Blockchain-Based Innovations for Population-Based Registries for Rare Neurodegenerative Diseases
- 1Institute of Public Health, Charité – Universitätsmedizin Berlin, Berlin, Germany
- 2Department of Clinical Research in Neurology, Center for Neurodegenerative Diseases and the Aging Brain, University of Bari Aldo Moro, Pia Fondazione Cardinale G. Panico, Tricase, Italy
- 3Department of Basic Medical Sciences, Neuroscience and Sense Organs, University of Bari Aldo Moro, Bari, Italy
Rare diseases are difficult if not impossible to study outside of population-based registries. Particularly in the context of rare neurodegenerative diseases characterized by case heterogeneity, difficult differential diagnosis by specialists, and small numbers of patients, registries make otherwise unfeasible incidence studies cost-effective and manageable. Building up and maintaining such registries is challenging and requires strong, active, and collaborative networks. Centralization around a leading institution provides structure and consistency, but this single-site storage leads to inefficiency and bottlenecks and is prone to failures, attacks, and manipulation. Furthermore, a substantial amount of trust is required between parties sharing data in a traditional registry. Patients are increasingly reluctant to share data in light of regular news reports about healthcare data breaches. Underfunded rare disease specialized centers are also hesitant to exchange with the leading institution out of fear that the low numbers of patients may seek treatment elsewhere. A lack of electronic health records and information system interoperability in certain settings leads to information silos and only further exacerbate the other issues. Blockchain technology may provide unique, innovative solutions to many of these challenges. Specifically, through digital trust and the use of an immutable distributed ledger, automated data transaction processing, guaranteed integrity, and enhanced security, blockchain technology seems to be perfectly suitable to optimize current population-based rare neurodegenerative disease registry construction and maintenance.
Introduction
The establishment and maintenance of a population-based registry is a joint effort of a network of facilities, general practitioners, patients’ associations, and other stakeholders, with the goal of identifying all cases of a disease of interest in a well-defined geographic area and time interval.
Population-based registries are particularly important in the context of rare neurodegenerative disorders (Rooney et al., 2017). These diseases are characterized by clinical heterogeneity, difficult differential diagnosis, unclear etiology, and low incidence and prevalence. Thus, prospective cohort studies investigating such conditions are generally unfeasible; they would require enormous funding, large sample sizes, the involvement of numerous specialized medical personnel in endpoint adjudication, and the proactive application of countermeasures to minimize selective enrollment and attrition (Rooney et al., 2017). However, by combining information about the number of new disease cases identified from the population-based registry in a specified area and time period with information about the population size from administrative data, it is possible to compute measures of incidence for a theoretical “reconstructed cohort” (Logroscino et al., 2020). In this way, population-based registries are the only cost-effective way to estimate the incidence of rare neurodegenerative diseases.
Population-based registries are important tools for studying geographical disease heterogeneity and trends over time. For example, estimates obtained from population-based registries for Amyotrophic Lateral Sclerosis (ALS) showed that ALS incidence varies across the world regions, and this finding informed research hypotheses about the possible etiological factors behind this observed geographical heterogeneity (Logroscino and Piccininni, 2019). Furthermore, registries play an important role in healthcare resource allocation planning (Rooney et al., 2017). For instance, a recent study using data from a population-based registry involving two different provinces indicated that frontotemporal lobar degeneration, a disease generally classified among early-onset dementias, was actually more frequent among the Italian elderly population than expected (Logroscino et al., 2019). Such insights can have important implications for both research and clinical practice.
The use of population-based registries can help reduce the risk of selection bias in data collection. Such registries improve overall representativeness by relying on an active search strategy using distinct referral patterns and multiple sources to collect information about individuals suspected to have the disease of interest (Rooney et al., 2017).
Building a Registry
Though beneficial once established, building a population-based registry for rare neurodegenerative diseases is a labor-intensive task. First, the leading institution determines all available sources of information in the geographical region to detect cases (Rooney et al., 2017). These include the hospital facilities, clinical professionals, general practitioners, patient associations, charities, pre-existing registries, and specialized centers, with whom an individual diagnosed with or suspected to have the disease in the defined geographic area would have contact. This process strongly depends on the size of the area considered, the health system organization, and intrinsic characteristics of the disease. Once all possible referral pathways are identified, the leading institution conducts an awareness campaign to bridge the different data sources and build the registry network.
