- 1 Department of Neurobiology, Yale University School of Medicine, USA
- 2 Institute of Biophysics, National Research Council, Italy
Sensory discrimination requires distributed arrays of processing units. In the olfactory bulb, the processing units for odor discrimination are believed to involve dendrodendritic synaptic interactions between mitral and granule cells. There is increasing anatomical evidence that these cells are organized in columns, and that the columns processing a given odor are arranged in widely distributed arrays. Experimental evidence is lacking on the underlying learning mechanisms for how these columns and arrays are formed. To gain insight into these mechanisms, we have used a simplified realistic circuit model to test the hypothesis that distributed connectivity can self-organize through an activity-dependent dendrodendritic synaptic mechanism. The results point to action potentials propagating in the mitral cell lateral dendrites as playing a critical role in this mechanism. The model predicts that columns emerge from the interaction between the local temporal dynamics of the action potentials and the synapses that they activate during dendritic propagation. The results suggest a novel and robust learning mechanism for the development of distributed processing units in a cortical structure.
Introduction
A recent development in studies of olfactory bulb functional organization is the recognition of the distributed nature of the circuits that process odor maps elicited in the glomerular layer (Cleland and Sethupathy, 2006; Migliore and Shepherd, 2008; Willhite et al., 2006 ). Experimental evidence, using pseudorabies virus to trace synaptic connections in the olfactory bulb, has provided direct evidence of distributed, non-topographical, connectivity between mitral cells belonging to different olfactory glomeruli (Willhite et al., 2006 ). In particular, an arrangement of mitral and granule cells in columns indicated that such distributed “glomerular units” are likely involved in processing similar odors.
It is evident that the functional wiring of such selected cell ensembles requires an appropriate learning rule. Due to the difficulty of experimentally studying in vivo such distributed systems, the underlying mechanisms that could be responsible for this kind of connectivity are currently unknown. Here we focus on the mitral–granule interactions which take place through dendrodendritic reciprocal synapses (Rall and Shepherd, 1968 ). While the properties of these synapses have been extensively studied (Balu et al., 2007; Chen et al., 2000; Egger et al., 2005; Isaacson and Vitten, 2003; Lowe, 2002; Pinato and Midtgaard, 2005; Schoppa et al., 1998; Shoppa and Westbrook, 1999 ), to our knowledge there is no experimental evidence of long term plasticity changes (for short term effects, see Dietz and Murthy, 2005 ). However, these changes must occur in order to account for the distributed connectivity between mitral and granule cells that is observed in the tracing (Willhite et al., 2006 ) and recording (Urban and Sakmann, 2002 ) experiments. We hypothesize that during development odor exposure is sufficient to potentiate or depress these synapses in such a way as to form the appropriate connectivity between separated glomerular units that is observed.
To explore these mechanisms we use a model of interconnected mitral and granule cells (Migliore and Shepherd, 2008 ) into which we introduce a synaptic learning mechanism. The results suggest that different temporal patterns of action potential propagation through the mitral cell lateral dendrites (Egger and Urban, 2006; Migliore and Shepherd, 2008 ) lead to selective potentiation or depression of mitral–granule synapses belonging to different glomerular units. We demonstrate how odor-induced glomerular activity patterns could direct these plastic changes in the dendrodendritic synapses in such a way as to potentiate those belonging to active glomerular units while depressing others, thus establishing the observed connectivity.
Materials and Methods
All the simulations were carried out with the NEURON simulation program (Hines and Carnevale, 1997 ) using its variable time step feature. In most cases, simulations were carried out on a 1024-processor IBM Linux cluster. The model and simulation files will be available for public download under the ModelDB section of the Senselab database (http://senselab.med.yale.edu/ ).
We used a model of one or two mitral cells (MT) and 50 granule cells (GCx) forming dendrodendritic contacts along a lateral dendrite at x μm from the soma, as schematically represented in Figure 1 a. Mitral and granule cell active and passive membrane properties, as well as synaptic properties, were those used in a previous paper (Migliore and Shepherd, 2008 ), and validated against a number of experimental findings. Briefly, mitral cells included an axon, soma, a primary dendrite, a tuft, and two 500 μm long lateral dendrites. Granule cells were modeled with a soma, a main radial dendrite (150 μm long) connected to 3, 100 μm long, second-order dendrites representing the medial and distal dendritic tree, one of which was used to make dendrodendritic synaptic contact with mitral cell(s) lateral dendrite(s).
