- 1Third Clinical Medical College, Zhejiang Chinese Medical University, Hangzhou, China
- 2Department of Acupuncture, Third Affiliated Hospital of Zhejiang Chinese Medical University, Hangzhou, China
Background: Prior investigations have indicated associations between Knee Osteoarthritis (KOA) and certain inflammatory cytokines, such as the interleukin series and tumor necrosis factor-alpha (TNFα). To further elaborate on these findings, our investigation utilizes Mendelian randomization to explore the causal relationships between KOA and 91 inflammatory cytokines.
Methods: This two-sample Mendelian randomization utilized genetic variations associated with KOA from a large, publicly accessible Genome-Wide Association Study (GWAS), comprising 2,227 cases and 454,121 controls of European descent. The genetic data for inflammatory cytokines were obtained from a GWAS summary involving 14,824 individuals of European ancestry. Causal relationships between exposures and outcomes were primarily investigated using the inverse variance weighted method. To enhance the robustness of the research results, other methods were combined to assist, such as weighted median, weighted model and so on. Multiple sensitivity analysis, including MR-Egger, MR-PRESSO and leave one out, was also carried out. These different analytical methods are used to enhance the validity and reliability of the final results.
Results: The results of Mendelian randomization indicated that Adenosine Deaminase (ADA), Fibroblast Growth Factor 5(FGF5), and Hepatocyte growth factor (HFG) proteins are protective factors for KOA (IVWADA: OR = 0.862, 95% CI: 0.771–0.963, p = 0.008; IVWFGF5: OR = 0.850, 95% CI: 0.764–0.946, p = 0.003; IVWHFG: OR = 0.798, 95% CI: 0.642–0.991, p = 0.042), while Tumor necrosis factor (TNFα), Colony-stimulating factor 1(CSF1), and Tumor necrosis factor ligand superfamily member 12(TWEAK) proteins are risk factors for KOA. (IVWTNFα: OR = 1.319, 95% CI: 1.067–1.631, p = 0.011; IVWCSF1: OR = 1.389, 95% CI: 1.125–1.714, p = 0.002; IVWTWEAK: OR = 1.206, 95% CI: 1.016–1.431, p = 0.032).
Conclusion: The six proteins identified in this study demonstrate a close association with the onset of KOA, offering valuable insights for future therapeutic interventions. These findings contribute to the growing understanding of KOA at the microscopic protein level, paving the way for potential targeted therapeutic approaches.
1 Introduction
Knee osteoarthritis (KOA) is a common degenerative joint disease characterized by subchondral bone remodeling (1), meniscal degeneration (2), and inflammation of the infrapatellar fat pad and synovium (3), significantly impacting people’s health and quality of life. Studies indicate that over 80% of KOA patients are over 60 years old, and the condition significantly contributes to annual hospital admissions in developed countries (4). The onset of KOA is associated with factors such as aging (5, 6), obesity (7), diabetes (8), joint trauma (6, 9), and overuse, which lead to cartilage damage, inflammation, and a loss of the knee joint’s homeostasis. Symptoms typically include knee pain (10), swelling (11), and crepitus (11). The development of this pathological condition is closely tied to the regulation of intrinsic mechanisms, and only by understanding these mechanisms can we precisely and effectively halt the further progression of KOA.
In recent years, the research in the field of KOA has been increasingly in-depth. Current studies focus on cytokines, particularly those crucial to inflammation (12, 13), as well as the mechanisms of gene expression (14, 15) and signal transduction pathways (16–18). The TNF family and MMP enzyme series have been frequently reported to be associated with KOA (13, 19–22). While there is no clear consensus on the pathogenesis of KOA, there is widespread support for inflammatory changes playing a key role in its development. KOA is thought to result from an imbalance between anti-inflammatory and pro-inflammatory factors (6, 23–25). Therefore, this study employs Mendelian randomization analysis to comprehensively explore the causal relationships between various inflammatory factors and KOA, aiming to provide a reference for the future treatment of KOA.
Mendelian randomization (MR) serves as a potent analytical tool employed to infer the causal impact of an exposure on an outcome by leveraging genetic variations within non-experimental datasets (26). The random allocation of alleles during meiosis equips MR with the unique capability to address both conventional confounding variables and reverse causation, thereby furnishing more robust evidence for causal inference (27). The use of a two-sample MR analysis enhances researchers’ ability to scrutinize the relationships between instruments and exposures, as well as instruments and outcomes, across distinct population samples, thereby amplifying the versatility and efficacy of the analysis (28). In this study, we meticulously extracted authentic genetic variants from the summary data of a genome-wide association study (GWAS) comprising 91 inflammatory cytokines, with the aim of exploring their intricate correlations with KOA.
2 Materials and methods
2.1 MR assumptions
Three fundamental assumptions underpin MR analyses, specifically relevance, independence, and exclusion restriction (29). The foundational premise is that the selected genetic variants demonstrate an association with the risk factor (relevance), remain unaffected by any confounding factors in the risk factor–outcome association (independence), and exert no influence on the outcome through pathways other than the targeted risk factor (exclusion restriction). In this study, two GWAS were employed to identify genetically significant single nucleotide polymorphisms (SNPs) associated with 91 inflammatory cytokines and KOA. An overview of the study design is illustrated in Figure 1.
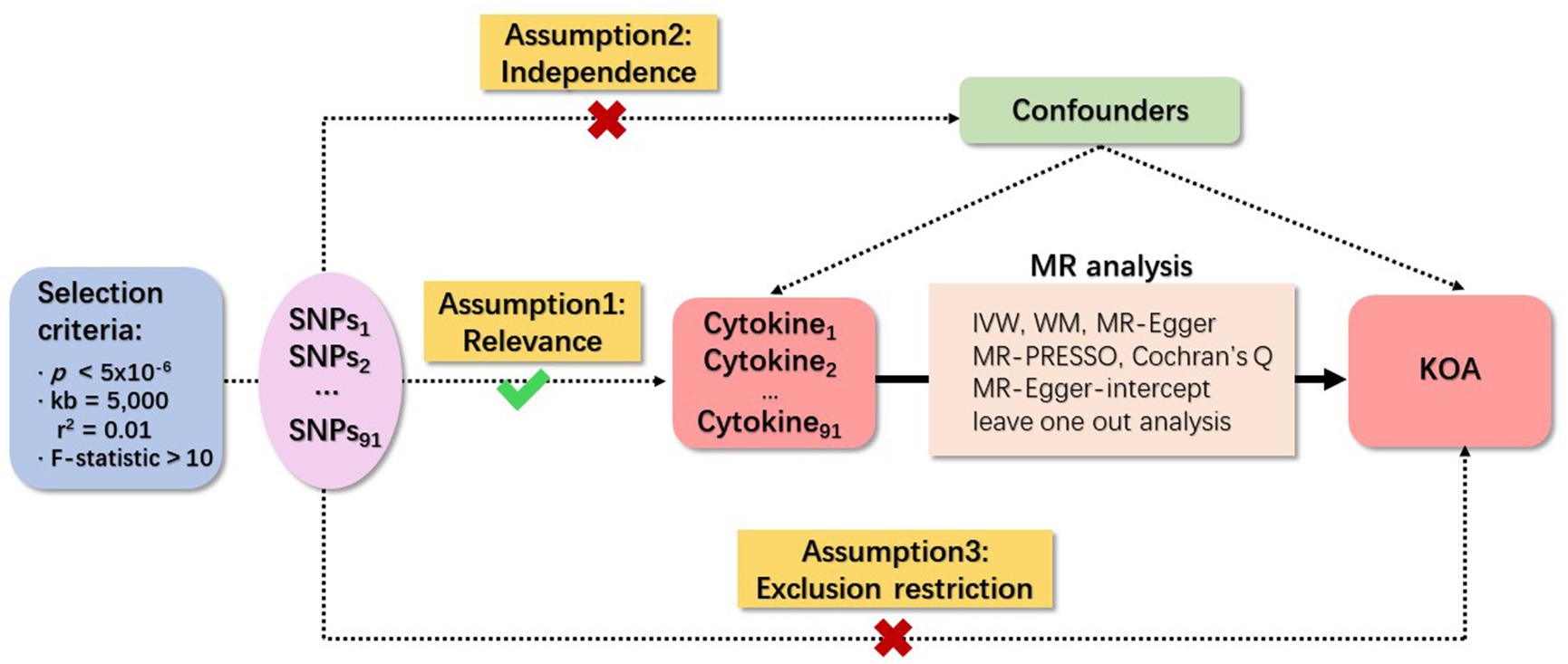
Figure 1. Schematic representation of study design in Mendelian randomization (MR) analysis. Significant instrumental variables were chosen for 91 inflammatory cytokines and KOA, facilitating an examination of the potential causal relationships between each protein and the occurrence of KOA. This flowchart illustrates the three basic assumptions of MR analysis, namely, dependence, independence, and exclusion restrictions.
