- School of Engineering, PP Savani University, Surat, India
As the (Covid-19) pandemic spreads, the creativity of the scientific community is thriving while trying to control the situation. They are trying to treat patients viably and work with the almost exhausted medical equipment and staff, while growing new, successful antibodies. Successful screening of SARS-CoV-2 empowers fast and proficient determination of COVID-19 and can relieve the weight on medical care frameworks. Numerous forecast models are being created to comprehend and prognosticate the spread of the pandemic and to stay away from the following wave. But in the coming time, we can be sure that the models would experience the ill effects of a few issues, security being one of them. All the models need to be built in such a way that the investigation task gets successfully conducted without compromising the privacy and security of the patients. To take care of this, we propose a blockchain framework for sharing patients’ personal data or medical reports. A blockchain will take care of the integrity part, but we still need to worry about confidentiality. Therefore, combining a genetic approach with a blockchain seemed like a good idea. A twofold hybrid methodology is proposed in this paper to tackle the issue of confidentiality. The outcomes displayed high entropy accomplishment for the utilized dataset. The sensitivity of the plaintext and ciphertext is also checked and compared with existing approaches which thus demonstrates the security of the proposed approach in the given setting.
Introduction
The World Health Organization (WHO) labeled the COVID-19 pandemic a reason for worrying on January 30, 2020, and requested for the severity of the health emergency to be raised. Following that, the governments of the bulk of nations took a series of stringent measures to contain the pandemic’s spread inside their respective territories. Many nations have put the WHO recommendations for identifying and isolating suspected COVID-19 patients into practice. Despite this, the pandemic has expanded rapidly, across over 197 million individuals affected and over 4 million deaths (Ibrahim et al., 2021). The pandemic’s explosive growth, alongside its ever-changing rhythms and ranging symptoms, makes it difficult to contain. Furthermore, the outbreak has overburdened the health infrastructures and medical staff in a number of nations around the globe, resulting in high mortality rates (Zoabi et al., 2021). If the individuals are monitored regularly with remote technology, it may help the rapid tracking of suspected COVID-19 cases. Furthermore, the utilization of such systems will generate an outsized amount of raw data, which can open up various possibilities for using big data analytics techniques (Zoabi et al., 2021) to enhance the standard of healthcare services. There are a substantial amount of open-source development tools, like the Apache project’s predictive analytics components (Alsunaidi et al., 2021), that are intended to function in a cloud computing and distributed environment to assist with the advancement of massive data-based solutions. The Six V’s (Value, Volume, Velocity, Variety, Veracity, and Variability) also are critical elements of massive data. However, the official definition of predictive analytics most vital points only describes three Vs: volume, velocity, and variety (Ibrahim et al., 2021). The features of massive data correspond to data collected within the healthcare market, increasing the likelihood of using advanced analytics techniques to spice up the sector’s infrastructure and profitability. Within the field of healthcare, predictive analytics features a broad array of applications, encompassing genomics (Shahid et al., 2019), drug development and biomedical practice (Kuo et al., 2017), customized healthcare (Xia et al., 2017), gynecology, nephrology, oncology, and a spread of other application scenarios (Abraham and George, 2015). Cloud storage is the easiest method to save lots of data or files online (Zhang et al., 2018). However, with cloud storage having an excessive amount of data could be a problem (DayoAlowolodu et al., 2018). A blockchain may be a technique for creating cloud storage faster and safer. A blockchain may be a distributed database or ledger which can be accessed by anybody on the web. Only authorized individuals have access to the present ledger, as it is encrypted. That seems attractive, but the information remains viewable to the users that have access to the ledger. We would like something that might make the info fuzzy, unless the intended and bonafide user has the key. Therefore, during this paper, we propose a double crossover-based encryption approach that might encrypt patients’ personal and medical data and store it on a blockchain framework.