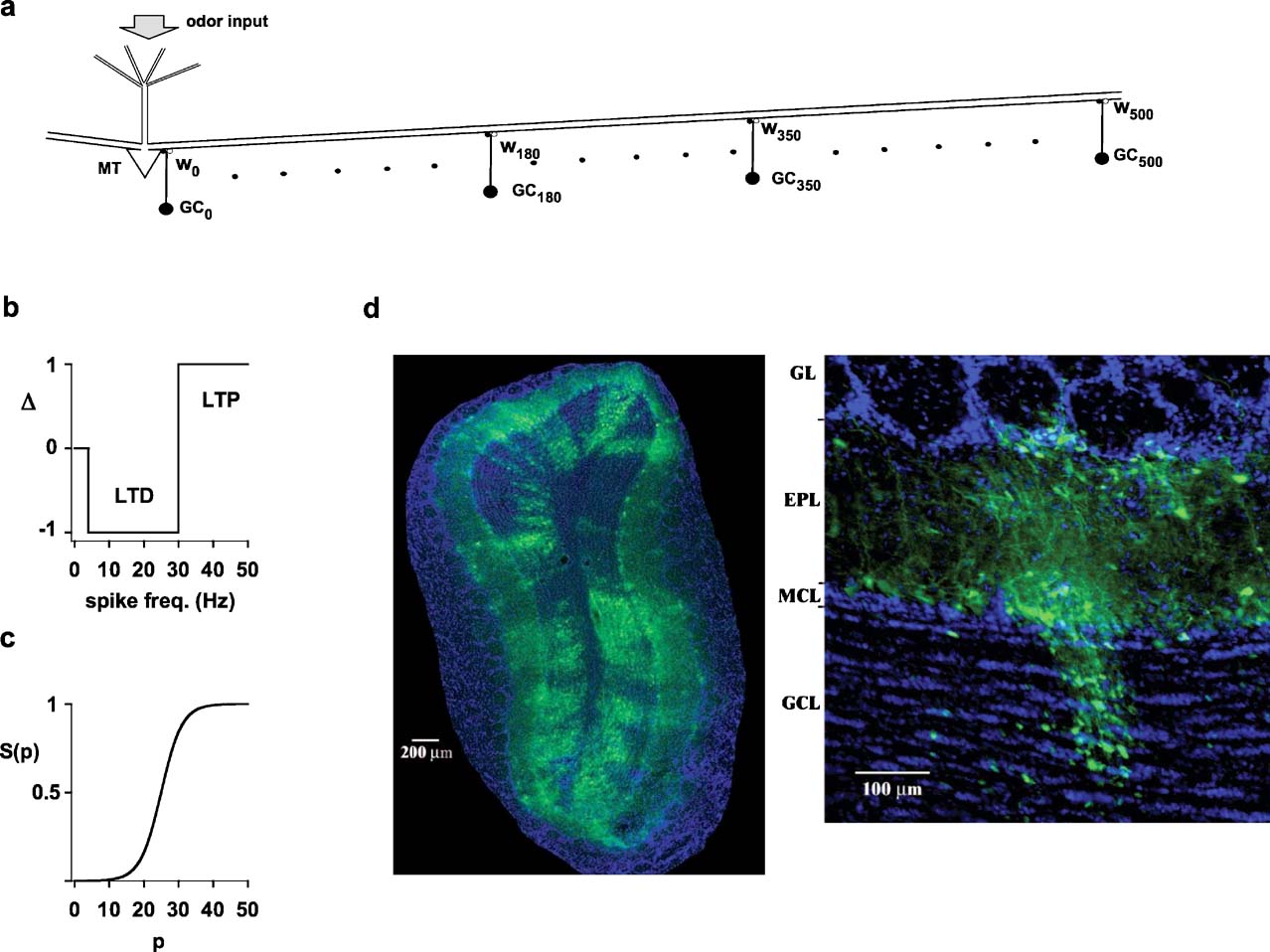
Figure 1. Experimental findings and model schematic.(a) Schematic representation of the network used for most simulations; MT indicates a mitral cell, and GCx(x = 0, 10, 20…500) indicates a granule cell at x m from the mitral cell soma; wx represents two (excitatory and inhibitory) synapses of the dendrodendritic synaptic contacts between a GCxand the MT lateral dendrite. (b) Schematic representation of the plasticity rule applied to modify the state of each component of each synaptic weight according to the local instantaneous spike frequency; LTP, long-term potentiation; LTD, long-term depression. (c) After each local spike the state, p, of each synapse was updated as wx,p = gsyn·S(p+Δ), where gsyn was the peak synaptic conductance, and Δ = {−1,0,+1} was determined as in b. (d) Photomicrographs of a rat olfactory bulb showing (left) a coronal section of the olfactory bulb demonstrating distributed connectivity and (right) a single glomerular column, using pseudorabies virus tracing (from ref. 1). Note columnar arrangement of labeled granule cells, mitral cells in the MCL, and glomerular layer cells. GL, glomerular layer; EPL, external plexiform layer; MCL, mitral cell body layer; GCL, granule cell layer.
Odor inputs were modeled using a synchronous activation, in all compartments of the mitral cell dendritic tuft, of synaptic inputs modeled with a double exponential conductance change (20 and 200 ms rise and decay time, respectively), with an individual peak conductance in the range of 0–0.5 nS, corresponding to a total aggregate maximum input conductance of 10 nS. In this way an isolated activation at the maximum strength resulted in six somatic spikes, within the range observed experimentally (Cang and Isaacson, 2003 ).
Dendrodendritic coupling between a granule cell and mitral cell lateral dendrites was implemented by connecting a GC dendrite containing AMPA and NMDA channels with the MT lateral dendrite, containing GABA channels. Synapses (excitatory or inhibitory) were activated whenever the corresponding presynaptic compartment reached the threshold of −40 mV. In agreement with experimental findings (Isaacson, 2001 ), this choice results in recurrent inhibition of the lateral dendrite of a mitral cell that did not necessarily require the generation/propagation of an action potential to the soma of the GC.
Synaptic plasticity
Each component (inhibitory or excitatory) of each dendrodendritic synapse was independently modified according to a plasticity rule using the local membrane potential, of the lateral dendrite of the mitral cell or the granule cell synapse, to calculate the instantaneous presynaptic interspike frequency (ISI). After each spike, the state, p, of a given mitral-to-granule or granule-to-mitral peak synaptic conductance at x μm from the soma, wx,{exc,inh},p, was updated to a new value. The functional form used in all simulations was wx,{exc,inh},p = gsyn,{exc,inh}·S(p+Δ), where Δ {0,+1,−1} followed a classical scheme (Bienenstock et al., 1982; Stanton, 1996 ) for long-term potentiation (LTP) or long-term depression (LTD), and S(p) was a typical sigmoidal activation function (Haykin, 1994 ). As schematically shown in Figure 1 b, the thresholds for LTD and LTP were set at 4 and 30 Hz, respectively. For the activation function (Figure 1 c), we used the functional form S(p) = 1/(1+exp((p−25)/3)), in such a way that the peak synaptic conductance of a given synapse could span the entire range, from close to 0 (for p = 0) to gsyn,{exc,inh} (for p = 50), in about 50 consecutive ISIs of the appropriate frequency. In an extensive series of preliminary simulations, we tested that the main results of the paper did not depend on the specific choice for the functional form used to update the synaptic weights.
Results
The anatomical evidence for the distributed connectivity analyzed by this study is shown in Figure 1 d. On the left, a single injection into the olfactory bulb or olfactory cortex resulted in distinct labeling of clusters of cells distributed throughout the olfactory bulb. Higher resolution (right) showed that the narrowest clusters were arranged in cell columns, consisting most prominently of granule and mitral cells aligned in relation to single glomeruli.
We address the possible learning mechanism involved in establishing this kind of connectivity using the plasticity rule described in the previous section. We focused first on a model of a single isolated column, consisting of one mitral cell (MT) and its associated 50 granule cells (GCx) (represented first by a single granule cell) forming dendrodendritic contacts along a lateral dendrite at x μm from the soma, as schematically shown in Figure 1 a.