2.2 Instrumental variable selection
Initially, a genome-wide significance threshold of p < 5 × 10−6 was established to identify strongly associated SNPs with inflammatory cytokines and KOA. Subsequently, to mitigate the impact of linkage disequilibrium, we performed clumping on these SNPs (kb = 5,000, r2 = 0.01). Palindromic SNPs were excluded from the analysis due to uncertainty regarding their alignment in the same direction for exposure and outcome in the GWAS of systemic inflammatory regulators. In the third step, the R2 value of each SNP was utilized to calculate the proportion of variance in exposure, and the F-statistic was employed to estimate the instrument strength, thereby preventing potential biases associated with weak instruments.
2.3 Data source
The dataset encompassing GWAS for 91 circulating inflammatory cytokines was obtained from the investigation conducted by Zhao et al. (30). In their research publication, the authors conducted protein quantitative trait loci (pQTL) mapping in a cohort of 14,824 individuals of European ancestry. The primary objective was to identify genetic variants linked to circulating inflammatory proteins by summarizing pQTL information within ±1 megabase surrounding 91 candidate genes associated with these proteins. Additionally, the GWAS summary statistics for KOA are accessible for download from the study by Jiang et al. (31). This dataset includes 2,227 cases of European ancestry with KOA and 454,121 controls of European ancestry. Importantly, there is no overlap in the selection of populations between the exposure group and the outcome group.
2.4 Statistical analysis
The Inverse Variance-Weighted analysis (IVW), a classical method in MR, mandates strict adherence to the fundamental assumptions of instrumental variables for all SNPs. The IVW method yields consistent estimates of causal effects with robust testing power when these instrumental variable assumptions are met. Therefore, the results of the IVW method were taken as the main results in our study. Despite its widespread use in practical applications, the IVW method’s assumption that all genetic variations serve as valid instrumental variables may face practical challenges (32). Consequently, this study incorporated supplementary MR methods based on distinct assumptions. The Weighted Median (WM) requires that 50% or more of SNPs function as valid instrumental variables to ensure the stability of effect values. The core idea behind WM is to utilize the median of Wald estimates from all SNPs as the ultimate MR effect value (33). This effectively mitigates the impact of outlier SNPs on results, enhancing result robustness. In situations where significant heterogeneity is observed in IVW estimation results, median-based methods can offer a valuable supplementary approach. In contrast to IVW, the MR-Egger method modifies the conventional requirement for the regression line to pass through the origin. This is achieved by introducing an intercept term in weighted linear regression to examine and correct overall horizontal pleiotropy (34). MR-Egger regression relaxes the exclusivity assumption of instrumental variables in MR methods. MR-Egger can generate consistent estimates of causal effects only when the direct effects of SNPs on outcomes are independent of the associations between SNPs and exposures (34). However, utilizing the same SNPs for IVW analysis when the intercept term is non-zero or statistically insignificant may introduce bias into results. Compared to other methods, MR-Egger exhibits lower testing power, potentially resulting in wider confidence intervals. Moreover, the method’s assumptions require that SNPs exhibit the same horizontal pleiotropy, a condition challenging to fully satisfy in practical applications.
Sensitivity analysis encompasses heterogeneity testing and pleiotropy testing, primarily investigated from three perspectives. (a) Heterogeneity testing aims to evaluate disparities among various instrumental variables (IVs). The Q-test method is applied to assess heterogeneity in both the MR-IVW model and MR-Egger model, conducting tests among instrumental variables using distinct statistical analysis methods. A p-value exceeding 0.05 indicates the absence of heterogeneity. In cases where heterogeneity is identified among instrumental variables, a random effects model (REM) based on the IVW method is employed to evaluate the impact of exposure on the outcome. (b) Pleiotropy testing primarily scrutinizes whether multiple IVs display horizontal pleiotropy, i.e., whether SNPs functioning as IVs are associated with other unrelated variables. The MR-Egger method is commonly utilized for assessing horizontal pleiotropy, where the intercept term indicates its presence if significantly different from zero (p < 0.05) (34). Additionally, the MR-PRESSO is utilized to identify and eliminate outlier SNPs, correcting horizontal pleiotropy and providing more robust causal estimates between exposure and outcome (35). (c) Leave-one-out sensitivity testing involves systematically removing each SNP locus using the leave-one-out method. MR analysis is then conducted using the remaining SNP loci to assess whether a specific SNP locus introduces bias into the results. Analyses were implemented by the package TwoSampleMR (version 0.4.25) and MR-PRESSO (version 1.0) in R (version 4.3.1).
3 Result
3.1 The selection results of instrumental variables
In adherence to the specified criteria for instrumental variable selection, we conducted a meticulous screening of the dataset encompassing diverse proteins to identify instrumental variables meeting the necessary conditions. Here, we present the outcome data solely for positively associated proteins. Taking the ADA protein as an illustrative case, we identified 21 SNPs from the GWAS dataset that exhibited close associations (p < 5 × 10−6), demonstrated no linkage disequilibrium, and overlapped with the dataset for KOA. We excluded one palindrome SNP (rs2620728). Using the PhenoScanner database,1 we searched for secondary phenotypes of the selected SNPs and excluded the potential confounding SNP (rs1608554). The F-statistic values for the remaining SNPs ranged from 20.91 to 1180.03, confirming the absence of weak instrumental variables in the MR analysis. Simultaneously, MR-PRESSO analysis identified no outliers. Ultimately, 19 SNPs were selected as instrumental variables for evaluating the association between ADA protein and KOA. Comprehensive information regarding SNPs for other positively associated proteins is presented in Table 1.