Literature Review
We additionally look into the pros and cons of using big data analytics tools to analyze COVID-19 data. Given its widespread affordability and acceptance, attractive wearable technology is likely to be amongst the key sources of health statistics. According to a survey performed in January 2020 in China, 88% of the 4,600 people polled said they would be willing to employ wearable technology to monitor and track their vital signs, while 47% of chronically ill individuals and 37% of non-chronically unwell patients said they were willing to share their health information with healthcare research companies without their permission. 59% of the same group indicated that they believed artificial intelligence (AI)-based services would likely be used to diagnose their health symptoms (Ouyang et al., 2021). People consistently exchanging such data would considerably increase the volume of data, necessitating the development and implementation of data analysis tools and models in this industry. For example, Reference (Agbehadji et al., 2020) used big data for feeling analysis and found a correlation between social media usage and political views, attitudes, and expressions. The study’s drawback is the lack of data sources, which were difficult to get by due to privacy and information preservation concerns. Furthermore, the authors of Reference (Wu and Zhu, 2020) reviewed a number of studies on mathematical models to improve the efficacy of COVID-19 detection and prediction. To improve detection accuracy, they suggested employing artificial intelligence to discover COVID-19 cases, big data to trace cases, and nature-inspired computing (NIC) to choose appropriate features. Some studies looked at heart-related ailments and came up with suggestions and guidelines, such as Reference (Agbehadji et al., 2020), to assist people to understand heart failure’s causes, symptoms, and the persons who are most afflicted. They stated that heart failure can worsen a patient’s injuries, particularly in those who have significant conditions. In prognostic and preventative healthcare, real-time analysis of health data using AI algorithms will play a critical role (Lo-Varco et al., 2003). It will, for example, aid in the prediction of infection sites and virus propagation. It will also aid in the estimation of the requirement for beds, medical professionals, and medical resources amidst pandemic emergencies, as well as the virus’s identification and characterization (Agbehadji et al., 2020). Numerous reviews of the literature have looked into various elements of clinical information systems. Therefore, it can be assumed that these studies discuss big data, and the sharing and storing of data, without really considering the security aspects (Kapur et al., 2013). Healthcare Data Gateways (HDG) is a blockchain-based smartphone application that allows patients to manage and control their own medical records through a purpose-centric access model to preserve patients’ privacy. This model organizes healthcare data through a simple and unified Indicator Centric Schema (ICS). The proposed application combines a traditional database with a gateway to manage the medical data on a blockchain storage system, evaluate data access requests, and utilize secure multi-party computation for further processing (Khan and Alotaibi, 2020). In this paper, we show the encryption and decryption aspect of COVID-19 data and discuss the idea of storing it in a blockchain framework. Table 1 summarizes a number of such studies. In this paper, we focus on identifying the applications of big data analytics for COVID-19 and the challenges that may hinder its utilization.
Applications of Computer Science in COVID-19
COVID-19 has left a tremendous and diverse amount of data, which is rapidly growing. This information can be used in a variety of ways, encompassing diagnosis, estimating or predicting risk scores, healthcare decision-making, and the pharmaceutical sector (Stanford Medicine, 2021). Figure 1 depicts some of the possible areas of research.
Patient metadata is extensively employed in COVID-19 testing and clinical trials because it allows researchers to discover the characteristics of the ailment that aid in the diagnosis and prediction of its reappearance. Supplementary COVID-19 data is also employed, which aids in determining the number of cases, their status, and the outcomes of the PCR COVID-19 test. Another sort of data is based on sampling in order to locate virus incubators and infected locations. Descriptive statistics is also used to design proactive solutions for resource risk assessment and risk supposition, such as optimum exploitation of ICU potential. Eventually, contextual data, which has piqued the curiosity of several investigators, is used to assess the risks of pandemic transmission and identify locations where the population will be more susceptible to infection.
Key Challenges
Numerous constraints that have been encountered when building workarounds to tackle the COVID-19 epidemic may obstruct the positive outcome from the implementation of blockchain-embedded cryptographic technologies in the healthcare sector.
