to human reports of perceptual similarity between odors and our results suggest that perceptual categories could be already embedded in glomerular activations and that spatial representations give a better match than population codes. This suggests that human and rat perceptual dimensions of odorant coding are related and indicates that perceptual qualities could be represented as continuous spatial codes of the olfactory bulb glomerulus population.
1 Introduction
The sense of smell is crucial for survival, including for aspects such as maternal bonding, mating, kinship recognition, territorial defense, and aggressive behavior (Savic et al., 2001; Sanchez-Andrade and Kendrick, 2009; Tirindelli et al., 2009; Martin et al., 2011; Schleich and Zenuto, 2010; Dehnhard, 2011). In order to perform functions underlying these behaviors, animals have to create meaningful perceptual representations of odor qualities. The mechanisms of information processing are still poorly understood, however they seem remarkably similar across phyla and species (Ache and Young, 2005).
Sensory nerves from olfactory receptor neurons (ORNs) converge and terminate on the surface of the OB in spheroidal neuropil structures, called glomeruli, where they synapse with periglomerular interneurons and OB output neurons (MT cells; Kosaka et al., 1998). Glomeruli are thought to be functional unit and each glomerulus is target for convergence of axons from many ORNs of just one type of olfactory receptor (OR; Kauer and Cinelli, 1993; Mori, 1999; Bozza et al., 2002). In the rat, each ORN expresses only one of a possible 1,200 OR and axons from ORNs that express the same OR are bundled in approximately two glomeruli at stereotyped positions (typically one on each of both mirror-symmetric sides) of about 1800 in each olfactory bulb (OB, Zhang et al., 2007). In this fashion, each odorant elicits a specific map of glomerular activation (Ressler et al., 1994). Olfactory bulb output neurons, mitral and tufted cells (MT cells), project to primary olfactory cortical areas, such as olfactory cortical areas, such as the anterior olfactory nucleus, piriform cortex, olfactory tubercle and lateral entorhinal cortex, and the amygdala (Shipley et al., 2008).
It has been found in independent studies that molecular odorant properties map differentially to clustered spatial locations (e.g., Vassar et al., 1994; Meister and Bonhoeffer, 2001; Lodovichi et al., 2003; Mori et al., 2006, 2009; Johnson and Leon, 2007) and our results from a systematic large-scale study of glomerular activity indicated that coding of molecular properties is in continuous zones and locally very restricted (Auffarth et al., 2011a). Experimental evidence and model studies point toward localized encoding also of behavioral or perceptual aspects in the rat (e.g., Dielenberg and McGregor, 2001; Linster et al., 2001; Kobayakawa et al., 2007; Raman and Gutierrez-Osuna, 2009; Sakano, 2010; Auffarth et al., 2011b). Furthermore, there could be an organizing principle of relative spatial locations of odorant representations, related to chemical similarity (Uchida et al., 2000; Cleland et al., 2002; Johnson et al., 2004), distribution of ORNs on the epithelium (rhinotopy; Miyamichi et al., 2005; Johnson and Leon, 2007), and behavioral relevance. As an example for the latter, carbon-chain length is a molecular property related to discrimination ability (Cleland et al., 2002) and there is evidence for a progression by carbon-chain length (Johnson et al., 2004). On the other side, Wilson and Stevenson (2006) argued for the plausibility of synergistic (population) coding in the OB and there is evidence supporting this interpretation (e.g., Rubin and Katz, 1999; Haddad et al., 2010). Leon and Johnson (2009) suggested that perceptually driven behavior could serve as a starting point to evaluate the coding schemes. We took this approach and analyzed population and spatial schemes with respect to coding for perceptual categories.
We applied a non-parametric statistical test with a bootstrapping resampling technique for localization of receptive fields (Auffarth et al., 2011a) on rat glomerular activation images taken by 2-deoxyglucose radiography by the group around Michael Leon and Brett Johnson (Johnson et al., 2002). We then compared glomerular response patterns at population and spatial levels to human-rated perceptual similarities of odorant categories (Zarzo, 2008; Zarzo and Stanton, 2009). Finally we discuss the relevance of our results for the understanding of early olfactory processing.
2 Materials and Methods
2.1 Data
In this study, we used a set of 2-deoxyglucose autoradiography images of glomeruli covering the entire lamina of the rat olfactory bulb1 (Johnson et al., 1999, 2006). These images, part of a data base collected by Michael Leon’s group over the course of many years, have formed the basis of many published studies. In the experiments, they stimulated unanesthetized and freely respiring animals with odorants. The animals were then sectioned and imaged. Each of these images corresponds to glomerular responses to one particular odorant. Figure 1, adapted from Leon and Johnson (2003), denominates locations in anatomical terms in a ventral-centered chart for the glomerular layer of the rat olfactory bulb. Leon and Johnson took averages over left and right bulbs and averaged over arrays from all animals exposed to the same concentration of the same odorant (around 3 to 5 animals for each combination). It is known that patterns are very similar across animals and that glomeruli are at stereotypic positions (Soucy et al., 2009). According to Johnson et al. (2006), the 2-DG method is capable of resolution down to a single glomerulus, it is however unable to resolve temporal dynamics of activity.
In our pre-processing of activation maps, we started with 472 maps, of which some represented responses to identical odorants in different concentrations. We eliminated by visual inspection five highly saturated images, where most or all of the glomeruli were activated and took means over responses corresponding to the same odorant in different concentrations (222 maps in on average 3 concentrations). Ten maps had to be discarded because of missing odorant information. This selection left us with 308 point maps, each of which corresponding to odor-induced glomerular activities. Missing values, caused by loss of tissue on the knife during cryosectioning, others due to loss of tissue during removal of the bulbs from the skull using microdissecting scissors, were ignored in the analysis, which left us with 1834 pixels. We mean-centered all pixels and normalized deviations to standard unit to compensate for differences in absolute pixel intensities.