3.2 Outcomes of Mendelian randomization
The IVW method unveiled a potential inverse association between genetically determined elevated levels of ADA protein (corresponding to a one-standard-deviation increase) and a 13.8% reduction in the odds of developing KOA (OR = 0.862, 95% CI: 0.771–0.963, p = 0.008). This finding was corroborated by results from the weighted median method (OR = 0.866, 95% CI: 0.758–0.991, p = 0.037). Although MR-Egger analysis did not identify a statistically significant association, it indicated a consistent trend (OR = 0.875, 95% CI: 0.759–1.009, p = 0.083). Similar causal associations were observed for FGF5 protein and KOA (IVW: OR = 0.850, 95% CI: 0.764–0.946, p = 0.003; weighted median: OR = 0.814, 95% CI: 0.709–0.934, p = 0.003). The HGF protein was identified as a potential protective factor for KOA (IVW: OR = 0.798, 95% CI: 0.642–0.991, p = 0.042; weighted median: OR = 0.786, 95% CI: 0.576–1.072, p = 0.129).
In contrast to the aforementioned proteins, our study revealed a significant increase in the risk of KOA associated with TNF (IVW: OR = 1.319, 95% CI: 1.067–1.631, p = 0.011; weighted median: OR = 1.356, 95% CI: 0.995–1.848, p = 0.054), CSF1 (IVW: OR = 1.389, 95% CI: 1.125–1.714, p = 0.002; weighted median: OR = 1.544, 95% CI: 1.135–2.099, p = 0.006), and TWEAK (IVW: OR = 1.206, 95% CI: 1.016–1.431, p = 0.032; weighted median: OR = 1.267, 95% CI: 0.997–1.610, p = 0.053). These proteins may serve as potential risk factors for the development of KOA. Results from the MR analysis of all inflammatory factors are depicted in Figure 2. The scatter plots of Mendelian randomization analyses for the aforementioned six proteins are exhibited in Figure 3.
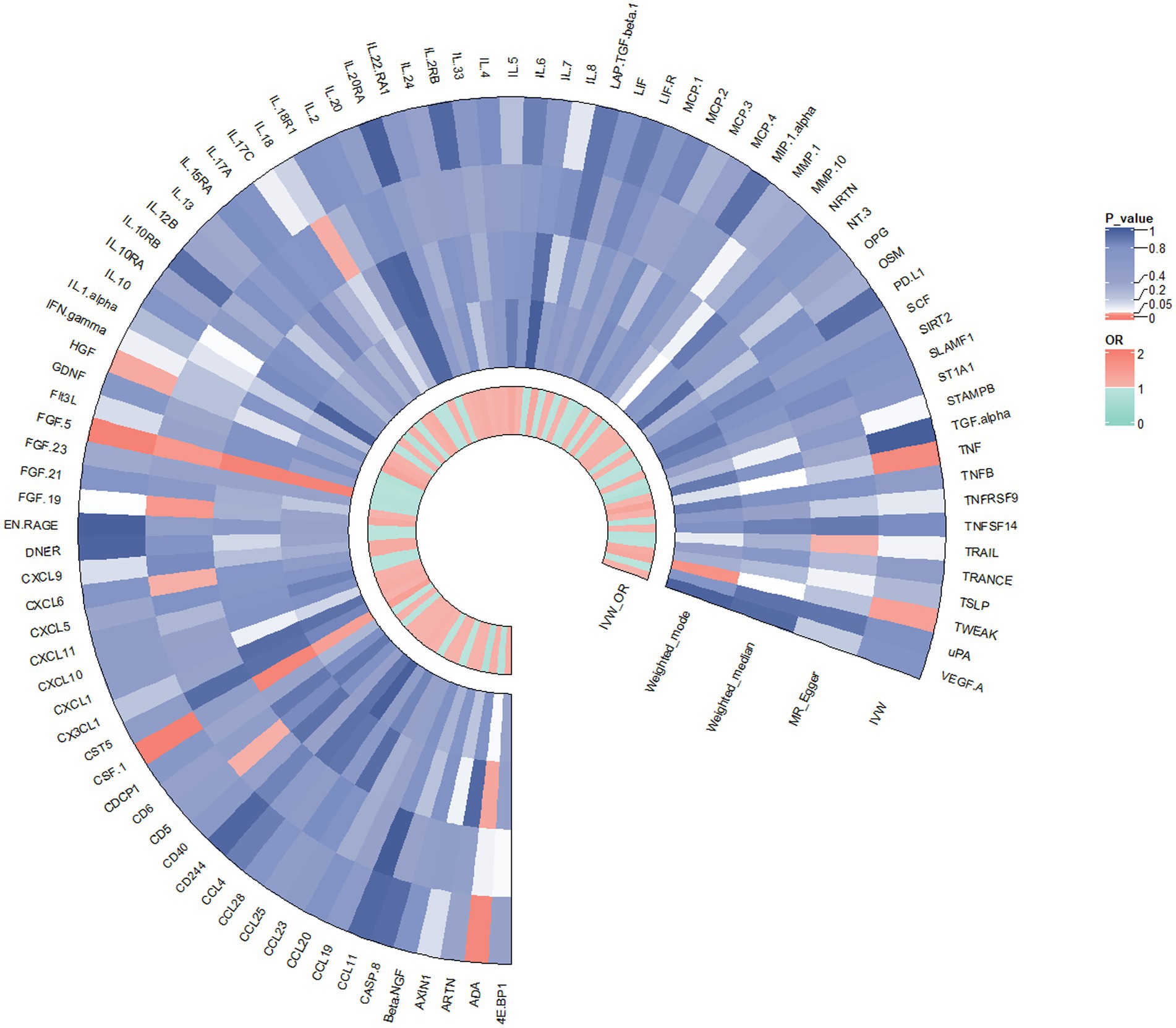
Figure 2. Result of the two-sample MR analysis of 91 and KOA: ADA (IVW: OR = 0.862, 95% CI: 0.771–0.963, p = 0.008; WM: OR = 0.866, 95% CI: 0.758–0.991, p = 0.037). FGF5 (IVW: OR = 0.850, 95% CI: 0.764–0.946, p = 0.003; WM: OR = 0.814, 95% CI: 0.709–0.934, p = 0.003). The HGF (IVW: OR = 0.798, 95% CI: 0.642–0.991, p = 0.042; WM: OR = 0.786, 95% CI: 0.576–1.072, p = 0.129).TNFα (IVW: OR = 1.319, 95% CI: 1.067–1.631, p = 0.011; WM: OR = 1.356, 95% CI: 0.995–1.848, p = 0.054), CSF1 (IVW: OR = 1.389, 95% CI: 1.125–1.714, p = 0.002; WM: OR = 1.544, 95% CI: 1.135–2.099, p = 0.006), TWEAK (IVW: OR = 1.206, 95% CI: 1.016–1.431, p = 0.032; WM: OR = 1.267, 95% CI: 0.997–1.610, p = 0.053).CD5 (MR Egger: OR = 0.621, 95% CI: 0.400–0.964,p = 0.046), CXCL6 (MR Egger: OR = 1.452, 95% CI: 1.205–1.749, p = 0.046), FGF19 (MR Egger:OR = 1.780, 95% CI: 1.126–2.815, p = 0.023), IL18 (MR Egger:OR = 0.878, 95% CI: 0.777–0.991,p = 0.044),TRAIL (MR Egger:OR = 0.808, 95% CI: 0.661–0.988, p = 0.049).
