- ITC Faculty of Geo-Information Science and Earth Observation, University of Twente, Enschede, Netherlands
A Viewpoint on the Frontiers in Science Lead Article
A Digital Twin of the terrestrial water cycle: a glimpse into the future through high-resolution Earth observations
Key points
- State-of-the-art digital replicas of the Earth system, such as the Digital Twin Earth (DTE) for hydrology, simulate Earth processes to accurately monitor and predict various phenomena, such as extreme climate occurrences.
- The soil-plant-atmosphere interaction influences the fluxes of heat, water and carbon and understanding these processes allows for a more accurate prediction of ecosystem response to climate and environmental pressures.
- Developing a digital twin of the soil-plant-atmosphere continuum (SPAC) augmented with Earth Observation (SPACEO) will allow seamless integration of functional (cellular functioning at timescales of seconds) and spatiotemporal processes into regional weather and climate modeling systems, enhancing the accuracy of climate predictions and resource management.
Introduction
The Digital Twin Earth (DTE) is a rapidly evolving concept, approach, and technology that is fueling the development of Earth system science. The significance of digital twins is being increasingly recognized due to their capability to accurately forecast extreme climate events and predict environmental impacts. In relation to this, the European Commission launched the Destination Earth (DestinE) initiative which aims to develop DTEs that will accurately monitor and predict the interactions between weather, climate, ecosystem, and land, as well as the water-energy-carbon cycles therein (1). Contributing to this process, the European Space Agency (ESA) is also launching its program for the development of digital twin components to ensure enhanced and extensive use of the latest Earth observation (EO) technology in the development of DTEs. The paper by Brocca et al. (2) offers an example of this approach by fusing high-resolution EO data with advanced modeling, creating a four-dimensional DTE Hydrology data cube that can be used as forcing or validation datasets for Earth system models (ESMs), as well as for monitoring, prediction and testing scenarios.
The three main pillars of DTEs are physics-based models, machine learning (ML) algorithms, and data assimilation techniques (3). These all need to be high resolution, as is the case with ESMs (4, 5). The Brocca et al. (2) paper describes how this can be achieved for a hydrological application at 1 km resolution. However, many important processes and scales have not generally been included in such approaches. Despite this, significant progress has been made in the simulation and inclusion of multiple processes in ESMs in recent years, such as biogeochemical cycles, plant hydraulics, coupled moisture and heat transfer, freeze-thaw dynamics or groundwater flow (6–9). In addition, physics-informed machine learning has been increasingly incorporated into DTEs (10). The inclusion of these processes and machine learning algorithms would ensure the digital replica is representative of the real world and complex water-energy-carbon interactions.
Soil-plant-atmosphere continuum augmented with Earth observations
The Earth’s water, energy, and carbon cycles and their interactions are regulated by the most fundamental life-sustaining process: photosynthesis. Leaf-level photosynthesis is driven by incoming radiation (direct or diffuse), atmospheric conditions, structural and functional properties of vegetations and their rooting systems, soil-root interactions, soil properties, and groundwater dynamics. The highly dynamic coordinated mechanisms of photosynthesis at leaf level control the turbulent fluxes of heat, moisture, and carbon dioxide at the canopy level. These fluxes in turn impact local climate and cloud formation, which ultimately determine the partitioning between direct and diffuse radiation and, therefore, feedback to leaf-level photosynthesis. In order to understand this self-organized, fully coupled soil-plant-turbulence-cloud process, we argue that a digital twin of the soil-plant-atmosphere continuum (SPAC) augmented with EO information (SPACEO) is needed (Figure 1).