Leon and Johnson provided molecular and flavor descriptors with the odorants. Flavors are such as sweet, camphoraceous, floral, or minty. We grouped flavors into odor qualities, perceptual odor categories, as described by Zarzo (2008). These categories are florals, cleaner, foul, woody, medicinal, nutty/spicy, balsamic, fruity, alcohol, oily, herbacious, musk, vegetable, and green.
We had odor quality information for 258 compounds.
2.2 Methods
2.2.1 Localization of Coding Zones
In order to determine the activation loci for odor qualities, we applied the following procedure: we tested statistically for each glomerulus whether it showed significant differences with respect to the odor quality by comparing responses to odors that belonged to a odor quality with responses to odors that did not belong to the odor quality. We did the comparison using the Wilcoxon ranked-sum test (also called Mann–Whitney U test). The test was applied within a bootstrap (Efron, 1982) resampling procedure in order to estimate distributions from small sample sizes and to account for unequal number of maps. We thresholded p-values at 5% significance. Thus, we found for each odor qualities, the glomeruli which are activated differentially. More details about this method are available (Auffarth et al., 2011a).
2.2.2 Analysis of perceptual spaces
Zarzo (2008) and Zarzo and Stanton (2009) published an analysis of perfumers’ odor perception space (BH, Boelens and Haring, 1981) and of similarity odor similarity ratings in a cross-cultural study (Chrea, 2004). In order to find out, how well relative spatial situations of representations reflected perceptual orderings, we mined figures of the two papers by Zarzo, which plotted perceptual distances (in the first two principal components) between odorant qualities. We extracted three distance matrices, DChrea, DBH small, DBH big. Chrea corresponded to floral/cosmetic, cleaner, foul/musty, woody, medicinal, nutty/spicy, balsamic, and fruity. BH small corresponded to qualities floral, woody, medicinal, balsamic, fruity. BH big included all categories of BH small and additionally oily, vegetable, and green. For the oily category we took means of the neighboring fatty and buttery.
We investigated how perceptual categories are represented in the glomerular responses and if the encoding makes use of the whole population of a layer (population code) or just a few units are enough (spatial code). We compared matches to perceptual categories from both population activities of the entire glomerular layer and from spatial codes in order to see which code reflected better these perceptual orderings.
As for population code, we took the mean map over all activity maps corresponding to the same odor quality, that is, we took the mean of relative levels of activation across the ensemble (cf. Rubin and Katz, 1999; Lin et al., 2006; Cleland et al., 2007). As an ordering between properties we calculated the Euclidean distances between these mean maps, thus obtaining pair-wise distances between odor qualities based on population code, DP.
As for the ordering between coding maps (as obtained by the statistical method described above), we applied the Hausdorff distance (cf. Alt et al., 2003), which calculates distances between two-dimensional shapes and therefore incorporates information about shape, size, orientation, and coding center distance. This is to test the perceptual relevance of distances between coding sites.
We applied the modified Hausdorff distance function (Dubuisson and Jain, 1994) between vertices of pairs of encoding zones. Vertices were obtained by running a two-dimensional Sobel edge filter over the binarized coding zone maps, as per above. Informally, the Hausdorff distance is the farthest distance of closest points between two sets. Formally, given X and Y, two non-empty subsets of a metric space (M,d), their Hausdorff distance dH(X,Y) is defined as follows:

Thus we obtained a matrix of pair-wise differences between properties based on coding maps, DS.
We normalized each of the three matrices of pair-wise distances to unit sum and calculated the sum of the absolute error between both DP, DS, and DChrea, DBH small, DBH big.
We also added spatial and population information linearly with the same weight to see if combined they provided a better fit to the perceptual space. For the baseline, 100,000 sets of points were sampled from random uniform distributions. Then the distances from their pair-wise distances to perceptual space was calculated.
3 Results
3.1 Correlations between Principal Components and Odor Qualities
As first analysis of coding on population level and to replicate results from the literature, we computed correlations between the principal component scores and odor categories. The significant correlations of categories to first four principal components are listed in Table 1.
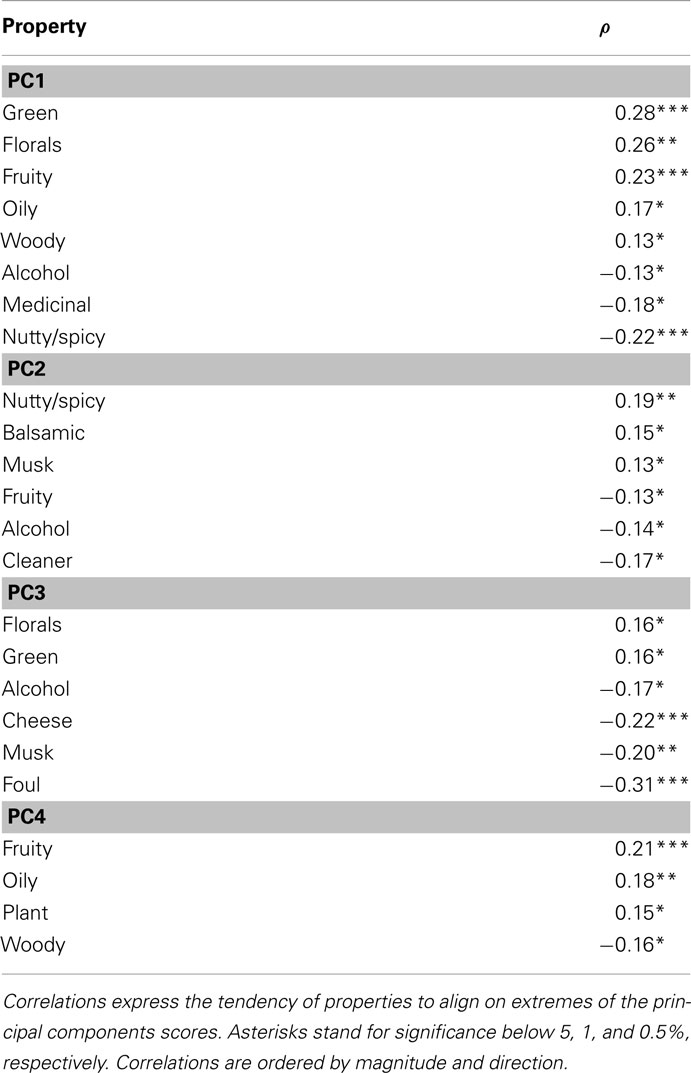
Table 1. Significant correlations between odorant categories and the first four principal components.