To increase engagement and awareness of the operators involved, many registries offer training in data collection and recognition of the disease, and actively promote the registry by using invitation letters, advertisements, public events, congresses, and similar initiatives.
In the end, only the individual operators and the contacted institutions willing to join the study will become nodes of the network. In turn, they actively refer all cases identified during the study period that fulfill the diagnostic criteria and have provided written informed consent (Rooney et al., 2017).
By using a standardized, dedicated, structured questionnaire, registry network members can ideally provide information about demographics, clinical history, clinical variables, risk factors, and in settings with appropriate infrastructure, biomarkers.
Network members contribute to data entry tasks using online interfaces, allowing for the anonymized information to be stored in platforms fulfilling data protection standards. Data access and availability is strictly regulated by predetermined access rights (Rooney et al., 2017), and the leading institutions are responsible for data management.
Commonly Encountered Challenges
Bringing together different healthcare providers in a network under the coordination of one or a few leading institutions is extremely challenging. In general, healthcare providers tend to over-interpret laws regulating data protection (Span, 2015) and data sharing is often limited (Ivan, 2016). A prevailing thought is that owning data represents a competitive advantage (Vest and Gamm, 2010; Ivan, 2016). Sharing data with other providers, institutions, or even with the patients themselves, is often perceived as threatening because healthcare providers fear losing patients who seek care from other institutions and have concerns the collected data could be misused (Ivan, 2016; Peterson et al., 2016). These concerns are especially widespread in the field of rare neurodegenerative diseases, in which centers within the same region compete for the care of few patients and struggle to secure adequate funding from the local health system. This high level of competition along with the misconception that medical records belong to the healthcare providers can make potentially important referral sources reluctant to join the registry network.
Unfortunately, in modern healthcare systems, it remains common that information remains exclusively within the system in which it was created. Differences between infrastructures and data organization systems across institutions further contribute to regional data immobility. This problem is often referred as lack of “interoperability,” which represents a major challenge national health infrastructure must overcome (Office of The National Coordinator for Health Information Technology, 2014). Another issue is the lack of use of electronic health records in many settings; relying only on paper documentation hinders data transfer.
These factors lead to the phenomenon of “information silos” observed in healthcare (Ivan, 2016), resulting in isolation and underutilization of data. Information silos are particularly dangerous for population-based registries, which depend on the willingness of individual registry network members to actively participate, trust the other institutions, and share health data in a standardized way via the integrated network.
The classic data flow scheme consists of building a centralized data source administered by the leading institution with compiled data transferred from the nodes of the network. Successful exchange of health data is hindered by interoperability differences between systems, both in terms of data structure and data semantics (Peterson et al., 2016). The centralized data source, itself, as well as the presence and role of the leading institution, may be at the root of many of these problems. First, centralization requires substantial trust in a single institution (Peterson et al., 2016). Aside from the logistical constraints of a single institution administrating the registry data, which are known to lead to bottlenecks and inefficiency (Dubovitskaya et al., 2017), such a centralized organizational scheme introduces a single point of failure in the system that poses a substantial security risk.
The adoption of electronic medical records initiated a new era of progress both in clinical practice and research. Simultaneously, however, this technology made healthcare cybersecurity a growing concern. According to the Department of Health and Human Services’ Office for Civil Rights, the United States had more than 2,500 healthcare data breaches involving more than 500 records between 2009 and 2019. In this period, almost 190 million healthcare records were compromised, which corresponds to almost 60% of the United States population. Hacking has become the leading cause of healthcare data breaches (HIPAA, 2019).
The fact that patients still experience restricted access to their own electronic medical information, exclusively controlled by the care provider, combined with patients’ growing concerns for privacy and security, introduce further complications (Ivan, 2016). This is especially relevant for rare neurodegenerative population-based registries, in which the willingness of both healthcare providers and patients to share data is essential.
For example, the inclusion of patients’ data without informed consent is forbidden by new data protection laws in the European Union (Rooney et al., 2017). Such regulations have been generally viewed as an obstacle for the long-term success of rare neurodegenerative population-based registries in Europe, as they have become increasingly strict in the amount, type, and circumstances of recordable information (Rooney et al., 2017). A similar trend has also been observed in United States’ regulations (Wilson, 2006).
By implementing newly updated data-sharing technologies to ensure the trust of patients in their own information’s security, the increasingly prevalent conflict between high-quality research and data protection can be resolved (Rooney et al., 2017).