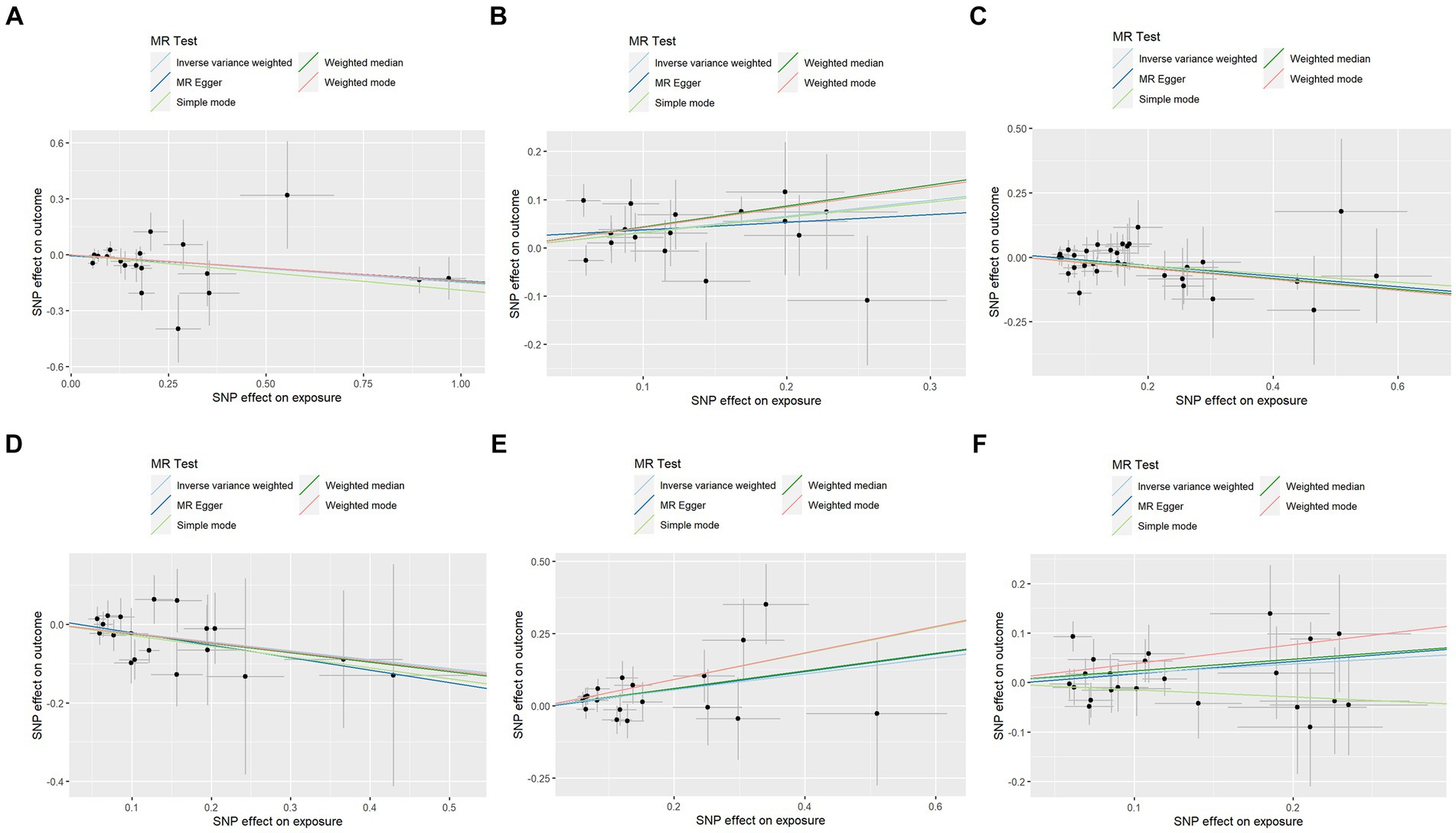
Figure 3. Scatter plots of Mendelian randomization analyses between KOA and 91 inflammatory cytokines. Individual inverse variance (IV) associations with KOA risk are displayed versus individual IV associations with cytokines in black dots. The 95%CI of the odds ratio for each IV is shown by the vertical and horizontal lines. The slope of the lines represents the estimated causal effect of the MR methods. (A–F): ADA, CSF1, FGF5, HGF, TNFα, and TWEAK.
3.3 Quality assurance
To assess the robustness of our findings, sensitivity analyses were performed using MR-Egger regression and Cochran’s Q test. The results demonstrate the absence of heterogeneity and pleiotropy in this study, as presented in Table 2. Furthermore, leave-one-out analysis indicates the consistent nature of the results even after systematically excluding individual SNPs, as illustrated in the figure. This consistency aligns with the outcomes of the earlier MR-PRESSO analysis. Collectively, these methodologies offer compelling evidence supporting the robustness of the study results. The diagram of leave-one-out analysis method for those six aforementioned proteins is illustrated in Figure 4.
4 Discussion
Recent studies have demonstrated a close association between the occurrence and progression of KOA and inflammatory cytokines (23). Therefore, in this two-sample MR analysis, we screened 91 inflammatory factors for potential causal associations with KOA, aiming to comprehensively identify factors closely related to the disease. The results indicate that ADA, FGF5, and HGF may serve as potential protective factors against KOA, while TNF, CSF1, and TWEAK may be considered as risk factors for KOA. Following this, we will endeavor to elucidate the potential mechanisms through which each factor may operate within the context of KOA.
Studies have conducted proteomic analysis on synovial fluid proteins in patients with psoriatic arthritis (PsA) and observed significant changes in the expression of ADA protein levels in synovial fluid with the progression of arthritis, suggesting a potential involvement of ADA protein in the immune processes of arthritis (36). A multi-omics study of serum ADA activity in rheumatoid arthritis patients found elevated levels of TNFα, IFN γ, and IL-10 in patients with hyperactive ADA, exacerbating inflammation, promoting osteoclast differentiation, and negatively impacting bone metabolism (37, 38). However, there is also research supporting the viewpoint that ADA protein acts as a protective factor in KOA. ADA is a key enzyme in purine metabolism, converting adenosine to inosine through its deaminase activity (39). In a mouse model of osteoarthritis, Mistry et al. observed that MC615 chondrocytes treated with adenosine exhibited significant apoptosis (40), suggesting cytotoxicity due to excessive intracellular adenosine levels (41). This apoptotic phenomenon was similarly observed in equine chondrocytes (42). Our study results align with the latter perspective, indicating ADA as a protective factor in KOA. However, the underlying mechanisms require further in-depth investigation for clarification.
Macrophage Colony-Stimulating Factor (M-CSF), also known as CSF1, is a member of the hematopoietic colony-stimulating factor (CSF) family (43). Under normal physiological conditions, M-CSF binds to the CSF1R receptor on the surface of macrophages, facilitating the differentiation of monocytes into mature macrophages and playing a crucial role in immune modulation (43) and tissue repair (44). Basic research has uncovered that synovial fibroblasts in patients with rheumatoid arthritis and osteoarthritis actively produce M-CSF, leading to the differentiation of synovial macrophages into osteoclasts (45). Research has found that TNF-α acts through M-CSF expressed by bone marrow stromal cells and stimulates the expression of the M-CSF gene (46, 47). In animal models, the exacerbation of collagen-induced arthritis (CIA) severity is observed upon the exogenous administration of CSF1. Conversely, the knockout or neutralization of CSF1 demonstrates a distinct therapeutic effect (48–50). Notably, Giordano et al. reported a significant upregulation of CSF1 expression in the serum of KOA patients (51). Linear regression analysis further revealed a positive correlation between CSF1 levels and pain intensity (52). These findings not only position CSF1 as a contributory risk factor in the pathogenesis of KOA but also suggest that reducing CSF1 levels holds promise as a potential therapeutic target for this debilitating condition.