The process of column formation
A typical simulation, illustrating how learning takes place through an interaction between a propagating action potential in the lateral dendrite and granule cell feedback inhibition, is shown in Figure 2 . We focus on the two recording locations indicated by the symbols in Figure 2 a, the mitral cell soma and the lateral dendrite at 350 μm from the soma, during the representative odor input activated at constant strength and constant frequency. All the synapses were initialized to a weight wx,0 at t = 0. During the first second of the simulation, the train of somatic action potentials (Figure 2 b, top trace) begins to potentiate the excitatory mitral-to-granule synapses. Note that the dendrite of GC0, the granule cell closest to the soma (Figure 2 b, GC0 dend), did not begin its firing activity until the excitatory mitral-to-granule component was substantially potentiated. The time course of the excitatory and inhibitory components then followed the corresponding local spike train (somatic spikes for w0,exc, and GC0 dendritic spikes for w0,inh). In this simulation, after about 3 seconds of stimulation, both synapses reached a steady state at their maximum value, maintained by a train of odor-evoked bursts of somatic APs until the end of the stimulation (10 seconds, only the first 3 seconds are shown). Thus, at or near the soma, the train of action potentials potentiates both the excitatory and inhibitory components of the dendrodendritic synapse.
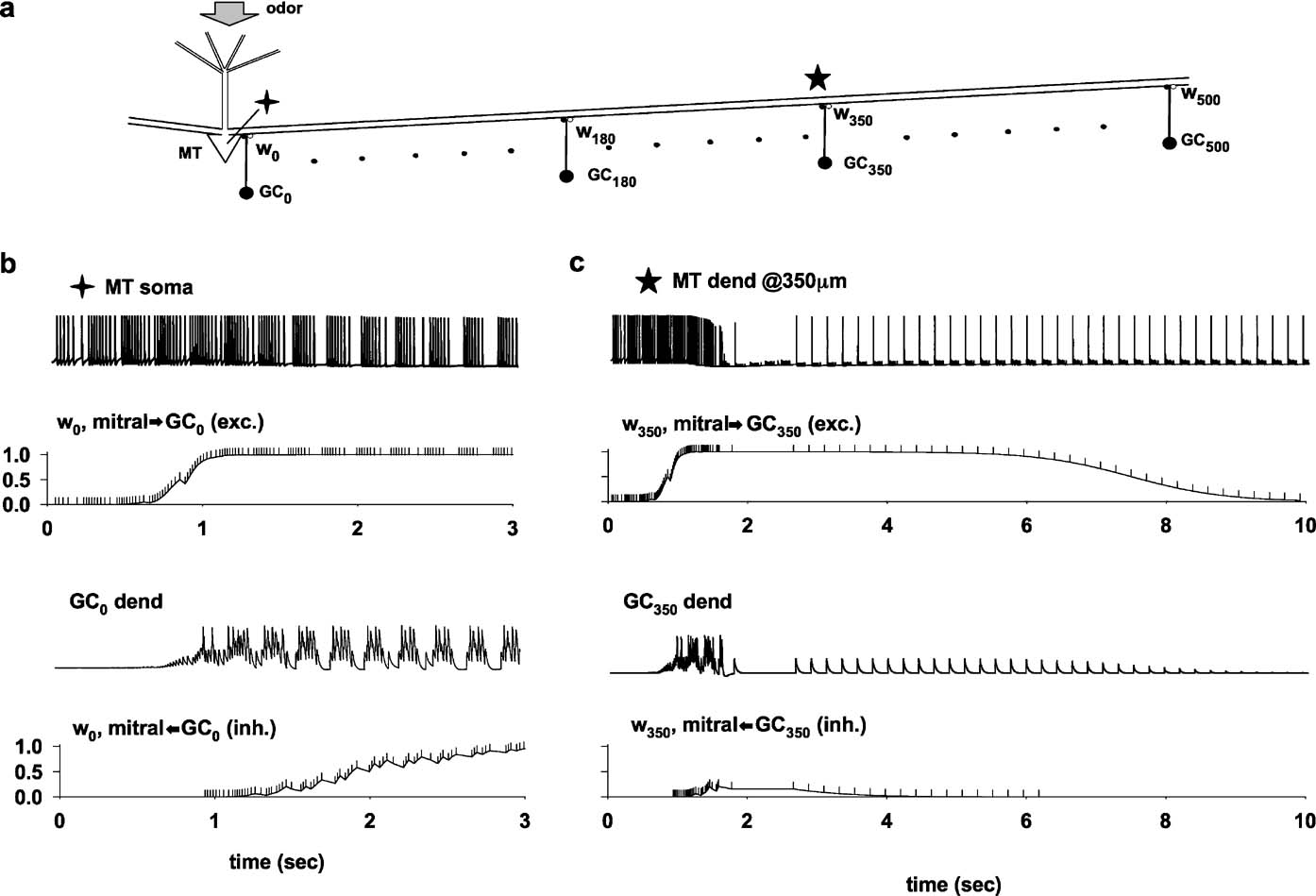
Figure 2. The process of column formation. (a) Schematic representation of the basic network architecture investigated; the symbols (cross and star) indicate the recording locations for parts b and c; a 10 nS odor input was activated in the MT tuft at a constant 4.5 Hz frequency; (b) membrane potential at the MT soma and at the synaptic location of the closest granule cell (GC0 dend); below each trace is plotted the time course of the relative component of w0 (normalized mitral-to-GC or GC-to-mitral peak synaptic conductance); The vertical markers along the w0 traces indicate the presynaptic spike times. (c) As in (b), but for a distal recording location on the lateral dendrite, at 350 m from the soma.
