- 1Division Laboratories, Pharmacy and Biomedical Genetics, Clinical Pharmacy, University Medical Centre Utrecht, Utrecht, Netherlands
- 2Department of Clinical Pharmacy, Medisch Spectrum Twente, Enschede, Netherlands
- 3Pharmacy and Clinical Pharmacology, Amsterdam University Medical Center, Vrije Universiteit Amsterdam, Amsterdam, Netherlands
- 4Division of Pharmacoepidemiology and Clinical Pharmacology, Utrecht Institute for Pharmaceutical Sciences, Utrecht University, Utrecht, Netherlands
- 5Department of Psychiatry, Rudolf Magnus Institute of Neuroscience, University Medical Center Utrecht, Utrecht, Netherlands
Introduction: Preventing side effects is important to ensure optimal psychopharmacotherapy and therapeutic adherence among psychiatric patients. Obtaining the pharmacogenetic profile of CYP2C19 and CYP2D6 can play an important role in this. When the genotype-predicted phenotype shifts because of the use of co-medication, this is called phenoconversion. The aim was to study the influence of the pharmacogenetic (PGx) profile and phenoconversion on side effects experienced by psychiatric patients.
Methods: A retrospective cohort study was performed using data from 117 patients from a psychiatric outpatient clinic. Patients were genotyped with a psychiatric PGx panel and side effects were evaluated using the Udvalg for Kliniske Undersølgelser side effects rating scale (UKU).
Results: Of all patients, 10.3% and 9.4% underwent phenoconversion (any shift in predicted phenotype) for CYP2C19 and CYP2D6 respectively. No significant associations were found between the phenotype and UKU-score. 75% of the patients with an Intermediate metabolizer (IM) or Poor metabolizer (PM) phenoconverted phenotype of CYP2C19 experienced nausea and vomiting compared to 9.1% of the Normal metabolizer (NM) and Ultrarapid metabolizer (UM) patients (p = 0.033). 64% of the patients with an IM or PM phenoconverted phenotype of CYP2D6 experienced the side effect depression compared to 30.4% NMs and UMs (p = 0.020). CYP2D6 IM and PM patients had a higher concentration-dose ratio than NM patients (p < 0.05).
Discussion: This study underlines the importance to consider phenoconversion when looking at a patient’s genotype. This is important for a better prediction of the phenotype and preventing possible side effects under a specific psychopharmacotherapy.
1 Introduction
A high prevalence of polypharmacy is seen among psychiatric patients (Hefner et al., 2020). Polypharmacy, the use of five or more drugs, is often associated with drug-drug interactions and the risk of side effects. Preventing these side effects is important to ensure optimal psychopharmacotherapy and therapeutic adherence (Bousman et al., 2021).
The use of pharmacogenetics (PGx) contributes to individual patient treatment and can play an important role in preventing side effects (Sharp et al., 2019). PGx can distinct the different genetic variants of genes encoding for cytochrome P450 enzymes (CYP) such as CYP2C19 and CYP2D6 with a different metabolic capacity (KNMP, 2022a; KNMP, 2022b). A patient’s genotype can be translated into the following predicted phenotypes: normal metabolizer (NM), intermediate metabolizer (IM), poor metabolizer (PM) or ultrarapid metabolizer (UM) (Brouwer et al., 2022). Different consortia such as the Dutch Pharmacogenetics Working Group (DPWG) or the Clinical Pharmacogenetics Implementation Consortium (CPIC) have written guidelines regarding dose and pharmacotherapeutic recommendation for each genotype with an actionable drug-gene interaction (DGI) (Abdullah-Koolmees et al., 2021).
CYP2C19 and CYP2D6 are responsible for the metabolism of many psychiatric drugs (Abdullah-Koolmees et al., 2021; Brouwer et al., 2022). In addition, these are also highly polymorphic enzymes and therefore pharmacogenetic advise on these DGIs are widely available (Bousman and Dunlop, 2018; Hahn and Roll, 2021). Furthermore, PGx can help improve and optimize pharmacotherapy for individual patients using psychiatric drugs. Other CYP-enzymes such as CYP1A2, CYP2C9 and CYP3A4 can also play a role in the metabolism of psychiatric drugs. However, the impact of their genotypes on the pharmacokinetics of commonly used psychiatric drugs is less distinct as compared to the effect of CYP2C19 and CYP2D6 genotypes. Previous studies have reported that patients with a predicted phenotype of PM for either CYP2C19 or CYP2D6 have a higher risk of side effects (Chou et al., 2000; Kobylecki et al., 2009; Mrazek et al., 2011). However, in other studies no association between the genotype and the development of side effects has been observed (Peters et al., 2008; Hodgson et al., 2015).
Other non-genetic factors, such as co-medication, also influence the patient’s phenotype, i.e., a patient’s metabolic capacity (Klomp et al., 2020). This can have a significant impact, especially on patients with polypharmacy. This phenomenon, where the predicted metabolic capacity shifts because of the use of co-medication or other non-genetic factors, is called phenoconversion (Hahn and Roll, 2021). In this study, phenoconversion by co-medication will be taken into account.
Research shows that CYP-inhibition or CYP-induction by co-medication often has the greatest influence on NMs and IMs, causing a change in the drug exposure (AUC) (Bahar et al., 2017). For instance, it has been shown that patients using (es)citalopram are more prone to a dose reduction or switching to another antidepressant when there is a drug-drug-interaction combined with a DGI with CYP2C19 (MuhA et al., 2020). However, the relationship between side effects, pharmacogenetic profile and phenoconversion remains to be studied. The aim was to study side effects experienced by psychiatric patients and to identify risk factors including but, not limited to, pharmacogenetic profile of CYP2C19 and CYP2D6 and phenoconversion.
2 Materials and methods
2.1 Study design and population
A retrospective cohort study was performed using the data of the “Body and Life” project (Dutch: Lijf en Leven), for which a non-WMO acknowledgment from the Medical Research Ethics Committee Utrecht has been authorized (number 19-447/C). All patients gave written informed consent. For this study, psychiatric patients were enrolled from the outpatient clinic also named “Body and Life” (Dutch: Lijf en Leven, LL-clinic) of the department of psychiatry from the University Medical Center Utrecht (UMC Utrecht) in the Netherlands (UMC Utrecht, 2022a). Patients were excluded if no genotyping was performed. Patients enrolled between February 2018 and March 2022 were included in the analysis. Data was extracted from the electronic health record.
For the comparison of the distribution of the genotypes, control populations were used. For the control population of CYP2C19, a group of 820 coronary artery disease (CAD) patients who underwent elective coronary stenting was used. For the control population of CYP2D6, a group of 134 healthy controls recruited from hospital personnel was used. These patients have been genotyped as part of other studies, which have been approved by Medical Research Ethics Committee of St. Antonius Hospital Nieuwegein, the Netherlands.
2.2 Drug classification and phenoconversion
The current drug use was documented. Within the drugs used, CYP-substrates as well as CYP-modulators for CYP2C19 and CYP2D6 were identified. CYP-substrate users were defined as a patient who uses a CYP2C19- or CYP2D6 substrate which has a psychiatric indication and where a therapeutic recommendation is given for the DGI by the DPWG (Abdullah-Koolmees et al., 2021; KNMP, 2022c). If no advice was given for a substrate, a literature search was conducted to see if the DGI was of clinical relevance. This was only the case for diazepam, which is considered a CYP2C19-substrate with a pharmacogenetic interaction. (Qin et al., 1999; Skryabin et al., 2020; KNMP, 2022d).
A CYP-modulator was defined as a drug that has a moderate or strong inhibitory or inducing effect on CYP2C19 and/or CYP2D6 (Cicali et al., 2021). There are no known inducers for CYP2D6 (Just et al., 2021). Relevant inhibitors and inducers can be found in Supplementary Material S1. In this study, phenoconversion was defined as the shift of a patient’s phenotype based on the use of co-medication consisting of CYP-modulators. The genotype-predicted phenotype was adjusted to a phenoconverted phenotype (P-CYP2C19 and P-CYP2D6) according to Table 1 (Hahn and Roll, 2021; Just et al., 2021). Phenotypes were classified in the next lower activity phenotype using a moderate inhibitor and an even lower activity phenotype when using a strong inhibitor. For example, a patient who is a CYP2D6 NM but uses fluoxetine, a strong CYP2D6-inhibitor, is a P-CYP2D6 PM. If a patient used a CYP2C19-inducer, a patient was classified into the next higher activity phenotype. Only PMs kept poor activity because increased synthesis of “loss of function proteins” does not change the drug clearance and thus the phenotype.