Research indicates that synovial-like fibroblast cells (FLS) from osteoarthritis patients secrete more growth factors, including Fibroblast Growth Factor 5 (FGF5), upon exposure to pro-inflammatory stimuli (53). Clase et al. propose that FGF5, as a mitogenic stimulus for mesenchymal fibroblasts, promotes the proliferation of these fibroblasts, contributing to the formation of connective tissues such as the synovium (54). The findings of our study suggest that FGF5 may serve as a potential protective factor in KOA. Therefore, we hypothesize that FGF5 might delay the progression of osteoarthritis by participating in the repair of the synovium, and further investigations are warranted to validate this assumption.
Hepatocyte growth factor (HGF) is a potent mitogen that plays a key role in the growth and differentiation of various tissues (55, 56). Its anti-inflammatory activity has been reported in several disease animal models and across multiple organ systems (57). Research indicates that HGF significantly induces FLS to secrete chemokine MCP-1, promoting the migration of monocytes and macrophages to sites of inflammation or injury (58). These cells then secrete TGF-β1 and BMP-2, contributing to the formation of osteophytes (58). Adding HGF to in vitro cultures of primary chondrocytes from rabbits and rats leads to a substantial increase in proteoglycan synthesis and cell proliferation rates, demonstrating its role in supporting articular cartilage repair (59). Mohammad A. J. and colleagues (60) found that HGF works in conjunction with TGF-β and IDO, immune-regulatory enzymes, to enhance the immunosuppressive properties of human mesenchymal stem cells (MSCs). This results in a reduction in the infiltration of inflammatory cells and supports tissue repair and regeneration. This is consistent with the findings of our study, suggesting that HGF is a protective factor in KOA. HGF may potentially delay disease progression by participating in the regulation and repair of articular cartilage homeostasis.
Tumor Necrosis Factor-α (TNFα) is a common inflammatory factor and a crucial participant in osteoarthritis. Terkeltaub R’s team (61) reported that their studies on primary chondrocytes from humans and mice revealed that TNFα can induce the production of matrix metalloproteinases (MMPs), prostaglandin E2 (PGE2), and nitric oxide (NO), leading to a decrease in chondrocytes and joint cartilage destruction. The infrapatellar fat pad can also produce TNF-α, and this autocrine effect plays an important role in the pathological changes seen in KOA (62). Studies have shown a significant increase in TNF-α expression in tissues from OA patients compared to normal subjects, and elevated levels of TNF-α in joint fluid may accelerate the progression of OA (12). A meta-analysis on TNF-α gene polymorphism and the risk of KOA indicated a close association between TNF-α expression levels and genetic polymorphism. Patients carrying alleles such as TNF-α-G308A exhibited higher TNF-α levels and a heightened risk of developing osteoarthritis (63). Clinical research indicates that targeting TNFα with adalimumab (64) and natural extracts such as curcumin (65) and Aflapin (66) can provide safe and effective relief from pain and other symptoms associated with KOA. This aligns with the results of our study: TNFα is a risk factor for KOA. Strategies to reduce TNF expression levels may be a potential avenue for the treatment of this condition.
Tumor necrosis factor ligand superfamily member 12, also known as tumor necrosis factor-like weak inducer of apoptosis (TWEAK), is associated with inflammation and plays a role in immune regulation, cell apoptosis, and tissue recovery and reconstruction before the onset of inflammation (67). Research has revealed that upon binding to its receptor Fn14, TWEAK induces the generation of matrix metalloproteinase-1 (MMP1) (68, 69). While MMP-1 maintains low expression in normal cells and promotes the regeneration of healthy cartilage, pathological conditions can lead to its overexpression. This excess expression results in the degradation of type I, II, and III fibers in the extracellular matrix, facilitating cartilage destruction and exacerbating the progression of OA (70). Additionally, TWEAK is believed to inhibit cartilage and bone formation, further intensifying the likelihood of cartilage degradation (71). Hwang et al. assessed TWEAK levels in joint fluid from patients with different stages of OA (72). They highlighted that high levels of TWEAK are linked to pro-inflammatory responses and accelerated cartilage destruction, particularly during the early stages of the disease. This interaction may be facilitated by the relationship between TWEAK and MMP1. Therefore, as a relevant risk factor for KOA, the expression levels of TWEAK can serve as an early screening marker for this condition.
In this investigation, we utilized Mendelian randomization to evaluate the causal association between KOA and 91 inflammatory cytokines. Regarding the interactions among the few factors mentioned in the article, we conducted further analysis to explore potential mediating effects. The results continue to support our original conclusions. (Refer to the Supplementary material for details) However, several limitations need consideration. Firstly, when selecting an SNP filtering threshold, a stringent threshold may lead to an insufficient number of SNPs available for analysis, resulting in inaccurate estimates of causal effects. Therefore, we opted for a more lenient threshold, which increased the number of SNPs included in the analysis within a certain range. However, this may introduce weak instrumental variables and increase interference from confounding factors and horizontal pleiotropy. To address this, we filtered out weak instrumental variables based on the F-value calculation and conducted sensitivity tests to exclude horizontal pleiotropy. We adopted a relatively strict confounder removal standard, though there may still be omissions, especially when SNPs are associated with unreported confounding factors. Secondly, our survey data originated from two extensive GWAS, and the absence of specific demographic information and clinical records for study patients hindered subgroup analysis. Thirdly, caution is warranted regarding potential racial bias, as the subjects exclusively belonged to European descent, limiting the generalizability of results to other ethnicities. Further studies are crucial to validate our findings and explore their applicability to clinical diagnostic procedures and treatment options.
Data availability statement
The original contributions presented in the study are included in the article/Supplementary materials, further inquiries can be directed to the corresponding authors.
Ethics statement
Ethical approval was not required for the study involving humans in accordance with the local legislation and institutional requirements. Written informed consent to participate in this study was not required from the participants or the participants' legal guardians/next of kin in accordance with the national legislation and the institutional requirements.
Author contributions
LX: Writing – original draft, Writing – review & editing. JM: Software, Writing – review & editing. QY: Writing – review & editing. KZ: Writing – review & editing. XW: Writing – review & editing. CZ: Funding acquisition, Writing – review & editing, Writing – original draft. XL: Funding acquisition, Writing – review & editing, Writing – original draft.
Funding
The author(s) declare that financial support was received for the research, authorship, and/or publication of this article. This study was funded by Lin Xianming’s Famous Chinese Medicine Doctor Studio Project (Z.W.F [2018]69).
Acknowledgments
The authors extend sincere gratitude to the investigators who generously provided the GWAS summary statistics utilized in this study.
Conflict of interest
The authors declare that the research was conducted in the absence of any commercial or financial relationships that could be construed as a potential conflict of interest.
Publisher’s note
All claims expressed in this article are solely those of the authors and do not necessarily represent those of their affiliated organizations, or those of the publisher, the editors and the reviewers. Any product that may be evaluated in this article, or claim that may be made by its manufacturer, is not guaranteed or endorsed by the publisher.