Blockchain to the Rescue
Blockchain is the technology behind Bitcoin (Nakamoto, 2008) and all other cryptocurrencies. Since its inception, blockchain technology has been rapidly evolving.
As a decentralized database (or distributed ledger), blockchain allows for the storage of information on assets and transactions in a peer-to-peer computer network. In this way, it is possible to have a shared public registry of ownership that is available to all blockchain network nodes, in which transactions are stored in “blocks” of data that are then linked and secured together in an immutable and unforgeable “chain” through a secure cryptographic system (Nakamoto, 2008; Radanović and Likić, 2018).
The revolutionary potential of blockchain technology lies in its decentralization. In blockchain-based monetary systems, money is not issued by a central authority, ownership of the money is not verified by a central authority, and transactions are not regulated by a central authority. By using strict algorithms and advanced cryptographic techniques, blockchain makes the role of an entrusted central authority that verifies and controls money transfers and ownership obsolete.
Instead, the central authority’s role in the blockchain paradigm is replaced by the network. All transactions are stored on the blockchain after being mathematically validated and confirmed by the nodes in the peer-to-peer blockchain network while cryptography ensures anonymity and security of the stored transactions (Nakamoto, 2008).
The emergence of the first blockchain technology (in the form of Bitcoin) in 2008 was likely catalyzed by the financial crisis, a time during which the mistrust in institutions such as governments, corporations, and banks that are traditionally in charge of managing, securing, and updating the financial ledger, reached its peak.
Healthcare data transactions and financial transactions rely on some common underlying requirements: (1) identifying the actors involved, (2) properly recording transactions, (3) securing transactions against possible alterations, and (4) keeping the transactions stored in a safe, stable, and secure infrastructure (Ivan, 2016). Currently, the majority of healthcare data transactions occur under the mediation of a hospital that represents the central authority (Ivan, 2016).
This framework is also typical in a rare neurodegenerative population-based registry: the leading institution acts as the central authority in managing transfers of data from the nodes to the registry’s central database, and, in turn, the nodes of the registry network act as a central authority in the transfer of data from the patients to the individual registry nodes.
Centralization certainly has positive aspects but also drawbacks, as we have outlined, including reliance on trust, inefficiency, high risk of manipulation, vulnerability to failures and attacks, and information siloing (Ivan, 2016).
