- 1UCLA Brain Tumor Imaging Laboratory (BTIL), Center for Computer Vision and Imaging Biomarkers, University of California, Los Angeles, Los Angeles, CA, United States
- 2Department of Radiological Sciences, David Geffen School of Medicine, University of California, Los Angeles, Los Angeles, CA, United States
- 3Department of Radiology, Mayo Clinic, Rochester, MN, United States
- 4UCLA Neuro-Oncology Program, University of California, Los Angeles, Los Angeles, CA, United States
- 5Department of Neurology, David Geffen School of Medicine, University of California, Los Angeles, Los Angeles, CA, United States
- 6Center for Neuro-Oncology, Dana-Farber/Brigham and Women’s Cancer Center, Harvard Medical School, Boston, MA, United States
- 7Department of Bioengineering, Henry Samueli School of Engineering and Applied Science, University of California, Los Angeles, Los Angeles, CA, United States
- 8Department of Neurosurgery, David Geffen School of Medicine, University of California, Los Angeles, Los Angeles, CA, United States
- 9Department of Psychiatry and Biobehavioral Sciences, David Geffen School of Medicine, University of California, Los Angeles, Los Angeles, CA, United States
Standardized MRI acquisition protocols are crucial for reducing the measurement and interpretation variability associated with response assessment in brain tumor clinical trials. The main challenge is that standardized protocols should ensure high image quality while maximizing the number of institutions meeting the acquisition requirements. In recent years, extensive effort has been made by consensus groups to propose different “ideal” and “minimum requirements” brain tumor imaging protocols (BTIPs) for gliomas, brain metastases (BM), and primary central nervous system lymphomas (PCSNL). In clinical practice, BTIPs for clinical trials can be easily integrated with additional MRI sequences that may be desired for clinical patient management at individual sites. In this review, we summarize the general concepts behind the choice and timing of sequences included in the current recommended BTIPs, we provide a comparative overview, and discuss tips and caveats to integrate additional clinical or research sequences while preserving the recommended BTIPs. Finally, we also reflect on potential future directions for brain tumor imaging in clinical trials.
1. General concepts
1.1. Role and aims of BTIPs in clinical trials
More than 80,000 patients are diagnosed with primary CNS tumors every year in the United States (incidence 24.7 per 100,000 population), with malignant forms accounting for 28.3% of the cases (1, 2). Among malignant primary tumors, gliomas have the highest incidence (4.26 per 100,000), while primary CNS lymphomas (PCNSL) are significantly rarer (0.46 per 100,000) (2). The incidence of metastatic CNS tumors is remarkably higher than primary tumors, with approximately 200,000 patients receiving a new diagnosis every year in the United States (3). Given the poor prognosis of these types of tumors, newer treatment options are constantly tested, including anti-angiogenic treatments, immunotherapy, and targeted therapy (3–7). Although the optimal endpoint of clinical trials for proving drug efficacy is an increase in overall survival (OS) in clinical trials, radiologic progression-free survival (PFS) and objective response rate (ORR) are considered valuable surrogate endpoints (8, 9). This concept applies to primary CNS tumors, and is even more relevant for patients with brain metastases (BM). In patients with BM, OS is frequently linked to systemic disease and the tested drugs may show heterogeneous efficacy on CNS localization compared to the systemic ones. For these two reasons, in BM patients the radiologic evaluation may be the most reliable measurement for drug efficacy in CNS.
One limitation of radiologic response assessment in clinical trials is the variability among images acquired in different institutions and on different scanners. Such variability arises from a number of factors including technique, acquisition parameters, two- vs. three-dimensional acquisition schemes, slice prescription and tilt/pitch differences, use of fat saturation, and timing of sequences with respect to the moment of gadolinium based contrast agent (GBCA) injection (10). In recent years, extensive effort has been made by consensus groups to propose standardized brain tumor imaging protocols (BTIPs), aiming to reduce such variability in measurement and interpretation (8, 11–13). The concept behind BTIPs is to ensure high image quality while maximizing the number of institutions meeting the acquisition requirements. Indeed, recommending ambitious guidelines that cannot be implemented in smaller institutions would dramatically reduce the number of centers eligible for clinical trials. As a partial solution for this compromise, BTIPs consensus papers feature both an “ideal” protocol and a “minimum” recommended protocol, with variations dependent on field strength. Additionally, proposed BTIPs comply to the will of limiting the protocols to 30 min, in order for them to be feasible in patients with low compliance on one hand, and compatible with the integration of additional clinically-required sequences on the other hand.
1.2. BTIPs serve RANO evaluation and beyond
The choice of BTIPs pulse sequences and the structure of the protocols are conceived to provide datasets whose evaluation can determine treatment response or failure as defined by the current recommended criteria. Treatment response in brain tumor clinical trials is assessed through Response Assessment in Neuro-Oncology (RANO) criteria. The first RANO criteria were originally proposed for high-grade gliomas (HGG), and are therefore often referred to as RANO-HGG (14). In the following decade, consensus groups proposed numerous variations and updates of RANO, including modified RANO (mRANO) for glioblastoma (15), specific criteria for lesions receiving immunotherapy (iRANO) (16), and for low-grade gliomas (LGG-RANO) (17). The latest effort in this regard is represented by the upcoming RANO 2.0, which aim to integrate previous considerations from different RANO guidelines and to address their reported limitations, to ultimately propose unified criteria (18). Of note, RANO 2.0 are conceived to be applicable to both LGG and HGG gliomas in adult patients, while brain metastases (BM) and pediatric patients may still be evaluated with dedicated criteria: RANO-BM (19) and RAPNO (20), respectively. Finally, separate response criteria have been proposed for PCNSL (21).
All variations of RANO criteria propose that, at every timepoint of the trial, each patient is assigned either progressive disease (PD), stable disease (SD), partial response (PR), or complete response (CR) compared to baseline or previous scans. The category assigned depends on the integrated evaluation of radiographic findings, clinical findings, and steroid dose. For the radiologic assessment, the most important sequence to evaluate is post-contrast T1-weighted (T1-post), since the enhancing tumor volume is the best radiologic surrogate of tumor burden in HGG (22–27), with some exceptions, and since BM tissue is exclusively enhancing. PCSNL radiologic evaluation, too, is based exclusively on enhancing lesions (21). Obviously, this does not apply to non-enhancing LGG, for which radiologic assessment is based on non-enhancing tumor volume (see LGG-RANO), evaluated on T2-weighted (T2) and T2-weighted fluid-attenuated inversion recovery (FLAIR). Notably, the evaluation of non-enhancing components in HGG is featured in RANO-HGG and iRANO, although no strict rules are mandated to perform such evaluation, while mRANO focus only on the enhancing tissue. The upcoming RANO 2.0 criteria propose to eschew the evaluation of non-enhancing components in tumors with contrast-enhancement, while preserving the evaluation of non-enhancing components in tumors that are largely-non-enhancing. The main role of pre-contrast T1-weighted (T1-pre) images is to exclude from the enhancing portion measurements any areas of spontaneous T1-hyperintensity (e.g., blood products, melanin). Interestingly, mRANO criteria propose a more thorough usage of T1-pre, by (optionally) evaluating the enhancement components based on T1-subtraction maps, which increase contrast-to-noise ratio of the enhancing tissue. T1-subtraction maps are obtained by normalizing T1-post and T1-pre signals, co-registering the two, and operating a voxel-wise subtraction (25). Finally, at this time none of the proposed RANO variations mandate an evaluation of diffusion MRI (dMRI) or perfusion MRI (pMRI), which are nonetheless included (dMRI) and encouraged (pMRI) in BTIPs, respectively.
While the main objective of the proposed BTIPs is to obtain high-quality images for response assessment in the present clinical trial, it must be emphasized that clinical trials represent an occasion to collect abundant longitudinal datasets from a patient population with set inclusion/exclusion criteria and with serial clinical evaluations. As such, images obtained during clinical trials are a valuable resource for subsequent retrospective radiologic studies on brain tumor patients, with the possibility of hypothesis testing with clinical and prognostic correlations. This should be taken into consideration when BTIPs are first implemented and potentially integrated with additional pulse sequences. Finally, BTIPs represent an occasion to reflect and reach consensus among panels of experts regarding the best strategies to obtain images to assess treatment efficacy. As such, concepts and guidelines emerging from BTIPs should also inspire and guide the choices of sequences and strategies for imaging protocols in the clinical routine.
2. Rationale behind BTIP sequences and technical considerations
2.1. T1-pre and T1-post images
As mentioned, the evaluation of the enhancing components with T1-weighted imaging is the most crucial step of the radiologic response assessment. The key concepts of T1-weighted imaging in BTIPs include matching parameters of T1-pre and T1-post, the timing, dosage, and type of contrast agent, the requirement for 3D imaging, and recommendations regarding the choice of gradient-echo (GRE) and/or spin-echo (SE) acquisitions (8, 11–13).
BTIPs recommend parameter-matched T1-pre and T1-post because acquiring both sequences with the same parameters and technique optimizes the comparative evaluation of enhancement with respect to inherent T1-pre signal, as well as the generation of T1-subtraction maps, if desired.
The timing of contrast injection is another relevant factor that can influence the evaluation. According to all BTIPs, T1-post images should be collected 4–8 min after contrast agent injection. This recommendation is based on previous evidence that the maximum contrast uptake takes place in this time window (28). Notably, for most lesions evaluated in this study the ratio between the enhancing tissue and normal gray matter reached its peak after 4–8 min and then plateaued for several minutes before slowly decreasing. Conversely, at 3.5 min 30% of lesions still hadn't reached their enhancement peak. Overall, this suggests that the highest lesion conspicuity is obtained by waiting at the very least 4 min from injection to T1-post acquisition, while waiting a little longer than 8 min may in theory not be as problematic. However, the 4–8 min time window should be respected also to maximize longitudinal reproducibility. This 4–8 min gap is typically filled with the acquisition of T2-weighted images. Additionally, the injection can be performed during the acquisition of dynamic susceptibility contrast (DSC) perfusion imaging. In this case, the time gap between injection and T1-post corresponds to the sum of the acquisition time of the post-bolus volumes of DSC and the acquisition time of the T2-weighted sequence.
BTIPs recommend to acquire T1-post after one single dose of GBCA of 0.1 mmol/kg. This corresponds to 1 ml/10 kg for contrast agents with molar concentration 1 mmol/ml, and 2 ml/10 kg for contrast agents with molar concentration 0.5 mmol/ml. This concept is important to keep in mind in case of integration with clinical or research protocols acquiring multiple boli of GBCA, for instance in case both DSC and DCE (dynamic contrast enhanced) imaging are performed, as discussed in the following paragraphs. On a related note, the commercially available gadolinium-based agents are characterized by remarkably different relaxivities, which impact the enhancement obtained for a given dose of contrast agent (29, 30). Therefore, the same type of GBCA should be used consistently at all follow-up scans for a given patient, to maximize the reliability of longitudinal comparisons, and should be reported on the DICOM header along with the dosage (8).
Moreover, 3D imaging is recommended. One of the reasons is that 3D imaging achieves thinner slices, which are known to allow a better evaluation of enhancement, including better detection of small lesions (31). 3D imaging also allows reformatting of the acquired imaging volume into other planes and to potentially adjust slice orientation. This is relevant because it has been shown that changes in head tilting can impact the treatment response evaluation (10). As an additional advantage, 3D imaging allows a better volumetric segmentation of the contrast-enhancing lesions. Improved segmentations have two advantages. First, they can be used to assess the radiologic response with volumetric thresholds as proposed by mRANO and RANO 2.0 (15, 18). Of note, the volumetric thresholds are applied to measurable disease, whose assessment is central for the response and progression criteria in RANO 2.0, together with the evaluation of the non-measurable disease, the clinical status, the steroid dosage, and the leptomeningeal involvement (18). Second, improved segmentations benefit further imaging analyses beyond clinical trials, for imaging studies focusing on tumor radiomics or advanced imaging.
Finally, BM and PCNSL BTIPs overall advocate that 3D TSE images should be preferred to 3D IR-GRE. This indication follows evidence supporting a better tumor-to-background contrast and lesion conspicuity using SE compared to GRE, when the slice thickness is comparable (31, 32). The historical reason for BTIPs including 3D IR-GRE (inversion recovery GRE, such as MPRAGE or IR-SPGR) is that on older scanners a thinner slice thickness is achievable with IR-GRE (8), and data showed that enhancement rate and contrast rate are higher for thin-sliced IR-GRE than for thick-sliced SE (31). When the slice thickness can be matched, though, and SE can be obtained with 1 mm voxels, the detectability of small lesions with SE is superior, as confirmed in a meta-analysis (33). This may be also ascribable to the high white matter signal in IR-GRE, which can mask small enhancing lesions for lack of tumor-to-background contrast. As an additional advantage, applying motion-sensitized driven-equilibrium preparation to TSE T1-post also allows black blood imaging (34), eliminating vascular enhancement that is typically seen in 3D IR-GRE and achieving a higher sensitivity in the identification of small superficial lesions of the cortex and leptomeningeal neoplastic involvement (35, 36). Finally, unlike IR-GRE, SE T1 allows for fat saturation, which is particularly helpful in case of metastastic involvement of the bony structures (12). Therefore, the recent BM and PCNSL BTIPs recommend employing pre- and post-contrast 3D TSE (turbo SE) in the “ideal” protocol, while leaving 3D IR-GRE in the “minimum” requirements together with an additional 2D SE T1 (12, 13), also considering that 3D TSE T1 superiority to 3D IR-GRE has been more thoroughly investigated at 3 T. As for gliomas, 3D IR-GRE is currently still the sequence of choice for T1-weighted imaging in clinical trials. In the original glioma BTIP initiative, 3D IR-GRE was preferred because it yields adequate image quality and is available on almost all MR systems as a result of the standardized ADNI initiative (37, 38), while 3D TSE is not available on all scanners and the various pulse sequences are not standardized across vendors. On a side note, adopting 3D TSE to improve lesion conspicuity and identify smaller lesions would be less relevant for gliomas in clinical trials, since, according to RANO 2.0, only measurable new contrast-enhancement (≥1 cm × ≥ 1 cm in-plane diameters) should be categorized as progressive disease. Overall, whether 3D TSE or 3D IR-GRE should be the T1-weighted sequence of choice for future glioma BTIPs is debated, as more institutions have been adopting 3D TSE in the clinical practice and pieces of evidence in its favor are being collected for gliomas (39). As of today, it is advisable to maintain 3D IR-GRE as the core T1-weighted sequence for gliomas to comply with glioma BTIP, with the option of acquiring additional post-contrast 3D TSE after, in case an institution has a preference toward it. However, this recommendation may change if a new agreement from a large panel of experts is reached.