Security and Privacy
Authorities and individuals are concerned about healthcare data security and patient privacy issues (Pan et al., 2018), and medical data is only shared under particular circumstances and with specific specialists/researchers for specific objectives (Banerjee and Patel, 2016). As a result, it is significant to ensure the pathways, interventions, and regulations that govern and foster access to medical data without jeopardizing patients’ privacy or defrauding the data for inexcusable purposes, particularly when critical conditions arise and frightening epidemics such as COVID-19 proliferate.
Sharing Data
When employing data analysis instruments, the variety and amounts of data play a pivotal role in harvesting actionable information as well as analyzing numerous events. The proliferation of COVID-19 in the Chinese city of Wuhan, for starters, sparked worries in other countries about the virus’s characteristics and impact, as well as determining which countries are affected by the epidemic and whether they have been visited by travelers in order to take preventive measures to reduce infection spread. This problem can be solved by employing blockchain technology (Yue et al., 2016), which allows for seamless large-scale data sharing by anonymizing both patients and validated data.
Information Correctness
Whilst the internet and sociocultural mainstream press play an important role in the dissemination of relevant data and communication, they are also a dominant contributor of erroneous medical misinformation and rumors (Banerjee and patel, 2016). It is also possible that they can have a bad psychosocial impact on societal structures. Likewise, the omission or inaccuracy of statistical analyses can contribute to skewed study results (Banerjee et al., 2020). Artificial intelligence and advanced analytics toolkits, on the other hand, can be leveraged to check and filter material on the internet, as well as to forewarn individuals of misleading information and eradicate it from the internet (Xia et al., 2017).
To address the abovementioned key issues, we propose a blockchain-based security framework to secularize and share the COVID-19 vaccination data.
Proposed Methodology
To address the security issues, we propose the idea of a blockchain environment for the storing and sharing of patients’ confidential data. Incorporating our double crossover-based approach with a blockchain would ensure confidentiality on the already-authentic blockchain framework. The pixels of the images are fetched and converted to bitstreams. Then the bitstream is broken up into fixed-size blocks and the encryption is performed on the block of bits. The flowchart below (Figure 2) explains the methodology for the overall process. The images are converted to a bitstream. A pseudo-random number is generated depending on the length of the bitstream. It is generated in python using the random module. The management hub fetches the images from the dataset or directly from the CT scan center. The bitstream is then encrypted using the proposed steps as explained in the following equations (see Eqs 1–14). After this, it is shared on the blockchain. After this, a hash value is calculated from the generated ciphertext and appended with the ciphertext to make sure that integrity is maintained.
Here a gamma function is used to model the continuously changing situation of the lungs of the COVID-19 patients. The Gaussian integral is employed to calculate the overall condition of the lungs in the present time.
The personal data along with the CT Scan data is concatenated with the status.
This value is then XORed with the random number generated using the pseudorandom number generator.
Using this
Now, the bits are swapped with the consecutive bit to provide double crossover.
The hash value is calculated from the generated ciphertext. This is in turn appended with the ciphertext and shared on the blockchain framework.
Results and Discussion
An application of the proposed approach was performed leveraging the Stanford university dataset of CT Scan images with COVID-19 pneumonia and without. The dataset is referred from (The Waterloo, 2009).
Before applying our algorithm to the lung images, we tested it out on the Lena image. The (Figure 3) shows the entropy of the Lena image before and after encryption along with the entropy of the cipher image. Furthermore, it also shows the Lena image after decryption and the corresponding entropy (Table 3).