TABLE 1. Phenoconverted phenotype for CYP2D6 and CYP2C19 based on concomitant use of inhibitor/inducer. The presented phenotypes are what the genotype-predicted phenotypes will convert to when a moderate inhibitor, strong inhibitor or inducers is taken concomitantly.
2.3 Genotyping
DNA-diagnostics were performed at Erasmus MC clinical laboratory in Rotterdam, Netherlands (Erasmus, 2022). Genotypes were translated to corresponding phenotypes recognized by the DPWG (Abdullah-Koolmees et al., 2021; KNMP, 2022a). Patients were tested for CYP1A2, CYP2C9, CYP2C19, CYP2D6 and CYP3A4, but only the genotypes of CYP2C19 and CYP2D6 were used. CYP1A2 was not included because there were no actionable DGIs according to the DPWG. CYP2C9 was not included because no patient used a CYP2C9-metabolized psychiatric drug with a relevant DGI and for CYP3A4 were no PMs identified (only phenotype with actionable PGx recommendation).
2.4 Side effect registration
Side effects were evaluated using the Udvalg for Kliniske Undersølgelser side effects rating scale (UKU) for the registration of side effects of psychotropic drugs (Lingjærde et al., 1987). In the UKU, every side effect is rated in a four-point scale, and all scores were added together to get a total score. A higher score implies a higher rate of side effects or more severe side effects. An adapted version of the UKU-rating scale specifically for the LL-clinic was used, from which the extrapyramidal symptoms were excluded from the category neurologic side effects and can be found in the Supplementary Material S2. The UKU-questionnaire was conducted orally by a nurse specialist via a semi structured interview with the patient.
2.5 Concentration-dose ratios
Next to a blood sample for the DNA-diagnostics, there was also a sample send to the pharmacy laboratory of UMC Utrecht for determination of drug levels in plasma (UMC Utrecht, 2022b). This was done for all psychiatric drugs a patient used at the time of measurement. For each patient a blood sample was taken in the morning, with medication taken the night before but not in the morning. Based on the drug concentration (in μg/L) and the registered dose (in mg), the concentration-dose ratio (CD-ratio) was calculated in μg/l/mg for further analysis. Only the drugs with at least two users in the different phenotypic groups were included for further analysis.
2.6 Statistical analysis
Continuous variables were presented as mean and standard deviation (median and range if non-normal distributed) and categorical variables as frequency and percentage. Comparisons of normal distributed continuous variables were performed with a student’s T-test. For non-normal distributed variables, a Mann-Whitney U-test was used or a Kruskal Wallis test if there were more than two groups. For categorical variables, a chi-squared test was used. Only CYP-substrate users were included in the phenotype-specific analysis and NM was seen as the reference phenotype.
Associations between the UKU-score and patient characteristics were analyzed using binary logistic regression. Phenotypes were combined, i.e., NM with UM and IM with PM, because of the small sample size per phenotype group. Although there are known distinct PK differences between these phenotypes, it is hypothesized that the IMs and PMs will experience more side effects than the NMs and UMs when receiving standard doses. For the analysis of the UKU-score the score was divided into three categories (low, moderate and high) based on tertiles. An univariate as well as a multivariate analysis was performed, adjusting for body mass index (BMI), psychiatric diagnosis and polypharmacy.
The comparison of the prevalence of specific side effects was only done for CYP-related side effects, which were determined for a project of the Ubiquitous Pharmacogenomics Consortium. Five researchers assessed each side effect based on clinical studies, the Summary of Product Characteristics and expert-opinions. The outcomes of the assessments [unpublished], i.e., whether specific side effects of drugs are genotype dependent, was used. Of the UKU-questionnaire, 33 of the 39 side effects were considered CYP-related (see Table 5; Table 6 for the included side effects).
A p-value < 0.05 was considered statistically significant. All statistical analyses were performed using IBM SPSS Statistics (Version 26.0.0.1, IBM Corp., Armonk, NY, United States).
3 Results
In total, 117 LL-patients were eligible for this study, of which 104 (88.9%) LL-patients filled in the UKU-questionnaire and could be included in the analysis (Figure 1). Of the 104 LL-patients who were included in the analysis, 15 (14,4%) LL-patients concomitantly used a CYP2C19-substrate and 49 (47,1%) a CYP2D6-substrate. Table 2 shows the baseline characteristics of the study population. Figure 2 compares the distribution of the different genotype-predicted phenotypes of CYP2C19 and CYP2D6. The proportion of UMs in CYP2C19 was significantly larger for the LL-patients (8.5%) compared to the CAD-patients (4.0%).
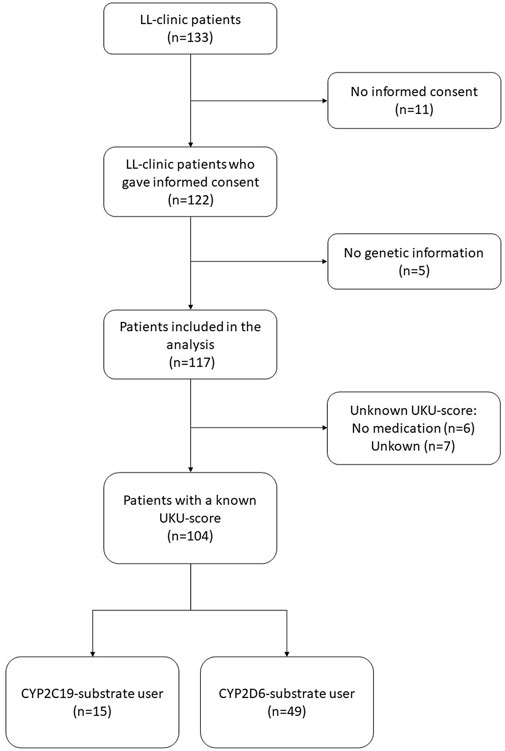
FIGURE 1. Selection of LL-clinic patients included into this study. Abbreviations LL-patients patients from “Body and Life” outpatient clinic; UKU Udvalg for Kliniske Undersølger side effects rating scale.
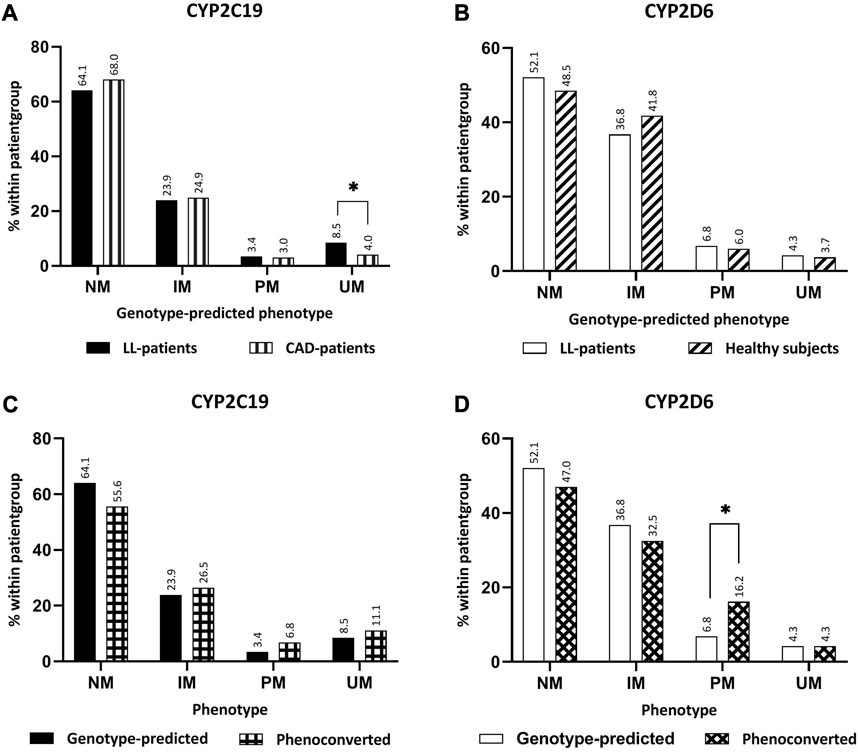
FIGURE 2. Comparison between phenotypes. (A) Comparison genotype-predicted phenotype of CYP2C19 between LL-patients (n = 117) and CAD-patients (n = 820). (B) Comparison genotype-predicted phenotype of CYP2D6 between LL-patients (n = 117) and healthy subjects (n = 134). (C) Comparison of genotype-predicted phenotypes versus phenoconverted phenotypes CYP2C19. (D) Comparison of genotype-predicted phenotypes versus phenoconverted phenotypes CYP2D6. Significant differences (p < 0.05) are depicted with an *. Abbreviations NM, normal metabolizer; IM, intermediate metabolizer; PM, poor metabolizer; UM, ultrarapid metabolizer; LL-patients patients from “Body and Life” outpatient clinic; CAD-patients Coronary artery disease patients.