2.2. T2-weighted images, T2-weighted FLAIR, and post-contrast T2-weighted FLAIR
T2-weighted TSE and T2-weighted FLAIR images are acquired to evaluate the non-enhancing components of tumors. As mentioned, the evaluation of non-enhancing tissue is central for LGG, marginal for HGG only according to RANO-HGG, iRANO, and RANO 2.0, and not acknowledged in mRANO, RANO-BM, and PCNSL response criteria. Overall, when the evaluation of non-enhancing sub-regions is required, response criteria do not dictate whether it should be performed using mainly T2 or FLAIR, and this choice is left to the preference of the reader, even though it is good practice to evaluate both.
Glioma BTIP proposes to optionally obtain T2 images through a dual-echo proton density and T2-weighted (PD/T2) TSE sequence (8). This approach allows to compute “effective T2”, a quantitative measurement of T2 relaxation with the potential of distinguishing vasogenic edema from non-enhancing tumor (40). While this quantitative metric is not currently used in response assessment, it has potential for the evaluation of non-enhancing tumor burden, and the acquisition of PD/T2 comes with no additional time penalty.
Both T2-weighted FLAIR and T2 images can be collected using 2D or 3D acquisition schemes. While 3D FLAIR has been encouraged since 2015 glioma BTIP (8), it only appeared as a required sequence in the recent PCNSL BTIP, in both the “ideal” and “minimum” protocols (13). The underlying reason is that, once again, BTIPs were structured to be inclusive of institutions with older scanners. However, 3D T2-weighted FLAIR images of good quality are progressively becoming more easily available on many scanners in use. As discussed for 3D T1, 3D T2-weighted FLAIR has the advantage of allowing tilting readjustment, reformatting in other planes, thinner slice thickness and better feasibility of lesion segmentation. For this reason, when images of good quality are obtainable, 3D FLAIR is highly recommended particularly for clinical trials involving LGG, where the assessment of the non-enhancing tumor is essential. Indeed, it has been recently demonstrated that 3D volumetric measurements obtained from lesion segmentation in LGG should be preferred for treatment response assessment, as they are have better inter-reader agreement and stable longitudinal measurements compared to 2D bidirectional diameters (41).
The use of fat saturation and the specific inversion time (TIs) prescribed are two aspects of FLAIR acquisition worth further discussion. The presence or absence of fat saturation can significantly impact the apparent extent and intensity of FLAIR signal alteration, constituting a potential confounding factor during interpretation of changes over time (10). Without the use of fat saturation, the bone marrow within the skull appears the brightest on T2-weighted FLAIR, while after fat saturation the bone marrow signal is nulled and T2 hyperintense areas are the brightest. Given the resulting images have a fixed dynamic range of signal intensities, the application of fat saturation can therefore dramatically change the contrast between non-enhancing tumor and surrounding brain tissue. Similar to other potential confounding factors (e.g., field strength, contrast agent type, scanning parameters), fat saturation is challenging to homogenize across institutions and the best practice is longitudinal consistency throughout all follow-up scans for a given patient. Currently, the PCNSL BTIP reports fat saturation on FLAIR as optional. As for TI, it has been shown that a lower TI (<2,400 ms at 3 T) enhances T2-FLAIR mismatch (T2FM) sign. T2FM is a radiogenomic sign with high specificity for IDH-mutant 1p19q-intact molecular status in gliomas (i.e., astrocytomas) when compared to IDH-mutant 1p19q-codeleted (i.e., oligodendrogliomas) and IDH-wild-type (i.e., glioblastomas), and arises from a partial T2 signal suppression on FLAIR images (42). The aforementioned study showed that a lower TI appears to increase the accuracy of T2FM to identify astrocytomas without causing an increase in false positive results (43). While a lower TI may improve the T2 signal suppression in some tumor regions for molecular profiling purposes, it also causes the corresponding tumor regions to appear isointense to normal tissue, with potential underestimation of non-enhancing tumor burden. Current BTIPs allow a certain flexibility when setting the TI (2,000–2,500 ms), with potential heterogeneity in T2 signal suppression in non-enhancing regions across different protocols. This once again advocates for evaluating both FLAIR and T2 during radiographic reads, if an assessment of the non-enhancing components is warranted, especially in protocols acquired with a higher FLAIR TI.
T2-weighted FLAIR images can also be acquired after the administration of contrast (FLAIR-post), which is preferred by some institutions and is featured in the PCNSL BTIP (13). FLAIR-post both display tissue with T2 hyperintensity and areas of contrast enhancement, since FLAIR images contain a mixture of both T1 and T2-weighting. In certain conditions such as low gadolinium concentration in the tissue, FLAIR-post has been reported to be more sensitive than T1-post in detecting subtle enhancement. FLAIR-post has potential applicability in several settings, including the detection of subtle intra-axial enhancement and the evaluation of lepto- and pachymeningeal neoplastic involvement (44). Such characteristics justify the inclusion of FLAIR-post in PCNSL BTIP, since lymphomas frequently disseminate to the meninges. Additionally, these characteristics support a potential application in gliomas and metastases to identify meningeal spread and for the detection of small intra-axial lesions (45–49), and some institutions prefer to acquire FLAIR-post in gliomas and BM, too. Acquiring FLAIR after contrast injection, as opposed to before, probably does not interfere with treatment response assessments. Indeed, the area of FLAIR intra-axial enhancement would lie within the T2-FLAIR hyperintense tissue, and therefore would not affect the measurement of the non-enhancing component in gliomas. As for meningeal spread, FLAIR-post may increase the sensitivity to meningeal involvement, which can be useful in both gliomas and BM. Overall, it is reasonable to consider FLAIR-post as an acceptable BTIL-compliant alternative in BM and gliomas—as long as protocol consistency is respected throughout timepoints. In the case FLAIR-post is preferred, the sequence order should be acquired as in PCNSL BTIP, with T2 before contrast and FLAIR after contrast. For efficiency, FLAIR-post should be acquired immediately after contrast agent injection per in PCNSL BTIP recommendations; however, data suggests contrast may be maximized through a delayed acquisition (20 min after contrast injection) (44), suggesting a potential role of collecting FLAIR-post after acquisition of conventional post-contrast T1-weighted images.
2.3. Diffusion and perfusion imaging
Diffusion-weighted imaging (DWI) and dynamic susceptibility contrast (DSC) perfusion imaging are common in both routine clinical and research studies in brain tumors (50–52).
DWI-derived apparent diffusion coefficient (ADC) images reflect microscopic water Brownian diffusion (53) and its values are considered a proxy for tumor cell density and tumor microstructure (52, 54). ADC has been proposed as a biomarker in neuro-oncology for molecular profiling (55–57), differential diagnosis (58), and treatment response assessment (54, 59). All current BTIPs propose 2D DWI acquisitions with at least 3 directions and at least 3 b-values (approximately 0, 500, and 1,000 s/mm2), per consensus recommendations by the International Society for Magnetic Resonance in Medicine (60).
While DWI is part of BTIPs and of the clinical neuroimaging protocols, ADC evaluation are currently not part of response assessment criteria. Part of the reason is that ADC interpretation is not trivial in the follow-up phase. Following treatment, and specifically radiation, changes in ADC values probably result from complex combined changes not only in cellularity, but also in extracellular matrix composition, in the presence of necrotic foci and edematous tissue, and possibly in vascular permeability. Additionally, ADC measurements suffer from susceptibility artifacts mainly induced by air-tissue interfaces and paramagnetic material (e.g., blood products) (61). Evidence from post-surgical case series (62) and from clinical trial images (63) suggest that artifacts and corrupted images may remarkably reduce the number of usable diffusion datasets, with a rate of images with unusable ADC values reported around 27.5% and 32%, respectively.
DSC perfusion is commonly employed in neuro-oncology to evaluate cerebral blood volume (CBV), and/or its derivates relative CBV and normalized relative CBV (rCBV and nrCBV, respectively), in order to quantify the degree of angiogenesis or tumor vascularity (64–66). Traditional measures of CBV has been shown to correlate with vascular density (67), thus providing a measure of relative tumor vascularity. Extensive literature has demonstrated the value of CBV for molecular profiling of gliomas (52, 55, 68), differential diagnosis (65, 69), treatment response assessment (70–72), and the discrimination between treatment effects and tumor recurrence (65, 73–75). From a technical standpoint, the estimation of CBV using DSC assumes the GBCA remains within the vasculature during acquisition (i.e., doesn't leak into the extravascular, extracellular space), and thus, the accuracy of CBV measurements is strongly affected by violations of this assumption (76). In particular, T1 leakage effects may result in CBV underestimation, therefore recent guidelines propose strategies to reduce CBV sensitivity to these effects (11). Current guidelines for DSC implementation in HGG include a combination of the following strategies: reducing the flip angle (FA) while using an appropriate field strength dependent echo time (TE), administering a preload bolus, and applying leakage correction during post-processing (11). FA and TE adjustments act on DSC sensitivity to T1 relaxation, since T1 sensitivity is mitigated by a low FA and/or high TE (76, 77). The administration of a preload bolus is aimed to partially saturate the baseline T1 contribution to the signal, therefore mitigating T1 leakage effects (11). Finally, post-hoc leakage correction using mathematical modeling to account for contrast leakage should be performed to further improve the accuracy of the measurements (11, 78). The current consensus guidelines for DSC on HGG recommend GRE echo planar imaging (GRE-EPI), either with a full preload GBCA dose (1 + 1 dosing) and FA 60° (“intermediate” FA) or with no preload (0 + 1 dosing) and FA 30° (“low” FA), with TE 30 ms (at 3 T) or 45 ms (at 1.5 T), and uni- or bidirectional leakage correction (11). BM and PCNSL BTIPs adopted DSC HGG guidelines and comply with the DSC consensus by proposing the alternative with low FA and no preload, if the 0 + 1 dosing is desired (12, 13). It is worth mentioning that only some of the previously proposed preload schemes are BTIP-compliant. The 0 + 1 (no preload), 1 + 1 (full dose preload) and ½ + ½ (half dose preload and half dose injection) schemes are all BTIP-compliant because they allow the acquisition of T1-post after a single dose of GBCA. Other schemes, such as ½ + 1 dosing, are not acceptable because T1-post would be acquired either after half dose or after one and a half.
In addition to CBV, DSC perfusion can potentially provide additional information about the tumor microenvironment. The comparison of post-bolus to pre-bolus DSC signal intensity, as measured with the percentage of signal recovery (PSR), is thought to be influenced by tissue microstructure, and therefore useful for differential diagnosis (65, 79). Post-bolus signal intensity is influenced by the balance between T2* and T1 leakage effects, which has been suggested to reflect tissue cytoarchitecture (77, 80, 81). PSR utility has been demonstrated in the diagnostic phase rather than in treatment response monitoring so far, thus its evaluation may not be directly relevant for clinical trials at the moment. However, it is worth noticing that a recent study supported the validity of PSR even when derived from BTIP-compliant DSC protocols that are optimized for CBV computation and therefore bear a weaker T1-weighting (82). This is relevant because it supports the adoption of BTIP-compliant DSC protocols for both CBV and PSR, both in the diagnostic and follow-up phases.
Although DSC is part of the clinical work-up of brain tumor and included in BTIPs, CBV assessment is not integrated in response criteria. During the monitoring of treatment response, CBV is potentially useful in cases of pseudoprogression (74, 83, 84), where tumor size assessment is not a reliable surrogate of tumor burden, since in such cases there is discrepancy between the apparent size changes and the actual response to the treatment. Another potential application of CBV in clinical trials is the demonstration of angiogenesis reduction following antiangiogenetic treatment (85, 86), even though such reduction does not appear to predict an extended OS (85). However, no reliable quantitative CBV cutoffs have been validated to identify disease progression. This is due to most studies being small and single center, inhomogeneity in DSC protocols, and a modest repeatability and reproducibility of CBV measurements (87). Overall, more efforts to achieve reliable and reproducible perfusion assessments are warranted in order to test CBV evaluations in multicenter trials.
