- 1 Rotman Research Institute, Baycrest Centre, Toronto, ON, Canada
- 2 Department of Psychology, University of Toronto, ON, Canada
- 3 Cognitive Neuroscience Unit, Montreal Neurological Institute, McGill University, Montreal, QC, Canada
Neural activity is irregular and unpredictable, yet little is known about why this is the case and how this property relates to the functional architecture of the brain. Here we show that the variability of a region’s activity systematically varies according to its topological role in functional networks. We recorded the resting-state electroencephalogram (EEG) and constructed undirected graphs of functional networks. We measured the centrality of each node in terms of the number of connections it makes (degree), the ease with which the node can be reached from other nodes in the network (efficiency) and the tendency of the node to occupy a position on the shortest paths between other pairs of nodes in the network (betweenness). As a proxy for variability, we estimated the information content of neural activity using multiscale entropy analysis. We found that the rate at which information was generated was largely predicted by centrality. Namely, nodes with greater degree, betweenness, and efficiency were more likely to have high information content, while peripheral nodes had relatively low information content. These results suggest that the variability of regional activity reflects functional embedding.
1. Introduction
The functional architecture of the cerebral cortex is configured in a manner that balances local segregation and global integration, endowing the system with a high degree of complexity (Tononi et al., 1994). The complexity of the system allows for a diverse dynamic répertoire and is reflected in the information content and variability of neural activity. The variability inherent in neurophysiological recordings is now considered a fundamental dynamical property of the brain, allowing for spontaneous transitions among several metastable states (Ghosh et al., 2008; McIntosh et al., 2008, 2010; Deco et al., 2009, 2011; Jirsa et al., 2010).
What determines the variability of neural activity? If brief functional associations allow information to be integrated, then regions which participate in the greatest number of functional subnetworks will facilitate the flow of information by bridging and integrating other regions that would be otherwise disconnected and topologically distant (Sporns et al., 2000, 2004; Stam, 2004; Stam and Reijneveld, 2007). Therefore, information content should depend on connectivity and more specifically on the functional integration enabled by individual nodes.
Recent findings are consistent with this notion and suggest that the information content of regional activity may depend on the configuration of functional networks and on their participation in such networks. For example, information – measured using entropy-based metrics – increases during normal brain development (McIntosh et al., 2008, 2010; Lippé et al., 2009; Mišic et al., 2010). This may reflect intensified global integration relative to local segregation and a gradual shift from local, clustered information processing in children to distributed processing in adults (Fair et al., 2009; Supekar et al., 2009; Hagmann et al., 2010). Indeed, the developmental increase in entropy is most robust in areas such as the precuneus and posterior cingulate (Mišic et al., 2010), which are known to be central to the topology of resting-state functional brain networks (Hagmann et al., 2008, 2010; Buckner et al., 2009; Tomasi and Volkow, 2010). Moreover, just as functional networks reconfigure in response to task-induced perturbations (Bassett et al., 2006), so too do spatial patterns of entropy (Lippé et al., 2009; Mišic et al., 2010).
In the present study we tested the notion that the functional embedding of a brain region is related to the information content of neural activity from that region. We addressed this hypothesis by recording the electroencephalogram (EEG) from 56 participants in the resting-state with eyes open and eyes closed. Whole-head functional networks were constructed by measuring the phase lag index (PLI; Stam et al., 2007, 2009) between all pairs of electrodes. Centrality of individual nodes was assessed in terms of node degree, betweenness, and efficiency. The degree of individual nodes was indexed by counting the number of connections they make with other nodes in the network. Betweenness was measured as the fraction of all shortest paths in the network that pass through the node (Freeman, 1977, 1978). Regional efficiency was calculated as the inverse of the minimum path length (Latora and Marchiori, 2001; Achard and Bullmore, 2007; Bassett et al., 2009). Thus, regions which have a short minimum path to all other regions will have high efficiency. We predicted that the centrality of a region (indexed by degree, betweenness, and efficiency) will be associated with greater information content.
We quantified the information content of regional activity using multiscale entropy (MSE) analysis (Costa et al., 2002, 2005). As an entropy-based measure, MSE is sensitive to the complexity of the signal and will assign low values to both completely regular and to uncorrelated random signals. Moreover, the multiscale nature of the index takes into account the fact that physiological dynamics underlying the expressed information are likely to unfold over multiple temporal scales (Honey et al., 2007). Although functional magnetic resonance imaging (fMRI) would have allowed us to delineate functional networks with greater precision, the number of data points required to estimate entropy from empirical time series is quite large and necessitated an imaging technique with fast sampling such as EEG.