Supplementary material
The Supplementary material for this article can be found online at: https://www.frontiersin.org/articles/10.3389/fmed.2024.1382836/full#supplementary-material
Footnotes
References
1. Zhu, X, Chan, YT, Yung, PSH, Tuan, RS, and Jiang, Y. Subchondral bone remodeling: a therapeutic target for osteoarthritis. Front. Cell Dev. Biol. (2020) 8:607764. doi: 10.3389/fcell.2020.607764
2. Battistelli, M, Favero, M, Burini, D, Trisolino, G, Dallari, D, De Franceschi, L, et al. Morphological and ultrastructural analysis of normal, injured and osteoarthritic human knee menisci. Eur. J. Histochem. (2019) 63:2998. doi: 10.4081/ejh.2019.2998
3. Favero, M, El-Hadi, H, Belluzzi, E, Granzotto, M, Porzionato, A, Sarasin, G, et al. Infrapatellar fat pad features in osteoarthritis: a histopathological and molecular study. Rheumatology (Oxford). (2017) 56:1784–93. doi: 10.1093/rheumatology/kex287
4. Ding, Y, Wang, L, Zhao, Q, Wu, Z, and Kong, L. MicroRNA-93 inhibits chondrocyte apoptosis and inflammation in osteoarthritis by targeting the TLR4/NF-κB signaling pathway. Int J Mol Med. (2019) 43:779–90. doi: 10.3892/ijmm.2018.4033
5. Du, X, Liu, ZY, Tao, XX, Mei, YL, Zhou, DQ, Cheng, K, et al. Research Progress on the pathogenesis of knee osteoarthritis. Orthop Surg. (2023) 15:2213–24. doi: 10.1111/os.13809
6. Geng, R, Li, J, Yu, C, Zhang, C, Chen, F, Chen, J, et al. Knee osteoarthritis: current status and research progress in treatment (review). Exp Ther Med. (2023) 26:481. doi: 10.3892/etm.2023.12180
7. Georgiev, T, and Angelov, AK. Modifiable risk factors in knee osteoarthritis: treatment implications. Rheumatol Int. (2019) 39:1145–57. doi: 10.1007/s00296-019-04290-z
8. Dubey, NK, Ningrum, DNA, Dubey, R, Deng, YH, Li, YC, Wang, PD, et al. Correlation between diabetes mellitus and knee osteoarthritis: a dry-to-wet lab approach. Int J Mol Sci. (2018) 19:3021. doi: 10.3390/ijms19103021
9. Richmond, SA, Fukuchi, RK, Ezzat, A, Schneider, K, Schneider, G, and Emery, CA. Are joint injury, sport activity, physical activity, obesity, or occupational activities predictors for osteoarthritis? A systematic review. J Orthop Sports Phys Ther. (2013) 43:515–b19. doi: 10.2519/jospt.2013.4796
10. Peprah, K, and Argáez, C. CADTH rapid response reports. Physical activity for chronic osteoarthritic knee pain: A review of clinical effectiveness. Ottawa (ON): Canadian Agency for Drugs and Technologies in Health (2020).
11. Liew, JW, King, LK, Mahmoudian, A, Wang, Q, Atkinson, HF, Flynn, DB, et al. A scoping review of how early-stage knee osteoarthritis has been defined. Osteoarthr Cartil. (2023) 31:1234–41. doi: 10.1016/j.joca.2023.04.015
12. Larsson, S, Englund, M, Struglics, A, and Lohmander, LS. Interleukin-6 and tumor necrosis factor alpha in synovial fluid are associated with progression of radiographic knee osteoarthritis in subjects with previous meniscectomy. Osteoarthr Cartil. (2015) 23:1906–14. doi: 10.1016/j.joca.2015.05.035
13. Kim, JR, Yoo, JJ, and Kim, HA. Therapeutics in osteoarthritis based on an understanding of its molecular pathogenesis. Int J Mol Sci. (2018) 19:674. doi: 10.3390/ijms19030674
14. Uchida, K, Takano, S, Takata, K, Mukai, M, Koyama, T, Ohashi, Y, et al. Differential synovial CGRP/RAMP1 expression in men and women with knee osteoarthritis. Cureus. (2021) 13:e15483. doi: 10.7759/cureus.15483
15. Mei, L, Zhang, Z, Chen, R, Liu, Z, Ren, X, and Li, Z. Identification of candidate genes and chemicals associated with osteoarthritis by transcriptome-wide association study and chemical-gene interaction analysis. Arthritis Res Ther. (2023) 25:179. doi: 10.1186/s13075-023-03164-x
16. Kwon, H, Paschos, NK, Hu, JC, and Athanasiou, K. Articular cartilage tissue engineering: the role of signaling molecules. Cell Mol Life Sci. (2016) 73:1173–94. doi: 10.1007/s00018-015-2115-8
17. Belluzzi, E, Stocco, E, Pozzuoli, A, Granzotto, M, Porzionato, A, Vettor, R, et al. Contribution of infrapatellar fat pad and synovial membrane to knee osteoarthritis pain. Biomed Res Int. (2019) 2019:1–18. doi: 10.1155/2019/6390182
18. Han, X, Lin, D, Huang, W, Li, D, Li, N, and Xie, X. Mechanism of NLRP3 inflammasome intervention for synovitis in knee osteoarthritis: a review of TCM intervention. Front Genet. (2023) 14:1159167. doi: 10.3389/fgene.2023.1159167
19. Bondeson, J, Wainwright, SD, Lauder, S, Amos, N, and Hughes, CE. The role of synovial macrophages and macrophage-produced cytokines in driving aggrecanases, matrix metalloproteinases, and other destructive and inflammatory responses in osteoarthritis. Arthritis Res Ther. (2006) 8:R187. doi: 10.1186/ar2099
20. Sanchez-Lopez, E, Coras, R, Torres, A, Lane, NE, and Guma, M. Synovial inflammation in osteoarthritis progression. Nat Rev Rheumatol. (2022) 18:258–75. doi: 10.1038/s41584-022-00749-9
21. Bondeson, J, Blom, AB, Wainwright, S, Hughes, C, Caterson, B, and van den Berg, WB. The role of synovial macrophages and macrophage-produced mediators in driving inflammatory and destructive responses in osteoarthritis. Arthritis Rheum. (2010) 62:647–57. doi: 10.1002/art.27290
22. Griffin, TM, and Scanzello, CR. Innate inflammation and synovial macrophages in osteoarthritis pathophysiology. Clin Exp Rheumatol. (2019) 37 Suppl 120:57–63.