Approximately 10% of the LL-patients underwent phenoconversion for either CYP2C19 or CYP2D6 (Table 2). The comparison of the distributions of CYP2D6 before and after phenoconversion, revealed a significant difference between the proportions of PMs (Figure 2). In the genotype-predicted phenotypes, 6.8% of the patients were a PM of CYP2D6, which was 16.2% of the P-CYP2D6 patients (p < 0.05). No significant differences were found within CYP2C19 phenotype groups.
Comparing the total UKU-score, significant differences were found for the main diagnosis when comparing a psychotic diagnosis category (median score 13) to non-psychotic category (median score 22, p < 0.05) (Table 3). There was also a significant difference in the total UKU-score considering polypharmacy (patients without polypharmacy had a median score of 11, patients with polypharmacy a median score of 20, p < 0.05). The UKU-score was higher for patients with BMI over 40, but this was not a significant difference (p = 0.064; median BMI < 30 is 13, median BMI 30–40 is 15 and median BMI > 40 is 23.5). No significant differences were found for age and gender.
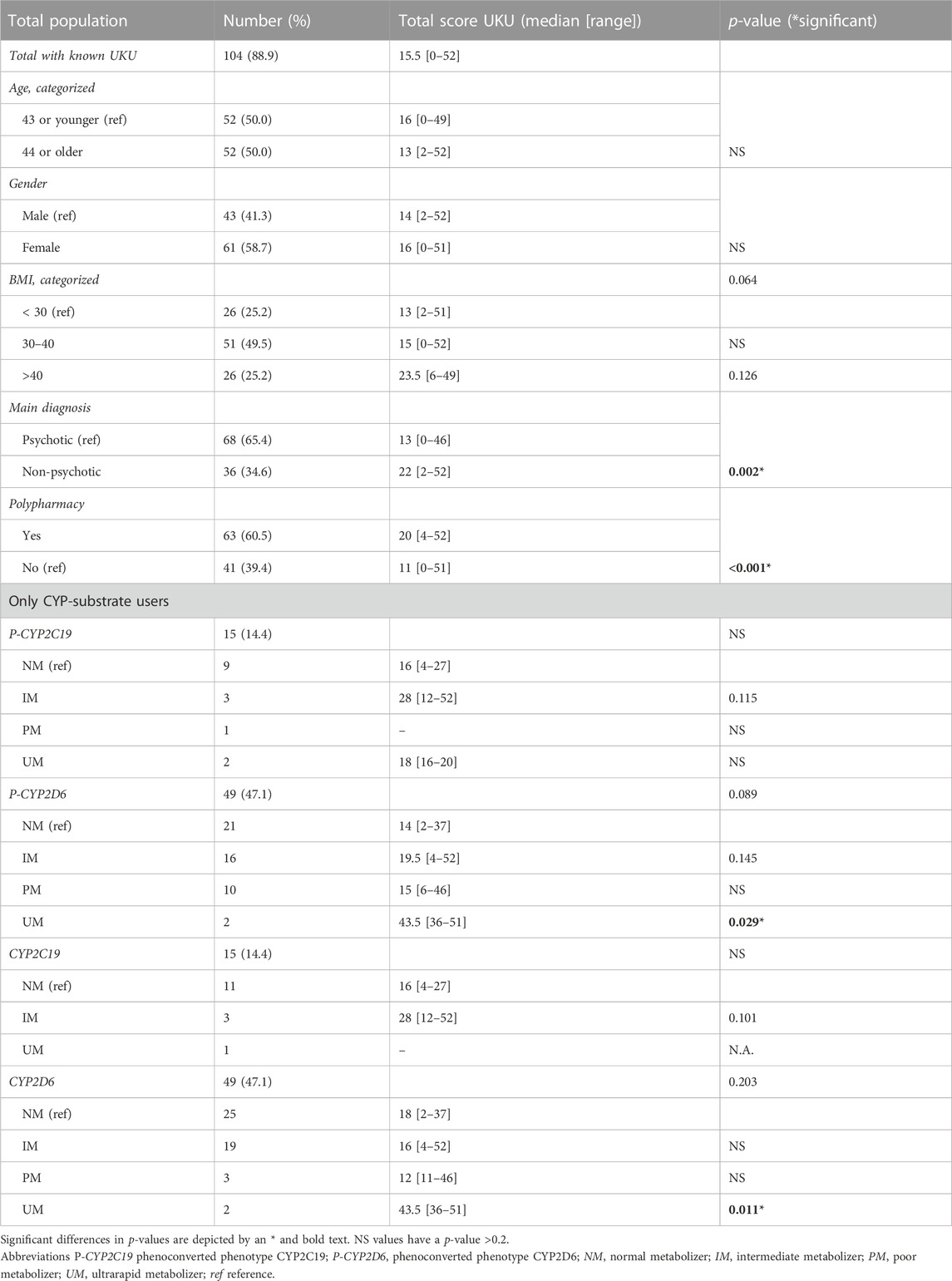
TABLE 3. Comparisons of UKU-score with baseline characteristics and comparison of phenotypes CYP-substrate users and UKU-score.
Patients with a non-psychotic main diagnosis had an odds ratio (OR) of 2.60 (95% CI: 1.04–6.54; adjusted OR 2.43; 95% CI: 0.90–6.55, Table 4) for higher UKU-score. Patients with polypharmacy had an OR of 4.47 (95% CI: 1.90–10.53; adjusted OR 4.26; 95% CI: 1.76–10.32) for higher UKU-score. No other statistical differences between UKU-score and covariates were found.
