Tether: A Study on Bubble-Networks
- Stake Lab, University of Molise, Campobasso, Italy
Tether is a stablecoin, namely a cryptocurrency associated with an underlying security. Tether provides one of the most relevant ways to buy bitcoins and has been the centre of many controversies. In fact, it has been hypothesized that new tethers are issued without the underlying reserves, and that new massive Tether emissions are the basis of strong speculative movements on the Bitcoin, with consequent bubble effects. In the course of this article, we conduct a Social Network Analysis focused on the Tether transaction graph to identify the main actors that play a leading role on the network and characterize the transaction flow between them. From our analysis, we conclude that 1) the Tether transaction network does not enjoy the Smallworld property, with the robustness and reliability it carries with it; 2) cryptopcurrency exchanges are the nodes with the greatest centrality; 3) even Assortativity is not found, as the subjects who move Tether on a large scale do not give continuity to their presence and operations, therefore do not get a chance to consolidate stable links between them; and 4) among the exchanges, Bitfinex, which has co-ownership and co-administration relationships with the Tether issuer, can be mostly associated with the Rich-gets-Richer property.
1 Introduction
Stablecoins are cryptocurrencies whose values are tied to external assets, such as the US dollar or gold, in order to maintain a stable price. There are also algorithmic stablecoins that, to keep volatility under control, use an algorithm, mostly working under the principle of issuing more coins when the price increases, and buying them from the market when the price falls. Stablecoins should thus in theory combine the best of two possible worlds, namely the disintermediation in the management of payments that characterizes cryptocurrencies such as Bitcoin and Ether and the stability of fiat currencies, such as the Dollar, the Euro and the Yen. If these promises are kept, stablecoins could become more common for buying and selling goods and services than other cryptocurrencies, which are highly volatile due to the strong speculative activities they are subject to and consequently are perceived to be unreliable for this purpose. And they could in turn become viable alternatives to those fiat currencies that are unstable from being linked to national economies affected by instability, hyperinflationary trends, financial defaults and other problems in the same category.
Usually, the issuing entity behind a stablecoin will create a securely stored “reserve” of assets to back it - for example, $ 1 million in a bricks-and-mortar bank to back up one million units of the stablecoin. A digital stablecoin and a real-world asset are thus tied together, with the money in the reserve acting as a collateral for the stablecoin. A user is in this way allowed to redeem one unit of a stablecoin for one unit of the asset supporting it. Based on such general principles, several stablecoin projects were started. Internet giant Facebook launched the Libra project, later renamed Diem1, with great fanfare in 2019 with the idea of creating a stablecoin for universal use in e-commerce; however, the project had to face heavy objections raised by regulators and supervisors, both in Europe and in the United States, and to date is substantially stalled. More successful, if less ambitious, were projects like USDC2, Binance USD3 and Pax4, launched, respectively, by leading exchanges coinbase and Binance, and trust company Paxos, all backed by the US Dollar; they focus on building a safe bridge between traditional banks and cryptocurrencies, whose buyers and sellers can go through the intermediate steps provided by such stablecoins, that are accepted by the banks by virtue of being both dollar-backed and regulated. Terra5 is another promising initiative aimed at creating algorithmic stablecoins.
That said, Tether6 is the stablecoin that came first (its coinage dates back to 2015) and reached the largest market capitalization (having reached the $ 60 billion mark just recently, USDC scoring a distant second place at $ 20 billion), being therefore known as the king of stablecoins. While deserving full credit for pioneering the practice of stablecoins, Tether is, however, far from having an irreproachable reputation of proven remedy against speculative or even illicit practices in the cryptocurrency market. On the contrary, it is suspected to be a deflagrator of speculative trends where large masses of tethers are in turn used to buy large masses of bitcoins so as to artificially push up the price of the latter and consequently generate bubbles that affect the whole world of cryptocurrencies, as the value of altcoins generally depends on that of Bitcoin. As a matter of fact, the issuing company of Tether has never given official evidence of holding enough reserves to cover the quantity of tethers issued 7. Furthermore, there are co-ownership and co-administration relationships between such issuing company and Bitfinex8, one of the largest cryptocurrency exchanges. These suspicions, coupled with the lack of transparency in the management of the currency and in the relationships between the various companies mentioned above, have led to a series of legal actions against diverse physical and legal persons involved in Tether trafficking9.
In this article, we intend to scrutinize the behaviour of the Tether under the magnifying glass of Social Network Analysis (SNA). The object of the investigation is therefore the Tether transaction graph. The basic hypothesis we want to test is the following: if Tether is indeed used essentially for speculative purposes, the subjects involved, even if not necessarily conniving in market manipulations, would be nodes where the Tether is in turn exchanged, directly or indirectly, with other currencies; in other words, they would be exchanges or entities related or cooperating with exchanges. We should also have structural indications that such a network reflects the bubbles consequence of the speculative waves associated with this currency. We can expect that a network associated with a bubble not to be a “good network”, and as such would lack those characteristics of resilience, robustness, efficiency and information reliability that distinguish networks such as the Internet, World-wide Web, effective infrastructures for logistics and energy distribution, successful social and natural ecosystems, etc. On the contrary, we would expect such a network to be as evanescent, labile and unreliable just as bubbles are. But at the same time a network of this type should have easily repeatable patterns, so that it can recreate itself and reappear over time, as the periodicity of bubbles, especially financial ones, teaches us. This appears all the more plausible and applicable in the case of Tether, if it were confirmed that the force behind its various speculative waves is always suspect number 1, namely Bitfinex.
The remainder of this article is structured as follows: Section 2 makes a concise review of works that are related to the topics explored in this article, while Section 3 similarly recalls the relevant SNA concepts as well as illustrates the design principles followed to carry out the study, Section 4 describes its results, and Section 5 describes limitations and future work. Section 6 closes the article by summarizing the main conclusions thus reached.