3. Overview of current BTIPs
Table 1 provides a comparative overview of “ideal” recommended BTIPs at 3 T for gliomas (8), BM (12), and PCNSL (13). Table 2 illustrates the corresponding “minimum” BTIPs at 1.5 T. While the tables compare the protocols at the extreme of the spectrum (ideal at 3 T and minimum at 1.5 T), the cited BTIPs also propose “minimum” protocols at 3 T (only BM and PCNSL BTIPs) or an “ideal” protocol at 1.5 T (only glioma BTIP) (not reported in the tables). In both tables, DSC recommendations for gliomas have been integrated from the separate consensus paper for DSC in HGG (11).
As previously mentioned, 3D IR-GRE T1 is featured in the glioma BTIP, while 3D TSE T1 sequences are recommended as ideal in PCNSL and BM BTIPs. In case 3D TSE T1 are not available, it is suggested to use 3D IR-GRE T1-pre and acquire an additional 2D SE T1-post before 3D IR-GRE T1-post. When both a 3D IR-GRE and a 2D SE T1-post are acquired according to the minimum requirement protocols, it is advisable to use 2D SE T1-post to detect new non-measurable intra-axial lesions and osseous involvement, thanks to the superior conspicuity of small lesions on SE and to the possibility of fat saturation. On the other hand, if SE T1-post is acquired 2D, it is advisable to base lesion measurements on 3D IR-GRE, as it is parameter-matched with T1-pre, allowing for a better pre-to-post comparison and to exclude from the measurements the spontaneous T1-hyperintensity (e.g., due to hemorrhage or melanin), whether T1-subtraction maps are employed or not.
Other differences include 3D imaging for FLAIR and T2 in the most recent PCNSL BTIP, although 3D FLAIR was already strongly recommended in the glioma BTIP. Additionally, 3D FLAIR is acquired after contrast (FLAIR-post) according to the PCNSL BTIP, which eliminates the need for T2 being acquired after contrast injection.
Overall, the ideal protocols and a higher magnetic field (3 T) should be preferred, if possible. However, large-scale multicenter clinical trials often involve smaller academic and non-academic community-based hospitals, where only the “minimum” protocols may be feasible. In such cases, it is advisable, for the sake of consistency and standardization, that all the centers involved in the same trial comply with MRI protocols applicable to all the trial locations. As a result, most later stage clinical trials may choose to adopt the “minimum” protocols, whereas the “ideal” protocols designed for high-performance 3 T scanners may be more applicable in smaller, early phase studies at specific academic institutions.
Figure 1 displays a representative BTIP-compliant MRI exam for a glioma case. Figure 2 shows demonstrative images of improved lesion conspicuity on TSE T1-post compared to IR-GRE T1-post in a BM patient.
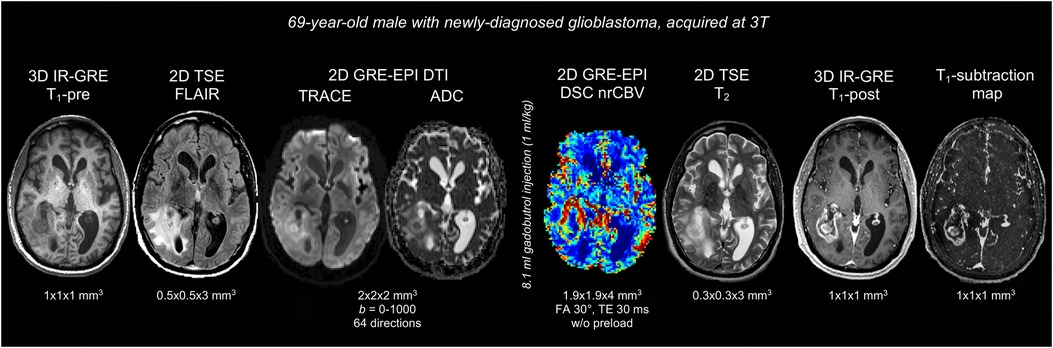
Figure 1. Representative case of BTIP-compliant glioma MRI exam. The images are shown in chronological order, and are displayed in their native acquisition space and voxel size. T1 images are acquired with matching technique (3D IR-GRE), parameters and voxel-size pre- and post-contrast, allowing for optimal T1-subtraction maps. FLAIR and T2 images are acquired with 2D TSE sequences. Diffusion imaging is obtained through a DTI acquisition (b = 0–1,000; 64 directions), as an alternative to axial DWI at 3 directions. DSC perfusion imaging is acquired without preload with a 30° flip-angle, in compliance with DSC guidelines.
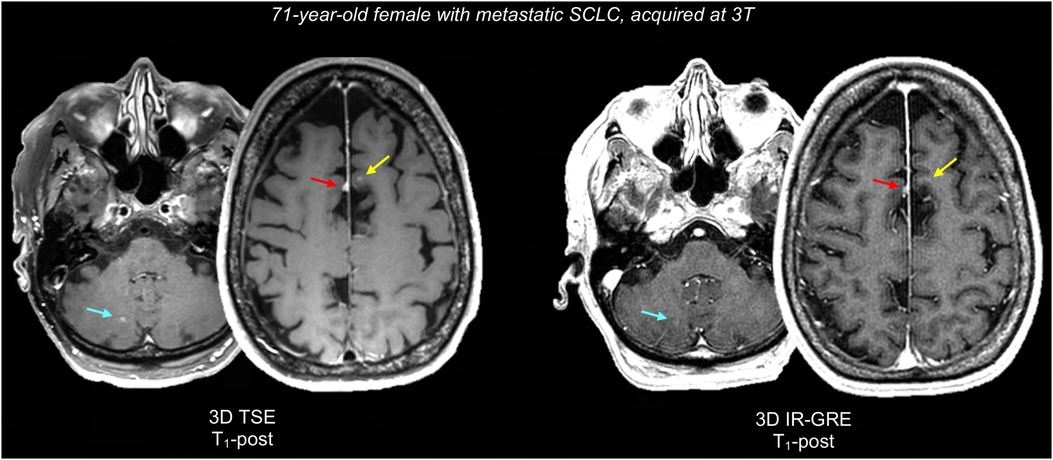
Figure 2. Representative case comparing lesion conspicuity between 3D TSE and 3D IR-GRE T1-post in a BM patient. 3D IR-GRE was acquired for SRS planning, one day after 3D TSE. 3D TSE shows a juxtacortical BM in the left superior frontal gyrus (2 mm, yellow arrow), that is not appreciable on 3D IR-GRE. Similarly, a right paravermian cerebellar BM (3 mm, teal arrow) is easily identifiable on 3D TSE, while it barely appreciable on 3D IR-GRE. Finally, both sequences show an incidental parafalcine meningioma (red arrow), which is also more conspicuous on 3D TSE, probably due to the reduced number of confounding bright cortical vessels. SCLC, small cell lung carcinoma.
4. Integration of additional sequences
BTIPs are typically acquired in the same MRI session as imaging needed for the clinical management of the patients and potentially also together with research sequences, especially in academic institutions. Therefore, it is important to be aware of caveats to be observed when integrating BTIPs and other clinical and research pulse sequences.
A crucial aspect to consider is the timing and dosage of GBCA administration with respects to additional sequences and BTIP sequences. Some additional sequences must be obtained before GBCA (e.g., functional MRI, fMRI), some others can be obtained either before or after (e.g., sodium imaging), and other ones require an additional GBCA administration (e.g., DCE). As for BTIP sequences, protocols integrating clinical or research acquisitions should be BTIP-compliant, meaning they should observe that T1-post images must be acquired around 4–8 min after contrast injection and after exactly one single dose of GBCA (0.1 mmol/kg).
Figure 3 shows some examples of additional advanced sequences that can be integrated with BTIPs.
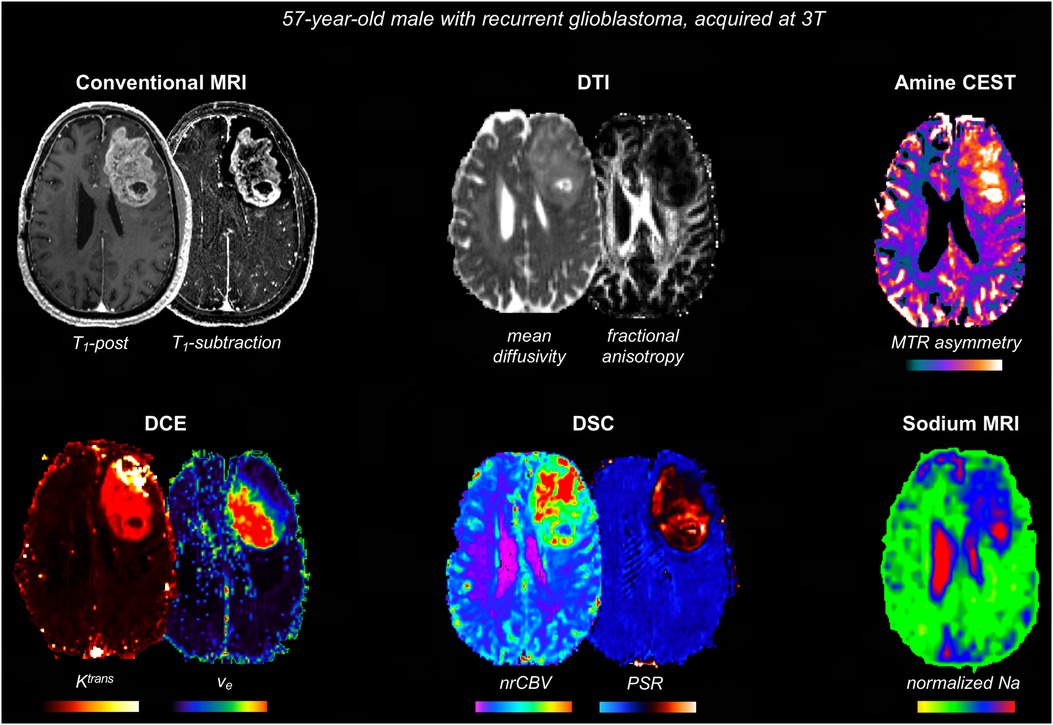
Figure 3. Representative case with additional advanced imaging acquisitions that can be integrated with BTIPs. DTI allows to compute fractional anisotropy and diffusivity, as well as perform tractography, if needed. Amine CEST can be employed to generate MTR asymmetry, reflecting tumor acidity. DSC maps are useful to assess tumor vasculature and angiogenesis (nrCBV) and tissue cytoarchitecture (PSR). DCE maps reflect the contrast leakage characteristics. In this case, DCE metrics were obtained simultaneously with DSC, using a dynamic spin-and-gradient-echo perfusion sequence as described in (81). Finally, sodium images were obtained at the end of the protocol and sodium signal voxel-wise was normalized to the eye sodium signal.
4.1. fMRI and DTI
fMRI and DTI (diffusion tensor imaging) may be acquired either for research projects or for presurgical planning (88), especially in gliomas. fMRI and DTI could be acquired as part of research protocols, for instance in studies investigating the value of DTI metrics for predicting treatment response (89) or in studies employing fMRI to evaluate neural plasticity and functional remapping (90). As for presurgical mapping, during the acquisition of BTIPs, the investigators usually do not have to include presurgical imaging, since clinical trials typically focus on systemic treatments initiated after surgery. In fact, radiologic evaluation of treatment response starts on post-surgical scans according to RANO (14) and on post-radiation scans according to mRANO (15) and RANO 2.0 (18). However, it may be useful to integrate presurgical fMRI and DTI during clinical trials when employing neoadjuvant treatments (in which patients can be imaged before surgery) or in case patients enrolled in clinical trials are considered for repeat surgery during follow-up. In both cases, it is possible that fMRI and DTI need to be integrated in BTIPs protocols for presurgical mapping purposes.
fMRI, either task-based or resting-state, should be acquired before contrast injection, since fMRI is based on T2*-weighted GRE-EPI sequences (91), similarly to DSC, and is therefore sensitive to T1 and T2* signal changes induced by gadolinium. fMRI acquisitions, tasks, and processing methods vary across institutions. The ASFNR guidelines propose to acquire fMRI with 3.4 × 3.4 mm2 in-plane resolution and 4–5 mm slice thickness, with six 10-volume blocks for tasks and six 10-volume blocks for rest, for a total of 120 EPI volumes.
DTI should be preferably acquired before contrast injection as well, in compliance to what is suggested for DWI in BTIPs. For completeness, it should be noted that there is evidence of contrast agent influencing DTI-metrics such as fractional anisotropy (92), while ADC measurements do not seem to be influenced by gadolinium (93, 94). The ASFNR guidelines for clinical DTI recommend to acquire DTI with a single-shot SE-EPI sequence, with isotropic voxels 2 × 2 × 2 mm3 at 3 T (2.5 × 2.5 × 2.5 mm3 at 1.5 T), and with three b = 0 images and at least 25 directions at b = 1,000 s/mm2 (https://www.asfnr.org/clinical-standards). Multicenter trials focusing on non-neoplastic diseases adopted similar parameters for DTI, with single-shell b = 1,000 s/mm2, 64 directions, and 2 × 2 × 2 mm3 isotropic voxels (95, 96). Other multicenter trials adopted similar protocols with some protocol variations, such as b = 700 s/mm2 (97) or b = 1,300 s/mm2 and voxel size 2.7 × 2.7 × 2.7 mm3 (98). Compared to BTIP-compliant DWI, these DTI sequences lack the b = 500 s/mm2 shell and have thinner slices, as well as a higher number of directions for diffusion-encoding gradients. The lack of b = 500 s/mm2 images may slightly affect the accuracy of ADC computation, while the thinner slices may decrease signal-to-noise ratio. However, in the case that DTI is integrated in BTIPs, it is overall reasonable to replace BTIP-compliant DWI with DTI (b = 1,000 s/mm2), as long as diffusion acquisitions are kept consistent in all follow-up scans of a given patient.
