- Department of Civil and Environmental Engineering, University of Illinois Urbana-Champaign, Champaign, IL, United States
An Editorial on the Frontiers in Science Lead Article
A Digital Twin of the terrestrial water cycle: a glimpse into the future through high-resolution Earth observations
Key points
- The National Aeronautics and Space Administration (NASA)’s classical Earth Information System (EIS) integrates models and remote-sensing data using assimilation techniques to produce geospatial information according to physical laws and meeting physical constraints.
- Digital Twins of Earth (DTE) are the “next-generation EIS”, leveraging a step-change in spatial resolution in Earth observations of a subset of key geophysical variables. These partial models provide a virtual representation of Earth processes and subcomponents of the Earth system.
- Understanding and quantifying uncertainty in DTE models and analyses is a scientific imperative—leading governmental agencies, together with international research programs, need to collaboratively define modern standards and new rules for scientific data exchange that facilitate rigorous and robust research and applications.
Introduction
The future of humans is fundamentally tied to preserving the habitability of planet Earth through safeguarding existing habitats and the sustainable use of natural resources. As the possibility of satellite-based remote sensing of Earth reached maturity in the post-Apollo era, the need to conduct systematic global measurements from space to support Earth System Science was outlined in the Bretherton report of 1986 (1). The report called for the development of an advanced information system—the Earth Observing System Data and Information System (EOSDIS). This would process and distribute data to describe and elucidate changes in Earth system processes through long-term global observations and subsequently allow scientists to develop new models to simulate and predict future Earth system changes. Originally aiming to support models describing Earth system dynamics and how Earth observations are tied to processes, regimes, and feedbacks in the Earth system, the EOSDIS has evolved into today’s Earth Information System (EIS).
The Earth system is composed of highly complex subsystems (e.g., the atmosphere, hydrosphere, cryosphere, geosphere, and biosphere). The subsystems interact with each other via coupling mechanisms at time and spatial scales, spanning 14–16 orders of magnitude from the nanoscale of molecules to the wavelengths of Rossby waves. These are highly complex nonlinear processes, and our understanding of the underlying physics is incomplete. Between the 1990s and 2000s, data-assimilation frameworks integrating ocean, weather, and hydrologic models with observations were developed and implemented with great success, improving weather prediction in particular. Consequently, three decades after the Bretherton report, the 2017 Earth Sciences and Applications from Space Decadal Survey (2) explicitly recommended the integration of models and all Earth observations to give a holistic description of the Earth system.
The EIS integrates observations and models to produce geospatial information that follows physical laws and constraints. It monitors and interrogates our understanding of our home planet, detects and diagnoses change to drive prediction, and explores decision-making strategies. Its predictions provide a foundation for actionable Earth system science (or “Earth science applications”).
Toward a Digital Twin Earth
The Digital Twin Earth (DTE) nomenclature was adopted from systems engineering to capture a step-change in spatial resolution of Earth observations enabled in the last decade by synthetic aperture radar, hyperspectral sensors, and high-performance computing. The term deliberately reflects the new era of big data in satellite-based remote sensing with the proliferation of CubeSats, small satellites, and constantly improving measurement technologies. Formally, there is no difference between the EIS and the DTE concept. The water cycle Digital Twin described in the lead article by Brocca et al. (3) is a partial DTE designed to represent terrestrial hydrologic processes and integrate observations of water fluxes and states (though not interactions between the water and biogeochemical cycles or feedbacks between the land and the atmosphere).
The Digital Twin ontology first emerged in engineering fields as a virtual modeling tool for industrial product design and life-cycle management of physical complex systems monitored by embedded networks of sensors (cyber systems). The purpose of the industrial Digital Twin is to anticipate and eliminate undesirable outcomes by iterative design before construction and to devise solutions for mitigating undesirable, unpredictable emergent outcomes in the final product.
Observing systems, such as the National Aeronautics and Space Administration (NASA) Earth Observatory, monitor the emergent behavior of target Earth system processes and components. Data-assimilation techniques integrate observations and models to produce Earth information on recent and past states. Thus, the EIS enables scientific exploration, helping us to elucidate emergent processes, identify processes not represented in the models, and/or improve process parameterizations. It provides updated realistic initial and boundary conditions for Earth system prediction and applications.