2. Materials and Methods
2.1. EEG Acquisition
Fifty-six (29 male) healthy children 10 years old (mean 10.0, SD 0.393 years) participated in the study (see Poulsen et al. (2009) for details). The protocol was approved by the Research Ethics Board of the Montreal Neurological Institute and Hospital. The participants were asked to keep their eyes open or closed in 8 alternating 30 s epochs (4 each). The electroencephalogram (EEG) was continuously recorded from 128 scalp locations using a HydroCel geodesic sensor net (Electrical Geodesics, Inc., Eugene, OR) referenced to the vertex (Cz). The signal was digitized at a rate of 500 Hz. Impedances did not exceed 60 kΩ. All offline signal processing and artifact correction was performed using the EEGLAB toolbox (Delorme and Makeig, 2004) for MATLAB (Mathworks, Inc.). Data were then average-referenced, digitally filtered (band-pass: 0.5–55 Hz; notch: 60 Hz) and epoched into 30 s segments. Only the middle 20 s of each epoch (5–25 s) were used in the analysis to avoid excessive contamination associated with opening and closing of the eyes. In the absence of a true baseline, the temporal mean was subtracted from each epoch. Ocular (blinks and lateral eye movements) and muscle artifacts were identified and subtracted on a subject-by-subject basis using the Infomax independent components analysis (ICA) algorithm (Bell and Sejnowski, 1995) implemented in EEGLAB. The analyses described below produced identical results for the eyes closed and eyes open resting-state runs, so for brevity only data from the eyes closed runs are displayed in the rest of this report.
2.2. Multiscale Entropy (MSE)
In multiscale entropy (MSE) analysis (Costa et al., 2002, 2005) each single trial time series is downsampled to multiple temporal scales and sample entropy (SE; Richman and Moorman, 2000) is calculated for each scale. For a given temporal scale τ, the corresponding time series is derived by averaging data points in non-overlapping windows of length τ from the original time series (τ = 1 corresponds to the original time series). The SE algorithm calculates the conditional probability that any two sequences of (m + 1) data points will be similar to each other given that they were similar for the first m points, which reflects the degree of regularity in a given time series. The SE metric is the negative of the natural logarithm of this quantity, so higher values of SE are associated with less regular and more variable time series. In the present study, pattern length was set to m = 2 and the similarity criterion to r = 0.5. The pattern length (otherwise known as the embedding dimension) was judged to be optimal following the method proposed by (Small and Tse, 2004). The similarity criterion (also known as the tolerance) was chosen following (Richman and Moorman, 2000). MSE was calculated for each of the 128 channels and averaged across epochs.
2.3. Functional Connectivity
The biggest challenge in estimating functional associations between EEG or magnetoencephalogram (MEG) surface sensors is the confounding influence of volume conduction (Nunez et al., 1997). Electromagnetic activity originating from a single brain region may be observed instantaneously by several scalp electrodes and misinterpreted as a functional connection. This zero-lag “blurring” tends to inflate the strength of short-length, neighbor-to-neighbor statistical interdependencies. Moreover, in EEG the effect is exacerbated if the montage involves an active reference (Nunez et al., 1997). One approach to address this problem has been to reformat EEG recorded with respect to an active reference to a reference-free montage, such as the bipolar (Rubinov et al., 2009a). Although the technique can be extended to include multiple bipolar orientations, it may still miss certain dipoles (Schiff, 2005). Another approach has been to estimate patterns of functional connectivity among reconstructed sources and to define the graph in source space (De Vico Fallani et al., 2007, 2008). However, source reconstruction techniques do not produce unique solutions and therefore different assumptions and models may lead to different results. Moreover, many common localization techniques such as beam forming operate by changing the covariance structure of the observed data.
To minimize the effect of volume conduction, we opted to use a measure of functional connectivity that is insensitive to spurious coherencies due to volume conduction, known as the Phase Lag Index (PLI; Stam et al., 2007, 2009). The measure takes advantage of the fact that functional connectivity between two channels due to volume conduction or an active reference cannot produce phase delays. Thus, PLI attempts to quantify the distribution of phase differences between two signals but is explicitly insensitive to differences that center around 0 mod π. As a result, PLI indexes synchronization between electrodes in a way that is minimally affected by volume conduction.