4.2. DCE
DCE (“permeability”) perfusion imaging is a dynamic T1-weighted imaging technique that is used in a variety of solid tumors to provide insight into vascular permeability. Although not included in BTIPs, DCE has applications in neuro-oncologic imaging. In the brain, DCE is thought to reflect alterations in the blood-brain barrier integrity (99). DCE-derived metrics such as Ktrans have been employed for differential diagnosis (100), to predict and/or monitor treatment response (101–103), and to distinguish between treatment effects and tumor recurrence (75, 104), similar to DSC perfusion measures of CBV. The QIBA alliance has provided consensus recommendations for brain DCE (https://qibawiki.rsna.org/index.php/Profiles) (105). According to QIBA, DCE should consist of a dynamic 3D T1w SPGR with TE/TR minimal/3–8 ms, FA 10–15° at 3 T (25–35° at 1.5 T), ≤10 s temporal resolution (ideally ≤5 s) with at least 5 dynamics acquired before the bolus injection, acquisition time of ≥5 min, FOV 220–240 mm, matrix 256 × 128–160, slice thickness ≤5 mm. Additionally, pre-injection T1 mapping with the variable FA approach is suggested. As an example, a recent multicenter study employed a 6-minute long DCE sequence with ∼5 s temporal resolution and 2.4 × 2.4 × 2.5 mm3 voxel size (106, 107).
While some research strategies have included both DCE and DSC perfusion to estimate vascular permeability and volume, respectively, DCE requires a separate injection of GBCA in addition to the one needed for DSC. BTIP-compliant protocols that include DCE and DSC can therefore be achieved with two alternative strategies (11). The first strategy (1 + 1 scheme) is to administer a full dose of GBCA while acquiring DCE and then acquire post-contrast T1-weighted images followed by administration of an additional full dose for DSC. An alternative strategy (½ + ½ scheme) would be to acquire DCE with half dose of contrast agent, then DSC with another half dose bolus, and lastly the post-contrast T1-weighted images. While the 1 + 1 scheme provides higher contrast-to-noise ratio and accuracy in perfusion/permeability metrics, double dosage raises concerns for gadolinium deposition in gray matter nuclei (even though little is known about potential clinical implications of such deposition) (108) and for toxicity in patients with chronic kidney disease (109), also considering that brain tumor patients are followed with many longitudinal scans. Both the ½ + ½ and the 1 + 1 schemes are BTIP-compliant, and the choice should be made depending on the institution experience/preference and on the type of GBCA employed, as for instance macrocyclic agents are possibly linked with a very low risk of nephrogenic systemic fibrosis. If the ½ + ½ scheme is preferred, a 30° FA should be set for DSC, which is more adherent to BTIP recommendations, while 60° FA should be preferred in case of full preload injected during DCE (1 + 1 scheme). Finally, it is worth mentioning that some studies proposed to employ dual-echo DSC to perform simultaneous DSC and DCE with a single bolus of GBCA and a single acquisition (87, 110), which is a potential future approach to obtain both DSC and DCE while limiting GBCA doses and reducing acquisition time.
4.3. ASL, spectroscopy, CEST, sodium imaging, and SWI
ASL (arterial spin labeling) is a technique that enables estimation of cerebral blood flow (CBF) by imaging the magnetically labeled inflowing blood (111), and it has been increasingly employed in brain tumor imaging studies as a perfusion technique (112–114). Similarly to DSC-derived CBV, ASL-derived CBF has also been shown to correlate with microvascular density (115). According to current guidelines (116, 117), ASL should be performed with either pseudo-continuous or pulsed labeling, with a post-labeling delay (or inversion time) of 2,000 ms in the clinical adult population, in-plane resolution of 3–4 mm, and slice thickness of 4–8 mm. As discussed in a recent article, exogenous GBCA administration causes significant signal loss in ASL, as GBCA-induced T1 shortening results in a rapid decay in the magnetic labeling of blood spins (118). Therefore, ASL sequences should be integrated in BTIPs before contrast injection.
MR spectroscopy (MRS) techniques are traditionally employed in brain tumor MRI mainly to assess the tissue concentration of creatine, choline, and n-acetylaspartate. More recently, MRS techniques to detect 2HG in gliomas have been optimized in some institutions (119–121). 2HG is a product of mutant IDH, and its detection is not only useful for IDH profiling at diagnosis, but also for the longitudinal evaluation of IDH inhibition (122). MRS sequences may be integrated with BTIPs in LGG clinical trials testing IDH inhibitors, in order to correlate clinical outcomes with longitudinal changes in 2HG. For non-enhancing lesions—e.g., most of IDH-mutant gliomas—MRS can be acquired either before or after GBCA administration, since no significant amount of GBCA is thought to be present in the extravascular extracellular space in such cases. For enhancing lesions, it is debatable whether MRS should be integrated in BTIPs before or after GBCA. In single-voxel MRS, the administration of GBCA allows to more accurately position the voxel on the enhancing component of the lesion, and better avoid sampling cystic or necrotic areas or vasogenic edema. However, distortions in the local magnetic field induced by GBCA susceptibility may potentially affect the accuracy of MRS measurements, particularly in long-TE protocols. Evidence in this regard is inconclusive, as some data advocate for GBCA not affecting the quantification of creatine, choline, and n-acetylaspartate (123), while other findings showed a choline underestimation only when using negatively-charged types of GBCA (e.g., gadoteric acid) (124). Overall, common sense suggests that partial volume effects due to necrotic/cystic/edematous tissue sampling may impact on measurement accuracy more than GBCA effects. Therefore, it is overall reasonable to acquire single-voxel MRS after GBCA administration—as often happens in the clinical practice –, as long as consistency is maintained across subjects and timepoints, and the GBCA type is reported in the article. Conversely, multi-voxel MRS is less impacted by an accurate voxel positioning, and is perhaps more feasible before GBCA.
Amine-weighted CEST (chemical exchange saturation transfer) and APT (amide proton transfer) CEST are techniques that have been proposed to sample tumor acidity (with a contribution to the signal dependent on protein content, especially in case of APT), providing insights into tumor metabolism, with potential applications in monitoring treatment response (125–127). CEST MRI is typically performed at 3 T field strength or higher, and should be integrated in BTIPs before contrast injection for consistency with the common practice.
Sodium MRI has been recently included in imaging protocols in some institutions, as it is a promising technique to study tumor electrolyte homeostasis, with potential insights into tissue biology and susceptibility to treatments (128–130). Sodium imaging can be integrated in BTIPs either before or after contrast injection, since there is evidence that the estimation of total sodium concentration should not be impacted by gadolinium (131).
SWI (susceptibility-weighted imaging) may be helpful in brain tumor imaging at diagnosis. Identifying intra-tumoral susceptibility signals may be helpful for differential diagnosis since they can be present in gliomas due to microhemorrhage or microcalcification, while they are substantially less frequent in PCNSL. Additionally, the evaluation of phase images can differentiate microcalcifications from microhemorrhage, which may aid the molecular prediction of oligodendrogliomas (132). Overall, the role of SWI in treatment response assessment seems marginal at the moment. For the aforementioned applications, SWI should be integrated in BTIPs before contrast agent administration. Post-contrast SWI, on the other hand, may have other applications, such as the evaluation of vascular malformations (133).
5. Future directions and conclusions
BTIPs overall lean towards conventional imaging (T1, T2, and FLAIR) acquisitions being performed with 3D imaging, with the caveat that 3D imaging can be more technically challenging and should be used when yields sufficient image quality. As discussed, 3D images are useful for adjusting the tilt of image planes, for multiplanar reconstructions, and for volumetric assessments of lesion volumes. Whether lesion size should be assessed through linear measurements (unidimensional 1D, or bidimensional 2D) or volumetric segmentations (3D) in clinical trials has been object of debate. Currently, both 2D and 3D assessments are contemplated in RANO 2.0 (18). Some studies showed good agreement between linear and volumetric measurements, and advocated for 2D being non-inferior to 3D when predicting overall survival (134–137). However, as already mentioned, evidence shows that measuring lesion size through 3D segmentations can reduce the inter-observer variability compared to 1D and 2D evaluations (138–144), and have smaller bias and variability for the measurement of nodules (145). As for the impact on response assessment, some studies in slower growing tumor show significant differences in progression-free survival between volumetric and 1D/2D methods (41, 134), and studies on malignant gliomas reported that only volumetric measures of tumor size were predictive of survival (146). Finally, 3D segmentations aid the quantification of tumor size in peculiar scenarios where the disease is considered non-measurable according to RANO-compliant 1D/2D measurements, for instance in the case of rim enhancement surrounding a cyst or surgical cavity. Overall, the current body of literature suggests that 3D measurements for tumor burden quantification is equal or better than 2D measurements. Therefore, adopting 3D measurements for the quantification of tumor burden is advisable, if technically feasible. Currently, the main technical factors limiting the application of volumetric assessments are that tumor segmentations are time-consuming and require dedicated software (often not available on PACS systems). A potential resource to overcome these obstacles and eventually make volumetric assessments more widely feasible will be the use of artificial intelligence (AI) for lesion segmentation, which has the potential to yield automated tumor masks without the need of human intervention (147). One recent study showed that PFS computed from AI segmentations on clinical trials datasets was comparable to the human centralized review read, while the local human read yielded different PFS, possibly due to training and expertise differences in the local institutions (148). Similarly, another study showed how an automatic assessment of RANO (AutoRANO) is feasible through AI (149). As of today, both open access and commercial AI segmentation tools are beginning to become more and more available, with variable performance. A general trend towards 3D acquisitions will contribute to speeding up the process of obtaining more longitudinal brain tumor imaging datasets to train AI, thereby improving their performance, and consequently paving the way for a future use of AI segmentations in clinical trials.
Another aspect that has been encouraged in BTIP articles is the generation of T1-subtraction maps. T1-subtraction maps are overall a more accurate approach to identify the enhancing tissue compared to a separate side-by-side qualitative evaluation of T1-pre and T1-post, and are essential in some otherwise difficult cases. One such case is the identification of subtle degree of enhancement, for instance in tumors treated with anti-angiogenic therapy, for which the use of T1-subtraction maps has been shown to improve the quantification of tumor burden and the predictive value of the radiologic assessment with regards to overall survival (25). Additionally, T1-subtraction maps are helpful to sort out inherent T1-pre hyperintensity due to hemorrhage (in glioblastoma, mostly), melanin (in melanoma BM), or calcification. In this regard, the voxel-wise subtraction is not only able to exclude from the enhancing voxels the ones with inherent T1-hyperintensity but also to capture potential enhancement in areas of inherent T1-hyperintensity, where T1 signal is high before contrast and even higher after contrast. As of today, T1-subtraction maps are obtainable quite easily in neuro-imaging laboratories, and could be generated during the central review of the scans in clinical trials. Conversely, they are more challenging to generate in clinical settings, since the normalization and co-registration of T1-pre and T1-post warranted before voxel-wise subtraction are commonly not feasible on the scanner nor on PACS at this time. A wider use of T1-subtraction maps and further evidence of their advantages may heighten interest towards the development of integrated software tools for their clinical implementation.
As for the efforts towards a better standardization of pulse sequence parameters across institutions, a remarkable advancement may be represented by synthetic MRI (SyMRI), using a technique named quantification of relaxation times and proton density by multi-echo acquisition of a saturation-recovery using turbo spin-echo readout (QRAPMASTER) (150). This technique estimates T2, T1, and PD values voxel-wise by fitting the Bloch equations to a QRAPMASTER SyMRI sequence: T2 values are computed from the multiple echoes acquired, T1 values from the saturation pulses acquired with different delays, and PD values by extrapolating the signal intensity at TE zero (151). This approach not only allows to obtain quantitative voxel-wise maps of T1, T2, and PD, but also to generate T1-, T2-weighted and T2-weighted FLAIR images with arbitrary TE, TR, and TI. This would potentially enable to more easily generate images with uniform pulse sequence parameters from multi-centric acquisitions. SyMRI is already available on some commercial scanners (e.g., “SyntAc” on Siemens, “MAGiC” on GE), and some studies advocate for the accuracy of SyMRI in parameter quantification (152) and for its potential usefulness in gliomas (151). An alternative approach to improve the comparability of images across institutions and manufacturers may be represented by standardization methods applied during post-processing (153), possibly with the aid of AI (154).
Finally, a number of novel approaches have been proposed to accelerate image acquisition with an enhanced exploitation of parallel imaging (155), including parallel imaging with multi-slice techniques (156) and controlled aliasing techniques (157), as well as deep learning reconstruction (158, 159). A promising example in this regard is the wave-CAIPI acceleration method, which has been shown to generate images with quality comparable to conventional acceleration methods in approximately one third of the time (acquisition times: 1min14s for 3D IR-GRE T1, 1min19s for 3D T2-weighted TSE, 2 min for 3D T2-weighted FLAIR, 1min29s for SWI) (160). Advancements in the field of accelerated image acquisition have the potential to reduce the acquisition time for BTIPs, which would alleviate the burden for the patient and improve flexibility in scan planning. Additionally, faster sequences also have the potential of reducing motion artifacts, especially in oncologic patients who cannot always be fully compliant.