The DTE is not to the Earth system as the Digital Twin construct used in engineering design is to the industrial product. The Earth system is a complex system of systems, and despite major advances in the last 50 years, there remain important limitations to our scientific understanding and our ability to measure and model processes. One can argue that any DTE is a multiscale, multi-physics waveguide of sorts, not unlike a low-pass filter that captures resolved and measured processes and system behavior at scales larger than the effective resolution of the model-data construct. It is possible to envision a hierarchy of Digital Twins as interconnected building blocks to capture networks of processes (i.e., regimes) within Earth subsystems (land, oceans, etc.) of increasing complexity—eventually scaling up to include interactions and feedback between Earth subsystems toward a holistic DTE (Figure 1).
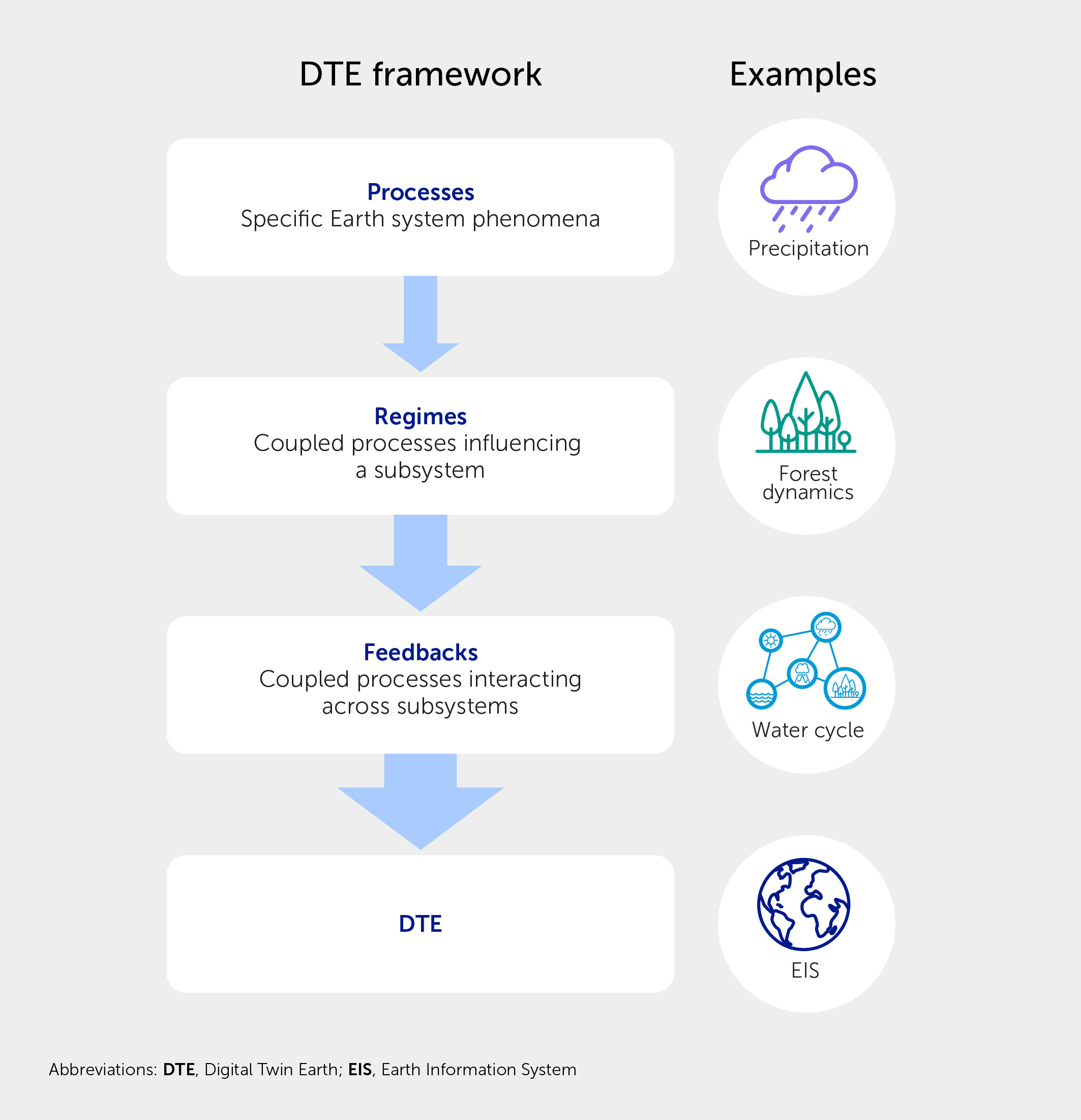
Figure 1 Hierarchical ontology of Digital Twins in Earth system science built upon a framework of increasing complexity from local to the global scales enabled by Earth observations. The Earth Information System (EIS) is a reduced Digital Twin of Earth in the sense that our understanding expressed by the model is not complete and Earth observations are limited to existing measurement technology and funding.