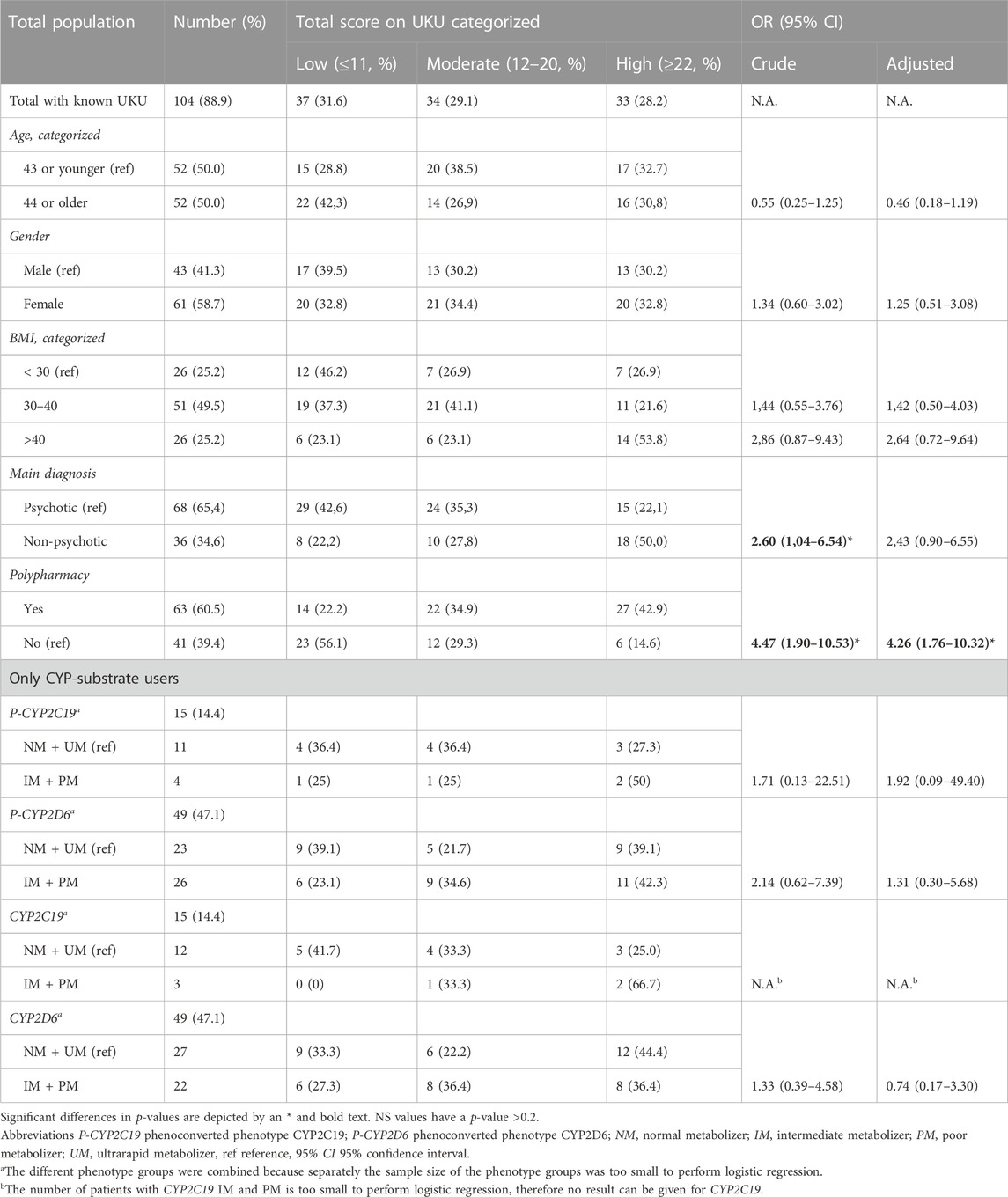
TABLE 4. Association between side effects with baseline characteristics and phenotypes of CYP-substrate users and UKU-score.
There was no increase of the total UKU-score associated with the genotype-predicted phenotypes of CYP2C19 and CYP2D6 (Table 3; Table 4). For P-CYP2D6, there was a significant difference between the total UKU-score of the NM (median 14) and UM (median 43.5, p < 0.05). There were no significant differences for IM (median 19.5) and PM (median 15). For P-CYP2C19, no increase in total UKU-score was seen considering the different phenotypes.
No significant differences were seen in the prevalence of certain side effects comparing the different genotype-predicted phenotypes of CYP2C19 (Table 5) and CYP2D6 (Table 6). Considering phenoconversion, several differences were noted. For P-CYP2C19, there was a higher prevalence of nausea and/or vomiting in the IM and PM group (75.0%) compared to the NM and UM group (9.1%, p < 0.05, Table 5). There were no further side effects with significant between-group differences. Comparing the prevalence of specific side effects and P-CYP2D6, there was a significant difference in the prevalence of depression (Table 6). 30.4% of the NMs and UMs experienced this side effect compared to 64.0% of the IMs and PMs. The IMs and PMs also experienced a higher rate of increased dream activity (48.0%) compared to the NMs and UMs (21.7%), but this result was not statistically significant (p = 0.057). The same is true for sleepiness, which 76.0% of the IMs and PMs experienced compared to 52.2% of the NMs and UMs (p = 0.085), and nausea and/or vomiting, which 36,0% of the IMs and PMs experienced compared to 13,0% for the NMs and UMs (p = 0.067). More patients in the NM and UM group of P-CYP2D6 (13.6%) experienced gynecomastia, compared to 0% of the IMs and PMs. However, this was also not statistically significant (p = 0.095). Other side effects did also not show any significant differences.
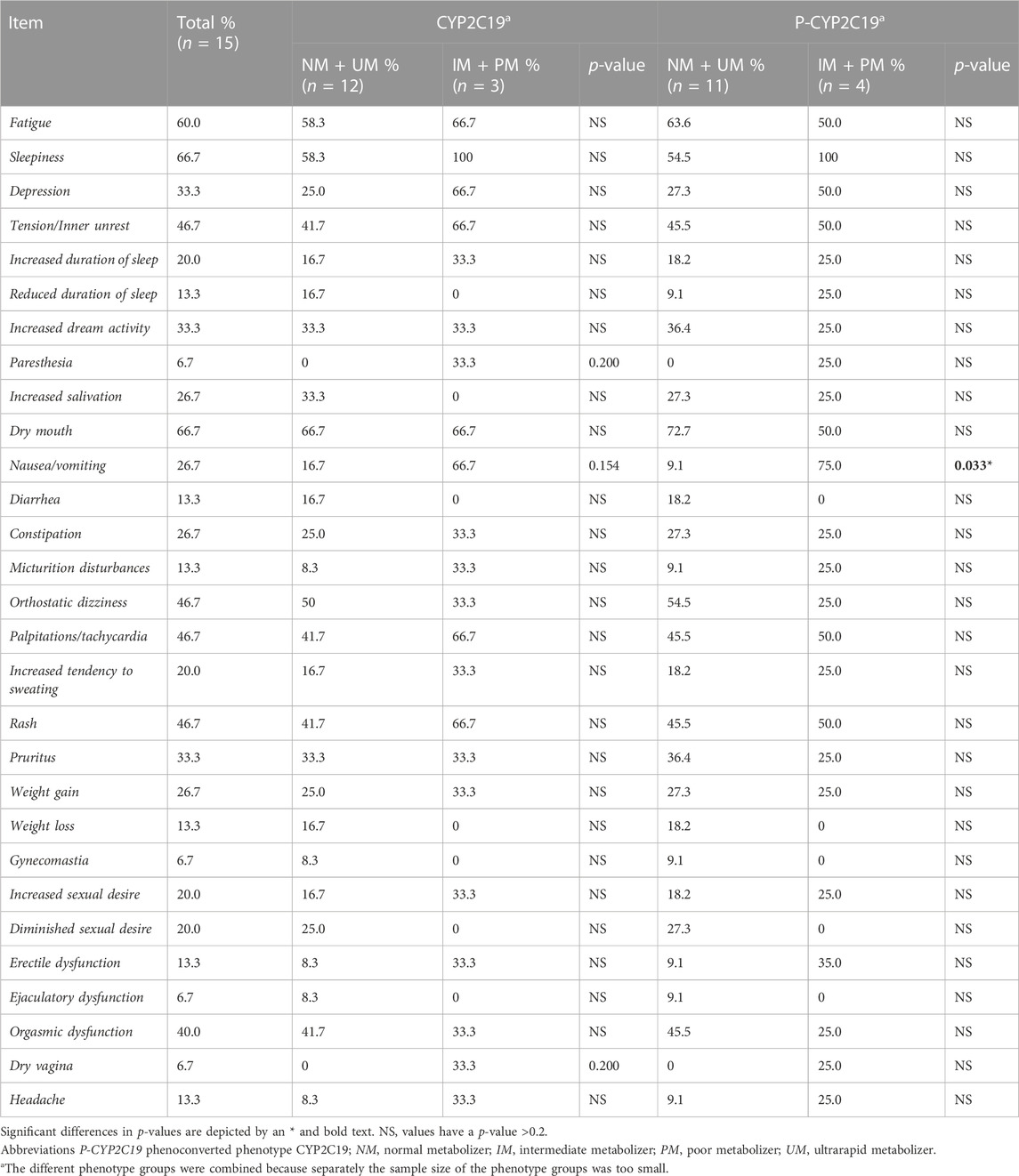
TABLE 5. Comparison of specific CYP-related side-effects and phenotype of CYP2C19 in CYP2C19-substrate users. The side effects epileptic seizures, amenorrhea, galactorrhea and gynecomastia were not included because they were not present in any of the patients.