In conclusion, imaging protocols complying with BTIPs should be implemented for clinical trials, with some degree of flexibility to accommodate institutional preferences that do not conflict with treatment response assessments (e.g., DTI in place of DWI). For the clinical routine, imaging centers can use BTIPs as suggestions for imaging protocols in brain tumors, keeping in mind that most BTIP recommendations are conceived for standardization and feasibility in multicenter clinical trials, and therefore the single institutions may need to adapt them to their clinical needs. More in general, obtaining standardized imaging datasets in brain tumor patients is not only a need for reliably assessing treatment response in clinical trials, but also a precious resource for future imaging studies which would retrospectively analyze such datasets. When implementing imaging protocols in neuro-oncology, general concepts regarding dosage and timing of GBCA administration, as well as optimal pulse sequence parameters, should be taken into consideration.
Author contributions
FS: Conceptualization, Data curation, Investigation, Methodology, Visualization, Writing – original draft, Writing – review & editing. TK: Conceptualization, Investigation, Visualization, Writing – review & editing. TC: Conceptualization, Supervision, Writing – review & editing. PW: Conceptualization, Supervision, Writing – review & editing. BE: Conceptualization, Investigation, Supervision, Visualization, Writing – review & editing.
Funding
The author(s) declare financial support was received for the research, authorship, and/or publication of this article.
NIH/NCI R01CA270027; NIH/NCI R01CA279984; NIH/NCI P50CA211015; DoD CA220732; National Brain Tumor Society; Sontag Foundation.
Conflict of interest
The authors declare that the research was conducted in the absence of any commercial or financial relationships that could be construed as a potential conflict of interest.
Publisher's note
All claims expressed in this article are solely those of the authors and do not necessarily represent those of their affiliated organizations, or those of the publisher, the editors and the reviewers. Any product that may be evaluated in this article, or claim that may be made by its manufacturer, is not guaranteed or endorsed by the publisher.
References
1. Miller KD, Ostrom QT, Kruchko C, Patil N, Tihan T, Cioffi G, et al. Brain and other central nervous system tumor statistics, 2021. CA Cancer J Clin. (2021) 71:381–406. doi: 10.3322/caac.21693
2. Ostrom QT, Price M, Neff C, Cioffi G, Waite KA, Kruchko C, et al. CBTRUS statistical report: primary brain and other central nervous system tumors diagnosed in the United States in 2015–2019. Neuro Oncol. (2022) 24:v1–v95. doi: 10.1093/neuonc/noac202
3. Vogelbaum MA, Brown PD, Messersmith H, Brastianos PK, Burri S, Cahill D, et al. Treatment for brain metastases: ASCO-SNO-ASTRO guideline. J Clin Oncol Off J Am Soc Clin Oncol. (2022) 40:492–516. doi: 10.1200/JCO.21.02314
4. Grommes C, Rubenstein JL, DeAngelis LM, Ferreri AJM, Batchelor TT. Comprehensive approach to diagnosis and treatment of newly diagnosed primary CNS lymphoma. Neuro Oncol. (2019) 21:296–305. doi: 10.1093/neuonc/noy192
5. Wen PY, Weller M, Lee EQ, Alexander BM, Barnholtz-Sloan JS, Barthel FP, et al. Glioblastoma in adults: a society for neuro-oncology (SNO) and European society of neuro-oncology (EANO) consensus review on current management and future directions. Neuro Oncol. (2020) 22:1073–113. doi: 10.1093/neuonc/noaa106
6. Weller M, van den Bent M, Preusser M, Le Rhun E, Tonn JC, Minniti G, et al. EANO guidelines on the diagnosis and treatment of diffuse gliomas of adulthood. Nat Rev Clin Oncol. (2021) 18:170–86. doi: 10.1038/s41571-020-00447-z
7. Miller JJ, Gonzalez Castro LN, McBrayer S, Weller M, Cloughesy T, Portnow J, et al. Isocitrate dehydrogenase (IDH) mutant gliomas: a society for neuro-oncology (SNO) consensus review on diagnosis, management, and future directions. Neuro Oncol. (2023) 25:4–25. doi: 10.1093/neuonc/noac207
8. Ellingson BM, Bendszus M, Boxerman J, Barboriak D, Erickson BJ, Smits M, et al. Consensus recommendations for a standardized brain tumor imaging protocol in clinical trials. Neuro Oncol. (2015) 17:1188–98. doi: 10.1093/neuonc/nov095
9. Ellingson BM, Wen PY, Chang SM, van den Bent M, Vogelbaum MA, Li G, et al. Objective response rate targets for recurrent glioblastoma clinical trials based on the historic association between objective response rate and median overall survival. Neuro Oncol. (2023) 25:1017–28. doi: 10.1093/neuonc/noad002
10. Ellingson BM, Brown MS, Boxerman JL, Gerstner ER, Kaufmann TJ, Cole PE, et al. Radiographic read paradigms and the roles of the central imaging laboratory in neuro-oncology clinical trials. Neuro Oncol. (2021) 23:189–98. doi: 10.1093/neuonc/noaa253
11. Boxerman JL, Quarles CC, Hu LS, Erickson BJ, Gerstner ER, Smits M, et al. Consensus recommendations for a dynamic susceptibility contrast MRI protocol for use in high-grade gliomas. Neuro Oncol. (2020) 22:1262–75. doi: 10.1093/neuonc/noaa141
12. Kaufmann TJ, Smits M, Boxerman J, Huang R, Barboriak DP, Weller M, et al. Consensus recommendations for a standardized brain tumor imaging protocol for clinical trials in brain metastases. Neuro Oncol. (2020) 22:757–72. doi: 10.1093/neuonc/noaa030
13. Barajas RF, Politi LS, Anzalone N, Schöder H, Fox CP, Boxerman JL, et al. Consensus recommendations for MRI and PET imaging of primary central nervous system lymphoma: guideline statement from the international primary CNS lymphoma collaborative group (IPCG). Neuro Oncol. (2021) 23:1056–71. doi: 10.1093/neuonc/noab020
14. Wen PY, Macdonald DR, Reardon DA, Cloughesy TF, Sorensen AG, Galanis E, et al. Updated response assessment criteria for high-grade gliomas: response assessment in neuro-oncology working group. J Clin Oncol. (2010) 28:1963–72. doi: 10.1200/JCO.2009.26.3541
15. Ellingson BM, Wen PY, Cloughesy TF. Modified criteria for radiographic response assessment in glioblastoma clinical trials. Neurother J Am Soc Exp Neurother. (2017) 14:307–20. doi: 10.1007/s13311-016-0507-6
16. Okada H, Weller M, Huang R, Finocchiaro G, Gilbert MR, Wick W, et al. Immunotherapy response assessment in neuro-oncology: a report of the RANO working group. Lancet Oncol. (2015) 16:e534–42. doi: 10.1016/S1470-2045(15)00088-1
17. van den Bent MJ, Wefel JS, Schiff D, Taphoorn MJB, Jaeckle K, Junck L, et al. Response assessment in neuro-oncology (a report of the RANO group): assessment of outcome in trials of diffuse low-grade gliomas. Lancet Oncol. (2011) 12:583–93. doi: 10.1016/S1470-2045(11)70057-2
18. Wen PY, Van Den Bent MJ, Youssef G, Cloughesy TF, Ellingson BM, Weller M, et al. RANO 2.0: proposal for an update to the response assessment in neuro-oncology (RANO) criteria for high- and low-grade gliomas in adults. J Clin Oncol. (2023) 41:2017. doi: 10.1200/JCO.2023.41.16\_suppl.2017
19. Lin NU, Lee EQ, Aoyama H, Barani IJ, Barboriak DP, Baumert BG, et al. Response assessment criteria for brain metastases: proposal from the RANO group. Lancet Oncol. (2015) 16:e270–8. doi: 10.1016/S1470-2045(15)70057-4
20. Erker C, Tamrazi B, Poussaint TY, Mueller S, Mata-Mbemba D, Franceschi E, et al. Response assessment in paediatric high-grade glioma: recommendations from the response assessment in pediatric neuro-oncology (RAPNO) working group. Lancet Oncol. (2020) 21:e317–29. doi: 10.1016/S1470-2045(20)30173-X
21. Abrey LE, Batchelor TT, Ferreri AJM, Gospodarowicz M, Pulczynski EJ, Zucca E, et al. Report of an international workshop to standardize baseline evaluation and response criteria for primary CNS lymphoma. J Clin Oncol Off J Am Soc Clin Oncol. (2005) 23:5034–43. doi: 10.1200/JCO.2005.13.524
22. Wood JR, Green SB, Shapiro WR. The prognostic importance of tumor size in malignant gliomas: a computed tomographic scan study by the brain tumor cooperative group. J Clin Oncol Off J Am Soc Clin Oncol. (1988) 6:338–43. doi: 10.1200/JCO.1988.6.2.338
23. Lacroix M, Abi-Said D, Fourney DR, Gokaslan ZL, Shi W, DeMonte F, et al. A multivariate analysis of 416 patients with glioblastoma multiforme: prognosis, extent of resection, and survival. J Neurosurg. (2001) 95:190–8. doi: 10.3171/jns.2001.95.2.0190
24. Ramakrishna R, Barber J, Kennedy G, Rizvi A, Goodkin R, Winn RH, et al. Imaging features of invasion and preoperative and postoperative tumor burden in previously untreated glioblastoma: correlation with survival. Surg Neurol Int. (2010) 1:40. doi: 10.4103/2152-7806.68337
25. Ellingson BM, Kim HJ, Woodworth DC, Pope WB, Cloughesy JN, Harris RJ, et al. Recurrent glioblastoma treated with bevacizumab: contrast-enhanced T1-weighted subtraction maps improve tumor delineation and aid prediction of survival in a multicenter clinical trial. Radiology. (2014) 271:200–10. doi: 10.1148/radiol.13131305
26. Ellingson BM, Harris RJ, Woodworth DC, Leu K, Zaw O, Mason WP, et al. Baseline pretreatment contrast enhancing tumor volume including central necrosis is a prognostic factor in recurrent glioblastoma: evidence from single and multicenter trials. Neuro Oncol. (2017) 19:89–98. doi: 10.1093/neuonc/now187
27. Ellingson BM, Abrey LE, Nelson SJ, Kaufmann TJ, Garcia J, Chinot O, et al. Validation of postoperative residual contrast-enhancing tumor volume as an independent prognostic factor for overall survival in newly diagnosed glioblastoma. Neuro Oncol. (2018) 20:1240–50. doi: 10.1093/neuonc/noy053
28. Akeson P, Nordström CH, Holtås S. Time-dependency in brain lesion enhancement with gadodiamide injection. Acta Radiol. (1997) 38:19–24. doi: 10.1080/02841859709171236
29. Rohrer M, Bauer H, Mintorovitch J, Requardt M, Weinmann H-J. Comparison of magnetic properties of MRI contrast media solutions at different magnetic field strengths. Invest Radiol. (2005) 40:715–24. doi: 10.1097/01.rli.0000184756.66360.d3
30. Szomolanyi P, Frenzel T, Noebauer-Huhmann IM, Rohrer M, Trattnig S, Pietsch H, et al. Impact of concentration and dilution of three macrocyclic gadolinium-based contrast agents on MRI signal intensity at 1.5T and 3T and different pulse sequences: results of a phantom study in human plasma. Acta Radiol. (2021) 62:51–7. doi: 10.1177/0284185120915674
31. Furutani K, Harada M, Mawlan M, Nishitani H. Difference in enhancement between spin echo and 3-dimensional fast spoiled gradient recalled acquisition in steady state magnetic resonance imaging of brain metastasis at 3-T magnetic resonance imaging. J Comput Assist Tomogr. (2008) 32:313–9. doi: 10.1097/RCT.0b013e318074fd9d
32. Elster AD. How much contrast is enough?. Dependence of enhancement on field strength and MR pulse sequence. Eur Radiol. (1997) 7(Suppl 5):276–80. doi: 10.1007/pl00006908
33. Suh CH, Jung SC, Kim KW, Pyo J. The detectability of brain metastases using contrast-enhanced spin-echo or gradient-echo images: a systematic review and meta-analysis. J Neurooncol. (2016) 129:363–71. doi: 10.1007/s11060-016-2185-y
34. Nagao E, Yoshiura T, Hiwatashi A, Obara M, Yamashita K, Kamano H, et al. 3D Turbo spin-echo sequence with motion-sensitized driven-equilibrium preparation for detection of brain metastases on 3T MR imaging. AJNR Am J Neuroradiol. (2011) 32:664–70. doi: 10.3174/ajnr.A2343