With PC1, green, florals, and fruity aligned best, while nutty/spicy and medicinal aligned in opposite direction. Oily and woody also have significant correlations with PC1, alcohol correlates negatively. As for PC2, nutty/spicy correlates most, followed by balsamic and musk. Cleaner, alcohol, and fruity correlate in opposite direction. With PC3, florals and green correlate positively, while several qualities, foul, musk, cheese, and alcohol, correlate negatively. Fruity, oily, and plant correlate with PC4, while woody correlates negatively.
3.2 Localization of Coding Zones
Images in Figure 2 show responsive regions for some odor qualities and molecular properties. For interpretation of these maps, please note that patterns are bilaterally symmetric across a tilted axis around the horizontal midline (cf. Khan et al., 2010). For anatomic terms of location, please refer to Figure 1.
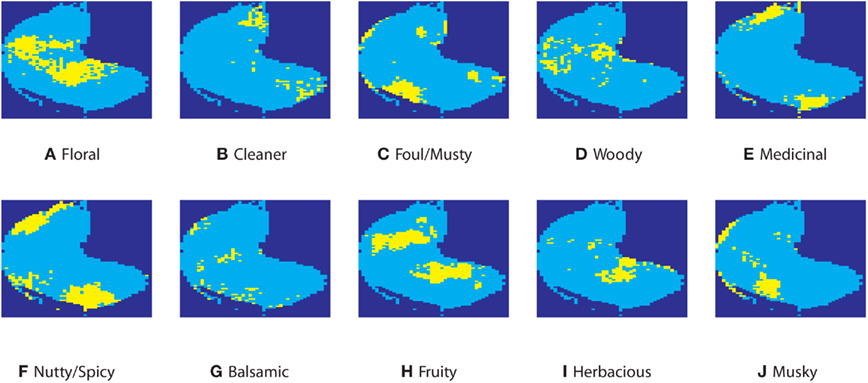
Figure 2. Representations of perceptual categories. Representations for odors (indicated in yellow) corresponding to floral (A), cleaner (B), foul (C), woody (D), medicinal (E), nutty/spicy (F), balsamic (G), fruity (H), herbacious (I), and musky (J).
For odor qualities, in Figure 2, yellow indicates the responsive region. Figures 2A–J show regions activated by a selected set of odor qualities. They include all odor qualities relevant to DChrea.
3.3 Perceptual Coding Space
Table 2 gives an indication about how accurately perceptual ordering of odors is reflected in the glomerular code. Distances, DP, generated from population code, based on the activity of all neurons, are compared to perceptual orderings of odors. Distances between spatial zones, DS, are compared to perceptual spaces. The numbers give the sum of the absolute error of fit. Figure 3 visualizes the perceptual spaces and the spaces between category representations.
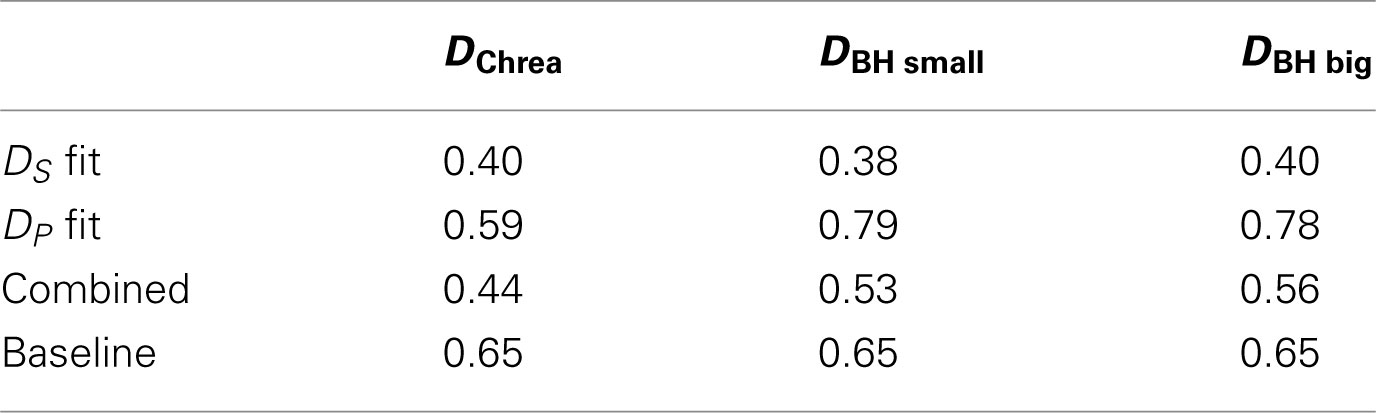
Table 2. Absolute (percentage) error of fit between coding spaces (DP, DS) and perceptual spaces (DChrea, DBH small, DBH big).