2 Related Work
There are various methodologies to analyze the connectedness characteristics of financial markets, including cryptocurrencies, drawing both from techniques originating from econometry and quantitative finance, and from the adaptation of SNA approaches. On the SNA side, Kondor et al. (2014) and Maesa et al. (2018) characterize structural properties of the Bitcoin transaction graph to which we will refer in the analysis of the Tether transaction graph. We therefore refer to the discussion of the results reported therein in the following sections of this article. Among the methodologies of financial origin, particularly relevant and pioneering are those illustrated in Diebold and Yilmaz (2012) and Diebold and Yılmaz (2014), in which Vector Auto Regressive (VAR) models are applied to characterize the daily volatility spillovers on US stocks, bonds and commodities, in order to explain the increased volatility as a consequence of the intensification of the global financial crisis that broke out in 2007. VAR models are very powerful tools to describe economic and financial historical series, which can also be used for prediction, and as such have been applied to issues of stablecoin design, as illustrated below. Other studies, focused on Bitcoin, apply Vector Error Correction (VEC) models to describe market dynamics. The work of Pagnottoni and Dimpfl (2019) aims to identify which are the most crucial platforms for Bitcoin in terms of price discovery, thus drawing up, in a period between January 2014 and March 2017, a list of cryptocurrency exchanges, headed by OKcoin and ranked by impact on the price formation process. In turn, Giudici and Pagnottoni (2019) examines the relationships of Bitcoin exchanges during the 2017 price increase and subsequent 2018 decline, performing a connectedness study to assess the effects of spillover and lead-lag in the relationships between exchanges that treat the same asset and consequently identifying Bitfinex and Gemini as leaders among exchanges in terms of return spillover transmission. Another example is the work of Giudici and Pagnottoni (2020), where the return connectedness across the major Bitcoin exchanges is evaluated, as the price can be different depending on the trading venues. As a result, Bitfinex and coinbase are identified as leading exchanges in the price formation process with a significant return spillover to other exchanges. VAR and VEC models can explain effectively the price formation process and quantify the impact the exchanges can have on market dynamics. On the other hand, SNA techniques can give a more general prospective about the transaction flow between the nodes of the network supporting a cryptocurrency, including the exchanges, and account for centrality roles in market dynamics.
Regarding stablecoins and the Tether in particular, for an overview of existing options for stablecoin design see Mita et al. (2019). Giudici et al. (2021) harnesses the power of the aforementioned VAR models to compare stablecoins pegged to baskets of securities with those pegged to major currencies, such as the US dollar. Using the methods of decomposing the volatility spillover with VAR models, the result is that stablecoins pegged to the value of a major currency are more volatile, meaning they are more exposed to price fluctuations A systematic verification of the characteristics of transactions on the Tether is carried out in Griffin and Shams (2020), where it is argued that the Tether is mostly “pushed” into the market by Bitfinex, rather than being “pulled” in response to a real market demand. These analyses corroborate the hypothesis that the use of Tether is essentially speculative, and possibly tainted by dodgy issuance practices, in that the effective availability of collateral reserves to guarantee the tethers placed on the market is under strong doubt, while instead the dollars would enter Bitfinex in reverse direction, i.e., as a result of profits from speculation through the exchange of tethers into bitcoins and the subsequent sale of bitcoins when certain price levels are reached. Bitfinex responded disdainfully to the allegations and arguments of the article, questioning the ability of its authors to understand the “digital token economy”. Quoting verbatim from the link https://www.bitfinex.com/posts/432: ” Simply stated, the digital token economy is driven by larger and more complex factors than the trading practices of any single player. Judging by the reaction to the updated paper, sophisticated and experienced traders in the ecosystem appear to fully understand this concept. To reduce the spike in the bitcoin price in 2017 to such simplistic terms is facile. It is also an insult to the millions of people in our community that believe in the sound principles governing the digital currency economy.” A powerful statement, which paradoxically could be overturned in an accusation of complicity to the thousands of speculative traders currently active in the cryptocurrency arena. As a matter of fact, the results of our analysis are fully compatible with the conclusions reached in the Griffin and Shams (2020) study. On the other hand, this does not exclude that the activities around the Tether, including the bubble effects, be characterized by a systemic complexity greater than the involvement of a single actor, and our analysis can also be extended to this expanded scenario.
As the title of our article states, beyond the specific aspects related to the Tether, ours wants to be a contribution to the study of financial bubble phenomena, for which cryptocurrencies have represented a particularly fertile ground, perhaps as an inevitable downside of the great entrepreneurial and intellectual vivacity that continues to characterize the community that has grown up around them. From this point of view, we are part of a growing line of contributions to financial forensics in the cryptocurrency sector, which also includes the identification of short-term bubbles deriving from Punp-and-Dump schemes, addressed by Li et al. (2021), and Ponzi scams disguised as Decentralized Finance, addressed by Chen et al. (2019).
3 Study Design
Social Network Analysis (SNA) is a methodology based on the assumption that complex structures can be modeled as networks, by exploiting the mathematics of graph theory and viewing the relationships between the entities involved as social interactions. Entities (actors) in networks correspond to nodes, while their interactions are represented as connecting edges. The specificity of SNA is indeed its focus on interaction, with the possibility of identifying properties that can be traced back to different types of interaction, and actors of a totally different nature that nevertheless can fit within the same type of interaction. For example, an energy distribution network and the set of people who gravitate around a certain work context (e.g., Hollywood actors) can share the property of being both Smallworld networks. These properties, that are computed from structural and quantitative input data, provide formally rigorous yet empirically grounded bases for qualitative assessments of aspects such as the robustness and efficiency of the investigated networks which can be harnessed for the purposes of diagnostics, auditing and decision-making. In the context of cryptocurrencies such as the Tether, the nodes are money holders and the edges that connect them are the transactions through which money change hands from one actor to another.