23. Livshits, G, Zhai, G, Hart, DJ, Kato, BS, Wang, H, Williams, FM, et al. Interleukin-6 is a significant predictor of radiographic knee osteoarthritis: the Chingford study. Arthritis Rheum. (2009) 60:2037–45. doi: 10.1002/art.24598
24. Rankothgedera, S, Atukorala, I, Fernando, C, Munidasa, D, Wijayaratne, L, and Udagama, P. A potential diagnostic serum immunological marker panel to differentiate between primary and secondary knee osteoarthritis. PLoS One. (2021) 16:e0257507. doi: 10.1371/journal.pone.0257507
25. Rai, V, Dilisio, MF, Samadi, F, and Agrawal, DK. Counteractive effects of IL-33 and IL-37 on inflammation in osteoarthritis. Int J Environ Res Public Health. (2022) 19:5690. doi: 10.3390/ijerph19095690
26. Wootton, RE, Richmond, RC, Stuijfzand, BG, Lawn, RB, Sallis, HM, Taylor, GMJ, et al. Evidence for causal effects of lifetime smoking on risk for depression and schizophrenia: a Mendelian randomisation study. Psychol Med. (2020) 50:2435–43. doi: 10.1017/S0033291719002678
27. Burgess, S, Scott, RA, Timpson, NJ, Davey Smith, G, and Thompson, SG. Using published data in Mendelian randomization: a blueprint for efficient identification of causal risk factors. Eur J Epidemiol. (2015) 30:543–52. doi: 10.1007/s10654-015-0011-z
28. Hartwig, FP, Davies, NM, Hemani, G, and Davey, SG. Two-sample Mendelian randomization: avoiding the downsides of a powerful, widely applicable but potentially fallible technique. Int J Epidemiol. (2016) 45:1717–26. doi: 10.1093/ije/dyx028
29. Emdin, CA, Khera, AV, and Kathiresan, S. Mendelian Randomization. JAMA. (2017) 318:1925–6. doi: 10.1001/jama.2017.17219
30. Zhao, JH, Stacey, D, Eriksson, N, Macdonald-Dunlop, E, Hedman, ÅK, Kalnapenkis, A, et al. Genetics of circulating inflammatory proteins identifies drivers of immune-mediated disease risk and therapeutic targets. Nat Immunol. (2023) 24:1540–51. doi: 10.1038/s41590-023-01588-w
31. Jiang, L, Zheng, Z, Fang, H, and Yang, J. A generalized linear mixed model association tool for biobank-scale data. Nat Genet. (2021) 53:1616–21. doi: 10.1038/s41588-021-00954-4
32. Burgess, S, Butterworth, A, and Thompson, SG. Mendelian randomization analysis with multiple genetic variants using summarized data. Genet Epidemiol. (2013) 37:658–65. doi: 10.1002/gepi.21758
33. Bowden, J, Davey Smith, G, Haycock, PC, and Burgess, S. Consistent estimation in Mendelian randomization with some invalid instruments using a weighted median estimator. Genet Epidemiol. (2016) 40:304–14. doi: 10.1002/gepi.21965
34. Bowden, J, Davey Smith, G, and Burgess, S. Mendelian randomization with invalid instruments: effect estimation and bias detection through egger regression. Int J Epidemiol. (2015) 44:512–25. doi: 10.1093/ije/dyv080
35. Verbanck, M, Chen, CY, Neale, B, and Do, R. Detection of widespread horizontal pleiotropy in causal relationships inferred from Mendelian randomization between complex traits and diseases. Nat Genet. (2018) 50:693–8. doi: 10.1038/s41588-018-0099-7
36. Barbarroja, N, López-Montilla, MD, Cuesta-López, L, Pérez-Sánchez, C, Ruiz-Ponce, M, López-Medina, C, et al. Characterization of the inflammatory proteome of synovial fluid from patients with psoriatic arthritis: potential treatment targets. Front Immunol. (2023) 14:1133435. doi: 10.3389/fimmu.2023.1133435
37. Yang, K, Li, J, and Tao, L. Purine metabolism in the development of osteoporosis. Biomed. Pharmacother. (2022) 155:113784. doi: 10.1016/j.biopha.2022.113784
38. Bhagavatham, SKS, Khanchandani, P, Kannan, V, Potikuri, D, Sridharan, D, Pulukool, SK, et al. Adenosine deaminase modulates metabolic remodeling and orchestrates joint destruction in rheumatoid arthritis. Sci Rep. (2021) 11:15129. doi: 10.1038/s41598-021-94607-5
39. Cristalli, G, Costanzi, S, Lambertucci, C, Lupidi, G, Vittori, S, Volpini, R, et al. Adenosine deaminase: functional implications and different classes of inhibitors. Med Res Rev. (2001) 21:105–28. doi: 10.1002/1098-1128(200103)21:2<105::AID-MED1002>3.0.CO;2-U
40. Mistry, D, Chambers, MG, and Mason, RM. The role of adenosine in chondrocyte death in murine osteoarthritis and in a murine chondrocyte cell line. Osteoarthr Cartil. (2006) 14:486–95. doi: 10.1016/j.joca.2005.11.015
41. Ceruti, S, Giammarioli, AM, Camurri, A, Falzano, L, Rufini, S, Frank, C, et al. Adenosine- and 2-chloro-adenosine-induced cytopathic effects on myoblastic cells and myotubes: involvement of different intracellular mechanisms. Neuromuscul. Disord. (2000) 10:436–46. doi: 10.1016/S0960-8966(00)00118-8
42. Tesch, AM, MacDonald, MH, Kollias-Baker, C, and Benton, HP. Effects of an adenosine kinase inhibitor and an adenosine deaminase inhibitor on accumulation of extracellular adenosine by equine articular chondrocytes. Am J Vet Res. (2002) 63:1512–9. doi: 10.2460/ajvr.2002.63.1512
43. Hamilton, JA, Cook, AD, and Tak, PP. Anti-colony-stimulating factor therapies for inflammatory and autoimmune diseases. Nat Rev Drug Discov. (2016) 16:53–70. doi: 10.1038/nrd.2016.231
44. Hume, DA, and MacDonald, KP. Therapeutic applications of macrophage colony-stimulating factor-1 (CSF-1) and antagonists of CSF-1 receptor (CSF-1R) signaling. Blood. (2012) 119:1810–20. doi: 10.1182/blood-2011-09-379214
45. Danks, L, Sabokbar, A, Gundle, R, and Athanasou, NA. Synovial macrophage-osteoclast differentiation in inflammatory arthritis. Ann Rheum Dis. (2002) 61:916–21. doi: 10.1136/ard.61.10.916
46. Kitaura, H, Zhou, P, Kim, HJ, Novack, DV, Ross, FP, and Teitelbaum, SL. M-CSF mediates TNF-induced inflammatory osteolysis. J Clin Invest. (2005) 115:3418–27. doi: 10.1172/JCI26132
47. Kodama, H, Yamasaki, A, Nose, M, Niida, S, Ohgame, Y, Abe, M, et al. Congenital osteoclast deficiency in osteopetrotic (op/op) mice is cured by injections of macrophage colony-stimulating factor. J Exp Med. (1991) 173:269–72. doi: 10.1084/jem.173.1.269
48. Bischof, RJ, Zafiropoulos, D, Hamilton, JA, and Campbell, IK. Exacerbation of acute inflammatory arthritis by the colony-stimulating factors CSF-1 and granulocyte macrophage (GM)-CSF: evidence of macrophage infiltration and local proliferation. Clin Exp Immunol. (2000) 119:361–7. doi: 10.1046/j.1365-2249.2000.01125.x
49. Campbell, IK, Rich, MJ, Bischof, RJ, and Hamilton, JA. The colony-stimulating factors and collagen-induced arthritis: exacerbation of disease by M-CSF and G-CSF and requirement for endogenous M-CSF. J Leukoc Biol. (2000) 68:144–50. doi: 10.1189/jlb.68.1.144
50. Ohno, H, Uemura, Y, Murooka, H, Takanashi, H, Tokieda, T, Ohzeki, Y, et al. The orally-active and selective c-Fms tyrosine kinase inhibitor Ki20227 inhibits disease progression in a collagen-induced arthritis mouse model. Eur J Immunol. (2008) 38:283–91. doi: 10.1002/eji.200737199
51. Giordano, R, Petersen, KK, Andersen, HH, Simonsen, O, and Arendt-Nielsen, L. Serum inflammatory markers in patients with knee osteoarthritis: a proteomic approach. Clin J Pain. (2020) 36:229–37. doi: 10.1097/AJP.0000000000000804
52. Saleh, R, Lee, MC, Khiew, SH, Louis, C, Fleetwood, AJ, Achuthan, A, et al. CSF-1 in inflammatory and arthritic pain development. J Immunol. (2018) 201:2042–53. doi: 10.4049/jimmunol.1800665
53. Chwastek, J, Kędziora, M, Borczyk, M, Korostyński, M, and Starowicz, K. Inflammation-driven secretion potential is upregulated in osteoarthritic fibroblast-like Synoviocytes. Int J Mol Sci. (2022) 23:11817. doi: 10.3390/ijms231911817
54. Clase, KL, Mitchell, PJ, Ward, PJ, Dorman, CM, Johnson, SE, and Hannon, K. FGF5 stimulates expansion of connective tissue fibroblasts and inhibits skeletal muscle development in the limb. Dev. Dyn. (2000) 219:368–80. doi: 10.1002/1097-0177(2000)9999:9999<::AID-DVDY1056>3.0.CO;2-8
55. Matsumoto, K, Hashimoto, K, Yoshikawa, K, and Nakamura, T. Marked stimulation of growth and motility of human keratinocytes by hepatocyte growth factor. Exp Cell Res. (1991) 196:114–20. doi: 10.1016/0014-4827(91)90462-4
56. Shiota, G, Wang, TC, Nakamura, T, and Schmidt, EV. Hepatocyte growth factor in transgenic mice: effects on hepatocyte growth, liver regeneration and gene expression. Hepatology. (1994) 19:962–72. doi: 10.1002/hep.1840190423
57. Gong, R . Multi-target anti-inflammatory action of hepatocyte growth factor. Curr. Opin. Investig. Drugs. (2008) 9:1163–70.