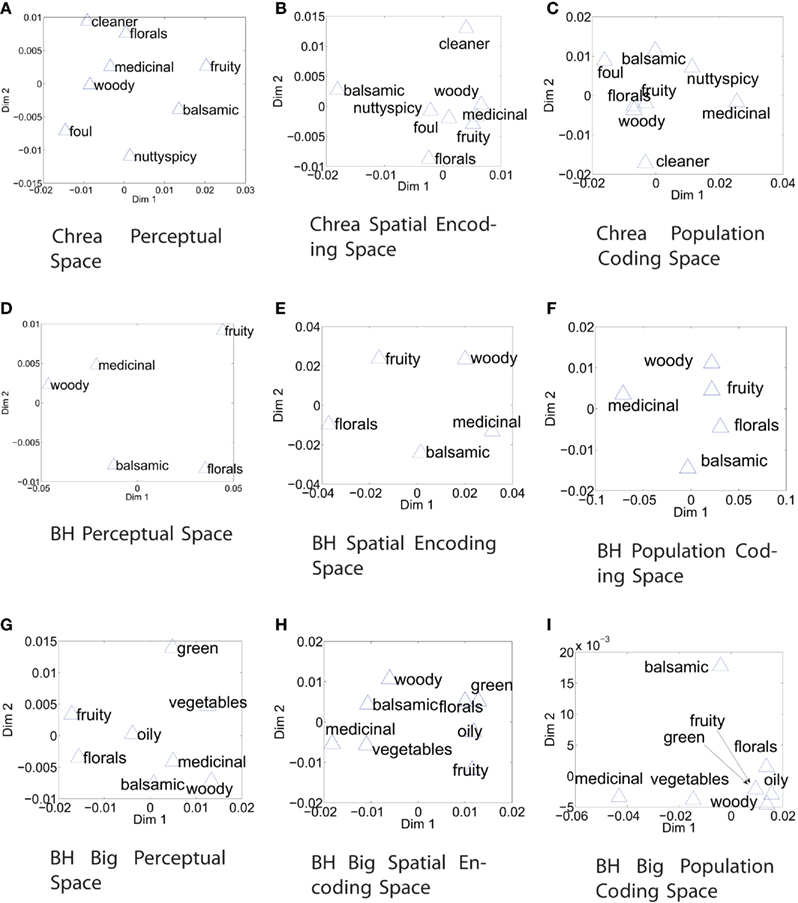
Figure 3. Absolute error of fit between coding spaces (DP, DS) and perceptual spaces (DChrea, DBH small, DBH big). Perceptual spaces constructed from pair-wise distances reduced to two-dimensions by multi-dimensional scaling. Please note for comparison that what matters is the relation between categories, i.e., the relative distances. (A) Shows the perceptual space in Chrea (2004), as per Zarzo (2008). (B) Shows the space as given by distances between spatial codes. (C) Shows the space as given by distances between activations of the entire glomerular layer, independent of spatial arrangement of glomeruli. The panel in the middle compares the perceptual space for the BH small data set (D), the distances between the categories in the spatial codes (E), and the population codes (F). The lower panel shows the perceptual ordering for the BH big data set (G), these distances between spatial codes (H), and population codes (I). In (I), fruity and green are indistinguishable.
4 Discussion
Many studies of odorant coding in the OB give evidence that odor codes are represented on the levels of glomeruli and M/T cells by spatio-temporal codes (cf. Laurent, 1997; Leon and Johnson, 2009), while interpretations differ with regard to four questions. These concern distributed vs. local representations, population vs. spatial codes, organizing principles of spatial relationships between representations, and the importance of temporal patterning. One of the most important advantages of out data is to have activity-related signals extending toward nearly the complete glomerular layer, which is quite different from other measurement methods, where typically only very few units can be taken into account. Therefore, from our dataset, we can calculate aspects of the spatial component of the code in terms of perceptual correlations.
We cannot resolve the temporal dynamics, however, since the 2-DG method disregards the temporal component. Spors and Grinvald (2002) described maintained spatial patterns at early and late stages of stimulation and suggested that latency of glomerular activation carries information about odorant concentration. Laurent (2002) discussed how bulbar dynamics on different timescales could subserve functions in optimizing stimulus representation, such as sparsening, feature binding, and decorrelation and how read-out mechanisms could make use of temporal patterns in different ways, however he concluded that after optimization of representations, initial complexity could be reduced. If or how much disregarding time dynamics impacts our results is not clear. Comparison of temporal response characteristics is generally difficult because of differences between recording techniques, because responses over time can show complicated spiking patterns, and because onsets to an odor have different time lags over receptors and may show different spiking patterns (Galizia et al., 2010).