35. Oh J, Choi SH, Lee E, Shin DJ, Jo SW, Yoo R-E, et al. Application of 3D fast spin-echo T1 black-blood imaging in the diagnosis and prognostic prediction of patients with leptomeningeal carcinomatosis. AJNR Am J Neuroradiol. (2018) 39:1453–9. doi: 10.3174/ajnr.A5721
36. Sommer NN, Pons Lucas R, Coppenrath E, Kooijman H, Galiè F, Hesse N, et al. Contrast-enhanced modified 3D T1-weighted TSE black-blood imaging can improve detection of infectious and neoplastic meningitis. Eur Radiol. (2020) 30:866–76. doi: 10.1007/s00330-019-06475-3
37. Mueller SG, Weiner MW, Thal LJ, Petersen RC, Jack CR, Jagust W, et al. Ways toward an early diagnosis in Alzheimer's Disease: the Alzheimer's disease neuroimaging initiative (ADNI). Alzheimers Dement. (2005) 1:55–66. doi: 10.1016/j.jalz.2005.06.003
38. Mueller SG, Weiner MW, Thal LJ, Petersen RC, Jack C, Jagust W, et al. The Alzheimer's disease neuroimaging initiative. Neuroimaging Clin N Am. (2005) 15:869–77, xi–xii. doi: 10.1016/j.nic.2005.09.008
39. Danieli L, Riccitelli GC, Distefano D, Prodi E, Ventura E, Cianfoni A, et al. Brain tumor-enhancement visualization and morphometric assessment: a comparison of MPRAGE, SPACE, and VIBE MRI techniques. AJNR Am J Neuroradiol. (2019) 40:1140–8. doi: 10.3174/ajnr.A6096
40. Ellingson BM, Lai A, Nguyen HN, Nghiemphu PL, Pope WB, Cloughesy TF. Quantification of nonenhancing tumor burden in gliomas using effective T2 maps derived from dual-Echo turbo spin-echo MRI. Clin Cancer Res an Off J Am Assoc Cancer Res. (2015) 21:4373–83. doi: 10.1158/1078-0432.CCR-14-2862
41. Ellingson BM, Kim GHJ, Brown M, Lee J, Salamon N, Steelman L, et al. Volumetric measurements are preferred in the evaluation of mutant IDH inhibition in non-enhancing diffuse gliomas: evidence from a phase I trial of ivosidenib. Neuro Oncol. (2022) 24:770–8. doi: 10.1093/neuonc/noab256
42. Jain R, Johnson DR, Patel SH, Castillo M, Smits M, van den Bent MJ, et al. “Real world” use of a highly reliable imaging sign: “T2-FLAIR mismatch” for identification of IDH mutant astrocytomas. Neuro Oncol. (2020) 22:936–43. doi: 10.1093/neuonc/noaa041
43. Kinoshita M, Arita H, Takahashi M, Uda T, Fukai J, Ishibashi K, et al. Impact of inversion time for FLAIR acquisition on the T2-FLAIR mismatch detectability for IDH-mutant, non-CODEL astrocytomas. Front Oncol. (2020) 10:596448. doi: 10.3389/fonc.2020.596448
44. Ahn SJ, Taoka T, Moon W-J, Naganawa S. Contrast-enhanced fluid-attenuated inversion recovery in neuroimaging: a narrative review on clinical applications and technical advances. J Magn Reson Imaging. (2022) 56:341–53. doi: 10.1002/jmri.28117
45. Misaki K, Nakada M, Hayashi Y, Tachibana O, Yamashita J, Ueda F, et al. Contrast-enhanced fluid-attenuated inversion recovery MRI is useful to detect the CSF dissemination of glioblastoma. J Comput Assist Tomogr. (2001) 25:953–6. doi: 10.1097/00004728-200111000-00020
46. Ercan N, Gultekin S, Celik H, Tali TE, Oner YA, Erbas G. Diagnostic value of contrast-enhanced fluid-attenuated inversion recovery MR imaging of intracranial metastases. AJNR Am J Neuroradiol. (2004) 25:761–5.15140715
47. Fukuoka H, Hirai T, Okuda T, Shigematsu Y, Sasao A, Kimura E, et al. Comparison of the added value of contrast-enhanced 3D fluid-attenuated inversion recovery and magnetization-prepared rapid acquisition of gradient echo sequences in relation to conventional postcontrast T1-weighted images for the evaluation of leptomenin. AJNR Am J Neuroradiol. (2010) 31:868–73. doi: 10.3174/ajnr.A1937
48. Ahn SJ, Chung T-S, Chang J-H, Lee S-K. The added value of double dose gadolinium enhanced 3D T2 fluid-attenuated inversion recovery for evaluating small brain metastases. Yonsei Med J. (2014) 55:1231–7. doi: 10.3349/ymj.2014.55.5.1231
49. Seong M, Park S, Kim ST, Park SG, Kim YK, Kim H-J, et al. Diagnostic accuracy of MR imaging of patients with leptomeningeal seeding from lung adenocarcinoma based on 2017 RANO proposal: added value of contrast-enhanced 2D axial T2 FLAIR. J Neurooncol. (2020) 149:367–72. doi: 10.1007/s11060-020-03617-2
50. Thust SC, Heiland S, Falini A, Jäger HR, Waldman AD, Sundgren PC, et al. Glioma imaging in Europe: a survey of 220 centres and recommendations for best clinical practice. Eur Radiol. (2018) 28:3306–17. doi: 10.1007/s00330-018-5314-5
51. Manfrini E, Smits M, Thust S, Geiger S, Bendella Z, Petr J, et al. From research to clinical practice: a European neuroradiological survey on quantitative advanced MRI implementation. Eur Radiol. (2021) 31:6334–41. doi: 10.1007/s00330-020-07582-2
52. Sanvito F, Castellano A, Falini A. Advancements in neuroimaging to unravel biological and molecular features of brain tumors. Cancers. (2021) 13:1–25. doi: 10.3390/cancers13030424
53. Le Bihan D. Looking into the functional architecture of the brain with diffusion MRI. Nat Rev Neurosci. (2003) 4:469–80. doi: 10.1038/nrn1119
54. Ellingson BM, Malkin MG, Rand SD, Connelly JM, Quinsey C, LaViolette PS, et al. Validation of functional diffusion maps (fDMs) as a biomarker for human glioma cellularity. J Magn Reson Imaging. (2010) 31:538–48. doi: 10.1002/jmri.22068
55. Leu K, Ott GA, Lai A, Nghiemphu PL, Pope WB, Yong WH, et al. Perfusion and diffusion MRI signatures in histologic and genetic subtypes of WHO grade II–III diffuse gliomas. J Neurooncol. (2017) 134:177–88. doi: 10.1007/s11060-017-2506-9
56. Thust SC, Hassanein S, Bisdas S, Rees JH, Hyare H, Maynard JA, et al. Apparent diffusion coefficient for molecular subtyping of non-gadolinium-enhancing WHO grade II/III glioma: volumetric segmentation versus two-dimensional region of interest analysis. Eur Radiol. (2018) 28:3779–88. doi: 10.1007/s00330-018-5351-0
57. Maynard J, Okuchi S, Wastling S, Al Busaidi A, Almossawi O, Mbatha W, et al. World health organization grade ii/iii glioma molecular status: prediction by mri morphologic features and apparent diffusion coefficient. Radiology. (2020) 296:111–21. doi: 10.1148/radiol.2020191832
58. Kickingereder P, Wiestler B, Sahm F, Heiland S, Roethke M, Schlemmer H-P, et al. Primary central nervous system lymphoma and atypical glioblastoma: multiparametric differentiation by using diffusion-, perfusion-, and susceptibility-weighted MR imaging. Radiology. (2014) 272:843–50. doi: 10.1148/radiol.14132740
59. Patel KS, Yao J, Raymond C, Yong W, Everson R, Liau LM, et al. Decorin expression is associated with predictive diffusion MR phenotypes of anti-VEGF efficacy in glioblastoma. Sci Rep. (2020) 10:14819. doi: 10.1038/s41598-020-71799-w
60. Padhani AR, Liu G, Koh DM, Chenevert TL, Thoeny HC, Takahara T, et al. Diffusion-weighted magnetic resonance imaging as a cancer biomarker: consensus and recommendations. Neoplasia. (2009) 11:102–25. doi: 10.1593/neo.81328
61. Maier SE, Sun Y, Mulkern RV. Diffusion imaging of brain tumors. NMR Biomed. (2010) 23:849–64. doi: 10.1002/nbm.1544
62. Negroni D, Bono R, Soligo E, Longo V, Cossandi C, Carriero A, et al. T1-weighted contrast enhancement, apparent diffusion coefficient, and cerebral-blood-volume changes after glioblastoma resection: mRI within 48 hours vs. Beyond 48 hours. Tomogr. (2023) 9:342–51. doi: 10.3390/tomography9010027
63. Ellingson BM, Kim E, Woodworth DC, Marques H, Boxerman JL, Safriel Y, et al. Diffusion MRI quality control and functional diffusion map results in ACRIN 6677/RTOG 0625: a multicenter, randomized, phase II trial of bevacizumab and chemotherapy in recurrent glioblastoma. Int J Oncol. (2015) 46:1883–92. doi: 10.3892/ijo.2015.2891
64. Shiroishi MS, Castellazzi G, Boxerman JL, D’Amore F, Essig M, Nguyen TB, et al. Principles of T2 *-weighted dynamic susceptibility contrast MRI technique in brain tumor imaging. J Magn Reson Imaging. (2015) 41:296–313. doi: 10.1002/jmri.24648
65. Zhang J, Liu H, Tong H, Wang S, Yang Y, Liu G, et al. Clinical applications of contrast-enhanced perfusion MRI techniques in gliomas: recent advances and current challenges. Contrast Media Mol Imaging. (2017) 2017. doi: 10.1155/2017/7064120
66. Henriksen OM, Del Mar Álvarez-Torres M, Figueiredo P, Hangel G, Keil VC, Nechifor RE, et al. High-grade glioma treatment response monitoring biomarkers: a position statement on the evidence supporting the use of advanced MRI techniques in the clinic, and the latest bench-to-bedside developments. Part 1: perfusion and diffusion techniques. Front Oncol. (2022) 12:810263. doi: 10.3389/fonc.2022.810263
67. Chakhoyan A, Yao J, Leu K, Pope WB, Salamon N, Yong W, et al. Validation of vessel size imaging (VSI) in high-grade human gliomas using magnetic resonance imaging, image-guided biopsies, and quantitative immunohistochemistry. Sci Rep. (2019) 9:2846. doi: 10.1038/s41598-018-37564-w
68. Kickingereder P, Sahm F, Radbruch A, Wick W, Heiland S, Von Deimling A, et al. IDH Mutation status is associated with a distinct hypoxia/angiogenesis transcriptome signature which is non-invasively predictable with rCBV imaging in human glioma. Sci Rep. (2015) 5:1–9. doi: 10.1038/srep16238
69. Chaganti J, Taylor M, Woodford H, Steel T. Differentiation of primary central nervous system lymphoma and high-grade glioma with dynamic susceptibility contrast-derived metrics: pilot study. World Neurosurg. (2021) 151:e979–87. doi: 10.1016/j.wneu.2021.05.026
70. Essig M, Waschkies M, Wenz F, Debus J, Hentrich HR, Knopp MV. Assessment of brain metastases with dynamic susceptibility-weighted contrast-enhanced MR imaging: initial results. Radiology. (2003) 228:193–9. doi: 10.1148/radiol.2281020298
71. Weber M-A, Thilmann C, Lichy MP, Günther M, Delorme S, Zuna I, et al. Assessment of irradiated brain metastases by means of arterial spin-labeling and dynamic susceptibility-weighted contrast-enhanced perfusion MRI: initial results. Invest Radiol. (2004) 39:277–87. doi: 10.1097/01.rli.0000119195.50515.04
72. Cho NS, Hagiwara A, Eldred BSC, Raymond C, Wang C, Sanvito F, et al. Early volumetric, perfusion, and diffusion MRI changes after mutant isocitrate dehydrogenase (IDH) inhibitor treatment in IDH1-mutant gliomas. Neurooncol Adv. (2022) 4:vdac124. doi: 10.1093/noajnl/vdac124
73. Shin KE, Ahn KJ, Choi HS, Jung SL, Kim BS, Jeon SS, et al. DCE And DSC MR perfusion imaging in the differentiation of recurrent tumour from treatment-related changes in patients with glioma. Clin Radiol. (2014) 69:e264–72. doi: 10.1016/j.crad.2014.01.016
74. Muto M, Frauenfelder G, Senese R, Zeccolini F, Schena E, Giurazza F, et al. Dynamic susceptibility contrast (DSC) perfusion MRI in differential diagnosis between radionecrosis and neoangiogenesis in cerebral metastases using rCBV, rCBF and K2. Radiol Med. (2018) 123:545–52. doi: 10.1007/s11547-018-0866-7
75. Nael K, Bauer AH, Hormigo A, Lemole M, Germano IM, Puig J, et al. Multiparametric MRI for differentiation of radiation necrosis from recurrent tumor in patients with treated glioblastoma. AJR Am J Roentgenol. (2018) 210:18–23. doi: 10.2214/AJR.17.18003
76. Leu K, Boxerman JL, Ellingson BM. Effects of MRI protocol parameters, preload injection dose, fractionation strategies, and leakage correction algorithms on the fidelity of dynamic-susceptibility contrast MRI estimates of relative cerebral blood volume in gliomas. AJNR Am J Neuroradiol. (2017) 38:478–84. doi: 10.3174/ajnr.A5027