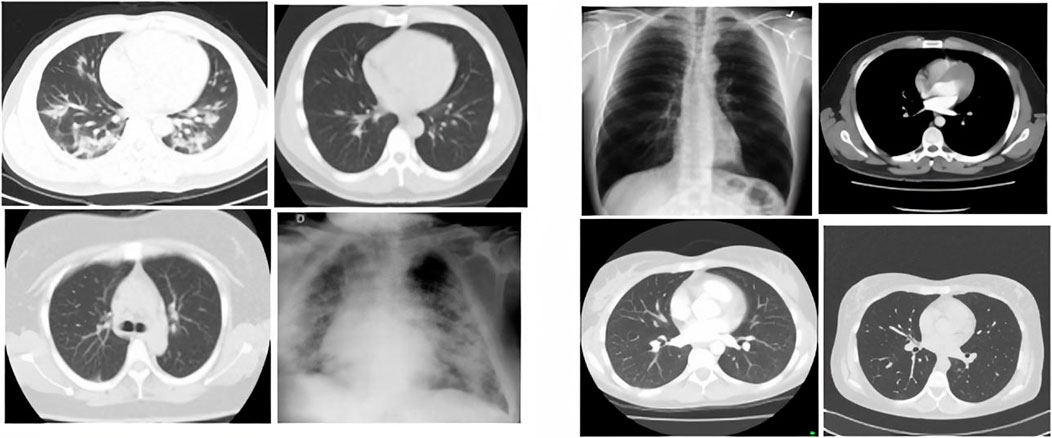
FIGURE 3. Lungs with COVID-19 pneumonia on the left and lungs without COVID-19 pneumonia on the right.
After this, we implemented the proposed double crossover approach on the lung images that were acquired from the Stanford University dataset. The (Figure 4) shows the entropy of a lung image before and after encryption. Moreover, we have displayed the entropy of cipher image as well for the same. Furthermore, it also shows the lung image (Figure 5) after decryption and the corresponding entropy.
Furthermore, this data is shared on a blockchain framework. The blockchain is simulated using Hyperledger in Ubuntu 20.04.
Information entropy has a magnitude in the range (0 1). When a system’s metadata entropy is 1, it suggests the system has no predictability at all. When the information entropy is zero, the system is free of unpredictability and imperfection. The valuation of information entropy can be exploited to represent the ultimate outcome of plaintext and ciphertext exclusivity. The higher the evidence entropy, the more secure the data (Table 4). The hereunder is the mechanism for predicting information entropy (Pan et al., 2018):
When there is no pixel association, the entropy is 8, because each pixel has 24 potential values (Table 5). However, because the digital image cannot be fully random, the actual information entropy is less than 8. The information entropy of photographs depicting real items or characters is usually between 2 and 4. The above-mentioned Lena and lung images’ information entropy are determined. Table 3 shows the entropy values of the original grayscale image, ciphertext, and decrypted image.
It has been observed in the Lena image that the entropy of the grayscale and decryption figures before encryption is about 2.3, indicating that there is a strong correlation between the various elements of the graph. But the information entropy of the ciphertext is very close to the extreme value of 8, indicating that the encrypted images are close to random distribution, and the security is higher. Similar results are observed for the lungs image as well, hence providing further assurance for the security of the proposed double crossover scheme.
Another parameter selected for performance measurement is the complexity of block addition and encryption. A blockchain was simulated using Hyperledger fabric in ubuntu. The results achieved are as follows. PQB is our approach whereas QKD (Yin et al., 2020) and QC (DayoAlowolodu et al., 2018) are existing approaches.
The third parameter is the avalanche effect. It is a measure of sensitivity, or the bit flip ratio. Whenever we changed 1 bit of the plaintext, almost 90% of the bits changed. This ensures high sensitivity. This means that even if we change just one bit of the plaintext, the entire ciphertext changes. It becomes almost impossible for an attacker or intruder to find a pattern between different ciphertexts.
The results clearly show that the proposed approach provides appropriate sensitivity in polynomial time in the given context. Hence, we can assume that for the given dataset the proposed approach performs quite well.
Conclusion
With the rapid increase in the spread of the COVID-19 pandemic, it is important to develop different data analytics and deep learning techniques for handling patients’ personal data and their health details without compromising security and privacy. The security of these techniques is currently a worry. Our proposal of a double crossover cryptosystem in a blockchain framework tries to play a small part in contributing to the confidentiality to the patients in this situation of the pandemic. The methodology is tested on a Stanford University dataset of lung images under the influence of SARS-CoV-2. The entropy of the images shows that the approach performs well for the chosen dataset in polynomial time. Through the pixel correlation of the encrypted image and the entropy followed by sensitivity tests, the analysis shows that the method has a definite advantage on the reliability and security of the Lena image and the lung image of the chosen dataset.