Arguments for and against local and spatial coding are not easily separated. Spatial coding refers to the case, where encoding for a certain type of information is spatially constricted to a part of the units of a population. Spatial coding can be distributed or localized. The concept of spatial coding is opposed to that of population coding, where information is coded in the responses of the population (we take this in the strict sense of referring to the entire population, cf. Rubin and Katz, 1999; Lin et al., 2006; Cleland et al., 2007). A general argument for distributed representations comes from models based on plausibility (Hinton and McClelland, 1986). Experimental support for distributed representations is however not conclusive (e.g., Haxby et al., 2001; Malach et al., 2002). General arguments for local and spatial coding can be found in Udin and Fawcett (1988) and Singer (1994), with evidence coming from studies of receptive fields in early visual and auditory processing, where afferent input from primary sensory neurons is spatially segregated (cf. Swindale, 2008; Humphries et al., 2010). As for evidence supporting populations codes in the OB, Rubin and Katz (1999) showed that global responses to similar molecules were more correlated than global responses to different molecules. Youngentob et al. (2006) found differences of global activity patterns very similar to differences in a discrimination task. Haddad et al. (2010) analyzed population activity of glomeruli and MT cells by principal component analysis and found significant correlations, which also supports the argument for population coding.
4.1 Correlations from Principal Component Analysis
We extracted flavor descriptors from two publicly available resources. We then obtained odor categories by assignment of odorant flavor descriptors. We first tried to relate principal components of glomerular response patterns to perceptual categories.
Haddad et al. (2010) analyzed population activity of glomeruli and MT cells from different studies and found that the first principal component was highly correlated to approach or withdrawal, or pleasantness. Furthermore, they found that the first principal component correlated with many odor flavors. We did not, however, find analyses of higher principal components, such as presented here.
It is known that edibility and pleasantness are related (cf. Khan et al., 2007) and that further that in humans florals and fruity are pleasant, while nutty/spicy, medicinal, and alcohol are rather unpleasant categories (Zarzo, 2008; Zarzo and Stanton, 2009). From that perspective, we speculate, even though we do not have information about how pleasant odors are for rats, that the first principal component could be related to pleasantness, confirming earlier studies.
The second component corresponds neither to pleasantness, nor to edibility. However, it could correspond to toxicity, similar as suggested by Haddad et al. (2010). The third and fourth principal components are very similar and run along dimensions corresponding to an axis between woody and fruity, between opposing odor effects as presented by Zarzo and Stanton (2009).
4.2 Representation of Perceptual Qualities in the Olfactory Bulb
We computed representations for perceptual categories from rat glomerular response patterns to odors corresponding to these categories. This was done in two manners, according to population and according to spatial coding schemes. As for population coding, we took the strict interpretation of uniform weights for all units. As for spatial coding, we used a statistical method to calculate receptive fields corresponding to odor qualities for each glomerulus. We compared how well a spatial code and population code matched human perceptual experiences. We found that for odor qualities many representations are spatially continuous as can be seen in Figure 2.
It has been suggested before (e.g., Leon and Johnson, 2009) that spatial distances could be related to behavioral (or perceptual) differences. In order to test this hypothesis, we investigate relations of distances between regions that are preferentially active for perceptual categories to perceptual orderings.
We analyzed variability of glomerular responses over different odor qualities. Table 2 gives an indication about how accurately the perceptual space is reflected by the two different coding methods. Distances DP are the distances between population codes, and DS are distances between zones that showed differential activation in response to odor qualities. Both were compared to perceptual orderings of odors. The errors between the perceptual space and the spatial encoding space is smaller compared to the population coding space for all three data sets and spatial coding spaces performed better than a baseline generated from random points.
We found that errors of fit between spatial representations were similar to perceptual orderings by human subjects (as compared to baseline) and were smaller than for population codes. Therefore, our results suggest that spatial coding has a stronger relation to perception than population codes and confirm previous studies which suggest a spatial coding.
However, we also found for DChrea and DBH big a better match than baseline for population coding of perceptual categories. Indication for population coding comes also from the principal component analysis.
The combined codes did not improve matches, but were better than could be expected from averages on DChrea and DBH small. Therefore, we think that no clear conclusion can be drawn with regard to whether population and spatial coding complement each other.
We found that main orientations of perceptual and encoding spaces matched, however internal distances over these two spaces were different, which suggests that perceptual qualities could already be represented to some degree in codes on the OB level.
As for processing beyond the glomerular layer, it is not straightforward to infer MT cell firing patterns from our data, although there are papers that show that inference from glomerular imaging signals to MT cell activation can be reliably done (e.g., Moreaux and Laurent, 2008). We do not pretend to know about the spatial patterning of responses on the MT layer, even though papers about the relative spatial organization of the glomerular and MT layers exist. Willhite et al. (2006) documented evidence for a columnar organization by OR identity that extends across glomerular, mitral cell, and granule cell layers, however quantitative analysis showed evidence that mitral cells instead of being activated by a single glomerulus rather receive input from diverse and spatially dispersed set of glomeruli (Fantana et al., 2008), which could facilitate a function as higher-order coincidence detectors (cf. Yaksi et al., 2009).
Evidence is accumulating that ORs and glomeruli are internalizations of environmental regularities (e.g., Dielenberg and McGregor, 2001; Hommel et al., 2002; Khan et al., 2007; Kobayakawa et al., 2007; Semmelhack and Wang, 2009; Sakano, 2010). In a model of map formation in the olfactory bulb, Auffarth et al. (2011b) used a topographic self-organizing learning process based on co-activation of olfactory neurons that accounts for at least part of the organization of perceptual representations. Together, results could imply the existence of a spatially organized network of detectors.
5 Conclusion
We extracted perceptual categories of odors from the literature and computed spatial and population codes for these categories from rat glomerular response patterns to odors corresponding to these perceptual categories. We first tried to relate principal components of glomerular response patterns to perceptual categories and confirm previous finding about population coding. We found that representations for categories are largely confined as spatial continuous zones. We then compared how well spatial and population codes reflected human-subject perceptual ordering of odors and found that the distances between local coding regions span a space that is similar to human-subject perceptual ordering, and that the distances between spatial representations reflected a perceptual odor space more accurately than a population code.