2.4. Graph Extraction and Analysis
As we sought to compare aspects of network structure with information, which was indexed by SE at multiple time scales, we extracted functional connectivity graphs at multiple time scales as well. Thus, each EEG epoch was downsampled using the same procedure as for MSE and functional connectivity was estimated for each of the resultant 20 coarse-grained time series. PLI was computed between all pairs of electrodes for each epoch, scale, subject, and condition, yielding a series of 128 × 128 matrices. For each time scale, these matrices were then averaged across the four epochs to yield subject- and condition-specific association matrices.
The importance of individual nodes was quantified by directly computing measures of centrality from each subject- and condition-specific weighted graph. All network measures except regional efficiency were computed using MATLAB routines implemented in the Brain Connectivity Toolbox (Rubinov and Sporns, 2010). The degree of each node was determined by taking the sum of all weighted connections to other nodes in the network. In order to calculate regional efficiency and betweenness for weighted graphs, we first defined an inverse mapping from weight to length. Thus, the length of a weighted edge between regions i and j (di,j) was calculated as the inverse of the edge weight between those two regions (wi,j)

The path length between any two nodes in the network can then be calculated as the sum of the lengths of edges along the path. Regional efficiency was calculated by taking the inverse of the harmonic mean of the minimum path length between a given node and all nodes in the network (Latora and Marchiori, 2001, 2003; Achard and Bullmore, 2007). Thus, if the minimum path length between regions i and j (i, j = 1, 2,…, N, and i ≠ j) is Li,j, the efficiency of region i (Ei) is given by

The normalized betweenness of a node j is the proportion of all shortest paths between all pairs of nodes i and k (ρik) that also pass through j (ρijk)

Together, the three measures offer complementary information about the topological role of a given node. Degree indexes the connectedness of a given node. Efficiency tells us how close a node is to other nodes in the network. Betweenness measures the tendency of a node to serve as a way station between other pairs of nodes in the network. Each of these regional measures was averaged across subjects and then correlated with SE, separately for the two conditions and for each temporal scale.
3. Results
To demonstrate and broadly summarize the regional variation in MSE, Figure 1A shows the spatial pattern of SE values that have been summed across all 20 scales. These patterns demonstrate that total MSE tends to be greatest at medial and mid-lateral posterior parietal channels, in concordance with previous studies (McIntosh et al., 2008). The complete MSE curves for two representative channels (marked by black dots in Figure 1A) are displayed in Figure 1B. The curves show increasing entropy with coarse-graining, similar to previous studies using electrophysiological (McIntosh et al., 2008) and neuromagnetic recordings (Mišic et al., 2010; Figure 1). Moreover, the curves show large differences in SE across most temporal scales, except the first two. This demonstrates that differences in variability occur at multiple time scales and suggests that network structure should likewise be considered at different levels of coarse-graining.
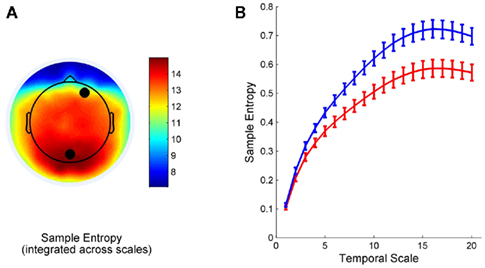
Figure 1. Multiscale entropy curves. Values of SE are summed across temporal scales and the spatial distribution is shown in (A). The complete multiscale entropy (MSE) curves for two representative channels (marked by black circles), showing SE at each level of coarse-graining, are displayed in (B). Error bars indicate standard errors of the mean.
The overall correspondence between information entropy and network embedding is explored in Figure 2. Node degree, regional efficiency, and betweenness were positively associated with entropy (Figure 2, top row). The relationships were statistically significant and were observed at all temporal scales (p ≪ 10−3). On average, node degree, efficiency, and betweenness accounted for approximately 44, 70, and 42% of the variance in SE across electrodes. We also observed an effect of time scale on the relationship between network embedding and MSE, whereby correlations were slightly weaker for fine time scales and increased with coarse-graining (Figure 2, middle row). This was expected given the fact that coarse-graining acts as a low-pass filter. Thus, the broad-band signal at fine time scales contains higher frequencies in addition to lower frequencies and estimates of phase synchronization are likely to be less robust. In contrast, the coarse-grained representations of the signal contain a narrower band of lower frequencies and therefore estimates of phase synchronization are more likely to be reliable.