58. Dankbar, B, Neugebauer, K, Wunrau, C, Tibesku, CO, Skwara, A, Pap, T, et al. Hepatocyte growth factor induction of macrophage chemoattractant protein-1 and osteophyte-inducing factors in osteoarthritis. J Orthop Res. (2007) 25:569–77. doi: 10.1002/jor.20338
59. Takebayashi, T, Iwamoto, M, Jikko, A, Matsumura, T, Enomoto-Iwamoto, M, Myoukai, F, et al. Hepatocyte growth factor/scatter factor modulates cell motility, proliferation, and proteoglycan synthesis of chondrocytes. J Cell Biol. (1995) 129:1411–9. doi: 10.1083/jcb.129.5.1411
60. Jafri, MA, Kalamegam, G, Abbas, M, Al-Kaff, M, Ahmed, F, Bakhashab, S, et al. Deciphering the Association of Cytokines, chemokines, and growth factors in Chondrogenic differentiation of human bone marrow mesenchymal stem cells using an ex vivo osteochondral culture system. Front. Cell Dev. Biol. (2019) 7:380. doi: 10.3389/fcell.2019.00380
61. Terkeltaub, R, Yang, B, Lotz, M, and Liu-Bryan, R. Chondrocyte AMP-activated protein kinase activity suppresses matrix degradation responses to proinflammatory cytokines interleukin-1β and tumor necrosis factor α. Arthritis Rheum. (2011) 63:1928–37. doi: 10.1002/art.30333
62. Ushiyama, T, Chano, T, Inoue, K, and Matsusue, Y. Cytokine production in the infrapatellar fat pad: another source of cytokines in knee synovial fluids. Ann Rheum Dis. (2003) 62:108–12. doi: 10.1136/ard.62.2.108
63. Kou, S, and Wu, Y. Meta-analysis of tumor necrosis factor alpha −308 polymorphism and knee osteoarthritis risk. BMC Musculoskelet Disord. (2014) 15:373. doi: 10.1186/1471-2474-15-373
64. Maksymowych, WP, Russell, AS, Chiu, P, Yan, A, Jones, N, Clare, T, et al. Targeting tumour necrosis factor alleviates signs and symptoms of inflammatory osteoarthritis of the knee. Arthritis Res Ther. (2012) 14:R206. doi: 10.1186/ar4044
65. Singhal, S, Hasan, N, Nirmal, K, Chawla, R, Chawla, S, Kalra, BS, et al. Bioavailable turmeric extract for knee osteoarthritis: a randomized, non-inferiority trial versus paracetamol. Trials. (2021) 22:105. doi: 10.1186/s13063-021-05053-7
66. Karlapudi, V, Sunkara, KB, Konda, PR, Sarma, KV, and Rokkam, MP. Efficacy and safety of Aflapin®, a novel Boswellia Serrata extract, in the treatment of osteoarthritis of the knee: a short-term 30-day randomized, double-blind, placebo-controlled clinical study. J. Am. Nutr. Assoc. (2023) 42:159–68. doi: 10.1080/07315724.2021.2014370
67. Burkly, LC, Michaelson, JS, Hahm, K, Jakubowski, A, and Zheng, TS. TWEAKing tissue remodeling by a multifunctional cytokine: role of TWEAK/Fn14 pathway in health and disease. Cytokine. (2007) 40:1–16. doi: 10.1016/j.cyto.2007.09.007
68. Kamijo, S, Nakajima, A, Kamata, K, Kurosawa, H, Yagita, H, and Okumura, K. Involvement of TWEAK/Fn14 interaction in the synovial inflammation of RA. Rheumatology (Oxford). (2008) 47:442–50. doi: 10.1093/rheumatology/ken006
69. Wiley, SR, and Winkles, JA. TWEAK, a member of the TNF superfamily, is a multifunctional cytokine that binds the TweakR/Fn14 receptor. Cytokine Growth Factor Rev. (2003) 14:241–9. doi: 10.1016/S1359-6101(03)00019-4
70. Vincenti, MP, and Brinckerhoff, CE. Transcriptional regulation of collagenase (MMP-1, MMP-13) genes in arthritis: integration of complex signaling pathways for the recruitment of gene-specific transcription factors. Arthritis Res. (2002) 4:157–64. doi: 10.1186/ar401
71. Campbell, S, Michaelson, J, Burkly, L, and Putterman, C. The role of TWEAK/Fn14 in the pathogenesis of inflammation and systemic autoimmunity. Front. Biosci. (2004) 9:2273–84. doi: 10.2741/1395
Keywords: knee osteoarthritis, biomarkers, Mendelian randomization, GWAS, inflammation
Citation: Xu L, Ma J, Yu Q, Zhu K, Wu X, Zhou C and Lin X (2024) Evidence supported by Mendelian randomization: impact on inflammatory factors in knee osteoarthritis. Front. Med. 11:1382836. doi: 10.3389/fmed.2024.1382836
Edited by:
Weikuan Gu, University of Tennessee Health Science Center (UTHSC), United StatesCopyright © 2024 Xu, Ma, Yu, Zhu, Wu, Zhou and Lin. This is an open-access article distributed under the terms of the Creative Commons Attribution License (CC BY). The use, distribution or reproduction in other forums is permitted, provided the original author(s) and the copyright owner(s) are credited and that the original publication in this journal is cited, in accordance with accepted academic practice. No use, distribution or reproduction is permitted which does not comply with these terms.
*Correspondence: Chuanlong Zhou, 20105016@zcmu.edu.cn; Xianming Lin, linxianming66@126.com
†These authors have contributed equally to this work and share first authorship