A very different result was obtained at 350 μm from the soma (Figure 2 c). During the first second of simulation, each action potential propagated from the soma relatively unperturbed along the lateral dendrite to its terminus (data not shown). The first second of stimulation was very similar to that observed at the soma, potentiating all the excitatory synapses along the same time course of w0,exc (Figure 2 b). During the next second of simulation, this activity in the lateral dendrite caused a strong GCx activity and the consequent potentiation of the inhibitory synapses. The building up of the inhibition on the lateral dendrites resulted in a drastic change in the time course of the lateral dendrite membrane potential, where most of the action potentials after each odor activation failed to propagate to 350 μm (Figure 2 c, MT dend). The action potentials that propagated did so at a frequency that resulted in depression of both the excitatory and inhibitory synapses (Figure 1 c, w350), until they reached their minimum value and could be considered effectively eliminated from the circuit.
The formation process is robust
Because the feedback inhibition from granule cells can modulate AP backpropagation in a mitral cell lateral dendrite (Christie and Westbrook, 2003; Lowe, 2002; Migliore and Shepherd, 2008; Xiong and Chen, 2002 ), we hypothesized that the width of a column could depend on the peak inhibitory synaptic conductance. To test this hypothesis we carried out several simulations using the same odor input as in Figure 2 , but using random values for the individual peak inhibitory conductance (Figure 3 a), drawn from a normal distribution with a standard deviation ±10% of the average. The results confirmed that higher peak inhibitory synaptic conductances form a narrower column, and suggested that the peak conductance required to form a narrow glomerular column corresponds to approximately two–five individual inhibitory granule-to-mitral synapses, assuming 0.6 nS per synapse (Schoppa et al., 1998 ).
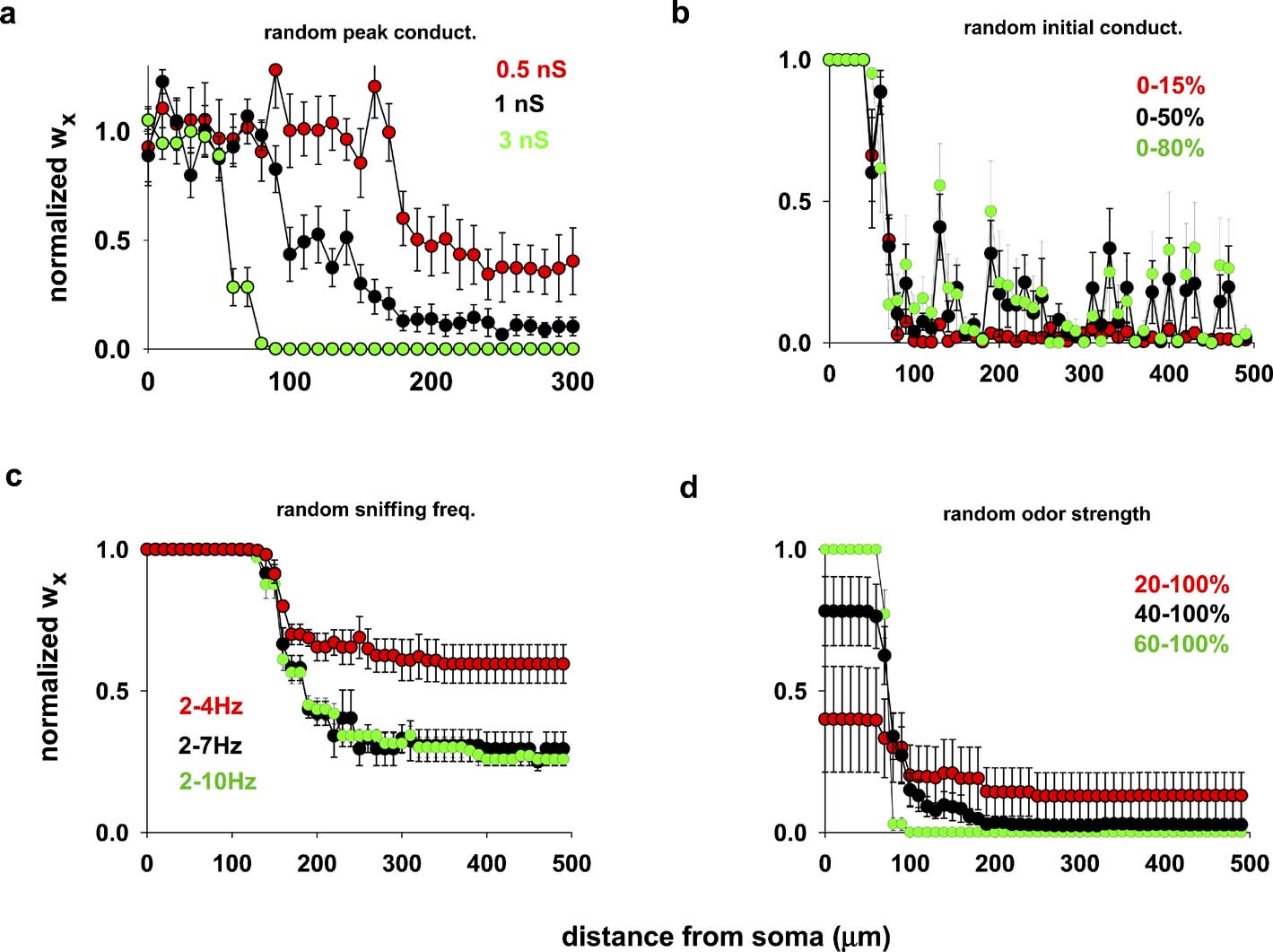
Figure 3. Column formation is robust. (a) Average final distribution of inhibitory synaptic weights, wx, using different random average values for the individual peak inhibitory synaptic conductances (drawn from a normal distribution, s.d. ± 10% of the average); in all cases, wx = 0 at the beginning of each simulation, the input (sniffing frequency) was activated at 4.5 Hz and constant strength (10 nS). (b) Average final distribution, using different random initial excitatory and inhibitory conductances (uniform distribution within the specified range of the maximum peak excitatory (2 nS) and inhibitory (3 nS) conductances); sniffing frequency was activated at 4.5 Hz and constant strength (10 nS); (c) average final distribution using different random sniffing frequency (uniform distribution within the specified range of the maximum value of 10 nS); initial conductances set to zero, and peak conductance to 2 nS (exc.) and 3 nS (inh.); (d) average final distribution, using different random odor strengths during the simulations (uniform distribution within the specified range of the peak value (10 nS); sniffing frequency was constant at 4.5 Hz, initial conductances set to zero, and peak dendrodendritic conductance to 2 nS (exc.) and 3 nS (inh.). In all cases, average values were calculated from five simulations 20 seconds in duration.