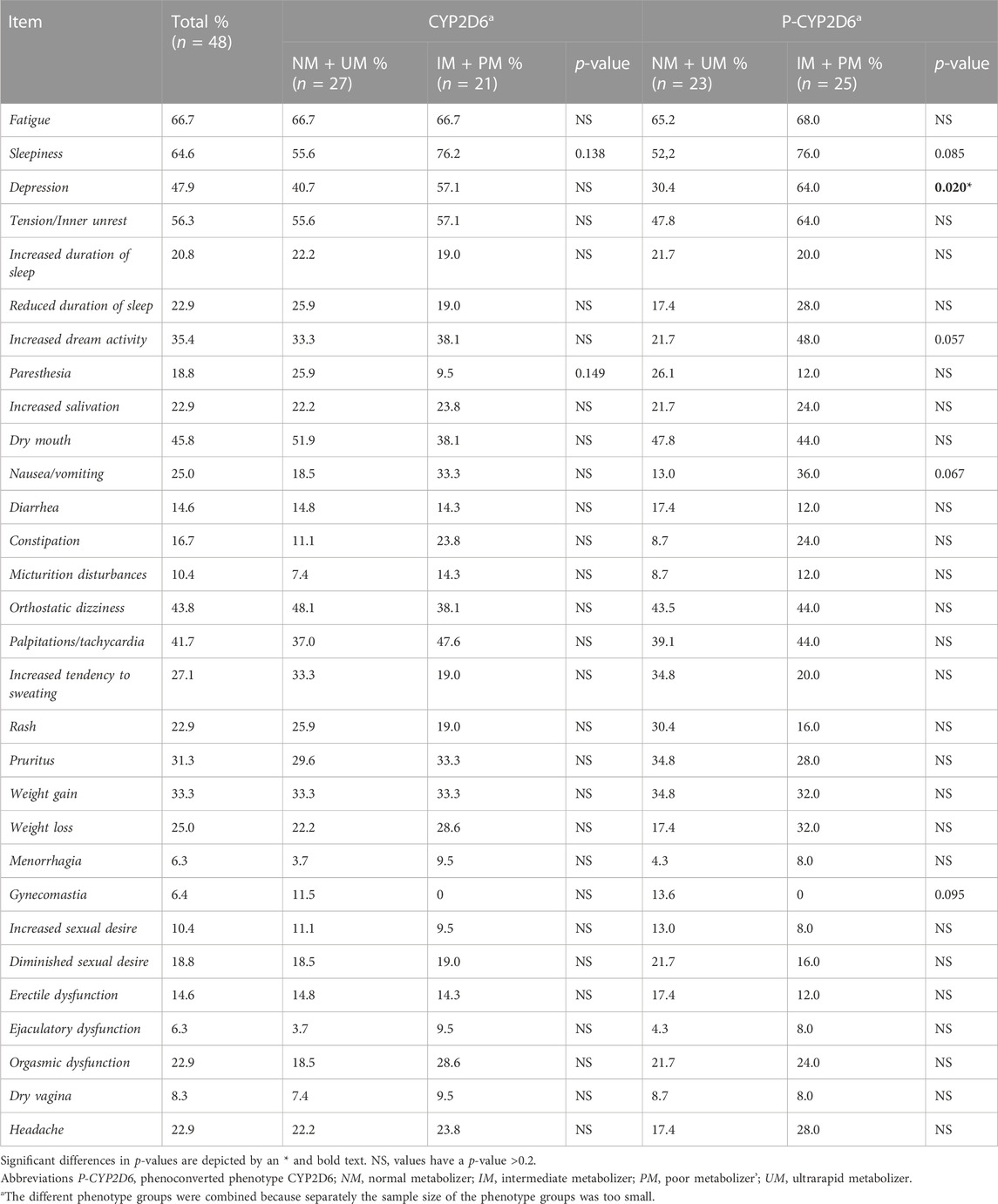
TABLE 6. Comparison of specific CYP-related side-effects and phenotype of CYP2D6 in CYP2D6-substrate users. The side effects epileptic seizures, amenorrhea and galactorrhea were not included because they were not present in any of the patients.
For the comparison of the CD-ratio, the number of patients was sufficient for the analysis of CYP2D6-substrates aripiprazole, risperidone, haloperidol and venlafaxine. From the data in Figure 3, it was apparent that the concentration-dose ratio of aripiprazole is significantly higher for IMs and PMs than NMs with (7.55 ± 3.46 μg/l/mg versus 16.03 ± 4.92 μg/l/mg) and without (8.98 ± 5.16 μg/l/mg versus 15.43 ± 5.25 μg/l/mg) considering phenoconversion. For risperidone, haloperidol and venlafaxine no statistic significant differences were seen.
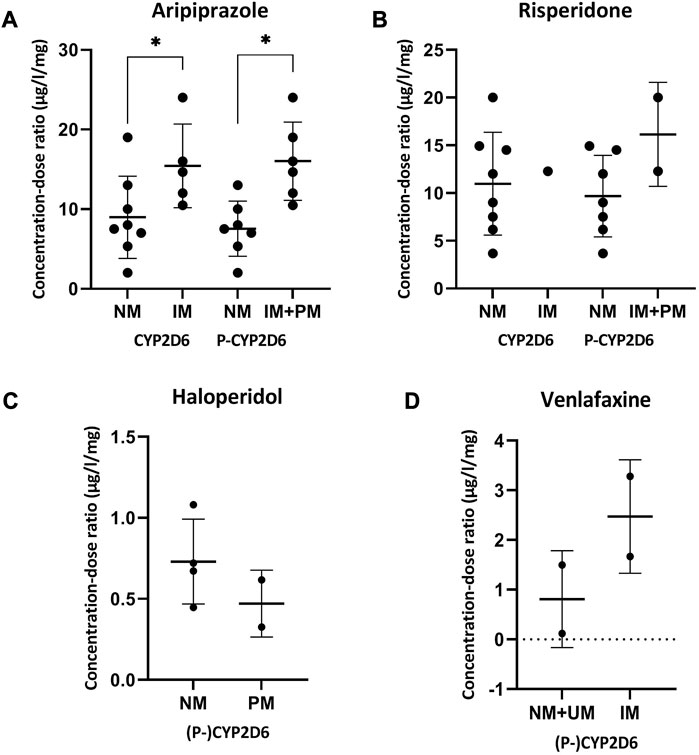
FIGURE 3. Concentration-dose ratios with SD of aripiprazole (A), risperidone (B), haloperidol (C) and venlafaxine (D) and the phenoconverted phenotype of CYP2D6. Significant differences (p < 0.05) are depicted with an *. The different phenotype groups were combined because separately the sample size of the phenotype groups was too small. For haloperidol and venlafaxine there was no difference in the patients in the different phenotype groups considering phenoconversion or not. Abbreviations P-CYP2C19, phenoconverted phenotype CYP2C19; P-CYP2D6, phenoconverted phenotype CYP2D6; SD, standard deviation; NM, normal metabolizer; IM, intermediate metabolizer; PM, poor metabolizer; UM, ultrarapid metabolizer.
4 Discussion
This study shows that in 10% of the psychiatric patients any form of phenoconversion, where the predicted phenotype shifts based on genotype and co-medication, occurred. It also plays a role in the side effects experienced by these patients. This study shows that specific side effects such as nausea and depression are more prevalent in patients with an IM or PM phenoconverted phenotype of CYP2C19 and CYP2D6.
In this study population, there were significantly more CYP2C19 UM patients compared to the control population consisting of CAD-patients. In the Dutch Caribbean population, in which the phenotype distribution is comparable to Caucasians, a study found no differences at all in the prevalence of specific CYP2D6 or CYP2C19 phenotypes in psychiatric patients (Koopmans et al., 2017). This difference may be explained by the fact that the UMC Utrecht is a tertiary care center, to which patients are only referred if the treatment in the first or secondary line of care was not adequate. In a previous study in an American tertiary psychiatric hospital, a higher prevalence of genetic variants leading to a phenotype other than NM was seen (Ruaño et al., 2008). Looking at the distribution of phenotypes before and after phenoconversion, there were specifically more P-CYP2D6 PM patients. These findings seem to be consistent with the existing literature (Preskorn et al., 2013; Mostafa et al., 2019). This shows it is important to consider phenoconversion when predicting a patient’s phenotype. For CYP2C19 and CYP2D6, the phenotype influences the efficacy and tolerability of antidepressants and consequently there are different pharmacotherapeutic recommendations for each specific genotype-predicted phenotype (Gressier et al., 2015; Brouwer et al., 2022; Campos et al., 2022). There are also pharmacotherapeutic recommendations available for antipsychotics (Beunk et al., 2023). So, if the genotype-predicted phenotype shifts because of phenoconversion, it is possible that other recommendations are given.