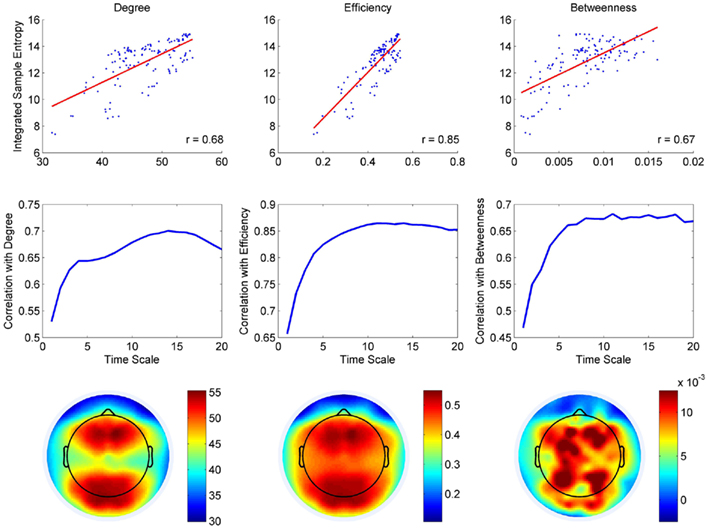
Figure 2. Multiscale entropy and functional embedding. Top row: scatter plots and regression lines depict the relationship between SE and centrality across all electrodes (both have been integrated across temporal scales). Middle row: the correlation coefficient between SE and each of the three network measures is plotted as a function of temporal scale. Bottom row: Scalp distributions for the three types of centrality. The measures were calculated for functional connectivity graphs at each time scale and then integrated across scales as a summary measure.
The bottom row of Figure 2 shows the scale-specific spatial distributions of node degree, regional efficiency, and betweenness, summed across all scales. Importantly, the pattern of gradations was similar for SE (Figure 1A) and the three network metrics. For all three measures, the highest values were observed at medial posterior channels and this peak typically extended around the vertex, forming a ring-like relief pattern over the superior aspect of the scalp, with a minute dip at the vertex. In some instances, the correspondence was not perfect and there were also minor differences. For example, some anterior channels had relatively high efficiency while the same was not true for SE. Likewise, the topographical map for betweenness did not perfectly match the map for SE, but this is not surprising since betweenness was not as good a predictor of SE as the other two measures of centrality. Overall, comparison of topographic maps for SE and centrality revealed the possibility of two separate effects: a posterior parietal peak in centrality that matched a similar peak in variability, as well as a fronto-central peak in centrality that was not consistently accompanied by a peak in SE.
In general, the effects associated with node degree and efficiency were similar and this is to be expected because the two measures are complementary. Namely, efficiency is the average minimum path length between a given node and all others, while node degree quantifies the total number of connections. In the case of weighted graphs, if direct connections are also the shortest, then the measures will index the same thing and may be redundant. We quantified this overlap and found that, on average, direct connections constituted 40% of the shortest paths. Therefore, there was considerable overlap but also significant divergence. Moreover, calculation of betweenness did not take into account this rather large proportion of direct connections and this may explain why the effects and the topography associated with betweenness was not as similar to degree and efficiency as they were to each other.
Although we took several steps to ensure that the effects of volume conduction would be minimized, we still sought to assess the extent to which this may have affected our estimates of synchronization. In practice, simultaneous activation due to spatial smearing will tend to be most pronounced between proximal electrodes. Therefore, for each possible pair of electrodes we calculated a vector of Euclidean distances as well as a vector of PLI values. We then estimated the degree to which physical distance between channels could predict the strength of their functional coupling, using both mutual information and simple linear regression. Both methods revealed that Euclidean distance typically accounted for 24% of the variance in functional connectivity (consistently across all time scales). This suggests a reasonable effect of spatial proximity on coupling strength and even compares favorably to previous studies using MEG (Bassett et al., 2006).
4. Discussion
The temporal evolution of neural activity is far from regular. This element of unpredictability is indicative of the information carried by the signal and represents an important facet of the brain’s organization at multiple scales of time and space (McIntosh et al., 2008, 2010; Deco et al., 2011). We have shown that the centrality of network nodes strongly predict the information content of their physiological activity during the resting-state.