We next tested the robustness of the process of column formation in relation to several other variables likely to apply during development. Different mechanisms can be responsible for a wide variation in the pattern of action potentials generated by an odor presentation, such as different sniffing frequencies or highly variable odor strengths. In addition, newly formed synapses may not have a negligible peak conductance. We carried out simulations to explore these variables. For this purpose, we calculated the average final distribution of inhibitory synapses wx using a 20 second odor exposure to achieve a stable response condition. This was repeated several times to simulate the hypothesized process of plasticity underlying column formation with repeated odor exposures (quantitative reduction of response due to adaptation is neglected in these simulations).
Figure 3 b illustrates the results from five repetitions, using different random initial synaptic conductance values with the other parameters held constant. Figure 3 c shows the results for different ranges of random sniffing frequency, within the experimentally observed range of 2–10 Hz (Kepecs et al., 2007 ), with a constant odor strength and initial conductance value. Finally, in Figure 3 d we report the results using different ranges of random odor strength during the simulation, with constant sniffing frequency and initial conductance. In all cases, the results consistently showed that column formation was a robust phenomenon, and that in all cases the formation of the column of potentiated synapses occurred close to the soma of the mitral cell.
Emergence of distributed columns
The PRV method has demonstrated not only that cells are organized into narrow columns, but that these columns are distributed widely within the olfactory bulb (see Figure 1 d (left)). To investigate the distributed glomerular activation required to produce such columns, we considered two mitral cells, connected by the same set of granule cells GCx, but now activated by two different inputs. The hypothesis, based on experimental observations (Willhite et al., 2006 ), is that if the corresponding glomerular units are far apart, two independent columns should be formed, around each mitral cell soma, whereas they should merge into a single column when odors activate neighboring glomeruli.
To test this, the original network of Figure 1 a was modified by adding a second mitral cell (MT2), connected to all GCx through its lateral dendrite, according to its position with respect to MT1 (Figure 4 a). Three relative MT distances were tested, with the soma of the two mitral cells separated by 100, 300, or 500 μm, as schematically indicated in Figure 4 a. All cases were tested using protocols as for the single column simulations illustrated in Figures 3 c and 3 d: random odor strengths for input-1 and input-2 (60–100% of the maximum) activated at random frequency (2–10 Hz). Under these conditions, the strength of the inhibitory conductances followed independent time courses, according to the local activation patterns caused by the complex interaction between the action potentials elicited in each mitral cell soma and propagating along their lateral dendrites, and the activity in each granule cells.
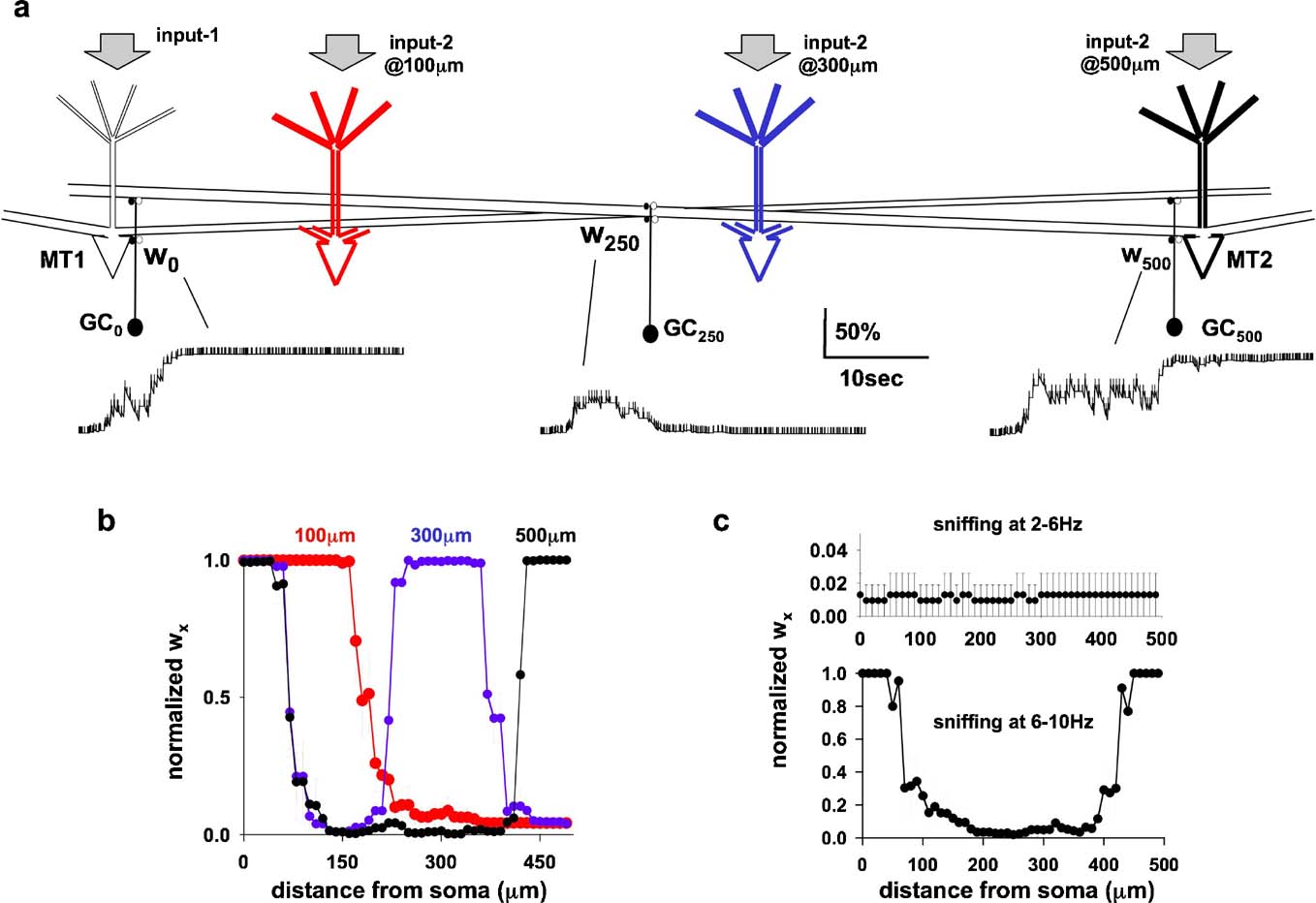
Figure 4. (a) Schematic representation of the network configuration using two mitral cells (MT1–2) with independent inputs (input-1 and input-2), randomly activated at 2–10 Hz; configurations using different reciprocal distances are represented by different colors for MT2 (black 500 m, blue 300 m, and red 100 m). (b) Distribution of inhibitory wx (average from five simulations of 30 seconds) using variable inputs on both MT (6–10 nS strength, 2–10 Hz sniffing), and MT at different reciprocal distances. (c) Average distribution (five simulations of 30 seconds) of the inhibitory wx for random sniffing frequency in the range of 2–6 Hz (top), or 6–10 Hz (bottom).