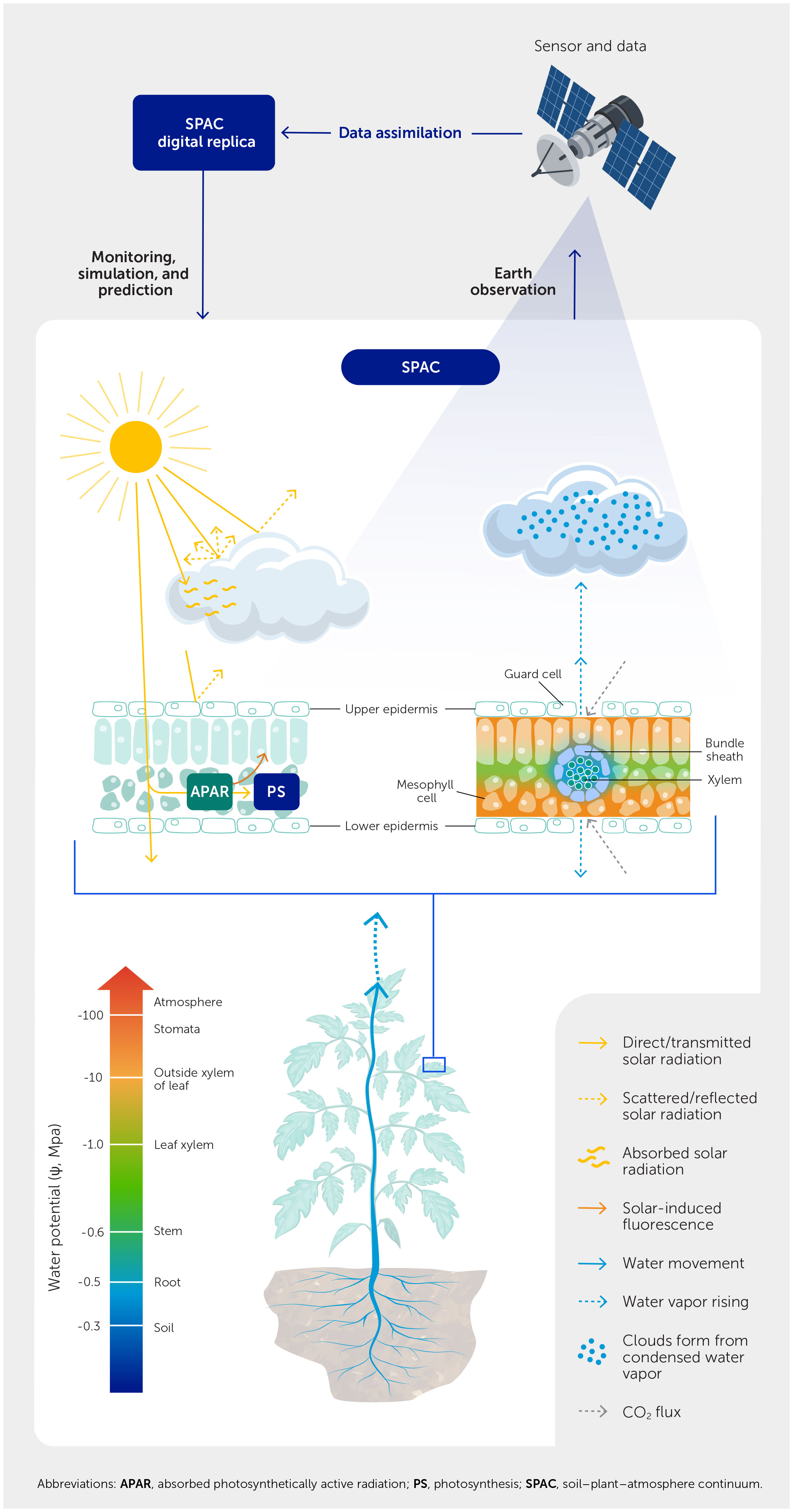
Figure 1 A digital twin of the soil-plant-atmosphere continuum (SPAC) augmented with Earth Observation (SPACEO). The SPACEO digital twin consists of three pillars: EO-collected data, the capability for assimilating such data (i.e., data assimilation), and a digital replica of the SPAC system. The soil-plant hydraulic connection is regulated by water potential gradients across the soil-root-stem-leaf-atmosphere interfaces, which is influenced by the cloud-radiation interaction. Clouds reflect, absorb, and scatter part of the solar radiation; the rest reaches the Earth’s surface and activates the photosynthetic process through photosynthetically active radiation (PAR). Together with PAR and other meteorological variables, soil-plant hydraulics modulate leaf water potential, which in turn influence photosynthesis and leaf gas exchange. The fluxes of water, energy, and carbon are determined by changes in CO2 and water vapor at a cellular scale. These cellular-scale processes will feed back to the cloud formation at the mesoscale via land surface water-energy-gas fluxes (e.g., latent and sensible heat fluxes), as well as volatile organic compounds that can serve as water vapor condensation nuclei for cloud formation.