The early olfactory system translates information about molecules into a space relevant for perception and action by exploiting regularities in the odor space to extract perceptually and behaviorally relevant information. Where exactly the mapping between physico-chemical properties and perceptual qualities occurs is unclear, however representations in the piriform cortex have been found to reflect perceptual qualities (Howard et al., 2009). Our results indicate that this transformation already begins on the level of the first sensory projection.
We find confirmative evidence in association with perceptual correlations which other studies showed before for molecular properties, localized representations which are of relatively small extent, i.e., spatial codes. Last and most important is our result that these spatial continuous representations are organized in a meaningful way in terms of perceptual relationships. Together results could suggest a link between human-subjective reports and objective physiological data of the rat and indicate that population and spatial codes could work together.
Conflict of Interest Statement
The authors declare that the research was conducted in the absence of any commercial or financial relationships that could be construed as a potential conflict of interest.
Acknowledgments
The authors thank the group around Michael Leon and Brett Johnson at the University of California at Irvine for collecting and providing the data. One of the authors, Benjamin Auffarth, was supported by a grant from the federal state government of Catalonia (formació de personal investigador, FI) during some time of the study. Benjamin Auffarth thanks Kensaku Mori and Manuel Zarzo for introducing him to olfaction and perceptual dimensions of odors. We are grateful to Bernhard Kaplan for proofreading of and commenting on an early draft of this paper. We thank both reviewers who made many valuable comments and thereby helped to improve this article.
Footnote
- ^Michael Leon, Brett Johnson, et al. “Glomerular Activity Response Archive.” Available at http://gara.bio.uci.edu/
References
Ache, B. W., and Young, J. M. (2005). Olfaction: diverse species, conserved principles. Neuron 48, 417–430.
Alt, H., Knauer, C., and Wenk, C. (2003). Comparison of distance measures for planar curves. Algorithmica 38, 45–58.
Auffarth, B., Gutierrez-Galvez, A., and Marco, S. (2011a). Statistical analysis of coding for molecular properties in the olfactory bulb. Front. Syst. Neurosci. 5:62.
Auffarth, B., Kaplan, B., and Lansner, A. (2011b). Map formation in the olfactory bulb by axon guidance of olfactory neurons. Front. Syst. Neurosci. 5:84.
Boelens, H., and Haring, H. (1981). Molecular Structure and Olfactive Quality. Technical Report. Bussum: Naarden International.
Bozza, T., Feinstein, P., Zheng, C., and Mombaerts, P. (2002). Odorant receptor expression defines functional units in the mouse olfactory system. J. Neurosci. 22, 3033–3043.
Chrea, C. (2004). Culture and odor categorization: agreement between cultures depends upon the odors. Food Qual. Prefer. 15, 669–679.
Cleland, T. A., Johnson, B. A., Leon, M., and Linster, C. (2007). Relational representation in the olfactory system. Proc. Natl. Acad. Sci. U.S.A. 104, 1953–1958.
Cleland, T. A., Morse, A., Yue, E. L., and Linster, C. (2002). Behavioral models of odor similarity. Behav. Neurosci. 116, 222–231.
Dehnhard, M. (2011). Mammal semiochemicals: understanding pheromones and signature mixtures for better zoo-animal husbandry and conservation. Int. Zoo Yearb. 45, 55–79.
Dielenberg, R. A., and McGregor, I. S. (2001). Defensive behavior in rats towards predatory odors: a review. Neurosci. Biobehav. Rev. 25, 597–609.
Dubuisson, M.-P., and Jain, A. (1994). “A modified Hausdorff distance for object matching,” in Pattern Recognition, 1994. Vol. 1 – Conference A: Computer Vision and Image Processing, Proceedings of the 12th IAPR International Conference on Pattern Recognition, Jerusalem, Israel, 566–568.
Efron, B. (1982). “The jackknife, the bootstrap and other resampling plans,” in CMBS Regional Conference Series in Applied Mathematics (Philadelphia: Society for Industrial and Applied Mathematics), 92.
Fantana, A. L., Soucy, E. R., and Meister, M. (2008). Rat olfactory bulb mitral cells receive sparse glomerular inputs. Neuron 59, 802–814.
Galizia, C. G., Münch, D., Strauch, M., Nissler, A., and Ma, S. (2010). Integrating heterogeneous odor response data into a common response model: a DoOR to the complete olfactome. Chem. Senses 35, 551–563.
Haddad, R., Weiss, T., Khan, R., Nadler, B., Mandairon, N., Bensafi, M., Schneidman, E., and Sobel, N. (2010). Global features of neural activity in the olfactory system form a parallel code that predicts olfactory behavior and perception. J. Neurosci. 30, 9017–9026.
Haxby, J. V., Gobbini, M. I., Furey, M. L., Ishai, A., Schouten, J. L., and Pietrini, P. (2001). Distributed and overlapping representations of faces and objects in ventral temporal cortex. Science 293, 2425–2430.
Hinton, G., and McClelland, J. (1986). “Distributed representations,” in Parallel Distributed Processing, eds D. E. Rumelhart, and J. L. McClelland PDP Research Group (Cambridge, MA: MIT Press), 77–109.
Hommel, B., Müsseler, J., Aschersleben, G., and Prinz, W. (2002). The theory of event coding (TEC): a framework for perception and action planning. Behav. Brain Sci. 24, 849–878.