77. Quarles CC, Gochberg DF, Gore JC, Yankeelov TE. A theoretical framework to model DSC-MRI data acquired in the presence of contrast agent extravasation. Phys Med Biol. (2009) 54:5749–66. doi: 10.1088/0031-9155/54/19/006
78. Leu K, Boxerman JL, Cloughesy TF, Lai A, Nghiemphu PL, Liau LM, et al. Improved leakage correction for single-Echo dynamic susceptibility contrast perfusion MRI estimates of relative cerebral blood volume in high-grade gliomas by accounting for bidirectional contrast agent exchange. AJNR Am J Neuroradiol. (2016) 37:1440–6. doi: 10.3174/ajnr.A4759
79. Mangla R, Kolar B, Zhu T, Zhong J, Almast J, Ekholm S. Percentage signal recovery derived from MR dynamic susceptibility contrast imaging is useful to differentiate common enhancing malignant lesions of the brain. AJNR Am J Neuroradiol. (2011) 32:1004–10. doi: 10.3174/ajnr.A2441
80. Semmineh NB, Xu J, Skinner JT, Xie J, Li H, Ayers G, et al. Assessing tumor cytoarchitecture using multiecho DSC-MRI derived measures of the transverse relaxivity at tracer equilibrium (TRATE). Magn Reson Med. (2015) 74:772–84. doi: 10.1002/mrm.25435
81. Sanvito F, Raymond C, Cho NS, Yao J, Hagiwara A, Orpilla J, et al. Simultaneous quantification of perfusion, permeability, and leakage effects in brain gliomas using dynamic spin-and-gradient-echo echoplanar imaging MRI. Eur Radiol. (2023). doi: 10.1007/s00330-023-10215-z
82. Lee MD, Baird GL, Bell LC, Quarles CC, Boxerman JL. Utility of percentage signal recovery and baseline signal in DSC-MRI optimized for relative CBV measurement for differentiating glioblastoma, lymphoma, metastasis, and meningioma. AJNR Am J Neuroradiol. (2019) 40:1445–50. doi: 10.3174/ajnr.A6153
83. Kuo F, Ng NN, Nagpal S, Pollom EL, Soltys S, Hayden-Gephart M, et al. DSC perfusion MRI-derived fractional tumor burden and relative CBV differentiate tumor progression and radiation necrosis in brain metastases treated with stereotactic radiosurgery. AJNR Am J Neuroradiol. (2022) 43:689–95. doi: 10.3174/ajnr.A7501
84. Mayo ZS, Halima A, Broughman JR, Smile TD, Tom MC, Murphy ES, et al. Radiation necrosis or tumor progression? A review of the radiographic modalities used in the diagnosis of cerebral radiation necrosis. J Neurooncol. (2023) 161:23–31. doi: 10.1007/s11060-022-04225-y
85. Kickingereder P, Brugnara G, Hansen MB, Nowosielski M, Pflüger I, Schell M, et al. Noninvasive characterization of tumor angiogenesis and oxygenation in bevacizumab-treated recurrent glioblastoma by using dynamic susceptibility MRI: secondary analysis of the European organization for research and treatment of cancer 26101 trial. Radiology. (2020) 297:164–75. doi: 10.1148/radiol.2020200978
86. Schmainda KM, Prah MA, Marques H, Kim E, Barboriak DP, Boxerman JL. Value of dynamic contrast perfusion MRI to predict early response to bevacizumab in newly diagnosed glioblastoma: results from ACRIN 6686 multicenter trial. Neuro Oncol. (2021) 23:314–23. doi: 10.1093/neuonc/noaa167
87. Smits M, Bendszus M, Collette S, Postma LA, Dhermain F, Hagenbeek RE, et al. Repeatability and reproducibility of relative cerebral blood volume measurement of recurrent glioma in a multicentre trial setting. Eur J Cancer. (2019) 114:89–96. doi: 10.1016/j.ejca.2019.03.007
88. Castellano A, Cirillo S, Bello L, Riva M, Falini A. Functional MRI for surgery of gliomas. Curr Treat Options Neurol. (2017) 19:34. doi: 10.1007/s11940-017-0469-y
89. Brancato V, Nuzzo S, Tramontano L, Condorelli G, Salvatore M, Cavaliere C. Predicting survival in glioblastoma patients using diffusion MR imaging metrics-A systematic review. Cancers (Basel). (2020) 12(10):2858. doi: 10.3390/cancers12102858
90. Idu A-A, Bogaciu N-S, Ciurea AV. Brain imaging and morphological plasticity in glioblastoma: a literature review. J Med Life. (2023) 16:344–7. doi: 10.25122/jml-2022-0201
91. Bizzi A, Blasi V, Falini A, Ferroli P, Cadioli M, Danesi U, et al. Presurgical functional MR imaging of language and motor functions: validation with intraoperative electrocortical mapping. Radiology. (2008) 248:579–89. doi: 10.1148/radiol.2482071214
92. Zolal A, Sameš M, Burian M, Nováková M, Malucelli A, Hejčl A, et al. The effect of a gadolinium-based contrast agent on diffusion tensor imaging. Eur J Radiol. (2012) 81:1877–82. doi: 10.1016/j.ejrad.2011.04.074
93. Fitzek C, Mentzel HJ, Fitzek S, Sauner D, Kaiser WA, Reichenbach JR. Echoplanar diffusion-weighted MRI with intravenous gadolinium-DTPA. Neuroradiology. (2003) 45:592–7. doi: 10.1007/s00234-003-0965-5
94. Ogura A, Hayakawa K, Miyati T, Maeda F. The effect of susceptibility of gadolinium contrast Media on diffusion-weighted imaging and the apparent diffusion coefficient. Acad Radiol. (2008) 15:867–72. doi: 10.1016/j.acra.2007.12.020
95. Alger JR, Ellingson BM, Ashe-McNalley C, Woodworth DC, Labus JS, Farmer M, et al. Multisite, multimodal neuroimaging of chronic urological pelvic pain: methodology of the MAPP research network. Neuroimage Clin. (2016) 12:65–77. doi: 10.1016/j.nicl.2015.12.009
96. Wang C, Kutch JJ, Labus JS, Yang CC, Harris RE, Mayer EA, et al. Reproducible microstructural changes in the brain associated with the presence and severity of urologic chronic pelvic pain syndrome (UCPPS): a 3-year longitudinal diffusion tensor imaging study from the MAPP network. J Pain. (2023) 24:627–42. doi: 10.1016/j.jpain.2022.11.008
97. Zhou X, Sakaie KE, Debbins JP, Narayanan S, Fox RJ, Lowe MJ. Scan-rescan repeatability and cross-scanner comparability of DTI metrics in healthy subjects in the SPRINT-MS multicenter trial. Magn Reson Imaging. (2018) 53:105–11. doi: 10.1016/j.mri.2018.07.011
98. Palacios EM, Yuh EL, Mac Donald CL, Bourla I, Wren-Jarvis J, Sun X, et al. Diffusion tensor imaging reveals elevated diffusivity of white matter microstructure that is independently associated with long-term outcome after mild traumatic brain injury: a TRACK-TBI study. J Neurotrauma. (2022) 39:1318–28. doi: 10.1089/neu.2021.0408
99. O’Connor JPB, Tofts PS, Miles KA, Parkes LM, Thompson G, Jackson A. Dynamic contrast-enhanced imaging techniques: cT and MRI. Br J Radiol. (2011) 84(Spec No 2):S112–20. doi: 10.1259/bjr/55166688
100. Kickingereder P, Sahm F, Wiestler B, Roethke M, Heiland S, Schlemmer H-P, et al. Evaluation of microvascular permeability with dynamic contrast-enhanced MRI for the differentiation of primary CNS lymphoma and glioblastoma: radiologic-pathologic correlation. AJNR Am J Neuroradiol. (2014) 35:1503–8. doi: 10.3174/ajnr.A3915
101. Almeida-Freitas DB, Pinho MC, Otaduy MCG, Braga HF, Meira-Freitas D, da Costa Leite C. Assessment of irradiated brain metastases using dynamic contrast-enhanced magnetic resonance imaging. Neuroradiology. (2014) 56:437–43. doi: 10.1007/s00234-014-1344-0
102. Jakubovic R, Sahgal A, Soliman H, Milwid R, Zhang L, Eilaghi A, et al. Magnetic resonance imaging-based tumour perfusion parameters are biomarkers predicting response after radiation to brain metastases. Clin Oncol (R Coll Radiol). (2014) 26:704–12. doi: 10.1016/j.clon.2014.06.010
103. Song J, Kadaba P, Kravitz A, Hormigo A, Friedman J, Belani P, et al. Multiparametric MRI for early identification of therapeutic response in recurrent glioblastoma treated with immune checkpoint inhibitors. Neuro Oncol. (2020) 22:1658–66. doi: 10.1093/neuonc/noaa066
104. Morabito R, Alafaci C, Pergolizzi S, Pontoriero A, Iati’ G, Bonanno L, et al. DCE And DSC perfusion MRI diagnostic accuracy in the follow-up of primary and metastatic intra-axial brain tumors treated by radiosurgery with cyberknife. Radiat Oncol. (2019) 14:65. doi: 10.1186/s13014-019-1271-7
105. Shukla-Dave A, Obuchowski NA, Chenevert TL, Jambawalikar S, Schwartz LH, Malyarenko D, et al. Quantitative imaging biomarkers alliance (QIBA) recommendations for improved precision of DWI and DCE-MRI derived biomarkers in multicenter oncology trials. J Magn Reson Imaging. (2019) 49:e101–21. doi: 10.1002/jmri.26518
106. Santarosa C, Castellano A, Conte GM, Cadioli M, Iadanza A, Terreni MR, et al. Dynamic contrast-enhanced and dynamic susceptibility contrast perfusion MR imaging for glioma grading: preliminary comparison of vessel compartment and permeability parameters using hotspot and histogram analysis. Eur J Radiol. (2016) 85:1147–56. doi: 10.1016/j.ejrad.2016.03.020
107. Anzalone N, Castellano A, Cadioli M, Conte GM, Cuccarini V, Bizzi A, et al. Brain gliomas: multicenter standardized assessment of dynamic contrast-enhanced and dynamic susceptibility contrast MR images. Radiology. (2018) 287:933–43. doi: 10.1148/radiol.2017170362
108. Gulani V, Calamante F, Shellock FG, Kanal E, Reeder SB. Gadolinium deposition in the brain: summary of evidence and recommendations. Lancet Neurol. (2017) 16:564–70. doi: 10.1016/S1474-4422(17)30158-8
109. Weinreb JC, Rodby RA, Yee J, Wang CL, Fine D, McDonald RJ, et al. Use of intravenous gadolinium-based contrast Media in patients with kidney disease: consensus statements from the American college of radiology and the national kidney foundation. Radiology. (2021) 298:28–35. doi: 10.1148/radiol.2020202903
110. Stokes AM, Bergamino M, Alhilali L, Hu LS, Karis JP, Baxter LC, et al. Evaluation of single bolus, dual-echo dynamic susceptibility contrast MRI protocols in brain tumor patients. J Cereb Blood Flow Metab Off J Int Soc Cereb Blood Flow Metab. (2021) 41:3378–90. doi: 10.1177/0271678X211039597
111. Grade M, Hernandez Tamames JA, Pizzini FB, Achten E, Golay X, Smits M. A neuroradiologist's guide to arterial spin labeling MRI in clinical practice. Neuroradiology. (2015) 57:1181–202. doi: 10.1007/s00234-015-1571-z
112. Subashi E, Cordero FJ, Halvorson KG, Qi Y, Nouls JC, Becher OJ, et al. Tumor location, but not H3.3K27M, significantly influences the blood–brain-barrier permeability in a genetic mouse model of pediatric high-grade glioma. J Neurooncol. (2016) 126:243–51. doi: 10.1007/s11060-015-1969-9
113. Yoo RE, Yun TJ, Hwang I, Hong EK, Kang KM, Choi SH, et al. Arterial spin labeling perfusion-weighted imaging aids in prediction of molecular biomarkers and survival in glioblastomas. Eur Radiol. (2020) 30:1202–11. doi: 10.1007/s00330-019-06379-2
114. Luna LP, Ahmed A, Daftaribesheli L, Deng F, Intrapiromkul J, Lanzman BA, et al. Arterial spin labeling clinical applications for brain tumors and tumor treatment complications: a comprehensive case-based review. Neuroradiol J. (2023) 36:129–41. doi: 10.1177/19714009221114444
115. Dangouloff-Ros V, Deroulers C, Foissac F, Badoual M, Shotar E, Grévent D, et al. Arterial spin labeling to predict brain tumor grading in children: correlations between histopathologic vascular density and perfusion MR imaging. Radiology. (2016) 281:553–66. doi: 10.1148/radiol.2016152228
116. Alsop DC, Detre JA, Golay X, Günther M, Hendrikse J, Hernandez-Garcia L, et al. Recommended implementation of arterial spin-labeled perfusion mri for clinical applications: a consensus of the ISMRM perfusion study group and the European consortium for ASL in dementia. Magn Reson Med. (2015) 73:102–16. doi: 10.1002/mrm.25197
117. Lindner T, Bolar DS, Achten E, Barkhof F, Bastos-Leite AJ, Detre JA, et al. Current state and guidance on arterial spin labeling perfusion MRI in clinical neuroimaging. Magn Reson Med. (2023) 89:2024–47. doi: 10.1002/mrm.29572