Although the current generation of ESMs consider most water, energy, and carbon exchange processes, as well as feedbacks between the land and atmosphere, their spatiotemporal resolution and process descriptions are insufficient to fully capture the detailed soil-plant-turbulence-cloud feedbacks. For example, the duration of cloud passage and variation in cloud thickness lead to rapid cloud-induced perturbations in radiation, that in turn impact the turbulent fluxes of evapotranspiration and net ecosystem exchange at the timescale of 1 minute (11). It is important to capture such rapid fluctuations of radiation and surface turbulent fluxes to understand land-atmosphere interactions. A remaining open question here is how EO can help track such interactions at high spatiotemporal resolutions (i.e., sub-daily, <1 km), which is also highlighted by Brocca et al. (2) as one of several high-level challenges facing a fully operational DTE-Hydrology. The proposed SPACEO digital twin is expected to address the above question, enabling the mechanistic link between the SPAC processes and EO data.
Figure 1 shows an overview of the SPACEO digital twin, which consists of three main components: EO-collected data, the capability for assimilating such data, and a digital replica (model) of the SPAC processes. Water potentials across the soil, root, stem, and leaf enable the soil-plant hydraulic link within the SPAC system. Meanwhile, the EO data includes, among other elements, reflectance, chlorophyll fluorescence, and soil/plant water contents. Figure 1 also shows the interaction of solar radiation with clouds. Part of the radiation is absorbed and scattered by the clouds, while the rest reaches the Earth’s surface. This, in synergy with the soil-plant hydraulics, activates the photosynthetic process. The cloud-radiation interaction highlights the importance of considering the rapid fluctuation of radiation due to cloud passages as this changes land surface water-energy-carbon fluxes, which in turn impacts cloud formation. The SPAC model, which represents these interconnected processes, will be deployed to assimilate the EO data collected from the SPAC system. This will provide the optimal estimate and updated understanding of the SPAC system, to manage, for example, ecosystem’s climate/drought resilience.
Spatiotemporally collocated cloud and vegetation EO data
The previous sections have underscored the significance of clouds in water-energy-carbon fluxes and emphasized the necessity of measuring rapid fluctuations within these dynamics. At the same time, accurate measurement of the water and photosynthetic state of vegetation is also important as it can deliver detailed insights into the SPAC system.
Teuling et al. (12) used multiple METOSAT scenes to illustrate how forests can enhance cloud formation under specific surface weather conditions, starting discussions about the dependence of cloud self-organization on land surface properties. A straightforward way of addressing such dependence is to use spatiotemporally collocated EO data of cloud and vegetation (13). However, concurrently observing clouds and vegetation via EO is difficult when using optical sensors, as clouds prohibit the sensing of vegetation, preventing a thorough explanation of their collocated interactions.
Microwave remote sensing-based vegetation optical depth (VOD) provides a solution to this problem. VOD is proportional to vegetation water content (VWC) and biomass, depending on the wavelengths of satellite sensors, plant species, plant function types, and structures (e.g., height, diameter, biomass density, and gap size), and microwave sensors have all-weather observational capabilities (i.e., regardless of cloud cover) with penetration depth beyond the top layer of plant canopies. As such, microwave sensors (e.g., AMSR-E, Soil Moisture Ocean Salinity, and Soil Moisture Active Passive) have been widely used to provide VOD observations (25–50 km) to monitor regional soil-plant water status (14, 15).
Microwave radiometers with different frequencies, from Ku-band to P-band can be used to detect VWC across different heights in the canopy (16, 17), with higher frequencies most sensitive to leaves and branches and lower frequencies more sensitive to trunks and soils. As such, multisensor microwave-based VOD/VWC data have the potential to provide information on canopy structure that strongly impacts the vertical distribution of solar radiation and its direct and diffuse partitioning, which has a direct effect on plant photosynthesis and surface turbulent fluxes of moisture, heat, and carbon (13). Furthermore, since there is a close relationship between solar-induced chlorophyll fluorescence (SIF) and the photosynthetic apparatus (18), terrestrial SIF emitted between the red and near-infrared spectrum (with two peaks centred around 685 nm and 740 nm) has been used as an indicator of the photosynthetic state of vegetation.