The time course of granule cell synaptic weight at three locations, w0,inh, w250,inh, and w500,inh is plotted in Figure 4 a. Although granule cell activity was very different at the different locations (Figure 4 a, vertical markers along each trace), the final result was consistent with the hypothesis: two columns of fully potentiated inhibitory synapses were formed, close to the somata of the two mitral cells (Figure 4 b, black). When MT2 was closer, at 300 μm from MT1 (Figure 4 b, blue), the columns associated with it were also closer (Figure 4 b, blue). The two columns merged into a single one when the two mitral cells were only 100 μm apart (Figure 4 b, red). This result is similar to the broad columns seen in the PRV studies (Figure 1 d, left).
We further tested if the model could give a physiological explanation for the experimental observation that, although sniffing patterns varied widely in the range of 2–12 Hz, odor discrimination was dependent on 6–9 Hz sniffing (Kepecs et al., 2007 ). Simulations were carried out using two mitral cells. They were activated by randomly varying odor strengths, with activation (sniffing) frequencies varying randomly within two behaviorally relevant ranges 2–6 Hz or 6–10 Hz as suggested by experiments (Kepecs et al., 2007 ). The results (Figure 4 c) showed that sniffing in the low frequency range was not able to induce plasticity of the dendrodendritic synapses, in contrast with the results for 6–10 Hz, where columns were formed.
Time course of column formation
Finally, the time evolution of the single and multiple columns is illustrated in Figure 5 . The strength wx of the inhibitory granule-to-mitral synapses is shown as a three-dimensional extension of Figure 2 in Figure 5 (left), and illustrates the fundamental steps for a column formation. The full propagation, along the lateral dendrite, of all the action potentials elicited by the odor input quickly potentiated all the mitral-to-granule excitatory synapses. Every action potential was now strong enough to depolarize substantially, during its propagation, the corresponding granule cell dendrite, GCx. This set the conditions for potentiation of the inhibitory synapse (Figure 5 , left, 0 < t < 2 seconds). However, not all the inhibitory synapses undergo the same amount of potentiation. As soon as those close to the mitral cell soma reach their maximum value (Figure 5 left, red region for 0 < x < 50 μm), the feedback inhibitory signal on the lateral dendrite hinders action potential propagation. The pattern of action potentials observed along the lateral dendrite thus depends on the specific distance from the soma (Migliore and Shepherd, 2008 ). In particular, with the building up of the inhibitory synapses close to the soma, some of the action potentials were missed during the propagation (as discussed in Figure 2 c). The direct consequence of this effect is that both the excitatory and inhibitory conductances will undergo different amounts of potentiation or depression, depending on their distance from the soma and, of course, from the specific activation pattern. For the simple stimulation protocol illustrated in Figure 2 , this resulted in a transient potentiation followed by depression of the excitatory (not shown) and inhibitory synapses beyond H100 μm from the soma (Figure 5 , traces as a function of time for x > 100 μm). The result is a column of fully potentiated synapses close to the soma of the active mitral cell, where action potentials are not easily blocked by granule cell activity. The same process operates during the formation of multiple columns, as illustrated in the right diagram of Figure 5 .
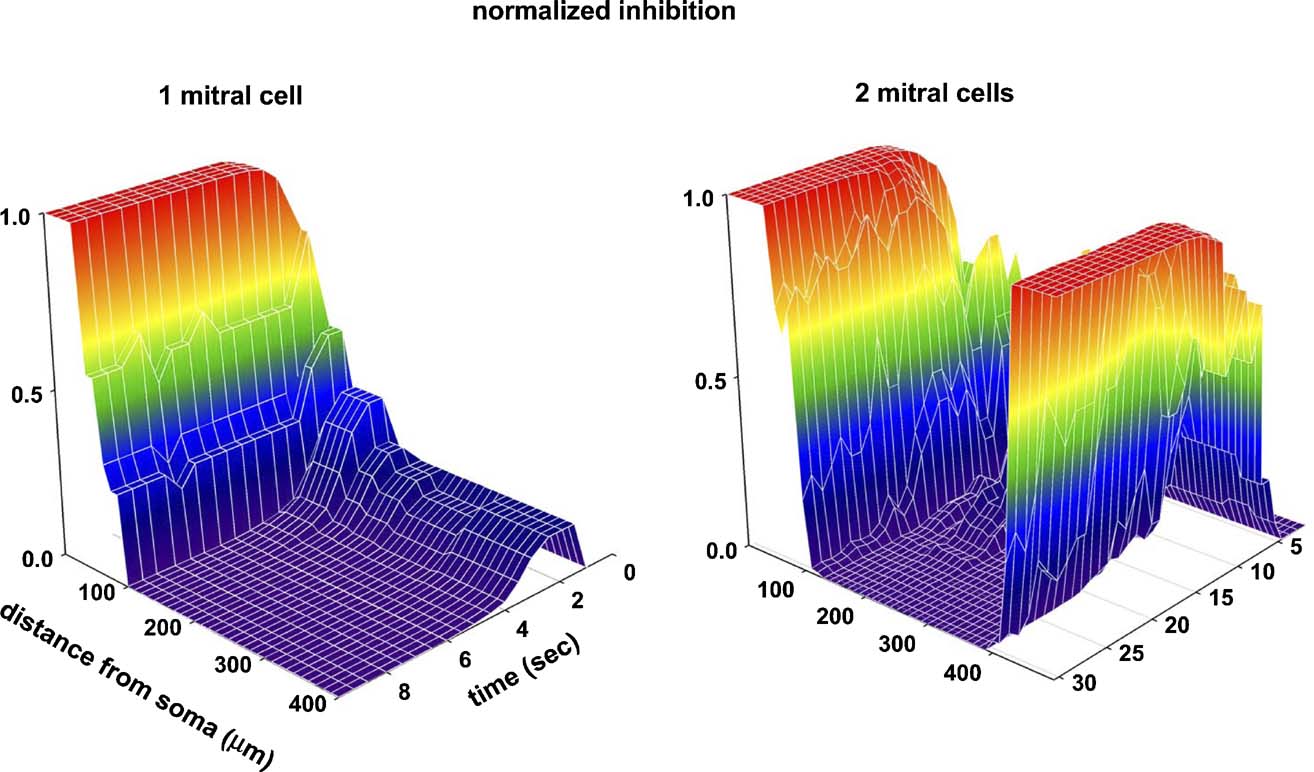
Figure 5. Time course of the GC-to-mitral synapses (normalized peak inhibitory conductance) as a function of distance from the MT soma. (Left) one active mitral cell, as in Figure 1 d; (right) two active mitral cells (model as in Figure 4 a, distance is from MT1). Values are plotted at 0.5 seconds intervals.