Uncertainties in Digital Twins of Earth
The Bretherton report proposed time as the coupling link to investigate interactions among components of the Earth system. As measurement capabilities advanced, the focus shifted to the joint space-time manifestation and evolution of Earth processes. In the Digital Twin framework, Earth observations are a snapshot of coupled processes. For instance, the local surface temperature is not only linked to net radiation but also to cloud formation, soil moisture, evaporation, and transpiration, which collectively constitute the water cycle across scales. Understanding these interlinked processes and how they change requires disentanglement of feedbacks across scales and subsystems.
Limitations exist even in the high-performance computing that supports high-resolution modeling, which is a topic that is explored by Brocca et al. (3). The measurements are averaged to coarser resolutions to meet measurement sensitivity requirements and to quantify change, introducing errors from sensors, data estimation algorithms, and uncertainty in the interpretation of the observations. Extensive studies over the last 30 years have documented the highly nonlinear and nonstationary nature of observational uncertainty in remote sensing of the water cycle, and precipitation in particular.
On the modeling side, physical uncertainty (PU) stems from missing processes, unresolved spatial and temporal scales, and the need to specify representative parameters that cannot be measured directly and cannot be quantified theoretically, owing to the lack of constitutive or functional models to capture their range of variability across scales and heterogeneous landscapes. Additional uncertainty emerges from the calibration of model parameters. For instance, in Brocca et al. (3), uncertainty in precipitation is transformed into uncertainty in infiltration and runoff processes, which then impacts soil moisture, evapotranspiration, streamflow, and land-atmosphere interactions. Computational uncertainty (CU) is another, often-neglected, type of model uncertainty. CU relates to the biases, artifacts in physical behavior, and instabilities introduced by the model’s numerical formulation and computational implementation, which can vary from subsystem to subsystem, including computational hardware and architecture. In hydrologic models, the total structural uncertainty (CU+PU) varies in space and time on account of the spatial and temporal variability of processes on complex landscapes.
Managing and minimizing uncertainty
In the water-cycle DTE, uncertainties include those in forcing elements (precipitation, winds, etc.) and in parameters that govern surface energy budget (e.g., albedo and surface roughness) and soil moisture dynamics (e.g., soil hydraulic properties). These create considerable challenges to model interrogation and predictive capability. Calibration of model parameters mixes the forcing and parameter uncertainties, thus introducing ambiguity. Because calibration involves some form of global error minimization, the calibrated parameters are tied to the uncertain forcing regime. This handicaps the ability to monitor change, and to make predictions also because of the heavy-tail statistical nature of water cycle processes, including extreme events.
Remote-sensing technologies provide a powerful tool for measuring various properties on the Earth’s surface for use in hydrological models (e.g., surface albedo or normalized difference vegetation index for characterizing vegetation). Typically, some of these parameters are either calibrated or fixed based on values extracted from the literature, or from datasets produced independently of each other. However, an alternative approach is available to DTEs, which is to leverage—to the largest extent possible—existing Earth observations to derive consistent multidimensional parameter datasets from direct measurements (reflectances, brightness, temperature, and backscatter) and thereby eliminate or dramatically reduce calibration. While uncertainty is still present, it is independent from the uncertainty in other model parameters, thus reducing the impact of model calibration. Tao and Barros (4) showed that the impact of deriving the diurnal cycle of albedo directly from reflectance measurements on the surface energy budget in complex terrain could be of an order of tens to 100 W/m2, i.e., a value similar in magnitude to cloud radiation feedbacks in the climate system.
Brocca et al. (3) refer to previous regional studies examining the sensitivity of the regional water cycle to different satellite precipitation products using a hydrologic model with parameters calibrated using a reference precipitation product. As reference products rely on ground-based observations, they are presumed to have lower uncertainty than satellite products. Nevertheless, ground-based reference products can also be riddled with systematic errors in observing geometries, sampling limitations, operational biases, and estimation algorithms, such as in the case of precipitation radar networks. Depending on the model structure and the objective function, the uncertainty in calibrated parameters captures the forcing uncertainty as processed by the model. The optimal set of parameters for one precipitation product may not be the same for another. Consequently, the model simulations will result in predictions with different uncertainty characteristics depending on the specific propagation pathway. This multiplicity begs key questions of potential Digital Twin families: how do we distinguish among them? How do we assess them? The Digital Twin hierarchy articulated earlier (Figure 1) provides a stepwise strategy to handle complexity and understand and characterize uncertainty.