Visible/infrared and microwave (MW) sensors provide complementary information on cloud microphysics for investigating cloud-radiation interactions (19, 20). Infrared (IR) sensors are more sensitive to smaller particles and the amount of cloud particles and water content; they can also “see” thin clouds that are otherwise transparent to MW sensors. However, IR radiation is not sensitive to cloud thickness and only provides information close to the cloud top. MW radiation, on the other hand, can “see” throughout the cloud deck and offer information on the total vertical cloud water content. As such cloud microphysical properties can be retrieved from the synergized use of passive MW and IR observations (20). Such cloud property datasets will be tremendously useful for understanding the rapid fluctuation of radiation induced by cloud passages, which consequently influence the land-atmosphere interaction processes as represented by the SPAC model.
Conclusion: think big, model small
Enhancing the representation of the SPAC via integration of EO data, in the same way as the DTE Hydrology discussed in Brocca et al. (2), will foster a more comprehensive understanding of the water-energy-carbon dynamics within an ecosystem.
A SPACEO digital twin would integrate functional processes (cellular functioning on the timescale of seconds) of multiscale land-atmosphere interaction processes with the EO-augmented characterization of the real-world, cloud-radiation interactions and the intertwined water-energy-carbon cycles. Furthermore, in order to directly assimilate EO data, the process-based SPAC model should be coupled to a radiative transfer model to enable the forward simulation of satellite signals (e.g., top of canopy radiance, reflectance, and fluorescence).
For example, the STEMMUS-SCOPE model simulates photosynthesis, leaf to canopy reflectance and SIF spectra, evapotranspiration, soil moisture and temperature, dynamic root growth, and corresponding root water uptake (9). As such, when it is coupled with a large-eddy simulation model of the atmospheric boundary layer (e.g., Dutch Atmospheric Large Eddy Simulation, or DALES) (21), it would facilitate the exploration of EO data across the VNIR-SWIR-TIR spectrum (VNIR, visible and near infrared: 0.4–1.0 μm; SWIR, short wave infrared: 1.0–2.5 μm; TIR, thermal infrared: 3–12 μm). To extend the capacity of the above model to also digest microwave (0.5–100 cm) satellite data, an observation model to simulate the brightness temperature could be coupled with backscattering coefficients such as the Community Land Active Passive Microwave Radiative Transfer Modelling (CLAP) platform (17).
The SPACEO can contribute to a better understanding of the interplay between (heterogeneous and yet organized) ecosystems, climate/weather extremes, and land-atmosphere interactions, with enhanced and extensive use of the latest EO technology. This is expected to improve on the interpretation of existing and future EO data and contribute to suggestions of novel future EO mission concepts. The SPACEO will facilitate monitoring and prediction of the interconnection between the soil-plant dynamics regulating CO2 flux and transpiration (on stomata, leaf, and canopy levels, and at timescales of seconds), as well as the atmospheric dynamics and cloud feedback (at turbulence length scale) that influence these soil-plant dynamics.
A SPACEO digital twin can serve as a forward observation-simulator, linking cellular-scale processes to EO observables, for example, by coupling the SIF signals to soil-plant hydraulics processes. This way, SIF remote sensing can be used to directly parameterize landscape vegetation traits for the SPAC model, calibrate internal functions (such as the gain-risk stomatal optimization approach), and constrain model predictions of water, energy, and carbon fluxes. Reciprocally, the SPACEO forward simulations can help explain the physical and physiological basis for the remotely sensed SIF–photosynthesis relationship and its variations across a range of species, geographic regions, and environmental conditions. As such, SPACEO can be deployed as a barometer on photosynthetic stress dynamics, which can be translated into a system of early warning and agricultural assessment of stress development and productivity in local, regional, and global food systems. Such a system will serve society’s growing need for crop production, food security, and other ecosystem services for a sustained quality of life by suggesting timely decisions for remedial measures to alleviate ecosystem stress from climate extremes.
Statements
Author contributions
YZ: Conceptualization, Data curation, Formal Analysis, Funding acquisition, Investigation, Methodology, Project administration, Resources, Software, Supervision, Validation, Visualization, Writing – original draft, Writing – review & editing. ZS: Writing – original draft, Writing – review & editing, Conceptualization, Data curation, Formal Analysis, Funding acquisition, Investigation, Methodology, Project administration, Resources, Software, Supervision, Validation, Visualization.