A first broad differentiation of networks is annotative, in that it stems from annotating the connections between nodes as either directed or undirected. In directed networks each edge involves a sender node at one end and a receiver node at the other, while in undirected networks the interactions between connected entities can be in both directions. Moreover, in the case of weighted networks, the strength of the interactions is recorded by assigning a weight to the edges. That said, the properties of a network are based on some relevant parameters and how they relate to each other, namely:
• The size of a network in terms of the number of nodes and edges determines its average degree, that gives a measure of the number of edges with respect to the number of nodes. The term degree refers to the number of connections of a node.
• For undirected networks, we have the average degree of all nodes, while for directed networks we have both the incoming and outgoing degree, where one refers to how many connections enter the node and the other to how many leave it.
• In weighted networks, we have the weighted degree which refers to the sum of the weights of the connections connected to a specific node.
• The clustering coefficient measures the interconnection of a graph: the higher its value, the denser the network. Clustering coefficients are the answer to the question: “What fraction of neighbors of a node are connected to each other?” as they tell us if the neighbors of the node are connected.
• The centrality property of the node is used to measure its importance. There are many measures of centrality, for example Betweenness Centrality hinges on the geometric characteristics of the network. The assumption is that important vertices are bridges over which information flows, so if information spreads through shortest paths, the important nodes must be on many shortest paths.
To carry out the SNA, we used Gephi10, an open source software specifically dedicated to the analysis and visualization of social networks, in combination with R and the igraph11 library, so as to operate on the Tether transaction data obtained through data mining. Moreover, we computed the value of the network statistics using the available Gephi plugins, such as graph average degree. The following sections describe the details of the data mining process and the SNA performed.
3.1 Study Context
TRON (TRX)12 is the blockchain with the largest number of Tether supply (about 50 billion of market cap), followed by the Ethereum blockchain and the Omni Layer (i.e., the Bitcoin blockchain) 13. In our analysis, we considered only the tokens traded on the OmniLayer. A dataset of Tether transactions represents the context of our study. It includes the data provided by Erskine (2018), obtained from Blockspur14, until about February 2018. Also, we mined transaction data from OmniExplorer.com. Using a script from Erskine (2018), the data is summarized for all single transactions by aggregating all transactions by sending and referral address, summing the total amount of Tether exchanged. In the end, we obtained a dataset of aggregated Tether transactions starting from October 2014 to February 15, 2021 (i.e., from block #324140 to #670831). In Figure 1, there are the ten most common transaction types extracted from our dataset. The transaction type specifies in which way the tokens are sent. For example, the Simple Send is a basic type of transaction where a sending address gives a certain amount of coins to a reference address. Transactions of this type can not be used to send Bitcoins. Also, Create Property - Fixed is used to create a new Smart Property where a fixed number of tokens is set. Moreover, there are transaction types related to the Distributed Exchange (i.e., DEX), where the tokens are traded without trusting a centralized website. The description of all the available transaction types is provided by the OmniLayer specification15. For our analysis, we considered only Simple Send transactions. Figure 2 shows the transaction amount over time, starting from 2014 to 2021. To provide visual feedback of our dataset, in Figure 3 we show a plot of a network graph built using the top 100 transactions until March 2020, aggregated by sender and receiver address. An important observation is that we have only considered aggregated Tether transactions having an amount greater than 50,000, mainly to limit the resulting graph’s size and easily calculate the properties of the network. Moreover, using the transaction data, we built different versions of the transaction graph, i.e., the complete network and a snapshot for each year, starting from 2014 to 2021, to analyze the temporal evolution of the Tether network.
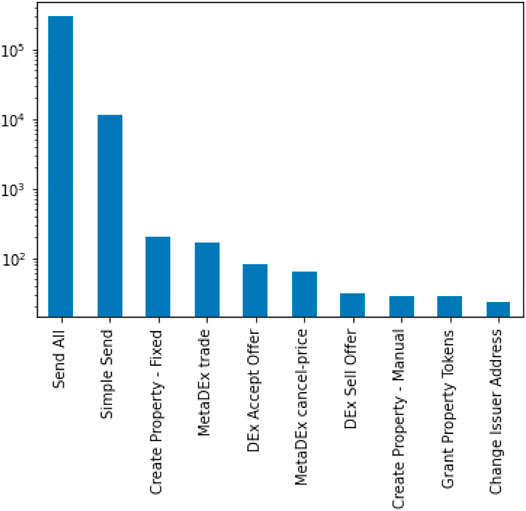
FIGURE 1. The ten most common transaction types from our dataset. Values on the y axis are reported on a logarithmic scale.
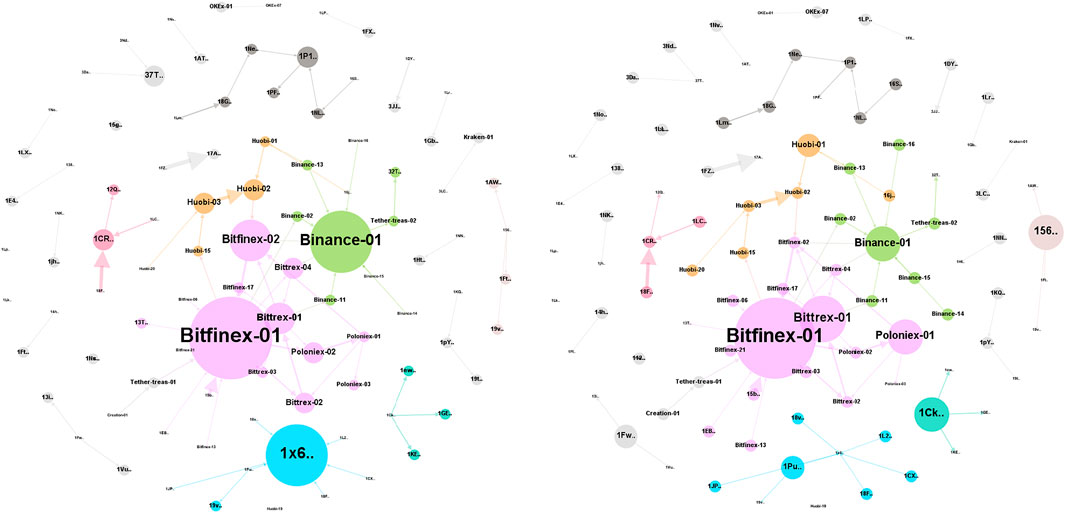
FIGURE 3. Network graph from the top 100 transactions until March 2020. Colors are related to modularity class, and node sizes describe the nodes in-degree (left) and out-degree (right).