Ephemeral functional associations among distributed regions constitute the dominant patterns of information flow in the brain. Vertices with many connections as well as short or direct paths to the rest of the network are conduits for system-wide communication. These nodes engender the exchange of information both within and between communities (Sporns et al., 2007). The fact that highly central areas also generate information at a high rate is consistent with the notion that they facilitate functional integration. Similar results were uncovered in a recent theoretical study which explored the relationship between node dynamics and connectivity using a simple deterministic model (Rubinov et al., 2009b). The authors found that highly connected, central nodes were more likely to receive discordant inputs and as a result exhibited variable, high-dimensional dynamics. Conversely, peripheral nodes were more likely to receive homogeneous inputs and exhibited less variable, low-dimensional dynamics.
Our data support the idea that the variability observed in neural activity is an important facet of the functional organization of the brain. Variability may take many forms and can be measured in a multitude of ways, from information content of neurophysiological signals (McIntosh et al., 2008) to SD of hemodynamic responses (Garrett et al., 2010). Increased variability is associated with many benefits, both for behavioral performance and physiology. For example, greater brain signal variability is linked to accurate responses and stable response times (McIntosh et al., 2008; Mišic et al., 2010). Network reorganization during healthy development is associated with increased variability (McIntosh et al., 2008; Lippé et al., 2009; Mišic et al., 2010). Conversely, pathologically low variability is associated with tissue damage due to seizures (Protzner et al., 2010), as well as autism spectrum disorder (Bosl et al., 2011).
The inherent variability of neurophysiological signals represents the trajectory of a metastable dynamical system with non-linearities (Ghosh et al., 2008; Deco et al., 2009, 2011; Jirsa et al., 2010). As the brain typically occupies a high-energy state, small intrinsic fluctuations (for example, due to low-fidelity cellular processes; Faisal et al., 2008) are sufficient to perturb the system and induce excursions to other states/configurations. Thus, the stochastic characteristics of local physiology cause the system to continuously traverse the state space and confer a high degree of flexibility to adapt to changing demands in the external environment (Breakspear et al., 2010). This fluid reconfiguration is reflected by the variable dynamics observed in gross neurophysiological recordings.
In the present study we measured the connectivity of all nodes with respect to the entire brain and then matched regional differences in connectivity with differences in variability. In future studies it would be interesting to focus on one select region and to manipulate the connectivity of that region using some form of stimulation or cognitive task. This complementary approach would allow us to study how the information generated in a given brain region dynamically changes under different external and internal conditions.
4.1. Methodological Considerations
Graphs are a natural model for anatomical networks, but their application to functional networks is subject to several methodological issues (Bullmore and Sporns, 2009; Rubinov and Sporns, 2010). First, graph theoretic measures of centrality tend to be based on the importance of shortest paths (such as betweenness and efficiency in the present study) and this may not be an accurate model for a system such as the brain where information is likely to be transmitted along multiple parallel paths that are not necessarily the shortest. Second, it is difficult to infer the directionality of functional connections and this limits the range of local and global metrics that can be calculated for the network. In the present investigation we chose simple indices to capture connectedness, centrality, and functional integration of individual nodes that are not sensitive to directionality of edges in the network. Third, whole-brain functional networks can only be defined with limited precision using neurophysiological measurements over the scalp. This was a necessary compromise that allowed us to estimate information content over a broad-band, which would not have been feasible with high-resolution methods such as fMRI.
In the present investigation, the resting-state paradigm was used instead of some cognitive task with multiple trials for two reasons. First, in the no-task setting cognitive processing could not be biased by external demand. Second, the resting-state allowed many measurements (long time series) to be recorded such that information and functional connectivity could be reliably estimated. For these reasons resting-state paradigms are often used to estimate functional connectivity and centrality (De Vico Fallani et al., 2007; Rubinov et al., 2009a; Boersma et al., 2011), as well as entropy (Escudero et al., 2006; Park et al., 2007; Mizuno et al., 2010; Takahashi et al., 2010; Bosl et al., 2011).
5. Conclusion
The dynamical properties of the brain give rise to a complex functional architecture that stays true to the underlying anatomy over long periods of time, but at short time scales reconfigures in a highly fluid fashion (Honey et al., 2007, 2009), thereby exploring its functional répertoire (Ghosh et al., 2008). The fluid landscape of functional associations drives and determines the statistical properties of neural activity. The present study demonstrates a link between functional topology and variability of neurophysiological activity.
Conflict of Interest Statement
The authors declare that the research was conducted in the absence of any commercial or financial relationships that could be construed as a potential conflict of interest.