Based on the total UKU-score, no statistically significant associations were found between the genotype-predicted or phenoconverted phenotype and amount and/or severity of side effects. However, the OR of the CYP2D6 phenoconverted phenotype was 1.31 and higher than 0.74, the OR of the CYP2D6 genotype-predicted phenotype. No comparison could be made for CYP2C19 because no logistic regression could be performed in the genotype-predicted group. In other studies, it has also been seen that there is a stronger association between phenoconverted phenotype and antidepressant efficacy and not necessarily between genotype-predicted phenotype and the antidepressant efficacy (Gressier et al., 2015).
This study found that there are also some non-genetic factors that influence the UKU-score of psychiatric patients. Patients who have polypharmacy have an OR of 4.26 for a moderate or high total UKU-score and therefore experience more or more severe side effects. These results are consistent with other studies, the higher the number of drugs a patient uses, the higher the chance of side effects and drug-drug interactions (Maher et al., 2014; Malki and Pearson, 2020).
A non-psychotic diagnosis also seemed to have an influence on the amount of side effects experienced. LL-patients with a non-psychotic diagnosis had a crude OR of 2.60 compared to LL-patients with a psychotic diagnosis. However, this result was not statistically significant when it was corrected for other covariables. The relationship between diagnosis and side effects is not clear, because several other underlying factors can play a role. The diagnosis of patients not only tells us something about the psychiatric disease, but also about the possible pharmacotherapy with associated side effects. Moreover, patients enrolled in the LL-clinic typically have more complex and more persistent mental disorders and therefore the current pharmacotherapy may lead to more side effects.
BMI may also play a role in the amount and/or severity of the side effects experienced. Patients with a BMI > 40 had a median UKU-score of 23.5, which was significantly higher than patients with a BMI between 30 and 40 or lower than 30, who respectively had scores of 15 and 13. However, this result was not seen when looking at the association between the UKU-score and the BMI as the OR was not statistically significant. Patients with a higher BMI often have a different response to antidepressants or antipsychotics, most of the time needing higher doses and thus experiencing more side effects (Warrings et al., 2021). Obesity and psychiatric disease have a complex bidirectional relationship (Holt and Peveler, 2009; Woo et al., 2016). Therefore, it is important to also consider the weight effects of a certain drug when choosing effective treatment for the mental disorder (McElroy, 2009). These aspects are already incorporated into the LL-clinic at the UMC Utrecht (UMC Utrecht, 2022a).
In this study it was also possible to look at the prevalence of CYP-specific side effects. It was found that 75.0% of the P-CYP2C19 IMs and PMs experience nausea and vomiting compared to 9.1% of the NMs and UMs. Another study also found CYP2C19 PMs have a higher chance of gastro-intestinal side effects when using an SSRI (Fabbri et al., 2018). Side effects that occurred more often in P-CYP2D6 IMs and PMs were depression, increased dream activity and sleepiness. 64.0% P-CYP2D6 IMs and PMs experienced the side effect depression compared to 30.4% of the NMs and UMs. The side effect depression is associated with antipsychotic use, possibly related to hyperprolactinemia (Milano et al., 2017). However, the relationship between hyperprolactinemia and CYP2D6 phenotype is unclear (Calafato et al., 2020). For the sleep-related side effects, some research indicates that these side effects are CYP-related, but different factors may influence this. For example, patients using a SSRI metabolized by CYP2D6 more often report nightmares as a side effect (Eugene, 2019). However, it also seems that psychiatric patients in general more often have more vivid dreams (Schredl and Schredl, 2018). The same goes for the relationship between sleep and psychiatric disorders (Krystal, 2012). Gynecomastia was a side effect more often seen in P-CYP2D6 NM + UM group. However, this side effect occurred specifically by the two UM patients. These two patients had a very complex background, having multiple comorbidities and/or switching drugs when the questionnaire was filled out, and therefore it is unsure if the occurrence of gynecomastia is due to the phenotype of these patients.
Lastly, next to the UKU-results, there was also an analysis of the drug concentration in plasma, corrected for the dose and phenotype. A two times higher CD-ratio was seen for (P-)CYP2D6 IMs and PMs, which was statistically significant. These results are consistent with the findings of previous work (Jukic et al., 2019; Kiss et al., 2020).
This study has several strengths. First, general genotyping (i.e., without a specific reason) is not standard clinical practice for psychiatric patients. However, for each patient enrolled in the LL-clinic, a psychiatric PGx panel was performed. Moreover, this study considered phenoconversion, specifically by co-medication, and its effect on the phenotype. Most studies in the field currently focus on the genotype-predicted phenotype and do not take the effect of co-medication into account (Shah et al., 2016). Specifically in the psychiatric population there is a lot of drugs that are known CYP-modulators, so it is an important factor (Flockhart et al., 2022). For future research it is important to look at other factors that influence phenoconversion such as smoking, alcohol consumption and disease state (Klomp et al., 2020). Third, the UKU is a validated standardized questionnaire, specifically for psychiatric drug-users (Lingjærde et al., 1987).
The current study was, however, limited by the sample size. Only one patient per week was enrolled in the LL-clinic. This could be a limitation specifically for phenotypes like UM and PM because of their lower prevalence. Moreover, not every patient used a CYP-substrate drug and therefore not all patients could be included in the analysis of CYP-substrate users and phenotype and phenotype groups had to be combined for some of the analyses. To include enough PMs and UMs, large-sample prospective trials need to be conducted. Another limitation is the data collection. Genotypes and drug use had to be obtained manually. To minimize the chance of errors, this was done in a standardized manner. Lastly, extrapyramidal symptoms were not included in the adapted version of the UKU-questionnaire. Also, although side effects were collected through the validated UKU-questionnaire, some side effects may need to be objectified using laboratory tests or physical examinations (Lingjærde et al., 1987).
In conclusion, this study shows that phenoconversion is important to consider when looking at a patient’s genotype. In the psychiatric population, where a difference in genotype distribution is observed, this phenomenon causes a shift from one phenotype to another. Although no significant associations were found between the phenotype and side effects experienced, there was a difference in the occurrence of specific side effects for the different phenoconverted phenotypes. More research on this topic is important to take the next step towards better prediction of a patient’s phenotype and possible prevention of side effects, contributing to personalized medicine.
Data availability statement
The original contributions presented in the study are included in the article/Supplementary Material, further inquiries can be directed to the corresponding author.
Ethics statement
The studies involving humans were approved by the Medical Research Ethics Committee Utrecht has been authorized (number 19-447/C). The studies were conducted in accordance with the local legislation and institutional requirements. The participants provided their written informed consent to participate in this study.
Author contributions
Conceptualization: MdU, HH, WC, IW, and VD; Methodology: MdU, VD, and HA-K; Formal Analysis: MdU; Supervision: VD and HA-K; Preparing manuscript: MdU; Writing–first draft: MdU, HH, and VD; Writing–review and editing: MdU, HH, KW, HA-K, WC, IW, and VD. All authors contributed to the article and approved the submitted version.
Acknowledgments
The authors would like to thank J. E. M. Van Baaren (research coordinator psychiatry), J. Van Driesum (nurse specialist psychiatry), Z. S. R. Boudewijns (datamanager) and W. H. M. Broeders (datamanager) for providing data and relevant information for this research.
Conflict of interest
The authors declare that the research was conducted in the absence of any commercial or financial relationships that could be construed as a potential conflict of interest.
Publisher’s note
All claims expressed in this article are solely those of the authors and do not necessarily represent those of their affiliated organizations, or those of the publisher, the editors and the reviewers. Any product that may be evaluated in this article, or claim that may be made by its manufacturer, is not guaranteed or endorsed by the publisher.