Not missing the trees for the forest—trustworthy information
The grand challenge of understanding, quantifying, representing, and propagating uncertainty is a sobering one as models become more complex (e.g., introducing dynamic root systems and plant phenology in water cycle models), the volume of observations increases, and models operate at increasingly higher resolution. The Earth sciences must exercise discerning scrutiny with a focus on reducing ambiguity and understanding uncertainty—much like focusing a system of lenses to obtain crisper images across scales.
From the early stages, NASA’s policy on Earth observations was to make them available to all, including detailed documentation of mission design and operations, retrieval algorithms including validation and error characterization, and extensive metadata. As algorithms improved, revised products were made available to the scientific community, while keeping the full history of measurements, algorithms, and products. This enabled steady scientific progress by establishing a research commons for exchanging data and advancing science via shared knowledge.
As the number of players in Earth observations expands—including the private sector, independent scientists, academics, and government agencies from multiple countries and with many missions (from CubeSats to geostationary platforms and with much promise for high spatial and temporal resolution from advanced sensors)—the volume of Earth data available outside of NASA’s EIS framework is growing exponentially. The DTE is the next-generation EIS. Ultimately, the quality and trustworthiness of the information it produces depends on how the many different observations at different spatial and temporal resolutions are integrated and which models are used. Crucially, the exhilaration created by the potential of this new era of Earth observations must not trump the need for rigorous characterization of the data and their uncertainty. The leading governmental agencies [NASA, the European Space Agency (ESA), the Japan Aerospace Exploration Agency (JAXA), and others], possibly with the help and leadership of international research programs, need to collaboratively define modern standards and new rules for scientific data exchange that facilitate rigorous and robust research and applications.
Statements
Author contributions
APB: Visualization, Writing – original draft, Writing – review & editing.
Funding
The author declares that no financial support was received for the research, authorship, and/or publication of this article.
Conflict of interest
The author declares that the research was conducted in the absence of any commercial or financial relationships that could be construed as a potential conflict of interest.
Publisher’s note
All claims expressed in this article are solely those of the authors and do not necessarily represent those of their affiliated organizations, or those of the publisher, the editors and the reviewers. Any product that may be evaluated in this article, or claim that may be made by its manufacturer, is not guaranteed or endorsed by the publisher.
References
1. National Research Council. Earth System Science: Overview: A Program for Global Change. Washington, DC: The National Academies Press (1986). doi: 10.17226/19210
2. National Academies of Sciences, Engineering, and Medicine. Thriving on Our Changing Planet: A Decadal Strategy for Earth Observation from Space. Washington, DC: The National Academies Press (2018). doi: 10.17226/24938
3. Brocca L, Barbetta S, Camici S, Ciabatta L, Dari J, Filippucci P, et al. A Digital Twin of the terrestrial water cycle: a glimpse into the future through high-resolution Earth observations. Front Sci (2024) 1:1190191. doi: 10.3389/fsci.2023.1190191
4. Tao J, Barros AP. Multi-year surface radiative properties and vegetation parameters for hydrologic modeling in regions of complex terrain—Methodology and evaluation over the Integrated Precipitation and Hydrology Experiment 2014 domain. J Hydrol Reg Stud (2019) 22:100596. doi: 10.1016/j.ejrh.2019.100596
Keywords: Earth Information System, Digital Twins of Earth, Earth observations, Earth system models, uncertainty
Citation: Barros AP. Digital Twin Earth: the next-generation Earth Information System. Front Sci (2024) 2:1383659. doi: 10.3389/fsci.2024.1383659
Received: 07 February 2024; Accepted: 15 February 2024;
Published: 05 March 2024.
Approved by:
Frontiers in Science Editorial Office, Frontiers Media SA, SwitzerlandCopyright © 2024 Barros. This is an open-access article distributed under the terms of the Creative Commons Attribution License (CC BY). The use, distribution or reproduction in other forums is permitted, provided the original author(s) and the copyright owner(s) are credited and that the original publication in this journal is cited, in accordance with accepted academic practice. No use, distribution or reproduction is permitted which does not comply with these terms.
*Correspondence: Ana P. Barros, barros@illinois.edu