3.2 Social Network Properties
In most cases, to compute and extract the relevant properties of the Tether transaction network, we resorted to Gephi. Gephi provides a wide range of algorithms for the extraction of network characteristics that can be used for both visualization and analysis and classification purposes. We have therefore extracted both the topological characteristics and the properties that can be associated with the nodes of the network, such as centrality and authority. The basic interaction with the functionalities offered by Gephi takes place via GUI, which severely limits the possibility of a massively concurrent execution of analyzes and computations. Reason why we first performed a preliminary analysis using the GUI, then we used the Gephi Toolkit, a headless version of Gephi, through which we automated and performed all the analyzes on all the network snapshots as resulting from the data preparation process. In our analysis, the following features provided by the Gephi Toolkit were applied:
• Average Degree and Average Weighted Degree: they measure the average number of connections to a node. For each node, the values of in-degree, out-degree and overall degree are computed. Besides, through the average weighted degree Gephi accounts for the link weights if the network is a weighted network. Using the node degree it is possible to evaluate the influence of a node in its neighborhood.
• Network Diameter: This function computes distance measures (Brandes, 2001) such as network diameter, betweenness centrality, closeness centrality and eccentricity. The network diameter is the shortest path between the two most distant nodes of the network. The betweenness centrality measures how many shortest paths go through a node, thus identifying the nodes that act as bridges between other nodes. The closeness centrality measures how easily reachable is a node. The eccentricity is the distance from a given node to the farthest one in the network.
• HITS: Hyperlink-Induced Topic Search (Kleinberg, 1999), or Hubs and Authorities, is a metric which determines two values for a node: the authority score, and the hub value. The hub value estimates the number of edges outgoing from the node, i.e. how many other nodes the node points to. The authority score measures how many hub nodes point to a given node.
• Modularity: this function identifies sub-regions of the network with a high degree of internal connections. A network with a high modularity score indicates a complex internal structure, where different communities (i.e., sub-graphs) can be identified. The network is thus decomposed into modular communities.
• Page Rank: this algorithm measures the influence of the nodes in the netowrk, assigning a score based on the nodes connections and the connections of their connections (Brin and Page, 1998). It also takes into account link direction and weight.
• Connected Components: this function looks for connected components in the network (Tarjan, 1972). For directed networks, it identifies strongly and weakly connected components. For undirected networks it only identifies weakly connected components.
Moreover, we verify the assortativity (and dis-assortativity) property using the built-in function in the igraph library, not included in Gephi Toolkit. The assortativity coefficient measures if the nodes tend to connect to similar ones. Correlations between nodes of similar degree are often found in the mixing patterns of many observable networks. For example, in the context of human social networks, nodes tend to be connected with other nodes with similar degree values. This tendency is described as assortative mixing, or assortativity. On the other hand, technological and biological networks typically show disassortative mixing (disassortativity), because high degree nodes tend to attach to low degree nodes.
4 Analysis Results
The main objective of our SNA is to identify Tether exchanges having a leading role in the Tether transaction graph and also characterize the transaction flow between them, with Bitfinex in the control room pulling the strings of everything, which in turn would make it plausible that the movements of Tether take place for speculative purposes.
To reduce the calculation time and the size of the network chart, we have considered only transactions with an amount equal to or greater than 50,000 Tether, following the same approach used by Baumann et al. (2014) (consequently, for the year 2014 we have no transaction data in our final dataset). Note that thresholds is set for the list of aggregated transactions, which means that a single transaction item is the result of the sum of all transaction between two entities. For example, all the transactions from entity A to entity B have been added up. We have reported some analyzes performed by Maesa et al. (2018) to make a comparison with Bitcoin. Furthermore, since the Tether transaction network does not provide information that would allow the wallets and their owners to be linked, we resorted to the address list provided by Erskine (2018), where, using the Tether Rich List 16, the addresses of the main exchanges are identified. Erskine investigated secondary nodes in the vicinity of exchanges, where, when the transactions of a secondary node converge in one of the nodes described in the Rich List, it is safe to assume that is a secondary address of a primary exchange. In the next sections we will describe some general network properties (Section 4.1), then we verify the small-world phenomenon in Section 4.2. In Section 4.3 we characterize the most relevant nodes in the Tether transaction network using various centrality measures. Finally, we verify the Rich-get-Richer property and the Assortativity degree of the network.
4.1 Network Overview
To provide a general overview of the Tether transaction network, we computed some basic network measures such as the number of nodes and edges, diameter and community. To perform these analyzes, we used the Modularity and Connected Components functions from Gephi Toolkit. In Table 1 there is a summary of each yearly snapshot of the analyzed Tether transaction network. As for nodes and edges, starting from 2017 they are constantly growing. This means that the amount of tethers traded, also in terms of the size of transactions, increases over the years. Furthermore, the number of communities identified by Gephi has quickly surged since 2017. The value of the diameter of the network has but slightly increased, with greater growth in the year 2019. Compared to the Bitcoin network analysis of (Maesa et al., 2018), our transaction network stays relatively small.