Data Availability Statement
Publicly available datasets were analyzed in this study. This data can be found here: COVID-19 + Imaging AI Resources | Center for Artificial Intelligence in Medicine and Imaging (stanford.edu). https://med.stanford.edu/gevaertlab/AI-basedCOVID.html.
Ethics Statement
Written informed consent was obtained from the individual(s) for the publication of any potentially identifiable images or data included in this article.
Author Contributions
BB is the research scholar. AJ and NS are the supervisors.
Conflict of Interest
The authors declare that the research was conducted in the absence of any commercial or financial relationships that could be construed as a potential conflict of interest.
Publisher’s Note
All claims expressed in this article are solely those of the authors and do not necessarily represent those of their affiliated organizations, or those of the publisher, the editors and the reviewers. Any product that may be evaluated in this article, or claim that may be made by its manufacturer, is not guaranteed or endorsed by the publisher.
References
Abraham, N. S., and George, A. (2015). A Secure Image Transmission Technique via Mosaic Image Using HSV Colour Converted Target Image and a Reversible Data Hiding Method. Int. J. Innovative Res. Comp. Commun. Eng. 3 (9), 1.
Agbehadji, I. E., Awuzie, B. O., Ngowi, A. B., and Millham, R. C. (2020). Review of Big Data Analytics, Artificial Intelligence and Nature-Inspired Computing Models towards Accurate Detection of COVID-19 Pandemic Cases and Contact Tracing. Ijerph 17, 5330. doi:10.3390/ijerph17155330
Alsunaidi, S. J., Almuhaideb, A. M., Ibrahim, N. M., Shaikh, F. S., Alqudaihi, K. S., Alhaidari, F. A., et al. (2021). Applications of Big Data Analytics to Control COVID-19 Pandemic. Sensors 21, 2282. doi:10.3390/s21072282
Banerjee, B., Jani, A., and Shah, N. (2020). Post Quantum Security Enhancement Scheme in IoT Blockchain Framework. GIS Sci. J. 7 (6), 664–672.
Banerjee, B., and Patel, J. (2016). A Symmetric Key Block Cipher to Provide Confidentiality in Wireless Sensor Networks. INFOCOMP J. Comp. Sci. 15 (1), 12–18.
Dayo Alowolodu, O., K Adelaja, G., K Alese, B., and Catherine Olayemi, O. (2018). Medical Image Security Using Quantum Cryptography. Iisit 15, 057–067. doi:10.28945/4008
Ibrahim, D. M., Elshennawy, N. M., and Sarhan, A. M. (2021). Deep-chest: Multi-Classification Deep Learning Model for Diagnosing Covid-19, Pneumonia, and Lung CANCER CHEST DISEASES. Comput. Biol. Med. 132, 104348. doi:10.1016/j.compbiomed.2021.104348
Kapur, J., and J. Baregar, A. (2013). Security Using Image Processing. Ijmit 5 (2), 13–21. doi:10.5121/ijmit.2013.5202
Khan, Z. F., and Alotaibi, S. R. (2020). Applications of Artificial Intelligence and Big Data Analytics in M-Health: A Healthcare System Perspective. J. Healthc. Eng. 2020, 1–15. doi:10.1155/2020/8894694
Kuo, T.-T., Kim, H.-E., and Ohno-Machado, L. (2017). Blockchain Distributed Ledger Technologies for Biomedical and Health Care Applications. J. Am. Med. Inform. Assoc. 24 (6), 1211–1220. doi:10.1093/jamia/ocx068
Lo-Varco, G., Puech, W., and Dumas, M. (2003). “DCT-based Watermarking Method Using Error Correction Coding,” in ICAPR’03: International Conference on Advances in Pattern Recognition (Springer), 347–350.