Howard, J. D., Plailly, J., Grueschow, M., Haynes, J.-D., and Gottfried, J. A. (2009). Odor quality coding and categorization in human posterior piriform cortex. Nat. Neurosci. 12, 932–938.
Humphries, C., Liebenthal, E., and Binder, J. R. (2010). Tonotopic organization of human auditory cortex. Neuroimage 50, 1202–1211.
Johnson, B. A., Farahbod, H., Xu, Z., Saber, S., and Leon, M. (2004). Local and global chemotopic organization: general features of the glomerular representations of aliphatic odorants differing in carbon number. J. Comp. Neurol. 480, 234–249.
Johnson, B. A., Ho, S. L., Xu, Z., Yihan, J. S., Yip, S., Hingco, E. E., and Leon, M. (2002). Functional mapping of the rat olfactory bulb using diverse odorants reveals modular responses to functional groups and hydrocarbon structural features. J. Comp. Neurol. 449, 180–194.
Johnson, B. A., and Leon, M. (2007). Chemotopic odorant coding in a mammalian olfactory system. J. Comp. Neurol. 503, 1–34.
Johnson, B. A., Woo, C. C., Hingco, E. E., Pham, K. L., and Leon, M. (1999). Multidimensional chemotopic responses to n-aliphatic acid odorants in the rat olfactory bulb. J. Comp. Neurol. 409, 529–548.
Johnson, B. A., Xu, Z., Pancoast, P., Kwok, J., Ong, J., and Leon, M. (2006). Differential specificity in the glomerular response profiles for alicyclic, bicyclic, and heterocyclic odorants. J. Comp. Neurol. 499, 1–16.
Kauer, J. S., and Cinelli, A. R. (1993). Are there structural and functional modules in the vertebrate olfactory bulb? Microsc. Res. Tech. 24, 157–167.
Khan, A. G., Parthasarathy, K., and Bhalla, U. S. (2010). Odor representations in the mammalian olfactory bulb. Wiley Interdiscip. Rev. Syst. Biol. Med. 2, 603–611.
Khan, R. M., Luk, C.-H., Flinker, A., Aggarwal, A., Lapid, H., Haddad, R., and Sobel, N. (2007). Predicting odor pleasantness from odorant structure: pleasantness as a reflection of the physical world. J. Neurosci. 27, 10015–10023.
Kobayakawa, K., Kobayakawa, R., Matsumoto, H., Oka, Y., Imai, T., Ikawa, M., Okabe, M., Ikeda, T., Itohara, S., Kikusui, T., Mori, K., and Sakano, H. (2007). Innate versus learned odour processing in the mouse olfactory bulb. Nature 450, 503–508.
Kosaka, K., Toida, K., Aika, Y., and Kosaka, T. (1998). How simple is the organization of the olfactory glomerulus?: the heterogeneity of so-called periglomerular cells. Neurosci. Res. 30, 101–110.
Laurent, G. (2002). Olfactory network dynamics and the coding of multidimensional signals. Nat. Rev. Neurosci. 3, 884–895.
Leon, M., and Johnson, B. A. (2003). Olfactory coding in the mammalian olfactory bulb. Brain Res. Rev. 42, 23–32.
Leon, M., and Johnson, B. A. (2009). Is there a space-time continuum in olfaction? Cell. Mol. Life Sci. 66, 2135–2150.
Lin, D. Y., Shea, S. D., and Katz, L. C. (2006). Representation of natural stimuli in the rodent main olfactory bulb. Neuron 50, 937–949.
Linster, C., Johnson, B. A., Yue, E., Morse, A., Xu, Z., Hingco, E. E., Choi, Y., Choi, M., Messiha, A., and Leon, M. (2001). Perceptual correlates of neural representations evoked by odorant enantiomers. J. Neurosci. 21, 9837–9843.
Lodovichi, C., Belluscio, L., and Katz, L. C. (2003). Functional topography of connections linking mirror-symmetric maps in the mouse olfactory bulb. Neuron 38, 265–276.
Malach, R., Levy, I., and Hasson, U. (2002). The topography of high-order human object areas. Trends Cogn. Sci. (Regul. Ed.) 6, 176–184.
Martin, S. J., Helanterä, H., and Drijfhout, F. P. (2011). Is parasite pressure a driver of chemical cue diversity in ants? Proc. Biol. Sci. 278, 496–503.
Meister, M., and Bonhoeffer, T. (2001). Tuning and topography in an odor map on the rat olfactory bulb. J. Neurosci. 21, 1351–1360.
Miyamichi, K., Serizawa, S., Kimura, H. M., and Sakano, H. (2005). Continuous and overlapping expression domains of odorant receptor genes in the olfactory epithelium determine the dorsal/ventral positioning of glomeruli in the olfactory bulb. J. Neurosci. 25, 3586–3592.
Moreaux, L., and Laurent, G. (2008). A simple method to reconstruct firing rates from dendritic calcium signals. Front. Neurosci. 2:176–185.
Mori, K. (1999). The olfactory bulb: coding and processing of odor molecule information. Science 286, 711–715.
Mori, K., Matsumoto, H., Tsuno, Y., and Igarashi, K. M. (2009). Dendrodendritic synapses and functional compartmentalization in the olfactory bulb. Ann. N. Y. Acad. Sci. 1170, 255–258.
Mori, K., Takahashi, Y. K., Igarashi, K. M., and Yamaguchi, M. (2006). Maps of odorant molecular features in the Mammalian olfactory bulb. Physiol. Rev. 86, 409–433.