Acknowledgments
The authors declare that the research was conducted in the absence of any commercial or financial relationships that could be construed as competing financial interests. This research was supported by grants from the Canadian Institutes of Health Research (CIHR) and Santa Fe Institute Consortium to Tomáš Paus and a J. S. McDonnell Foundation grant to Anthony R. McIntosh.
References
Achard, S., and Bullmore, E. (2007). Efficiency and cost of economical brain functional networks. PLoS Comput. Biol. 3, e17. doi:10.1371/journal.pcbi.0030017
Bassett, D., Bullmore, E., Meyer-Lindenberg, A., Apud, J., Weinberger, D., and Coppola, R. (2009). Cognitive fitness of cost-efficient brain functional networks. Proc. Natl. Acad. Sci. U.S.A. 106, 11747–11752.
Bassett, D., Meyer-Lindenberg, A., Achard, S., Duke, T., and Bullmore, E. (2006). Adaptive reconfiguration of fractal small-world human brain functional networks. Proc. Natl. Acad. Sci. U.S.A. 103, 19518–19523.
Bell, A., and Sejnowski, T. (1995). An information-maximization approach to blind separation and blind deconvolution. Neural Comput. 7, 1129–1159.
Boersma, M., Smit, D., de Bie, H., Van Baal, G., Boomsma, D., de Geus, E., Delemarre-van de Waal, H., and Stam, C. (2011). Network analysis of resting state eeg in the developing young brain: structure comes with maturation. Hum. Brain Mapp. 32, 413–425.
Bosl, W., Tierney, A., Tager-Flusberg, H., and Nelson, C. (2011). EEG complexity as a biomarker for autism spectrum disorder risk. BMC Med. 9, 18. doi:10.1186/1741-7015-9-18
Breakspear, M., Jirsa, V., and Deco, G. (2010). Computational models of the brain: from structure to function. Neuroimage 52, 727–730.
Buckner, R., Sepulcre, J., Talukdar, T., Krienen, F., Liu, H., Hedden, T., Andrews-Hanna, J., Sperling, R., and Johnson, K. (2009). Cortical hubs revealed by intrinsic functional connectivity: mapping, assessment of stability, and relation to Alzheimer’s disease. J. Neurosci. 29, 1860–1873.
Bullmore, E., and Sporns, O. (2009). Complex brain networks: graph theoretical analysis of structural and functional systems. Nat. Rev. Neurosci. 10, 186–198.
Costa, M., Goldberger, A., and Peng, C. (2002). Multiscale entropy analysis of complex physiologic time series. Phys. Rev. Lett. 89, 68102.
Costa, M., Goldberger, A., and Peng, C. (2005). Multiscale entropy analysis of biological signals. Phys. Rev. E Stat. Nonlin. Soft Matter Phys. 71, 021906.
De Vico Fallani, F., Astolfi, L., Cincotti, F., Mattia, D., Marciani, M., Salinari, S., Kurths, J., Gao, S., Cichocki, A., Colosimo, A., and Babiloni, F. (2007). Cortical functional connectivity networks in normal and spinal cord injured patients: evaluation by graph analysis. Hum. Brain Mapp. 28, 1334–1346.
De Vico Fallani, F., Astolfi, L., Cincotti, F., Mattia, D., Marciani, M., Tocci, A., Salinari, S., Witte, H., Hesse, W., Gao, S., Colosimo, A., and Babiloni, F. (2008). Cortical network dynamics during foot movements. Neuroinformatics 6, 23–34.
Deco, G., Jirsa, V., and McIntosh, A. (2011). Emerging concepts for the dynamical organization of resting-state activity in the brain. Nat. Rev. Neurosci. 12, 43–56.
Deco, G., Jirsa, V., McIntosh, A., Sporns, O., and Kötter, R. (2009). Key role of coupling, delay, and noise in resting brain fluctuations. Proc. Natl. Acad. Sci. U.S.A. 106, 10302–10307.
Delorme, A., and Makeig, S. (2004). EEGLAB: an open source toolbox for analysis of single-trial EEG dynamics including independent component analysis. J. Neurosci. Methods 134, 9–21.
Escudero, J., Abásolo, D., Hornero, R., Espino, P., and López, M. (2006). Analysis of electroencephalograms in Alzheimer’s disease patients with multiscale entropy. Physiol. Meas. 27, 1091.