Similar to what has previously been done in the financial sector, blockchain technology offers intriguing possibilities to solve these issues affecting registries (Table 1). Specifically, blockchain alleviates the need for trust in a central authority since the blockchain is a distributed ledger, therefore no party or institution controls it (Nakamoto, 2008; Ivan, 2016). The transaction processing is more efficient since it is based on digital trust. The validation is automatic and the assets are transferred directly without intermediaries (Angraal et al., 2017). Once transactions are validated and stored on the blockchain, it is essentially impossible to manipulate them, ensuring immutability, and integrity (Nakamoto, 2008; Linn and Koo, 2016).
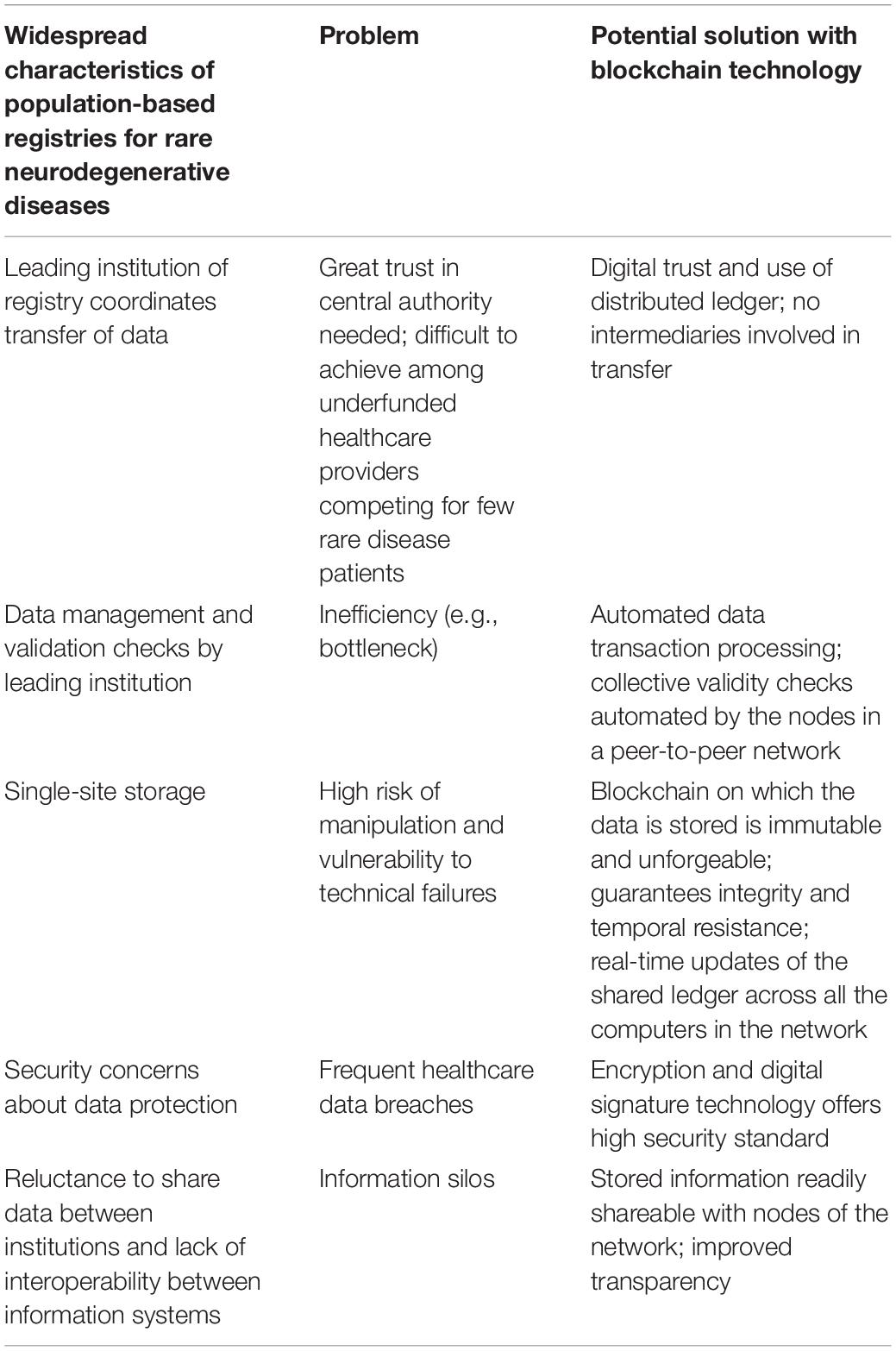
Table 1. Potential innovative solutions for commonly encountered registry challenges using blockchain applications.
Furthermore, the blockchain is available to all computers connected to the network, however, pseudo-anonymity is guaranteed and the content of transactions can be encrypted, which ensures both transparency and security (Ivan, 2016). All of this is possible because blockchain uses a peer-to-peer architecture (Linn and Koo, 2016) that is able to “create an append-only, immutable, and timestamped chain of content” and relies on a public key cryptography system (Ekblaw et al., 2016).
Encrypted transactions are digitally signed, guaranteeing both authenticity and anonymity (Nakamoto, 2008; Linn and Koo, 2016). A group of transactions is stored into a block, which is then linked to the previous one through a cryptographic hash function, ensuring that blocks are added in chronological order (Nakamoto, 2008).
This continuously growing, chronologically ordered list of blocks (“chain”) is readily shared among all the participating nodes of the network (Angraal et al., 2017).
Participating blockchain network nodes also contribute to the process of collectively validating and approving the new transactions contained in newly generated blocks (Nakamoto, 2008; Linn and Koo, 2016). New transactions are automatically validated by the network of nodes using a mechanism to reach consensus, which replaces the need for trust in the central authority to conduct validity checks (Nakamoto, 2008).
Blockchain technology offers a unique opportunity to store healthcare data in a distributed, transparent, temporally resistant, and secure way. This could boost efficiency and incentivize participation in a continuous communication process among different stakeholders.
An example of applying blockchain technology to healthcare data is the platform MedRec (2017). This project, resulting from a collaboration between the MIT Media Lab and the Beth Israel Deaconess Medical Center, aims to give patients the knowledge of who has access to their healthcare data and the power to directly share their data and manage permissions (Angraal et al., 2017).
The fact that patients could digitally move and share all of their own healthcare data records across different healthcare providers in a fast, private, and secure way is attractive both for applications in clinical practice and to improve research efficiency (Radanović and Likić, 2018).