Supplementary material
The Supplementary Material for this article can be found online at: https://www.frontiersin.org/articles/10.3389/fgene.2023.1249164/full#supplementary-material
Abbreviations
CAD, Coronary artery disease; DGI, Drug-gene interaction; DPWG, Dutch Pharmacogenetics Working Group; EPS, Extrapyramidal symptoms; LL-clinic, “Body and Life” outpatient clinic (Dutch: Lijf en Leven); LL-patients, Patients from “Body and Life” outpatient clinic; P-CYP2C19, Phenotype CYP2C19 after phenoconversion; P-CYP2D6, Phenotype CYP2D6 after phenoconversion; UKU, Udvalg for Kliniske Undersølger side effects rating scale.
References
Abdullah-Koolmees, H., van Keulen, A. M., Nijenhuis, M., and Deneer, V. H. M. (2021). Pharmacogenetics guidelines: overview and comparison of the DPWG, CPIC, CPNDS, and RNPGx guidelines. Front. Pharmacol. 11, 595219. doi:10.3389/fphar.2020.595219
Bahar, M. A., Setiawan, D., Hak, E., and Wilffert, B. (2017). Pharmacogenetics of drug–drug interaction and drug–drug–gene interaction: a systematic review on CYP2C9, CYP2C19 and CYP2D6. Pharmacogenomics 18 (7), 701–739. doi:10.2217/pgs-2017-0194
Beunk, L., Nijenhuis, M., Soree, B., de Boer-Veger, N. J., Buunk, A. M., Guchelaar, H. J., et al. (2023). Dutch Pharmacogenetics Working Group (DPWG) guideline for the gene-drug interaction between CYP2D6, CYP3A4 and CYP1A2 and antipsychotics. Eur. J. Hum. Genet. 2023. doi:10.1038/s41431-023-01347-3
Bousman, C. A., Bengesser, S. A., Aitchison, K. J., Amare, A. T., Aschauer, H., Baune, B. T., et al. (2021). Review and consensus on pharmacogenomic testing in psychiatry. Pharmacopsychiatry 54 (1), 5–17. doi:10.1055/a-1288-1061
Bousman, C. A., and Dunlop, B. W. (2018). Genotype, phenotype, and medication recommendation agreement among commercial pharmacogenetic-based decision support tools. Pharmacogenomics J. 18 (5), 613–622. doi:10.1038/s41397-018-0027-3
Brouwer, J. M. J. L., Nijenhuis, M., Soree, B., Guchelaar, H. J., Swen, J. J., van Schaik, R. H. N., et al. (2022). Dutch Pharmacogenetics Working Group (DPWG) guideline for the gene-drug interaction between CYP2C19 and CYP2D6 and SSRIs. Eur. J. Hum. Genet. 30 (10), 1114–1120. doi:10.1038/s41431-021-01004-7
Calafato, M. S., Austin-Zimmerman, I., Thygesen, J. H., Sairam, M., Metastasio, A., Marston, L., et al. (2020). The effect of CYP2D6 variation on antipsychotic-induced hyperprolactinaemia: a systematic review and meta-analysis. Pharmacogenomics J. 20 (5), 629–637. doi:10.1038/s41397-019-0142-9
Campos, A. I., Byrne, E. M., Mitchell, B. L., Wray, N. R., Lind, P. A., Licinio, J., et al. (2022). Impact of CYP2C19 metaboliser status on SSRI response: a retrospective study of 9500 participants of the Australian genetics of depression study. Pharmacogenomics J. 22 (2), 130–135. doi:10.1038/s41397-022-00267-7
Chou, W. H., Yan, F. X., de Leon, J., Barnhill, J., Rogers, T., Cronin, M., et al. (2000). Extension of a pilot study: impact from the cytochrome P450 2D6 polymorphism on outcome and costs associated with severe mental illness. J. Clin. Psychopharmacol. 20 (2), 246–251. doi:10.1097/00004714-200004000-00019
Cicali, E. J., Elchynski, A. L., Cook, K. J., Houder, J. T., Thomas, C. D., Smith, D. M., et al. (2021). How to integrate CYP2D6 phenoconversion into clinical pharmacogenetics: a tutorial. Clin. Pharmacol. Ther. 110 (3), 677–687. doi:10.1002/cpt.2354
Erasmus, M. C. (2022). Farmacogenetica [internet]. Available from: https://www.erasmusmc.nl/nl-nl/patientenzorg/laboratoriumspecialismen/farmacogenetica (Accessed April 13, 2022).
Eugene, A. R. (2019). Optimizing drug selection in psychopharmacology based on 40 significant CYP2C19- and CYP2D6-biased adverse drug reactions of selective serotonin reuptake inhibitors. PeerJ 7, e7860. doi:10.7717/peerj.7860
Fabbri, C., Tansey, K. E., Perlis, R. H., Hauser, J., Henigsberg, N., Maier, W., et al. (2018). Effect of cytochrome CYP2C19 metabolizing activity on antidepressant response and side effects: meta-analysis of data from genome-wide association studies. Eur. Neuropsychopharmacol. 28 (8), 945–954. doi:10.1016/j.euroneuro.2018.05.009
Flockhart, D. A., Thacker, D., and McDonald, C. D. Z. (2022). The flockhart cytochrome P450 drug-drug interaction table. Indiana: Division of Clinical Pharmacology, Indiana University School of Medicine.
Gressier, F., Verstuyft, C., Hardy, P., Becquemont, L., and Corruble, E. (2015). Response to CYP2D6 substrate antidepressants is predicted by a CYP2D6 composite phenotype based on genotype and comedications with CYP2D6 inhibitors. J. Neural Transm. 122 (1), 35–42. doi:10.1007/s00702-014-1273-4
Hahn, M., and Roll, S. C. (2021). The influence of pharmacogenetics on the clinical relevance of pharmacokinetic drug-drug interactions: drug-gene, drug-gene-gene and drug-drug-gene interactions. Pharm. (Basel) 14 (5), 487. doi:10.3390/ph14050487
Hefner, G., Wolff, J., Hahn, M., Hiemke, C., Toto, S., Roll, S. C., et al. (2020). Prevalence and sort of pharmacokinetic drug–drug interactions in hospitalized psychiatric patients. J. Neural Transm. 127 (8), 1185–1198. doi:10.1007/s00702-020-02214-x
Hodgson, K., Tansey, K. E., Uher, R., Dernovšek, M. Z., Mors, O., Hauser, J., et al. (2015). Exploring the role of drug-metabolising enzymes in antidepressant side effects. Psychopharmacol. Berl. 232 (14), 2609–2617. doi:10.1007/s00213-015-3898-x
Holt, R. I. G., and Peveler, R. C. (2009). Obesity, serious mental illness and antipsychotic drugs. Diabetes Obes. Metab. 11 (7), 665–679. doi:10.1111/j.1463-1326.2009.01038.x
Jukic, M. M., Smith, R. L., Haslemo, T., Molden, E., and Ingelman-Sundberg, M. (2019). Effect of CYP2D6 genotype on exposure and efficacy of risperidone and aripiprazole: a retrospective, cohort study. Lancet Psychiatry 6 (5), 418–426. doi:10.1016/S2215-0366(19)30088-4
Just, K. S., Dormann, H., Freitag, M., Schurig, M., Böhme, M., Steffens, M., et al. (2021). CYP2D6 in the brain: potential impact on adverse drug reactions in the central nervous system—results from the ADRED study. Front. Pharmacol. 12, 624104. doi:10.3389/fphar.2021.624104
Kiss, Á., Menus, Á., Tóth, K., Déri, M., Sirok, D., Gabri, E., et al. (2020). Phenoconversion of CYP2D6 by inhibitors modifies aripiprazole exposure. Eur. Arch. Psychiatry Clin. Neurosci. 270 (1), 71–82. doi:10.1007/s00406-018-0975-2
Klomp, S. D., Manson, M. L., Guchelaar, H. J., and Swen, J. J. (2020). Phenoconversion of cytochrome P450 metabolism: a systematic review. J. Clin. Med. 9, 2890. doi:10.3390/jcm9092890
KNMP (2022a). Algemene achtergrondtekst farmacogenetica - CYP2C19 [internet]. Available from: https://kennisbank.knmp.nl/files/farmacogenetica/Achtergrondteksten/cyp2c19.pdf (Accessed March 8, 2022).
KNMP (2022b). Algemene achtergrondtekst farmacogenetica - CYP2D6 [internet]. Available from: https://kennisbank.knmp.nl/files/farmacogenetica/Achtergrondteksten/cyp2d6.pdf (Accessed March 8, 2022).