Funding
The author(s) declare financial support was received for the research, authorship, and/or publication of this article. This study received funding from The Netherlands Organisation for Scientific Research (NWO) KIC WUNDER project (grant no. KICH1. LWV02.20.004), Netherlands eScience Center, EcoExtreML project (grant ID. 27020G07), and Water JPI project “iAqueduct” (Project number: ENWWW.2018.5). We also thank the National Natural Science Foundation of China (grant no. 41971033) and the Fundamental Research Funds for the Central Universities, CHD (grant no. 300102298307). The funders were not involved in the study design, collection, analysis, interpretation of data, the writing of this article or the decision to submit it for publication.
Conflict of interest
The authors declare that the research was conducted in the absence of financial relationships that could be construed as a potential conflict of interest.
The author YZ declared a past co-authorship with the Lead Article’s author LB to the handling editor.
The author YZ declared that they were an editorial board member of Frontiers, at the time of submission. This had no impact on the peer review process and the final decision.
Publisher’s note
All claims expressed in this article are solely those of the authors and do not necessarily represent those of their affiliated organizations, or those of the publisher, the editors and the reviewers. Any product that may be evaluated in this article, or claim that may be made by its manufacturer, is not guaranteed or endorsed by the publisher.
References
1. Bauer P, Stevens B, Hazeleger W. A Digital Twin of Earth for the green transition. Nat Clim Change (2021) 11(2):80–3. doi: 10.1038/s41558-021-00986-y
2. Brocca L, Barbetta S, Camici S, Ciabatta L, Dari J, Filippucci P, et al. A Digital Twin of the terrestrial water cycle: a glimpse into the future through high-resolution Earth observations. Front Sci (2024) 1:1190191. doi: 10.3389/fsci.2023.1190191
3. Bauer P, Dueben PD, Hoefler T, Quintino T, Schulthess TC, Wedi NP. The digital revolution of Earth-system science. Nat Comput Sci (2021) 1(2):104–13. doi: 10.1038/s43588-021-00023-0
4. Palmer T, Stevens B. The scientific challenge of understanding and estimating climate change. Proc Natl Acad Sci (2019) 116(49):24390–5. doi: 10.1073/pnas.1906691116
5. Wedi NP, Polichtchouk I, Dueben P, Anantharaj VG, Bauer P, Boussetta S, et al. A Baseline for Global Weather and Climate Simulations at 1 km Resolution. J Adv Model Earth Syst (2020) 12(11). doi: 10.1029/2020MS002192
6. Fisher RA, Koven CD. Perspectives on the future of land surface models and the challenges of representing complex terrestrial systems. J Adv Model Earth Syst (2020) 12(4). doi: 10.1029/2018ms001453
7. Yu L, Fatichi S, Zeng Y, Su Z. The role of vadose zone physics in the ecohydrological response of a Tibetan meadow to freeze–thaw cycles. Cryosphere (2020) 14(12):4653–73. doi: 10.5194/tc-14-4653-2020
8. Blyth EM, Arora VK, Clark DB, Dadson SJ, De Kauwe MG, Lawrence DM, et al. Advances in land surface modelling. Curr Clim Change Rep (2021) 7(2):45–71. doi: 10.1007/s40641-021-00171-5
9. Wang Y, Zeng Y, Yu L, Yang P, van der Tol C, Yu Q, et al. Integrated modeling of canopy photosynthesis, fluorescence, and the transfer of energy, mass, and momentum in the soil–plant–atmosphere continuum (STEMMUS–SCOPE v1.0.0). Geosci Model Dev (2021) 14(3):1379–407. doi: 10.5194/gmd-14-1379-2021
10. Reichstein M, Camps-Valls G, Stevens B, Jung M, Denzler J, Carvalhais N, et al. Deep learning and process understanding for data-driven Earth system science. Nature (2019) 566(7743):195–204. doi: 10.1038/s41586-019-0912-1