118. Jaganmohan D, Pan S, Kesavadas C, Thomas B. A pictorial review of brain arterial spin labelling artefacts and their potential remedies in clinical studies. Neuroradiol J. (2021) 34:154–68. doi: 10.1177/1971400920977031
119. Choi C, Ganji SK, DeBerardinis RJ, Hatanpaa KJ, Rakheja D, Kovacs Z, et al. 2-hydroxyglutarate detection by magnetic resonance spectroscopy in IDH-mutated patients with gliomas. Nat Med. (2012) 18:624–9. doi: 10.1038/nm.2682
120. Branzoli F, Di Stefano AL, Capelle L, Ottolenghi C, Valabrègue R, Deelchand DK, et al. Highly specific determination of IDH status using edited in vivo magnetic resonance spectroscopy. Neuro Oncol. (2018) 20:907–16. doi: 10.1093/neuonc/nox214
121. Nguyen TB, Melkus G, Taccone M, Moldovan ID, Ghinda D, Gotfrit R, et al. Preoperative determination of isocitrate dehydrogenase mutation in gliomas using spectral editing MRS: a prospective study. J Magn Reson Imaging. (2021) 53(2):416–26. doi: 10.1002/jmri.27366
122. Andronesi OC, Arrillaga-Romany IC, Ly KI, Bogner W, Ratai EM, Reitz K, et al. Pharmacodynamics of mutant-IDH1 inhibitors in glioma patients probed by in vivo 3D MRS imaging of 2-hydroxyglutarate. Nat Commun. (2018) 9(1):1474. doi: 10.1038/s41467-018-03905-6
123. Smith JK, Kwock L, Castillo M. Effects of contrast material on single-volume proton MR spectroscopy. AJNR Am J Neuroradiol. (2000) 21:1084–9.10871019
124. Lenkinski RE, Wang X, Elian M, Goldberg SN. Interaction of gadolinium-based MR contrast agents with choline: implications for MR spectroscopy (MRS) of the breast. Magn Reson Med. (2009) 61:1286–92. doi: 10.1002/mrm.21937
125. Zhou J, Heo H-Y, Knutsson L, van Zijl PCM, Jiang S. APT-weighted MRI: techniques, current neuro applications, and challenging issues. J Magn Reson Imaging. (2019) 50:347–64. doi: 10.1002/jmri.26645
126. Booth TC, Wiegers EC, Warnert EAH, Schmainda KM, Riemer F, Nechifor RE, et al. High-grade glioma treatment response monitoring biomarkers: a position statement on the evidence supporting the use of advanced MRI techniques in the clinic, and the latest bench-to-bedside developments. Part 2: spectroscopy, chemical exchange saturation. Front Oncol. (2021) 11:811425. doi: 10.3389/fonc.2021.811425
127. Cho NS, Hagiwara A, Yao J, Nathanson DA, Prins RM, Wang C, et al. Amine-weighted chemical exchange saturation transfer magnetic resonance imaging in brain tumors. NMR Biomed. (2023) 36:e4785. doi: 10.1002/nbm.4785
128. Madelin G, Lee J-S, Regatte RR, Jerschow A. Sodium MRI: methods and applications. Prog Nucl Magn Reson Spectrosc. (2014) 79:14–47. doi: 10.1016/j.pnmrs.2014.02.001
129. Hagiwara A, Bydder M, Oughourlian TC, Yao J, Salamon N, Jahan R, et al. Sodium MR neuroimaging. AJNR Am J Neuroradiol. (2021) 42:1920–6. doi: 10.3174/ajnr.A7261
130. Cho NS, Sanvito F, Thakuria S, Wang C, Hagiwara A, Nagaraj R, et al. Multi-nuclear sodium, diffusion, and perfusion MRI in human gliomas. J Neurooncol. (2023) 163(2):417–27. doi: 10.1007/s11060-023-04363-x
131. Paschke NK, Neumann W, Uhrig T, Winkler M, Neumaier-Probst E, Fatar M, et al. Influence of gadolinium-based contrast agents on tissue sodium quantification in sodium magnetic resonance imaging. Invest Radiol. (2018) 53:555–62. doi: 10.1097/RLI.0000000000000487
132. Haller S, Haacke EM, Thurnher MM, Barkhof F. Susceptibility-weighted imaging: technical essentials and clinical neurologic applications. Radiology. (2021) 299:3–26. doi: 10.1148/radiol.2021203071
133. Jagadeesan BD, Delgado Almandoz JE, Benzinger TLS, Moran CJ. Postcontrast susceptibility-weighted imaging: a novel technique for the detection of arteriovenous shunting in vascular malformations of the brain. Stroke. (2011) 42:3127–31. doi: 10.1161/STROKEAHA.111.623587
134. Warren KE, Patronas N, Aikin AA, Albert PS, Balis FM. Comparison of one-, two-, and three-dimensional measurements of childhood brain tumors. J Natl Cancer Inst. (2001) 93:1401–5. doi: 10.1093/jnci/93.18.1401
135. Galanis E, Buckner JC, Maurer MJ, Sykora R, Castillo R, Ballman KV, et al. Validation of neuroradiologic response assessment in gliomas: measurement by RECIST, two-dimensional, computer-assisted tumor area, and computer-assisted tumor volume methods. Neuro Oncol. (2006) 8:156–65. doi: 10.1215/15228517-2005-005
136. Shah GD, Kesari S, Xu R, Batchelor TT, O’Neill AM, Hochberg FH, et al. Comparison of linear and volumetric criteria in assessing tumor response in adult high-grade gliomas. Neuro Oncol. (2006) 8:38–46. doi: 10.1215/S1522851705000529
137. Gahrmann R, van den Bent M, van der Holt B, Vernhout RM, Taal W, Vos M, et al. Comparison of 2D (RANO) and volumetric methods for assessment of recurrent glioblastoma treated with bevacizumab-a report from the BELOB trial. Neuro Oncol. (2017) 19:853–61. doi: 10.1093/neuonc/now311
138. Lavin PT, Flowerdew G. Studies in variation associated with the measurement of solid tumors. Cancer. (1980) 46:1286–90. doi: 10.1002/1097-0142(19800901)46:5<1286::aid-cncr2820460533>3.0.co;2-f7214310
139. Warr D, McKinney S, Tannock I. Influence of measurement error on assessment of response to anticancer chemotherapy: proposal for new criteria of tumor response. J Clin Oncol Off J Am Soc Clin Oncol. (1984) 2:1040–6. doi: 10.1200/JCO.1984.2.9.1040
140. Quoix E, Wolkove N, Hanley J, Kreisman H. Problems in radiographic estimation of response to chemotherapy and radiotherapy in small cell lung cancer. Cancer. (1988) 62:489–93. doi: 10.1002/1097-0142(19880801)62:3<489::aid-cncr2820620308>3.0.co;2-e2839281
141. Hopper KD, Kasales CJ, Van Slyke MA, Schwartz TA, TenHave TR, Jozefiak JA. Analysis of interobserver and intraobserver variability in CT tumor measurements. AJR Am J Roentgenol. (1996) 167:851–4. doi: 10.2214/ajr.167.4.8819370
142. Thiesse P, Ollivier L, Di Stefano-Louineau D, Négrier S, Savary J, Pignard K, et al. Response rate accuracy in oncology trials: reasons for interobserver variability. Groupe français d’Immunothérapie of the fédération nationale des centres de lutte contre le cancer. J Clin Oncol Off J Am Soc Clin Oncol. (1997) 15:3507–14. doi: 10.1200/JCO.1997.15.12.3507
143. D’Arco F, O’Hare P, Dashti F, Lassaletta A, Loka T, Tabori U, et al. Volumetric assessment of tumor size changes in pediatric low-grade gliomas: feasibility and comparison with linear measurements. Neuroradiology. (2018) 60:427–36. doi: 10.1007/s00234-018-1979-3
144. Berntsen EM, Stensjøen AL, Langlo MS, Simonsen SQ, Christensen P, Moholdt VA, et al. Volumetric segmentation of glioblastoma progression compared to bidimensional products and clinical radiological reports. Acta Neurochir. (2020) 162:379–87. doi: 10.1007/s00701-019-04110-0
145. Petrick N, Kim HJG, Clunie D, Borradaile K, Ford R, Zeng R, et al. Comparison of 1D, 2D, and 3D nodule sizing methods by radiologists for spherical and complex nodules on thoracic CT phantom images. Acad Radiol. (2014) 21:30–40. doi: 10.1016/j.acra.2013.09.020
146. Dempsey MF, Condon BR, Hadley DM. Measurement of tumor “size” in recurrent malignant glioma: 1D, 2D, or 3D? AJNR Am J Neuroradiol. (2005) 26:770–6.15814919
147. Lotan E, Jain R, Razavian N, Fatterpekar GM, Lui YW. State of the art: machine learning applications in glioma imaging. Am J Roentgenol. (2019) 212:26–37. doi: 10.2214/AJR.18.20218
148. Kickingereder P, Isensee F, Tursunova I, Petersen J, Neuberger U, Bonekamp D, et al. Automated quantitative tumour response assessment of MRI in neuro-oncology with artificial neural networks: a multicentre, retrospective study. Lancet Oncol. (2019) 20:728–40. doi: 10.1016/S1470-2045(19)30098-1
149. Chang K, Beers AL, Bai HX, Brown JM, Ly KI, Li X, et al. Automatic assessment of glioma burden: a deep learning algorithm for fully automated volumetric and bidimensional measurement. Neuro Oncol. (2019) 21:1412–22. doi: 10.1093/neuonc/noz106
150. Warntjes JBM, Leinhard OD, West J, Lundberg P. Rapid magnetic resonance quantification on the brain: optimization for clinical usage. Magn Reson Med. (2008) 60:320–9. doi: 10.1002/mrm.21635
151. Nunez-Gonzalez L, van Garderen KA, Smits M, Jaspers J, Romero AM, Poot DHJ, et al. Pre-contrast MAGiC in treated gliomas: a pilot study of quantitative MRI. Sci Rep. (2022) 12:21820. doi: 10.1038/s41598-022-24276-5
152. Krauss W, Gunnarsson M, Andersson T, Thunberg P. Accuracy and reproducibility of a quantitative magnetic resonance imaging method for concurrent measurements of tissue relaxation times and proton density. Magn Reson Imaging. (2015) 33:584–91. doi: 10.1016/j.mri.2015.02.013
153. Da-Ano R, Visvikis D, Hatt M. Harmonization strategies for multicenter radiomics investigations. Phys Med Biol. (2020) 65:24TR02. doi: 10.1088/1361-6560/aba798
154. Wen G, Shim V, Holdsworth SJ, Fernandez J, Qiao M, Kasabov N, et al. Machine learning for brain MRI data harmonisation: a systematic review. Bioengineering (Basel). (2023) 10(4):397. doi: 10.3390/bioengineering10040397
155. Deshmane A, Gulani V, Griswold MA, Seiberlich N. Parallel MR imaging. J Magn Reson Imaging. (2012) 36:55–72. doi: 10.1002/jmri.23639
156. Barth M, Breuer F, Koopmans PJ, Norris DG, Poser BA. Simultaneous multislice (SMS) imaging techniques. Magn Reson Med. (2016) 75:63–81. doi: 10.1002/mrm.25897
157. Bilgic B, Gagoski BA, Cauley SF, Fan AP, Polimeni JR, Grant PE, et al. Wave-CAIPI for highly accelerated 3D imaging. Magn Reson Med. (2015) 73:2152–62. doi: 10.1002/mrm.25347
158. Radmanesh A, Muckley MJ, Murrell T, Lindsey E, Sriram A, Knoll F, et al. Exploring the acceleration limits of deep learning variational network-based two-dimensional brain MRI. Radiol. Artif Intell. (2022) 4:e210313. doi: 10.1148/ryai.210313
159. Estler A, Hauser T-K, Mengel A, Brunnée M, Zerweck L, Richter V, et al. Deep learning accelerated image reconstruction of fluid-attenuated inversion recovery sequence in brain imaging: reduction of acquisition time and improvement of image quality. Acad Radiol. (2023). doi: 10.1016/j.acra.2023.05.010
Keywords: brain tumors, neuro-oncology, clinical trials, brain tumor imaging protocols, BTIP, treatment response assessment, RANO criteria
Citation: Sanvito F, Kaufmann TJ, Cloughesy TF, Wen PY and Ellingson BM (2023) Standardized brain tumor imaging protocols for clinical trials: current recommendations and tips for integration. Front. Radiol. 3:1267615. doi: 10.3389/fradi.2023.1267615
Received: 26 July 2023; Accepted: 24 November 2023;
Published: 13 December 2023.
Edited by:
Sotirios Bisdas, University College London, United KingdomReviewed by:
Thomas Booth, King’s College London, United KingdomAgostino Tessitore, University of Messina, Italy
© 2023 Sanvito, Kaufmann, Cloughesy, Wen and Ellingson. This is an open-access article distributed under the terms of the Creative Commons Attribution License (CC BY). The use, distribution or reproduction in other forums is permitted, provided the original author(s) and the copyright owner(s) are credited and that the original publication in this journal is cited, in accordance with accepted academic practice. No use, distribution or reproduction is permitted which does not comply with these terms.
*Correspondence: Benjamin M. Ellingson YmVsbGluZ3NvbkBtZWRuZXQudWNsYS5lZHU=