KNMP (2022c). Diazepam [internet]. Available from: https://kennisbank.knmp.nl/article/Informatorium_Medicamentorum/S167.html (Accessed June 2, 2022).
KNMP (2022d). Farmacogenetica [internet]. Available from: https://kennisbank.knmp.nl/article/farmacogenetica/intro.html (Accessed March 13, 2022).
Kobylecki, C. J., Jakobsen, K. D., Hansen, T., Jakobsen, I. V., Rasmussen, H. B., and Werge, T. (2009). CYP2D6 genotype predicts antipsychotic side effects in schizophrenia inpatients: a retrospective matched case-control study. Neuropsychobiology 59 (4), 222–226. doi:10.1159/000223734
Koopmans, A. B., Vinkers, D. J., Gelan, P. J. A., Hoek, H. W., and van Harten, P. N. (2017). CYP2D6 and CYP2C19 genotyping in psychiatric patients on psychotropic medication in the former Dutch Antilles. Pharmacogenomics 18 (10), 1003–1012. doi:10.2217/pgs-2017-0011
Krystal, A. D. (2012). Psychiatric disorders and sleep. Neurol. Clin. 30 (4), 1389–1413. doi:10.1016/j.ncl.2012.08.018
Lingjærde, O., Ahlfors, U. G., Bech, P., Dencker, S. J., and Elgen, K. (1987). The UKU side effect rating scale: a new comprehensive rating scale for psychotropic drugs and a cross-sectional study of side effects in neuroleptic-treated patients. Acta Psychiatr. Scand. 76 (334), 1–100. doi:10.1111/j.1600-0447.1987.tb10566.x
Maher, R. L., Hanlon, J., and Hajjar, E. R. (2014). Clinical consequences of polypharmacy in elderly. Expert Opin. Drug Saf. 13 (1), 57–65. doi:10.1517/14740338.2013.827660
Malki, M. A., and Pearson, E. R. (2020). Drug–drug–gene interactions and adverse drug reactions. Pharmacogenomics J. 20 (3), 355–366. doi:10.1038/s41397-019-0122-0
McElroy, S. L. (2009). Obesity in patients with severe mental illness: overview and management. J. Clin. Psychiatry 70 (3), 12–21. doi:10.4088/JCP.7075su1c.03
Milano, W., Colletti, C., and Capasso, A. (2017). Hyperprolactinemia induced by antipsychotics: from diagnosis to treatment approach. Endocr. Metab. Imune Disord. Drug Targets 17 (1), 38–55. doi:10.2174/1871530317666170424102332
Mostafa, S., Kirkpatrick, C. M. J., Byron, K., and Sheffield, L. (2019). An analysis of allele, genotype and phenotype frequencies, actionable pharmacogenomic (PGx) variants and phenoconversion in 5408 Australian patients genotyped for CYP2D6, CYP2C19, CYP2C9 and VKORC1 genes. J. Neural Transm. 126 (1), 5–18. doi:10.1007/s00702-018-1922-0
Mrazek, D. A., Biernacka, J. M., O’Kane, D. J., Black, J. L., Cunningham, J. M., Drews, M. S., et al. (2011). CYP2C19 variation and citalopram response. Pharmacogenet Genomics 21 (1), 1–9. doi:10.1097/fpc.0b013e328340bc5a
MuhA, B., Lanting, P., Bos, J. H. J., Sijmons, R. H., Hak, E., and Wilffert, B. (2020). Impact of drug-gene-interaction, drug-drug-interaction, and drug-drug-gene-interaction on (es)Citalopram therapy: the PharmLines initiative. J. Personalized Med. 10, 256. doi:10.3390/jpm10040256
Peters, E. J., Slager, S. L., Kraft, J. B., Jenkins, G. D., Reinalda, M. S., McGrath, P. J., et al. (2008). Pharmacokinetic genes do not influence response or tolerance to citalopram in the STAR*D sample. PLoS One 3 (4), e1872. doi:10.1371/journal.pone.0001872
Preskorn, S. H., Kane, C. P., Lobello, K., Nichols, A. I., Fayyad, R., Buckley, G., et al. (2013). Cytochrome P450 2D6 phenoconversion is common in patients being treated for depression: implications for personalized medicine. J. Clin. Psychiatry 74 (6), 614–621. doi:10.4088/JCP.12m07807
Qin, X. P., Xie, H. G., Wang, W., He, N., Huang, S. L., Xu, Z. H., et al. (1999). Effect of the gene dosage of CgammaP2C19 on diazepam metabolism in Chinese subjects. Clin. Pharmacol. Ther. 66 (6), 642–646. doi:10.1016/S0009-9236(99)90075-9
Ruaño, G., Villagra, D., Rahim, U. S., Windemuth, A., Kocherla, M., Bower, B., et al. (2008). Increased carrier prevalence of deficient CYP2C9, CYP2C19 and CYP2D6 alleles in depressed patients referred to a tertiary psychiatric hospital. Per Med. 5 (6), 579–587. doi:10.2217/17410541.5.6.579
Schredl, M. (2018). in Dreams and mental disorders BT - researching dreams: The fundamentals. Editor M. Schredl (Cham: Springer International Publishing), 123–146.
Shah, R. R., Gaedigk, A., Llerena, A., Eichelbaum, M., Stingl, J., and Smith, R. L. (2016). CYP450 genotype and pharmacogenetic association studies: a critical appraisal. Pharmacogenomics 17 (3), 259–275. doi:10.2217/pgs.15.172
Sharp, C. N., Linder, M. W., and Valdes, R. (2019). Polypharmacy: a healthcare conundrum with a pharmacogenetic solution. Crit. Rev. Clin. Lab. Sci. 57, 161–180. doi:10.1080/10408363.2019.1678568
Skryabin, V. Y., Zastrozhin, M. S., Torrado, M. V., Grishina, E. A., Ryzhikova, K. A., Shipitsyn, V. V., et al. (2020). How do CYP2C19*2 and CYP2C19*17 genetic polymorphisms affect the efficacy and safety of diazepam in patients with alcohol withdrawal syndrome? Drug Metab. Pers. Ther. 35 (1). doi:10.1515/dmpt-2019-0026
UMC Utrecht (2022b). Farmalab [internet]. Available from: https://www.umcutrecht.nl/nl/farmalab (Accessed June 2, 2022).
UMC Utrecht (2022a). Zorgprogramma Lijf & leven [internet]. Available from: https://www.umcutrecht.nl/nl/ziekenhuis/behandeling/zorgprogramma-lijf-leven (Accessed March 8, 2022).
Warrings, B., Samanski, L., Deckert, J., Unterecker, S., and Scherf-Clavel, M. (2021). Impact of Body Mass Index on serum concentrations of antidepressants and antipsychotics. Ther. Drug Monit. 43 (2), 286–291. doi:10.1097/FTD.0000000000000812
Keywords: pharmacogenetics, phenoconversion, side effects, psychiatric drugs, CYP2C19, CYP2D6
Citation: den Uil MG, Hut HW, Wagelaar KR, Abdullah-Koolmees H, Cahn W, Wilting I and Deneer VHM (2023) Pharmacogenetics and phenoconversion: the influence on side effects experienced by psychiatric patients. Front. Genet. 14:1249164. doi: 10.3389/fgene.2023.1249164
Received: 28 June 2023; Accepted: 04 August 2023;
Published: 25 August 2023.
Edited by:
Miriam Saiz-Rodríguez, Hospital Universitario de Burgos, SpainReviewed by:
Simran D. S. Maggo, Children’s Hospital of Los Angeles, United StatesKariofyllis Karamperis, University of Patras, Greece
Copyright © 2023 den Uil, Hut, Wagelaar, Abdullah-Koolmees, Cahn, Wilting and Deneer. This is an open-access article distributed under the terms of the Creative Commons Attribution License (CC BY). The use, distribution or reproduction in other forums is permitted, provided the original author(s) and the copyright owner(s) are credited and that the original publication in this journal is cited, in accordance with accepted academic practice. No use, distribution or reproduction is permitted which does not comply with these terms.
*Correspondence: Vera H. M. Deneer, v.h.m.deneer@umcutrecht.nl