Discussion
The results suggest a novel learning mechanism for the development of distributed processing units in a cortical structure. The propagation of action potentials along mitral cell lateral dendrites can be seen to be an essential property underlying a distributed, non-topographical, connectivity of mitral and granule cells, leading to the formation of clusters of potentiated synapses arranged in relation to individual glomeruli. The results are in agreement with, and explain in computational terms, the experimental findings obtained with pseudorabies virus tracing of synaptic connections (Willhite et al., 2006 ).
This specific mechanism is now open to anatomical and physiological analysis. It will be important to focus on several specific properties demonstrated by the model. First are the long lateral dendrites, supporting propagating action potentials, as has been shown experimentally (Christie and Westbrook, 2003; Lowe, 2002; Xiong and Chen, 2002 ). Second is the constraint of connectivity of a mitral cell to a single glomerulus, as known anatomically (Shepherd et al., 2004 ). Third are the reciprocal dendrodendritic synapses feeding back inhibition on the cell bodies and lateral dendrites; this also has been demonstrated experimentally (Rall and Shepherd, 1968 ). We show that, with these properties, the system is able to generate selective connectivity by an activity-dependent mechanism.
The model has enabled an analysis of the steps in the emergence of the activity-dependent connectivity. At or near the mitral cell soma, natural exposure to odors during early development elicits trains of action potentials which initially potentiate both the excitatory and inhibitory components of the dendrodendritic synapse (Figure 2 ). A column results from the differential dendritic propagation of action potentials originating from the soma of the active mitral cells, as modulated by the inhibitory component of the dendrodendritic synapses (2 ). The effect is robust (Figure 3 ), and it critically depends only on the dendritic (as opposed to somatic) temporal pattern of action potentials. These properties apply to formation of both isolated or distributed multiglomerular units (Figure 4 ).
For these reasons, it is apparent that this specific mechanism has general properties, and that any general learning rule, such as involving LTP/LTD, is likely to result in a qualitatively similar effect. However, it should be stressed that our assumption that newly formed synapses have small conductances limits the induction process to non-Hebbian mechanisms, i.e., to purely presynaptic learning rules. If the initial conductances are small, the synapses will not be able to generate enough postsynaptic depolarization, and any plasticity rule based on coincident pre- and post-synaptic activity would fail from the beginning, since there will be no postsynaptic (granule cell) activity without a preceding LTP of the mitral-to-granule component. A possible Hebbian alternative is one in which the learning process occurs in the presence of independent granule cell activity, from its intrinsic (e.g., axon collaterals) or extrinsic (e.g., centrifugal fiber) inputs. In these cases, the results would be qualitatively similar, because the action potentials in the lateral dendrites would also be involved. Experiments are needed to test these specific hypotheses.
The present mechanism for the activity-dependent development of synaptic connections focuses on the initial formation of the connections. The results provide an explanation for the connectivity revealed by the PRV tracing experiments. Beyond establishing these initial connections there are two possibilities. One is that after initial formation there are additional mechanisms which come into play for maintenance and stability of these synapses (cf. Ehrlich et al., 2007 ), and that it is the patterns of these established synaptic connections that are revealed by the tracing experiments. The other possibility is that the system remains dynamic, so that with repeated odor exposures the relative strengths of the lateral inhibition between glomerular units is in flux. In this case, the tracing experiments would constitute a kind of snapshot of connectivity that was actually varying reflecting the ongoing odor exposure of the animal. The odor map in the glomerular layer would remain relatively fixed by the inputs from the subsets of receptor cell axons, but the lateral inhibitory network through the granule cells would function as a kind of working memory of the ongoing odor environment. Odor learning/reinforcement would be constantly adapting the set of active granule cells, eliminating depressed synapses with a pruning process, while growing/maintaining potentiated synapses at those dendritic locations involving the activation of specific glomeruli during presentation of new or known odors. These hypotheses are all open to experimental testing, probably most effectively with multielectrode recordings and multiple cell imaging to assess the distributed system.
The results thus suggest a novel and robust learning mechanism for the development of distributed processing units in a cortical structure. In this model both the spatial pattern of the odor map in the olfactory glomerular layer (Shepherd et al., 2004 ) and the temporal pattern of action potential generation in the mitral cells (Friedrich and Laurent, 2004 ) play a role. This approach to identifying the learning rule during development may therefore help to bring about a synthesis of the spatial and temporal properties that underlie sensory coding in this cortical structure.
Conflict of Interest Statement
The authors declare that the research was conducted in the absence of any commercial or financial relationships that could be construed as a potential conflict of interest.
Acknowledgements
We are grateful for support by National Institutes of Health Grant DC00086 and the Human Brain Project (National Institute of Deafness and Other Communication Disorders, National Institute of Mental Health, National Institute of Neurological Disorders and Stroke, National Institute on Aging, and the National Science Foundation).