Ouyang, L., Yuan, Y., Cao, Y., and Wang, F.-Y. (2021). A Novel Framework of Collaborative Early Warning for Covid-19 Based on Blockchain and Smart Contracts. Inf. Sci. 570, 124–143. doi:10.1016/j.ins.2021.04.021
Pan, H., Lei, Y., and Jian, C. (2018). Research on Digital Image Encryption Algorithm Based on Double Logistic Chaotic Map. J. Image Video Proc. 2018 (1). doi:10.1186/s13640-018-0386-3
Shahid, N., Rappon, T., and Berta, W. (2019). Applications of Artificial Neural Networks in Health Care Organizational Decision-Making: A Scoping Review. PLoS ONE 14, e0212356. doi:10.1371/journal.pone.0212356
Stanford Medicine (2021). COVID-19 + Imaging AI Resources | Center for Artificial Intelligence in Medicine & Imaging. California: Stanford.edu.
Tsoi, K. K. F., Sung, J. J. Y., Lee, H. W. Y., Yiu, K. K. L., Fung, H., and Wong, S. Y. S. (2021). The Way Forward after Covid-19 Vaccination: Vaccine Passports with Blockchain to Protect Personal Privacy. BMJ Innov. 7 (2), 337–341. doi:10.1136/bmjinnov-2021-000661
Wu, H., and Zhu, X. (2020). Developing a Reliable Service System of Charity Donation during the Covid-19 Outbreak. IEEE Access 8, 848–860. doi:10.1109/access.2020.3017654
Xia, Q., Sifah, E. B., Asamoah, K. O., Gao, J., Du, X., and Guizani, M. (2017). Medshare: Trust-Less Medical Data Sharing Among Cloud Service Providers via Blockchain. IEEE Access 5, 757–767. doi:10.1109/access.2017.2730843
Yin, J., Li, Y.-H., Liao, S.-K., Yang, M., Cao, Y., Zhang, L., et al. (2020). Entanglement-based Secure Quantum Cryptography over 1,120 Kilometres. Nature 582, 501–505. doi:10.1038/s41586-020-2401-y
Yue, X., Wang, H., Jin, D., Li, M., and Jiang, W. (2016). Healthcare Data Gateways: Found Healthcare Intelligence on Blockchain with Novel Privacy Risk Control. J. Med. Syst. 40, 218. doi:10.1007/s10916-016-0574-6
Zhang, P., White, J., Schmidt, D. C., Lenz, G., and Rosenbloom, S. T. (2018). Fhirchain: Applying Blockchain to Securely and Scalably Share Clinical Data. Comput. Struct. Biotechnol. J. 16, 267–278. doi:10.1016/j.csbj.2018.07.004
Keywords: cryptography, image encryption, medical data encryption, blockchain, smart healthcare
Citation: Banerjee B, Jani A and Shah N (2021) Digital Image Encryption Using Double Crossover Approach for SARS-CoV-2 Infected Lungs in a Blockchain Framework. Front. Blockchain 4:771241. doi: 10.3389/fbloc.2021.771241
Received: 06 September 2021; Accepted: 20 October 2021;
Published: 14 December 2021.
Edited by:
Mohammad Jabed Morshed Chowdhury, La Trobe University, AustraliaReviewed by:
Shahriar Badsha, University of Nevada, Reno, United StatesMohammad Nazmul Haque, the University of Newcastle, Australia
Arif Ansori, Airlangga University, Indonesia
Copyright © 2021 Banerjee, Jani and Shah. This is an open-access article distributed under the terms of the Creative Commons Attribution License (CC BY). The use, distribution or reproduction in other forums is permitted, provided the original author(s) and the copyright owner(s) are credited and that the original publication in this journal is cited, in accordance with accepted academic practice. No use, distribution or reproduction is permitted which does not comply with these terms.
*Correspondence: Bannishikha Banerjee, YmFubmlzaGlraGEuYmFuZXJqZWVAcHBzdS5hYy5pbg==
†ORCID: Bannishikha Banerjee, orcid.org/0000-0003-4848-8749