Therefore, we think it is crucial to evaluate further benefits and drawbacks for the use of blockchain specifically in population-based registries. Recent work describing the use and potential for blockchain in healthcare (Yaeger et al., 2019) and in clinical trials (Benchoufi et al., 2019) provided some stimulating thoughts for the present perspective.
Beyond First-Generation Blockchains
Since the emergence of blockchain technology in 2008, many advances and variations have been proposed to handle new challenges and allow for new applications to settings outside of its origins in digital currency. Some of these variations may be particularly suitable for population-based rare neurodegenerative disease registries.
For example, the “permissioned” blockchain, in which only specific members can validate, write, or read data transactions, offers a more restricted structure particularly suitable for healthcare applications (Radanović and Likić, 2018), as it guarantees more privacy and control. On the other hand, a permissioned blockchain architecture would require additional challenges in the implementation and inevitably introduce a certain level of centralization in the network. Indeed, a control layer must be implemented to restrict permissions and access to the ledger, implying a hierarchical architecture built on the basis of centralized decisions. We think a particular type of permissioned blockchain, called “consortium blockchain” or “partially decentralized blockchain,” could be particularly suitable for population-based registries. In this architecture, the consensus process is controlled only by a pre-specified set of nodes (Vitalik Buterin, 2015) that in a population-based registry could correspond to a group of recognized hospitals and health organizations in the region of interest.
Moreover, clinical data could be encrypted and stored off-chain in a “data lake” while the information necessary to access the data could be stored on the blockchain, as in a recently proposed architecture for healthcare aims (Linn and Koo, 2016). Of course, the use of a data lake must be weighed in a trade-off with corresponding losses in advantages of proper data storage on the blockchain (Radanović and Likić, 2018). However, using an appropriate repository could allow registries to store large amounts of data of different sizes, circumventing problems such as scalability (Linn and Koo, 2016) and immutable characteristic of the blockchain, the latter of which is otherwise difficult to reconcile with strict data protection laws (Van Humbeeck, 2017).
Blockchain technology further could ensure direct involvement of patients in the data management process. They could grant other parties access to their own data (e.g., to a different doctor or another clinic) by flexibly specifying permissions and time frames (Ivan, 2016; Linn and Koo, 2016; Yue et al., 2016). This could also apply to consenting and providing their data to a registry.
Bitcoin blockchain uses the highest consumption of CPU power (one-CPU-one-vote) as mechanism to reach consensus among blockchain network nodes (Nakamoto, 2008). This “proof-of-work” system (Nakamoto, 2008), has been criticized, and particularly due to its waste of energy (O’Dwyer and Malone, 2014). Since bitcoin’s inception, other protocols for the agreement on the validity of the transactions stored in the blockchain between the network nodes have been proposed. Relevant to the context of registries, the “proof-of-stake” (QuantumMechanic, 2011) consensus mechanism lends itself well to permissioned blockchains and is lower in costs and energy consumption. This concept has been translated in different ways, ranging from centralized consensus mechanisms, such as the “proof-of-authority” (Wikipedia contributors, 2019), to decentralized and flexible ones, such as the “pure proof-of-stake” (Algorand, 2019).
Additional innovative features, such as the use of smart contract technologies, are also imaginable (Radanović and Likić, 2018). Smart contracts involve a protocol to create self-executing digital contracts between multiple parties. These contracts are written in a programming language and built on a blockchain, requiring no intermediaries. The use of this technology, which in some cases replaces legal contracts and lawyers, could facilitate automatic patient enrollment in population-based disease registries, provided that particular diagnostic conditions can be automatically detected in medical health records managed by the blockchain and previous digital consent was obtained.
Given these innovations, we can conceptualize two levels of distributed ledger architectures for population-based neurodegenerative disease registry applications. First, a lower level, in which a blockchain architecture would be designed and built specifically for the population-based registry use. This would allow the registry nodes to only share data specifically collected for the purposes of the registry, guaranteeing high-quality data collection. Second, a higher level, in which blockchain technology would be used to store data from electronic health records routinely collected in clinical practice that would integrate in real-time with the population-based registry database. Smart contract technology could be employed to automatically flag suspected or diagnosed cases of interest (Yaeger et al., 2019).