References
Balu, R., Pressler, R. T., and Strowbridge, B. W. (2007). Multiple modes of synaptic excitation of olfactory bulb granule cells. J. Neurosci. 27, 5621–5632.
Bienenstock, E. L., Cooper, L. N., and Munro, P. W. (1982). Theory for the development of neuron selectivity: orientation specificity and binocular interaction in visual cortex. J. Neurosci. 2, 32–48.
Cang, I., and Isaacson, J. S. (2003). In vivo whole-cell recording of odor-evoked synaptic transmission in the rat olfactory bulb. J. Neurosci. 23, 4108–4116.
Chen, W. R., Xiong, W., and Shepherd, G. M. (2000). Analysis of relations between NMDA receptors and GABA release at olfactory bulb reciprocal synapses. Neuron 25, 625–633.
Christie, J. M., and Westbrook, G. L. (2003). Regulation of backpropagating action potentials in mitral cell lateral dendrites by A-type potassium currents. J. Neurophysiol. 89, 2466–2472.
Cleland, T. A., and Sethupathy, P. (2006). Non-topographical contrast enhancement in the olfactory bulb. BMC Neurosci. 7, 7.
Dietz, S. B., and Murthy, V. N. (2005). Contrasting short-term plasticity at two sides of the mitral-granule reciprocal synapse in the mammalian olfactory bulb. J. Physiol. 569, 475–488.
Egger, V., and Urban, N. N. (2006). Dynamic connectivity in the mitral cell-granule cell microcircuit. Semin. Cell Dev. Biol. 17, 424–432.
Egger, V., Svoboda, K., and Mainen, Z. F. (2005). Dendrodendritic synaptic signals in olfactory bulb granule cells: local spine boost and global low-threshold spike. J. Neurosci. 25, 3521–3530.
Ehrlich, I., Klein, M., Rumpel, S., and Malinow, R. (2007). PSD-95 is required for activity-driven synapse stabilization. Proc. Natl. Acad. Sci. USA 104, 4176–4181.
Friedrich, R. W., and Laurent, G. (2004). Dynamics of olfactory bulb input and output activity during odor stimulation in zebrafish. J. Neurophysiol. 91, 2658–2669.
Hines, M., and Carnevale, T. (1997). The NEURON simulation environment. Neural Comput. 9, 1179–1209.
Isaacson, J. S. (2001). Mechanisms governing dendritic gamma-aminobutyric acid (GABA) release in the rat olfactory bulb. Proc. Natl. Acad. Sci. USA 98, 337–342.
Isaacson, J. S., and Vitten, H. (2003). GABA(B) receptors inhibit dendrodendritic transmission in the rat olfactory bulb. J. Neurosci. 23, 2032–2039.
Kepecs, A., Uchida, N., and Mainen, Z. F. (2007). Rapid and precise control of sniffing during olfactory discrimination in rats. J. Neurophysiol. 98, 205–213.
Lowe, G. (2002). Inhibition of backpropagating action potentials in mitral cell secondary dendrites. J. Neurophysiol. 88, 64–85.
Migliore, M., and Shepherd, G. M. (2008). Dendritic action potentials connect distributed dendrodendritic microcircuits. J. Comput. Neurosci. in press.
Pinato, G., and Midtgaard, J. (2005). Dendritic sodium spikelets and low-threshold calcium spikes in turtle olfactory bulb granule cells. J. Neurophysiol. 93, 1285–1294.
Rall, W., and Shepherd, G. M. (1968). Theoretical reconstruction of field potentials and dendrodendritic synaptic interactions in olfactory bulb. J. Neurophysiol. 31, 884–915.
Schoppa, N. E., Kinzie, J. M., Sahara, Y., Segerson, T. P., and Westbrook, G. L. (1998). Dendrodendritic inhibition in the olfactory bulb is driven by NMDA receptors. J. Neurosci. 18, 6790–6802.
Shepherd, G. M., Chen, W. R., and Greer, C. A. (2004). The olfactory bulb. In The synaptic organization of the brain Chapter 5, 5 edn., G. M. Shepherd, ed. (New York, Oxford University Press).
Shoppa, N. E., and Westbrook, G. L. (1999). Regulation of synaptic timing in the olfactory bulb by an A-type potassium current. Nat. Neurosci. 2, 1106–1113.
Stanton, P. K. (1996). LTD, LTP, and the sliding threshold for long-term synaptic plasticity. Hippocampus 6, 35–42.
Urban, N. N., and Sakmann, B. (2002). Reciprocal intraglomerular excitation and intra- and interglomerular lateral inhibition between mouse olfactory bulb mitral cells. J. Physiol. 542, 355–367.
Willhite, D. C., Nguyen, K. T., Masurkar, A. V., Greer, C. A., Shepherd, G. M., and Chen, W. R. (2006). Viral tracing identifies distributed columnar organization in the olfactory bulb. Proc. Natl. Acad. Sci. USA 103, 12592–12597.
Keywords: olfactory bulb, olfactory glomeruli, olfactory maps, dendrodendritic synapses, active dendrites, non-Hebbian learning
Citation: M. Migliore, Carlo Inzirillo and Gordon M. Shepherd (2007). Learning mechanism for column formation in the olfactory bulb. Front. Integr. Neurosci. 1:12. doi: 10.3389/neuro.07/012.2007
Received: 5 November 2007;
Paper pending published: 20 November 2007;
Accepted: 5 Decemeber 2007;
Published online: 30 December 2007.
Edited by:
Sidney Simon, Duke University Medical Center, USAReviewed by:
Bernard W. Balleine, University of California Los Angeles, USASidney Simon, Duke University Medical Center, USA
Copyright: © 2007 Migliore, Inzirillo and Shepherd. This is an open-access article subject to an exclusive license agreement between the authors and the Frontiers Research Foundation, which permits unrestricted use, distribution, and reproduction in any medium, provided the original authors and source are credited.
*Correspondence: Gordon M. Shepherd, Department of Neurobiology, Yale University School of Medicine, P. O. Box 208001, New Haven, CT 06520-8001, USA. Tel.: (293)-785-4336. e-mail: gordon.shepherd@yale.edu