4.2 SmallWorld
A Smallworld, or small-world, (Watts, 2004), is a social network having a high clustering coefficient with short average path lengths. In a social network characterized by this property, each node is just a few steps (as edges to be traversed) away from the other nodes. To assess the small-world phenomenon on the Tether transaction graph, we used the same approach of Maesa et al. (2018). In their case the network diameter is high and is not increasing over time, the average distance (i.e., the average path length) is low and remains approximately constant over time. In our case, the average path length is high and got growing fast too since year 2018. Hence, our network does not resemble a small-world. Figure 4 shows the average path length of the Tether transaction network over time which is computed by Gephi using the methods described in (Brandes, 2001) and (Watts, 2004). The reasons for the absence of the small-world phenomenon are likely manifold, but two seem to stand out as highly plausible as well as relevant and compelling: on the one hand, the lengthening of the tether exchange chains through the insertion of secondary nodes whose low connectivity precipitates the clustering coefficient; on the other hand, the inability of nodes, albeit characterized by a high temporary centrality, to consolidate over time into hubs, which are the pillars over which small-worlds rest, because the only important actor that persists over time is the Bitfinex exchange, notoriously intertwined with the Tether issuer. We address the latter aspect in the next two sections.
4.3 Centrality Analysis
The measurement of the centrality of a network graph helps to discover which are the most relevant nodes in a social network. In our investigation of the structural characteristics of the Tether transaction graph, this aspect is crucial because it allows us to verify whether the most relevant nodes of the network are indeed exchanges, thus confirming their strong impact on the flow of transactions. The Gephi Toolkit implements as algorithms for centrality the approaches proposed by (Brandes, 2001), (Brin and Page, 1998), and (Kleinberg, 1999). We reported as centrality properties: 1) the node authority, which measures if the majority of nodes it connects to are highly connected nodes; 2) the betweenness centrality, which shows the nodes that are bridges for other nodes, measuring how many shortest paths go through a node; 3) the node degree values, to evaluate locally which nodes have an high influence, and 4) the page rank score, used primarily in Google to sort search results by importance, scores nodes based on the number of incoming interactions and relevance across the network of corresponding source nodes. In Table 2 we reported the page rank score over time, where we can conclude that the crypto-exchanges are the main actors in the Tether network. Their influence has increased over the years, as we can see from the wallets of the currently best known exchanges, such as Bitfinex, Binance, Huobi, and Poloniex. Moreover, in Table 3 other centrality measures are reported, regarding the full transaction network, such as node degree, authority and betwenness centrality. Again, exchanges take the lion’s share of both inbound (i.e., in-degree) and outbound (i.e., in out-degree) transactions among the nodes in their neighborhood. There are also some unidentified addresses that have relevance in terms of centrality. In the case of the centrality measures reported in Maesa et al. (2018), exchanges are still among the most influential nodes, but there are other types of nodes such as e-markets that play an equally important role, testifying to the evolution of Bitcoin towards the role of money in all respects, thus going beyond the purely financial and speculative sphere.
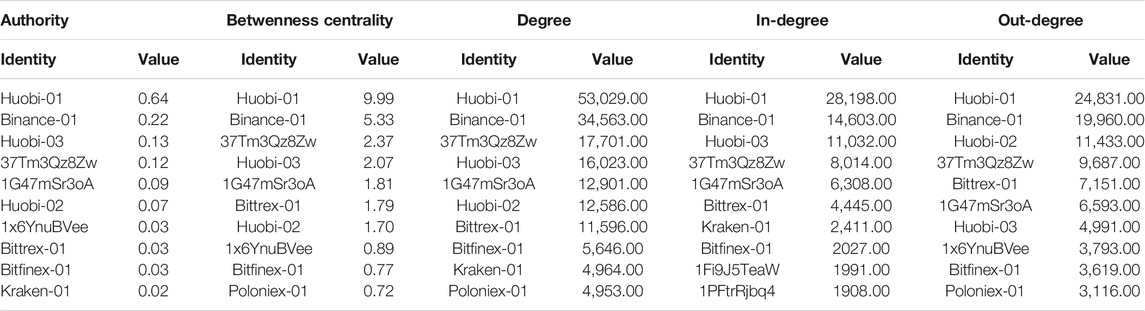
TABLE 3. Top 10 central nodes according to Authority, Betwenness Centrality score, In-degree, Out-degree and Degree.
4.4 Rich-Gets-Richer Property
The Rich-gets-richer property measures the concentration of richness (Kondor et al., 2014). To assess if the richest users stay the richest over time, we simply used the weighted version of the nodes centrality so as to take into account the number of transactions executed by a node owner and also the amount of tokens that remains to the owner. In this way, we used the transaction amounts over time to calculate the subtraction between inbound and outbound amounts. What we can observe in Table 4 is that the richest addresses are different for each network snapshot. The one that appears most over the years is the Bitfinex exchange. This means that Bitfinex maintains a certain ratio of sent and received Tethers over time and thus strengthens the hypothesis that Bitfinex could be the more or less hidden force behind speculative waves involving Tether.

TABLE 4. The difference between in-degree and out-degree to assess the node richness over the time. We have reported only the top 10 nodes in terms of richness for each year.