Raman, B., and Gutierrez-Osuna, R. (2009). “Relating sensor responses of odorants to their organoleptic properties by means of a biologically-inspired model of receptor neuron convergence onto olfactory bulb,” in Biologically Inspired Signal Processing for Chemical Sensing, eds A. Gutiérrez, and S. Marco (Heidelberg: Springer), 93–108.
Ressler, K. J., Sullivan, S. L., and Buck, L. B. (1994). Information coding in the olfactory system: evidence for a stereotyped and highly organized epitope map in the olfactory bulb. Cell 79, 1245–1255.
Rubin, B., and Katz, L. (1999). Optical imaging of odorant representations in the mammalian olfactory bulb. Neuron 23, 499–511.
Sanchez-Andrade, G., and Kendrick, K. M. (2009). The main olfactory system and social learning in mammals. Behav. Brain Res. 200, 323–335.
Savic, I., Berglund, H., Gulyas, B., and Roland, P. (2001). Smelling of odorous sex hormone-like compounds causes sex-differentiated hypothalamic activations in humans. Neuron 31, 661–668.
Schleich, C. E., and Zenuto, R. (2010). Testing detection and discrimination of vegetation chemical cues in the subterranean rodent Ctenomys talarum. Ethol. Ecol. Evol. 22, 257–264.
Semmelhack, J. L., and Wang, J. W. (2009). Select Drosophila glomeruli mediate innate olfactory attraction and aversion. Nature 459, 218–223.
Shipley, M. T., Ennis, M., and Puche, A.C. (2008). “The olfactory system,” in The Rat Nervous System, 3rd Edn. ed. G. Paxinos (San Diego, CA: Elsevier Inc.), 611–622.
Singer, W. (1994). “The organization of sensory motor representations in the neocortex: a hypothesis based on temporal coding,” in Attention and Performance XV: Conscious and Nonconscious Information Processing, eds C. Umilta, and M. Moscovitch (Cambridge, MA: MIT Press), 77–107.
Soucy, E. R., Albeanu, D. F., Fantana, A. L., Murthy, V. N., and Meister, M. (2009). Precision and diversity in an odor map on the olfactory bulb. Nat. Neurosci. 12, 210–220.
Spors, H., and Grinvald, A. (2002). Spatio-temporal dynamics of odor representations in the mammalian olfactory bulb. Neuron 34, 301–315.
Tirindelli, R., Dibattista, M., Pifferi, S., and Menini, A. (2009). From pheromones to behavior. Physiol. Rev. 89, 921.
Uchida, N., Takahashi, Y. K., Tanifuji, M., and Mori, K. (2000). Odor maps in the mammalian olfactory bulb: domain organization and odorant structural features. Nat. Neurosci. 3, 1035–1043.
Udin, S. B., and Fawcett, J. W. (1988). Formation of topographic maps. Annu. Rev. Neurosci. 11, 289–327.
Vassar, R., Chao, S. K., Sitcheran, R., Nuñez, J. M., Vosshall, L. B., and Axel, R. (1994). Topographic organization of sensory projections to the olfactory bulb. Cell 79, 981–991.
Willhite, D. C., Nguyen, K. T., Masurkar, A. V., Greer, C. A., Shepherd, G. M., and Chen, W. R. (2006). Viral tracing identifies distributed columnar organization in the olfactory bulb. Proc. Natl. Acad. Sci. U.S.A. 103, 12592–12597.
Wilson, D., and Stevenson, R. (2006). Learning to Smell: Olfactory Perception From Neurobiology to Behavior. Baltimore, MD: Johns Hopkins University Press.
Yaksi, E., von Saint Paul, F., Niessing, J., Bundschuh, S. T., and Friedrich, R. W. (2009). Transformation of odor representations in target areas of the olfactory bulb. Nat. Neurosci. 12, 474–482.
Youngentob, S. L., Johnson, B. A., Leon, M., Sheehe, P. R., and Kent, P. F. (2006). Predicting odorant quality perceptions from multidimensional scaling of olfactory bulb glomerular activity patterns. Behav. Neurosci. 120, 1337–1345.
Zarzo, M. (2008). Psychologic dimensions in the perception of everyday odors: pleasantness and edibility. J. Sens. Stud. 23, 354–376.
Zarzo, M., and Stanton, D. T. (2009). Understanding the underlying dimensions in perfumers’ odor perception space as a basis for developing meaningful odor maps. Atten. Percept. Psychophys. 71, 225–247.
Keywords: olfaction, olfactory bulb, glomeruli, spatial coding, population coding, memory organization, odor quality, perception
Citation: Auffarth B, Gutierrez-Galvez A and Marco S (2011) Continuous spatial representations in the olfactory bulb may reflect perceptual categories. Front. Syst. Neurosci. 5:82. doi: 10.3389/fnsys.2011.00082
Received: 22 August 2011; Accepted: 20 September 2011;
Published online: 10 October 2011.
Edited by:
Raphael Pinaud, University of Oklahoma Health Sciences Center, USAReviewed by:
Max L. Fletcher, University of Tennessee Health Science Center, USADavid C. Willhite, Yale University School of Medicine, USA
Copyright: © 2011 Auffarth, Gutierrez-Galvez and Marco. This is an open-access article subject to a non-exclusive license between the authors and Frontiers Media SA, which permits use, distribution and reproduction in other forums, provided the original authors and source are credited and other Frontiers conditions are complied with.
*Correspondence: Benjamin Auffarth, Department of Computational Biology, School of Computer Science and Communication, KTH Royal Institute of Technology, Albanova Universitetscentrum, Roslagstullsbacken 35, S-11421 Stockholm, Sweden. e-mail:YXVmZmFydGhAY3NjLmt0aC5zZQ==