Fair, D., Cohen, A., Power, J., Dosenbach, N., Church, J., Miezin, F., Schlaggar, B., and Petersen, S. (2009). Functional brain networks develop from a “local to distributed” organization. PLoS Comput. Biol. 5, e1000381. doi:10.1371/journal.pcbi.1000381
Faisal, A., Selen, L., and Wolpert, D. (2008). Noise in the nervous system. Nat. Rev. Neurosci. 9, 292–303.
Garrett, D., Kovacevic, N., McIntosh, A., and Grady, C. (2010). Blood oxygen level-dependent signal variability is more than just noise. J. Neurosci. 30, 4914–4921.
Ghosh, A., Rho, Y., McIntosh, A., Kötter, R., and Jirsa, V. (2008). Noise during rest enables the exploration of the brain’s dynamic repertoire. PLoS Comput. Biol. 4, e1000196. doi:10.1371/journal.pcbi.1000196
Hagmann, P., Cammoun, L., Gigandet, X., Meuli, R., Honey, C., Wedeen, V., and Sporns, O. (2008). Mapping the structural core of human cerebral cortex. PLoS Biol. 6, e159. doi:10.1371/journal.pbio.0060159
Hagmann, P., Sporns, O., Madan, N., Cammoun, L., Pienaar, R., Wedeen, V., Meuli, R., Thiran, J., and Grant, P. (2010). White matter maturation reshapes structural connectivity in the late developing human brain. Proc. Natl. Acad. Sci. U.S.A. 107, 19067–19072.
Honey, C., Kötter, R., Breakspear, M., and Sporns, O. (2007). Network structure of cerebral cortex shapes functional connectivity on multiple time scales. Proc. Natl. Acad. Sci. U.S.A. 104, 10240–10245.
Honey, C., Sporns, O., Cammoun, L., Gigandet, X., Thiran, J., Meuli, R., and Hagmann, P. (2009). Predicting human resting-state functional connectivity from structural connectivity. Proc. Natl. Acad. Sci. U.S.A. 106, 2035–2040.
Jirsa, V., Sporns, O., Breakspear, M., Deco, G., and McIntosh, A. (2010). Towards the virtual brain: network modeling of the intact and the damaged brain. Arch. Ital. Biol. 148, 189–205.
Latora, V., and Marchiori, M. (2001). Efficient behavior of small-world networks. Phys. Rev. Lett. 87(19):198701.
Latora, V., and Marchiori, M. (2003). Economic small-world behavior in weighted networks. Eur. Phys. J. B 32, 249–263.
Lippé, S., Kovacevic, N., and McIntosh, A. (2009). Differential maturation of brain signal complexity in the human auditory and visual system. Front. Hum. Neurosci. 3:48. doi:10.3389/neuro.09.048.2009
McIntosh, A., Kovacevic, N., and Itier, R. (2008). Increased brain signal variability accompanies lower behavioral variability in development. PLoS Comput. Biol. 4, e1000106. doi:10.1371/journal.pcbi.1000106
McIntosh, A., Kovacevic, N., Lippé, S., Garrett, D., Grady, C., and Jirsa, V. (2010). The development of a noisy brain. Arch. Ital. Biol. 148, 323–337.
Mišic, B., Mills, T., Taylor, M., and McIntosh, A. (2010). Brain noise is task-dependent and region-specific. J. Neurophysiol. 104, 2667–2676.
Mizuno, T., Takahashi, T., Cho, R., Kikuchi, M., Murata, T., Takahashi, K., and Wada, Y. (2010). Assessment of EEG dynamical complexity in Alzheimer’s disease using multiscale entropy. Clin. Neurophysiol. 121, 1438–1446.
Nunez, P., Srinivasan, R., Westdorp, A., Wijesinghe, R., Tucker, D., Silberstein, R., and Cadusch, P. (1997). EEG coherency. I: statistics, reference electrode, volume conduction, Laplacians, cortical imaging, and interpretation at multiple scales. Electroencephalogr. Clin. Neurophysiol. 103, 499–515.
Park, J., Kim, S., Kim, C., Cichocki, A., and Kim, K. (2007). Multiscale entropy analysis of EEG from patients under different pathological conditions. Fractals 15, 399–404.
Poulsen, C., Picton, T., and Paus, T. (2009). Age-related changes in transient and oscillatory brain responses to auditory stimulation during early adolescence. Dev. Sci. 12, 220–235.