Conclusion
Distributed ledger blockchain technology represents a unique set of innovative, technological opportunities well suited to match the decentralized nature of population-based registries for rare neurodegenerative diseases. The applications we have outlined are certainly not limited to the domain of neurodegenerative diseases and are likely also useful for rare disease registries in general, in which traditional centralized digital information storage and dissemination strategies lead to a multitude of challenges. Feasibility of the practical application of blockchain technology in this context should be further explored.
Author Contributions
MP conceptualized and designed the work and drafted the manuscript for intellectual content. JR designed the work and drafted the manuscript for intellectual content. GL and TK designed and supervised the work. All authors contributed to manuscript revision, read, and approved the submitted version.
Conflict of Interest
GL is an Associate Editor of Neuroepidemiology/Karger. He received speaking honoraria from Lilly, GE Healthcare, Lundbeck. TK reports having contributed to an advisory board of CoLucid and a research project funded by Amgen, for which the Charité – Universitätsmedizin Berlin received an unrestricted compensation. He further reports having received honoraria from Lilly, Newsenselab, and Total for providing methodological advice, from Novartis and from Daiichi Sankyo for providing a lecture on neuroepidemiology and research methods, and from the BMJ for editorial services.
The remaining authors declare that the research was conducted in the absence of any commercial or financial relationships that could be construed as a potential conflict of interest.
Funding
JR’s research position is funded by a grant from the Else-Kröner-Fresenius Foundation (www.ekfs.de, GSO/EKFS-17, and granted to TK). GL received funding by Regione Puglia (D.G.R. n. 1284 – 27.05.2015) for the project “Registro Regionale per la SLA, la FTD-P e le patologie affini in Regione Puglia” (SLAPDem register- www.slapdem.it) and by Regione Puglia (D.G.R. n. 2117 – 21.11.2018) and “Tecnomed Puglia per la Medicina di Precisione” (Tecnomed Puglia for Precision Medicine). We further acknowledge support from the German Research Foundation (DFG) and the Open Access Publication Fund of the Charité – Universitätsmedizin Berlin.
Acknowledgments
We thank Christopher Vogel for English language editing assistance.
References
Algorand (2019). Algorand’s Pure Proof-Of-Stake Approach. Available online at: https://www.algorand.com/what-we-do/technology/pure-proof-of-stake/ (accessed November 21, 2019)Google Scholar
Angraal, S., Krumholz, H. M., and Schulz, W. L. (2017). Blockchain technology: applications in health care. Circ. Cardiovasc. Qual. Outcomes 10:e003800. doi: 10.1161/CIRCOUTCOMES.117.003800
Benchoufi, M., Altman, D., and Ravaud, P. (2019). From clinical trials to highly trustable clinical trials: blockchain in clinical trials, a game changer for improving transparency? Front. Blockchain 2:23.
Dubovitskaya, A., Xu, Z., Ryu, S., Schumacher, M., and Wang, F. (2017). Secure and trustable electronic medical records sharing using blockchain. AMIA Annu. Symp. Proc. 2017, 650–659.
Ekblaw, A., Azaria, A., Halamka, J. D., and Lippman, A. (2016). “A Case Study for Blockchain in Healthcare:“MedRec” prototype for electronic health records and medical research data,” in Proceedings of IEEE open & Big Data Conference, Piscataway, NJ: IEEE, 13.
HIPAA Journal (2019). Healthcare Data Breach Statistics. Available online at: https://www.hipaajournal.com/healthcare-data-breach-statistics/ (accessed September 17, 2019).
Ivan, D. (2016). “Moving toward a blockchain-based method for the secure storage of patient records,” in ONC/NIST Use of Blockchain for Healthcare and Research Workshop, Gaithersburg, MA, 1–11.
Linn, L. A., and Koo, M. B. (2016). “Blockchain for health data and its potential use in health it and health care related research,” in ONC/NIST Use of Blockchain for Healthcare and Research Workshop, Gaithersburg, MA, 1–10.
Logroscino, G., Kurth, T., and Piccininni, M. (2020). The reconstructed cohort design: a method to study rare neurodegenerative diseases in population-based settings. Neuroepidemiology 54, 114–122. doi: 10.1159/000502863
Logroscino, G., and Piccininni, M. (2019). Amyotrophic lateral sclerosis descriptive epidemiology: the origin of geographic difference. Neuroepidemiology 52, 93–103. doi: 10.1159/000493386
Logroscino, G., Piccininni, M., Binetti, G., Zecca, C., Turrone, R., Capozzo, R., et al. (2019). Incidence of frontotemporal lobar degeneration in Italy: the salento-brescia registry study. Neurology 92, e2355–e2363. doi: 10.1212/WNL.0000000000007498
MedRec (2017). MedRec. Available online at: https://medrec.media.mit.edu/ (accessed September 17, 2019).