4.5 Assortativity
An assortative network is a network which has nodes that have the preference to connect to similar nodes (Newman, 2002). The Assortativity degree is expressed as a value between –1 and 1. A network is assortative when on average the high-degree nodes are connected to other nodes with high-degree, and the same will be for low-degree nodes with other low-degree nodes. On the other hand, a network is dis-assortative when the connections between high-degree nodes and low-degree nodes are inverted, i.e., high-degree nodes are connected to lower degree nodes, and low-degree nodes are connected to nodes with high degree. Generally, an assortative network is robust against selective node failure, and for this reason a network’s assortativity can be modified to increase its robustness, as illustrated in Noldus and Van Mieghem (2015). To compute the Assortativity degree for the Tether transactions network, we used the dedicated function from R igraph package17. In Figure 5 we plotted the Assortativity degree over time. We have mostly a negative Assortativity degree for the different transactions network snapshots, hence the network is dis-assortative. In the latest period the Assortativity degree has approached 0, but the current trend, for the year 2021, is that it is decreasing again. The reasons for the disassortativity of the Tether network appear to be more or less the same at the root of the impediment to the consolidation of the small-world phenomenon, namely the fact that hubs and authority nodes are transient and replaced with other nodes of the same type over time, except for Bitfinex. This means that long-term bonds do not develop between nodes with these characteristics, while the secondary nodes are mostly attracted to the primary nodes, and therefore the phenomenon of assortativity never manages to materialize in a lasting form.
5 Limitations and Future Work
We divide the description of future work in two parts: in the first we summarize extensions of the empirical study illustrated here aimed at overcoming its limitations; in the second we outline some further research directions and applications to which our work can contribute.
Our analysis based on SNA techniques has led to some plausible and significant indications on the movements of the Tether that align with indications of other analyzes carried out outside the SNA context. In this way we have also provided a new point of view through which to evaluate the behavior of a type of cryptocurrency, i.e., stablecoins, of increasing diffusion. In the specific case of the Tether, the scenario outlined provides a kind of symptomatic picture, with a diagnosis that warns about possible anomalies, including bubble effects. Given that, in order to analyse social networks, there are consolidated tools, whose use we have illustrated here, and that cryptocurrencies move on public blockchains open to auditing and data acquisition, this type of diagnostics can be easily replicated and exploited for risk management purposes, an aspect that we will dwell on later in this section.
Having said this, our analysis can be suitably deepened by overcoming the current limits in processed data, so as to strengthen the circumstantial indications obtained so far and possibly introduce further relevant elements into the scenario. First, the current dataset needs to include data from other platforms where the Tether is traded, such as TRON (TRX) and BSC. Additionally, a comparison between OmniLayer and Theter’s other blockchains could provide further insight into market dynamics, with focus on centralized exchanges and the flow of transactions between them. Furthermore, transactions occurring on decentralized exchanges (DEX), like UniSwap18 and PancakeSwap19, should also be analyzed, taking into account that in a DEX the transactions do not include an intermediary but happen peer-to-peer, and must consequently be aggregated into nodes of the network. This type of data can be obtained from OmniLayer by picking out transactions of type “DEX”. Many small investor transactions are to be found in DEX while, by excluding all aggregate transactions below 50,000 tethers, we have not treated these data here, being interested in large rather than small movements. However, it may be interesting in the future to look at the flow of transactions between small investors and take a closer view at how the Tether trades in this particular context. Another path that we have not taken here, but can be a source of interesting information, is to build and analyze the graph relating to those transactions in which the Tether is exchanged with other currencies. This can better characterize the way in which the Tether is traded outside its blockchain, by gaining direct visibility on relevant inter-currency correlations and their possible anomalies as reflected on fluctuations of prices. Taken all together, these elements would let us go considerably deeper, so as to give full consideration to Bitfinex’s response to Griffin and Shams (2020) (see Section 2), according to which it cannot be a single subject to pull the strings of the digital token economy, but rather all of its stakeholders. Clearly, our approach is consistent with this assumption from the very beginning, given the systemic nature of the SNA, yet a finer-grained analysis can certainly bring to light further relevant results.
As for the broader and longer-term evolutions of our research, the study of bubble networks, especially in the financial field, is certainly one of the directions to take. Here the bubbleness of the Tether network has been characterized more than anything else by subtraction, that is, by the elements it lacks compared to the types of “good” networks that we know of, such as small-world and assortative networks. But obviously it will also be a question of identifying structural components, however structurally “bad” they may be, that are bubble-specific. We have already mentioned in Section 2 the possible applications in the field of financial forensics; linked to these is risk management, especially for investment portfolios that include cryptocurrencies, which are becoming of increasing interest also for institutional investors. In fact, to complement the evolution of quantitative finance to suit the new markets (as in the methodologies illustrated by Peralta and Zareei (2016), Pagnottoni (2019), Giudici et al. (2020), Resta et al. (2020), Pichler et al. (2021)), a methodological toolkit is in the course of being assembled to analyze social phenomena contextual to financial markets that have been brought about by the advent of the Internet and social media. Social network analysis, together with content analysis (Linton et al., 2017; Phillips and Gorse, 2018) and sentiment analysis (Valencia et al., 2019; Zamuda et al., 2019; Wolk, 2020), can contribute significantly to this toolkit and obviously the study of financial bubbles can provide important indicators to mitigate investment risks by avoiding securities involved in the phenomena in question. The integration of the SNA diagnostics applicable in this regard with the toolkit as well as with the financial methodologies above is therefore an interesting and achievable objective.
6 Conclusion
Summing up, the following indications can be drawn from our analysis of the Tether network: 1) this network (unlike that of Bitcoin) does not enjoy the small-world property with its characteristic traits of robustness and reliability; 2) the nodes with greater centrality are all exchanges, thus adding evidence to the hypothesis of the merely financial and supposedly speculative use of Tether; 3) on the other hand, this is not enough to create an effect of assortativity binding these nodes together, apparently due to the temporal transience of their role which bars the development of long-lasting relationships, since exchanges that are particularly active in a phase of heavy Tether traffic may be replaced at a later stage by other exchanges; 4) to these, however, Bitfinex is an exception, as it maintains a central role over times. These indications, taken together with the shareholding relationship between Bitfinex and the Tether issuer, are circumstantial elements in favor of a significant role played by Bitfinex in the maneuvers on the Tether that might influence the trend in Bitcoin prices.