11. Vilà-Guerau de Arellano J, Ney P, Hartogensis O, de Boer H, van Diepen K, Emin D, et al. CloudRoots: integration of advanced instrumental techniques and process modelling of sub-hourly and sub-kilometre land–atmosphere interactions. Biogeosciences (2020) 17(17):4375–404. doi: 10.5194/bg-17-4375-2020
12. Teuling AJ, Taylor CM, Meirink JF, Melsen LA, Miralles DG, van Heerwaarden CC, et al. Observational evidence for cloud cover enhancement over western European forests. Nat Commun (2017) 8(1):1–7. doi: 10.1038/ncomms14065
13. Vilà-Guerau de Arellano J, Hartogensis O, Benedict I, de Boer H, Bosman PJM, Botía S, et al. Advancing understanding of land–atmosphere interactions by breaking discipline and scale barriers. Ann N Y Acad Sci (2023) 1522(1):74–97. doi: 10.1111/nyas.14956
14. Konings AG, Yu Y, Xu L, Yang Y, Schimel DS, Saatchi SS. Active microwave observations of diurnal and seasonal variations of canopy water content across the humid African tropical forests. Geophys Res Lett (2017) 44(5):2290–9. doi: 10.1002/2016gl072388
15. Li X, Wigneron J-P, Frappart F, Lannoy GD, Fan L, Zhao T, et al. The first global soil moisture and vegetation optical depth product retrieved from fused SMOS and SMAP L-band observations. Remote Sens Environ (2022) 282(113272):113272. doi: 10.1016/j.rse.2022.113272
16. Konings AG, Saatchi SS, Frankenberg C, Keller M, Leshyk V, Anderegg WRL, et al. Detecting forest response to droughts with global observations of vegetation water content. Glob Chang Biol (2021) 27(23):6005–24. doi: 10.1111/gcb.15872
17. Zhao H, Zeng Y, Hofste JG, Duan T, Wen J, Su Z, et al. Modelling of multi-frequency microwave backscatter and emission of land surface by a community land active passive microwave radiative transfer modelling platform (CLAP). Hydrol Earth Syst Sci [preprint] (2022). Available at: doi: 10.5194/hess-2022-333 (Accessed January 25th, 2024).
18. Drusch M, Moreno J, Del Bello U, Franco R, Goulas Y, Huth A, et al. The FLuorescence EXplorer mission concept—ESA’s earth explorer 8. IEEE Trans Geosci Remote Sens (2017) 55(3):1273–84. doi: 10.1109/TGRS.2016.2621820
19. Su Z, Fernández-Prieto D, Timmermans J, Chen X, Hungershoefer K, Roebeling R, et al. First results of the earth observation Water Cycle Multi-mission Observation Strategy (WACMOS). Int J Appl Earth Obs Geoinf (2014) 26:270–85. doi: 10.1016/j.jag.2013.08.002
20. Cimini D, Serio C, Masiello G, Mastro P, Ricciardelli E, Di Paola F, et al. Spectrum synergy for investigating cloud microphysics. Bull Am Meteorol Soc (2023) 104(3):E606–22. doi: 10.1175/bams-d-22-0008.1
21. Pedruzo-Bagazgoitia X, Patton EG, Moene AF, Ouwersloot HG, Gerken T, MaChado LAT, et al. Investigating the diurnal radiative, turbulent, and biophysical processes in the Amazonian canopy-atmosphere interface by combining LES simulations and observations. J Adv Model Earth Syst (2023) 15(2). doi: 10.1029/2022ms003210
Keywords: Digital twin Earth, soil-plant-atmosphere continuum, soil-plant hydraulics, leaf water potential, cellular scale, turbulence length scale
Citation: Zeng Y and Su Z. Digital twin approach for the soil-plant-atmosphere continuum: think big, model small. Front Sci (2024) 2:1376950. doi: 10.3389/fsci.2024.1376950
Received: 26 January 2024; Accepted: 26 February 2024;
Published: 05 March 2024.
Edited and Reviewed by:
Justin Sheffield, University of Southampton, United KingdomCopyright © 2024 Zeng and Su. This is an open-access article distributed under the terms of the Creative Commons Attribution License (CC BY). The use, distribution or reproduction in other forums is permitted, provided the original author(s) and the copyright owner(s) are credited and that the original publication in this journal is cited, in accordance with accepted academic practice. No use, distribution or reproduction is permitted which does not comply with these terms.
*Correspondence: Yijian Zeng, y.zeng@utwente.nl; Zhongbo Su, z.su@utwente.nl