Nakamoto, S. (2008). Bitcoin: A Peer-to-Peer Electronic Cash System. Avaliable at: https://bitcoin.org/bitcoin.pdf (accessed: May 21. 2019).
O’Dwyer, K. J., and Malone, D. (2014). Bitcoin Mining and its Energy Footprint. Kildare: National University of Ireland Maynooth, 280–285.
Office of The National Coordinator for Health Information Technology (2014). Connecting Health And Care For The Nation: A 10-Year Vision To Achieve An Interoperable Health It Infrastructure. Washington, DC: U.S. Department of Health and Human Services.
Peterson, K., Deeduvanu, R., Kanjamala, P., and Boles, K. (2016). “A blockchain-based approach to health information exchange networks,” in Procceding. NIST Workshop Blockchain Healthcare, Gaithersburg, MA, 1–10.
QuantumMechanic (2011). Proof of Stake Instead Of Proof Of Work. Available online at: https://bitcointalk.org/index.php?topic=27787.0 (accessed October 29, 2019).
Radanović, I., and Likić, R. (2018). Opportunities for use of blockchain technology in medicine. Appl. Health Econ. Health Policy 16, 583–590. doi: 10.1007/s40258-018-0412-8
Rooney, J. P. K., Brayne, C., Tobin, K., Logroscino, G., Glymour, M. M., and Hardiman, O. (2017). Benefits, pitfalls, and future design of population-based registers in neurodegenerative disease. Neurology 88, 2321–2329. doi: 10.1212/WNL.0000000000004038
Span, P. (2015). Hipaa’s Use as Code of Silence Often Misinterprets the Law. New York, NY: The New York Times.
Van Humbeeck, A. (2017). The Blockchain-GDPR Paradox. Available online at: https://medium.com/wearetheledger/the-blockchain-gdpr-paradox-fc51e663d047 (accessed November 1, 2019).
Vest, J. R., and Gamm, L. D. (2010). Health information exchange: persistent challenges and new strategies. J. Am. Med. Inform. Assoc. 17, 288–294. doi: 10.1136/jamia.2010.003673
Vitalik Buterin (2015). On Public and Private Blockchains. Available online at: https://blog.ethereum.org/2015/08/07/on-public-and-private-blockchains/ (accessed November 16, 2019).
Wikipedia contributors (2019). Proof of Authority. Wikipedia. Available online at: https://en.wikipedia.org/w/index.php?title=Proof_of_authority&oldid=921049486 (accessed October 29, 2019).
Wilson, J. F. (2006). Health insurance portability and accountability act privacy rule causes ongoing concerns among clinicians and researchers. Ann. Intern. Med. 145, 313–316. doi: 10.7326/0003-4819-145-4-200608150-00019
Yaeger, K., Martini, M., Rasouli, J., and Costa, A. (2019). Emerging Blockchain Technology Solutions for Modern Healthcare Infrastructure. J. Sci. Innov. Med. 2:1.
Keywords: population-based registry, rare diseases, neurodegenerative diseases, blockchain, decentralization
Citation: Piccininni M, Rohmann JL, Logroscino G and Kurth T (2020) Blockchain-Based Innovations for Population-Based Registries for Rare Neurodegenerative Diseases. Front. Blockchain 3:20. doi: 10.3389/fbloc.2020.00020
Received: 21 December 2019; Accepted: 09 April 2020;
Published: 19 May 2020.
Edited by:
Victoria L. Lemieux, The University of British Columbia, CanadaReviewed by:
Erika Beerbower, Independent Researcher, Denver, CO, United StatesJonathan M. Fuchs, The Kidder Street Consulting Group, United States
Copyright © 2020 Piccininni, Rohmann, Logroscino and Kurth. This is an open-access article distributed under the terms of the Creative Commons Attribution License (CC BY). The use, distribution or reproduction in other forums is permitted, provided the original author(s) and the copyright owner(s) are credited and that the original publication in this journal is cited, in accordance with accepted academic practice. No use, distribution or reproduction is permitted which does not comply with these terms.
*Correspondence: Marco Piccininni, marco.piccininni@charite.de