Of course, all this implies that the Tether speculative network operates and interacts effectively with the Bitcoin network, which is much larger and not merely speculative. as described in Section 5, an investigation of this interaction will be part of future work, as well as an extension of the results obtained here to the general phenomenon of financial bubbles and scams which have so far been generally neglected in the study of social networks. This is presumably because they lack the properties characterizing networks that are considered relevant for society, technology and nature. However, in spite of their apparent evanescence and structural brittleness, bubble-networks are in practice quite frequent and pervasive, thus opening an area of research where there could be much to be discovered.
Last but not least, this study contributes evidence of the double-edged nature of stablecoins, whose complex structure makes them instrumental both in mitigating and in intensifying phenomena of instability and speculative distortion of the cryptocurrency ecosystem. As is to be expected, the outcome of using tools of this kind largely depends on the user’s plans and intentions.
Data Availability Statement
Publicly available datasets were analyzed in this study. This data can be found here: The datasets are public in that have been collected from the public Tether transaction graph.
Author Contributions
GR designed and carried out the data collection and then the analyzes underlying the study. RP provided the initial motivation for the study, and managed the organization of its writing. Both authors contributed in its drafting, revision and finalization.
Conflict of Interest
The authors declare that the research was conducted in the absence of any commercial or financial relationships that could be construed as a potential conflict of interest.
Publisher’s Note
All claims expressed in this article are solely those of the authors and do not necessarily represent those of their affiliated organizations, or those of the publisher, the editors and the reviewers. Any product that may be evaluated in this article, or claim that may be made by its manufacturer, is not guaranteed or endorsed by the publisher.
Footnotes
1Diem - https://www.diem.com/en-us/
2USDC - https://www.coinbase.com/it/usdc
3BUSD - https://www.binance.com/en/busd
4Pax - https://www.paxos.com/pax/
5Terra - https://terra.money/
6Tether - https://tether.to/
7Initially, each tether issued had to be backed by a US dollar, subsequently the Tether issuer relaxed this constraint by referring to a reserve consisting mainly of US dollars plus other collateral, without, in any case, ever providing verifiable evidence on any type of reserve
8The Tether issuer is owned by iFinex, which is owned by DigFinex, which also owns Bitfinex. These companies are all owned and managed by the same people
9As a consequence of one of such legal actions, on February 21, 2021, Bitfinex and the Tether issuer agreed to a settlement of $ 18,500,000 with the General Attorney of New York State regarding allegations over Bitfinex parent iFinex making false statements about the backing of Tether and the movement of hundreds of millions of dollars between the two companies to cover up massive losses by Bitfinex in 2017 and 2018. As part of the agreement, Bitfinex will end all trading activity with New Yorkers. https://ag.ny.gov/press-release/2021/attorney-general-james-ends-virtual-currency-trading-platform-bitfinexs-illegal.
10Gephi - https://gephi.org/
11R igraph - https://igraph.org/r/
12TRON (TRX) - https://tron.network/usdt
13Omni Layer - https://www.omnilayer.org
14Blockspur - http://blockspur.com
15OmniLayer specification - https://github.com/OmniLayer/spec/blob/master/OmniSpecification.adoc
16https://wallet.tether.to/richlist
17igraph Assortativity - https://igraph.org/r/doc/assortativity.html
18UniSwap - https://uniswap.org/
19PancakeSwap - https://pancakeswap.finance/
References
Baumann, A., Fabian, B., and Lischke, M. (2014). “Exploring the Bitcoin Network,” in WEBIST, 369–374.
Brandes, U. (2001). A Faster Algorithm for Betweenness Centrality*. J. Math. Sociol. 25, 163–177. doi:10.1080/0022250x.2001.9990249
Brin, S., and Page, L. (1998). The Anatomy of a Large-Scale Hypertextual Web Search Engine. Comp. networks ISDN Syst. 30, 107–117. doi:10.1016/s0169-7552(98)00110-x
Chen, W., Zheng, Z., Ngai, E. C.-H., Zheng, P., and Zhou, Y. (2019). Exploiting Blockchain Data to Detect Smart Ponzi Schemes on Ethereum. IEEE Access 7, 37575–37586. doi:10.1109/ACCESS.2019.2905769
Di Francesco Maesa, D., Marino, A., and Ricci, L. (2018). Data-driven Analysis of Bitcoin Properties: Exploiting the Users Graph. Int. J. Data Sci. Anal. 6, 63–80. doi:10.1007/s41060-017-0074-x
Diebold, F. X., and Yilmaz, K. (2012). Better to Give Than to Receive: Predictive Directional Measurement of Volatility Spillovers. Int. J. Forecast. 28, 57–66. doi:10.1016/j.ijforecast.2011.02.006
Diebold, F. X., and Yılmaz, K. (2014). On the Network Topology of Variance Decompositions: Measuring the Connectedness of Financial Firms. J. Econom. 182, 119–134. doi:10.1016/j.jeconom.2014.04.012
Erskine, K. (2018). Data Exploration and Visualization of the Tether Blockchain. Available at: https://rpubs.com/keith_erskine/381106.