Protzner, A., Valiante, T., Kovacevic, N., McCormick, C., and McAndrews, M. (2010). Hippocampal signal complexity in mesial temporal lobe epilepsy: a noisy brain is a healthy brain. Arch. Ital. Biol. 148, 289–297.
Richman, J., and Moorman, J. (2000). Physiological time-series analysis using approximate entropy and sample entropy. Am. J. Physiol. Heart Circ. Physiol. 47, 2039–2049.
Rubinov, M., Knock, S., Stam, C., Micheloyannis, S., Harris, A., Williams, L., and Breakspear, M. (2009a). Small-world properties of nonlinear brain activity in schizophrenia. Hum. Brain Mapp. 30, 403–416.
Rubinov, M., Sporns, O., Van Leeuwen, C., and Breakspear, M. (2009b). Symbiotic relationship between brain structure and dynamics. BMC Neurosci. 10, 55. doi:10.1186/1471-2202-10-55
Rubinov, M., and Sporns, O. (2010). Complex network measures of brain connectivity: uses and interpretations. Neuroimage 52, 1059–1069.
Small, M., and Tse, C. (2004). Optimal embedding parameters: a modelling paradigm. Physica D 194, 283–296.
Sporns, O., Chialvo, D., Kaiser, M., and Hilgetag, C. (2004). Organization, development and function of complex brain networks. Trends Cogn. Sci. 8, 418–425.
Sporns, O., Honey, C., and Kötter, R. (2007). Identification and classification of hubs in brain networks. PLoS ONE 2, e1049. doi:10.1371/journal.pone.0001049
Sporns, O., Tononi, G., and Edelman, G. (2000). Theoretical neuroanatomy: relating anatomical and functional connectivity in graphs and cortical connection matrices. Cereb. Cortex 10, 127–141.
Stam, C. (2004). Functional connectivity patterns of human magnetoencephalographic recordings: a “small-world”network? Neurosci. Lett. 355, 25–28.
Stam, C., De Haan, W., Daffertshofer, A., Jones, B., Manshanden, I., Van Cappellen van Walsum, A., Montez, T., Verbunt, J., De Munck, J., Van Dijk, B., Berendse, H., and Scheltens, P. (2009). Graph theoretical analysis of magnetoencephalographic functional connectivity in Alzheimer’s disease. Brain 132, 213–224.
Stam, C., Nolte, G., and Daffertshofer, A. (2007). Phase lag index: assessment of functional connectivity from multichannel EEG and MEG with diminished bias from common sources. Hum. Brain Mapp. 28, 1178–1193.
Stam, C., and Reijneveld, J. (2007). Graph theoretical analysis of complex networks in the brain. Nonlinear Biomed. Phys. 1, 1–19.
Supekar, K., Musen, M., and Menon, V. (2009). Development of large-scale functional brain networks in children. PLoS Biol. 7, e1000157. doi:10.1371/journal.pbio.1000157
Takahashi, T., Cho, R., Mizuno, T., Kikuchi, M., Murata, T., Takahashi, K., and Wada, Y. (2010). Antipsychotics reverse abnormal EEG complexity in drug-naive schizophrenia: a multiscale entropy analysis. Neuroimage 51, 173–182.
Tomasi, D., and Volkow, N. (2010). Functional connectivity density mapping. Proc. Natl. Acad. Sci. U.S.A. 107, 9885–9890.
Keywords: variability, entropy, degree, efficiency, connectivity, centrality, functional integration
Citation: Mišić B, Vakorin VA, Paus T and McIntosh AR (2011) Functional embedding predicts the variability of neural activity. Front. Syst. Neurosci. 5:90. doi: 10.3389/fnsys.2011.00090
Received: 05 June 2011;
Accepted: 21 October 2011;
Published online: 22 November 2011.
Edited by:
Claus Hilgetag, Jacobs University Bremen, GermanyReviewed by:
Mika Rubinov, University of Cambridge, UKGuadalupe Clara Garcia, Jacobs University, Germany
Copyright: © 2011 Mišić, Vakorin, Paus and McIntosh. This is an open-access article subject to a non-exclusive license between the authors and Frontiers Media SA, which permits use, distribution and reproduction in other forums, provided the original authors and source are credited and other Frontiers conditions are complied with.
*Correspondence: Bratislav Mišić, Rotman Research Institute, Baycrest, 3560 Bathurst Street, Toronto, ON, Canada M6A 2E1. e-mail:YnJhdGlzbGF2Lm1pc2ljQHV0b3JvbnRvLmNh