Giudici, P., Leach, T., and Pagnottoni, P. (2021). Libra or Librae? Basket Based Stablecoins to Mitigate Foreign Exchange Volatility Spillovers. Finance Res. Lett. 7, 102054. doi:10.1016/j.frl.2021.102054
Giudici, P., and Pagnottoni, P. (2019). High Frequency price Change Spillovers in Bitcoin Markets. Risks 7, 111. doi:10.3390/risks7040111
Giudici, P., Pagnottoni, P., and Polinesi, G. (2020). Network Models to Enhance Automated Cryptocurrency Portfolio Management. Front. Artif. Intell. 3, 22. doi:10.3389/frai.2020.00022
Giudici, P., and Pagnottoni, P. (2020). Vector Error Correction Models to Measure Connectedness of Bitcoin Exchange Markets. Appl. Stochastic Models Bus Ind. 36, 95–109. doi:10.1002/asmb.2478
Griffin, J. M., and Shams, A. (2020). Is Bitcoin Really Untethered? J. Finance 75, 1913–1964. doi:10.1111/jofi.12903
Kleinberg, J. M. (1999). Authoritative Sources in a Hyperlinked Environment. J. Acm 46, 604–632. doi:10.1145/324133.324140
Kondor, D., Pósfai, M., Csabai, I., and Vattay, G. (2014). Do the Rich Get Richer? an Empirical Analysis of the Bitcoin Transaction Network. PloS one 9, e86197. doi:10.1371/journal.pone.0086197
Li, T., Shin, D., and Wang, B. (2021). Cryptocurrency Pump-And-Dump Schemes. SSRN Journal. doi:10.2139/ssrn.3267041
Linton, M., Teo, E. G. S., Bommes, E., Chen, C. Y.-H., and Härdle, W. K. (2017). “Dynamic Topic Modelling for Cryptocurrency Community Forums,” in Applied Quantitative Finance. Statistics and Computing. Editors W. Härdle, and C. H. Chen (Springer). doi:10.1007/978-3-662-54486-0_18
Mita, M., Ito, K., Ohsawa, S., and Tanaka, H. (2019). “What Is Stablecoin?: A Survey on price Stabilization Mechanisms for Decentralized Payment Systems,” in 8th International Congress on Advanced Applied Informatics, IIAI-AAI 2019 (Toyama, Japan: IEEE), 60–66. doi:10.1109/IIAI-AAI.2019.00023
Newman, M. E. J. (2002). Assortative Mixing in Networks. Phys. Rev. Lett. 89, 208701. doi:10.1103/physrevlett.89.208701
Noldus, R., and Van Mieghem, P. (2015). Assortativity in Complex Networks. jcomplexnetw 3, 507–542. doi:10.1093/comnet/cnv005
Pagnottoni, P., and Dimpfl, T. (2019). Price Discovery on Bitcoin Markets. Digit Finance 1, 139–161. doi:10.1007/s42521-019-00006-x
Pagnottoni, P. (2019). Neural Network Models for Bitcoin Option Pricing. Front. Artif. Intell. 2, 5. doi:10.3389/frai.2019.00005
Peralta, G., and Zareei, A. (2016). A Network Approach to Portfolio Selection. J. Empirical Finance 38, 157–180. doi:10.1016/j.jempfin.2016.06.003
Phillips, R. C., and Gorse, D. (2018). Mutual-excitation of Cryptocurrency Market Returns and Social media Topics. Clin. Orthop. Relat. Res.. CoRR abs/1806.11093.
Pichler, A., Poledna, S., and Thurner, S. (2021). Systemic Risk-Efficient Asset Allocations: Minimization of Systemic Risk as a Network Optimization Problem. J. Financial Stab. 52, 100809. doi:10.1016/j.jfs.2020.100809
Resta, M., Pagnottoni, P., and De Giuli, M. E. (2020). Technical Analysis on the Bitcoin Market: Trading Opportunities or Investors' Pitfall? Risks 8, 44. doi:10.3390/risks8020044
Tarjan, R. (1972). Depth-first Search and Linear Graph Algorithms. SIAM J. Comput. 1, 146–160. doi:10.1137/0201010
Valencia, F., Gómez-Espinosa, A., and Valdés-Aguirre, B. (2019). Price Movement Prediction of Cryptocurrencies Using Sentiment Analysis and Machine Learning. Entropy 21, 589. doi:10.3390/e21060589
Watts, D. J. (2004). Small Worlds: The Dynamics of Networks between Order and Randomness. Princeton university press.
Wolk, K. (2020). Advanced Social media Sentiment Analysis for Short-Term Cryptocurrency price Prediction. Expert Syst. J. Knowl. Eng. 37, 1. doi:10.1111/exsy.12493
Zamuda, A., Crescimanna, V., Burguillo, J. C., Matos Dias, J., Wegrzyn-Wolska, K., Rached, I., et al. (2019). “Forecasting Cryptocurrency Value by Sentiment Analysis: An Hpc-Oriented Survey of the State-Of-The-Art in the Cloud Era,” in High-Performance Modelling and Simulation for Big Data Applications - Selected Results of the COST Action IC1406 cHiPSet. Editors J. Kolodziej, and H. González-Vélez (Springer), 325–349. doi:10.1007/978-3-030-16272-6_12
Keywords: tether, blockchain, stablecoin, social network analysis, transaction graph, bubble effect
Citation: Rosa G and Pareschi R (2021) Tether: A Study on Bubble-Networks. Front. Blockchain 4:686484. doi: 10.3389/fbloc.2021.686484
Received: 26 March 2021; Accepted: 05 August 2021;
Published: 20 August 2021.
Edited by:
Radoslaw Michalski, Wrocław University of Science and Technology, PolandReviewed by:
Ladislav Kristoufek, Institute of Information Theory and Automation (ASCR), CzechiaPaolo Pagnottoni, University of Pavia, Italy
Copyright © 2021 Rosa and Pareschi. This is an open-access article distributed under the terms of the Creative Commons Attribution License (CC BY). The use, distribution or reproduction in other forums is permitted, provided the original author(s) and the copyright owner(s) are credited and that the original publication in this journal is cited, in accordance with accepted academic practice. No use, distribution or reproduction is permitted which does not comply with these terms.
*Correspondence: Remo Pareschi, remo.pareschi@unimol.it