- Department of Civil, Environmental, Aerospace and Materials Engineering, University of Palermo, Palermo, Italy
Several studies and researches have shown that modern roundabouts are safe and effective as engineering countermeasures for traffic calming, and they are now widely used worldwide. The increasing use of roundabouts and, more recently, turbo and flower roundabouts, has induced a great variety of experiences in the field of intersection design, traffic safety, and capacity modeling. As for unsignalized intersections, which represent the starting point to extend knowledge about the operational analysis to roundabouts, the general situation in capacity estimation is still characterized by the discussion between gap acceptance models and empirical regression models. However, capacity modeling must contain both the analytical construction and then solution of the model, and the implementation of driver behavior. Thus, issues on a realistic modeling of driver behavior by the parameters that are included into the models are always of interest for practitioners and analysts in transportation and road infrastructure engineering. Based on these considerations, this paper presents a literature review about the key methodological issues in the operational analysis of modern roundabouts. Focus is made on the aspects associated with the gap acceptance behavior, the derivation of the analytical-based models, and the calculation of parameters included into the capacity equations, as well as steady-state and non-steady-state conditions and uncertainty in entry capacity estimation. At last, insights on future developments of the research in this field of investigation will be also outlined.
Introduction
The roundabout technologies continue to grow across the world mostly because of the long-lasting benefits associated with planning, geometric design, safety, operations, environmental, and landscaping issues. Indeed, roundabouts often represent safer and more efficient solutions than other at-grade intersections; with regard to the installation context, the shape, and the dimensions, roundabouts can be also esthetically appealing design alternatives compared to other unsignalized intersections (Rodegerdts et al., 2010). Modern roundabouts have also gradually gained ground as traffic calming countermeasures, since they employ physical changes to the roadway and signage, which cause reductions in vehicle speeds. Single-lane roundabouts represent a proven strategy for improving safety performances; indeed, they eliminate (or alter) some conflict types so that crash severity is reduced compared to stop-controlled and signalized intersections. Multilane roundabouts, in turn, cannot normally reach the same level of safety performance as their single-lane counterpart, because of the increased number of conflict points and then the additional complexity in decision-making due to many movements among interacting users. However, multilane roundabouts work better if the traffic volume is sufficiently high and are safer than comparable large signalized intersections. Roundabouts can also represent a type of road engineering treatment complementary to other transportation objectives such as corridor access management and multimodal networks. In the context of a corridor, indeed, the further space needed near a roundabout – due its size and shape – may be offset by the reduced space required between consecutive intersections (Rodegerdts et al., 2010). Roundabouts are also evolving. Recent evolutions of roundabouts include turbo roundabouts, characterized by a spiraling traffic flow through physical barriers between the lanes on the ring, and flower roundabouts characterized by physically separated lanes for right turning so that the circulatory roadway is mostly used by vehicles driving straight through or turning left. However, for further details on turbo and flower roundabouts, the reader is referred to more specialized sources [see, e.g., Fortuijn (2009) and Tollazzi (2015)].
Calculation of Roundabout Operations
Over time, a large number of studies have been developed to analyze operations of existing or planned roundabouts. However, the topical discussion between gap acceptance theory and empirical regression models still characterizes the general situation about capacity calculation of unsignalized intersections and roundabouts (Brilon et al., 1999). Empirical and analytical models for roundabout analysis under steady-state conditions are currently in use worldwide, given their specific advantages and drawbacks. Empirical regression models are generated from field data and establish relationships between capacity (and/or delay) and geometry [see, e.g., Kimber and Coombe (1980) and Brilon and Vandehey (1998)]; however, analyst needs a great data collection from saturated roundabout entries to obtain reliable results. Based on the concept of gap acceptance, analytical models, in turn, can be developed starting from uncongested conditions; they require that the critical headway and the follow-up headway are specified. It is noteworthy that all methods of capacity analysis for unsignalized intersections and roundabouts are derived from the simple queuing model where one can observe an intersection between two one-way streets (Troutbeck and Brilon, 2016). Indeed, before entering the intersection, drivers having lower priority have to seek an acceptable gap in the major stream; in the case of bunched vehicles that form a block in a major stream, minor stream vehicles can merge into the major stream, only when the gap following the last vehicle of the block is equal to (or greater than) the critical headway (Tanner, 1962). Troutbeck and Brilon (2016) highlighted that, in gap acceptance theory, consistent and homogeneous driver behavior is assumed. Thus, a consistent driver is expected to behave in the same way every time the same situation occurs: he/she is not expected to reject a gap and then subsequently accept a smaller gap; moreover, all drivers of a homogeneous population are expected to behave exactly in the same way. However, it can be unreasonable to expect drivers to be consistent and homogeneous, since the critical headway and the follow-up headway change over time; they also vary among roundabouts, drivers, types of movements, and traffic situations. Due to this variability, there is no doubt that gap acceptance process is of stochastic nature and the critical headways and the follow-up headways can be regarded as random variables (Brilon et al., 1999). At multilane installations, drivers can wait for headways both in the inner and outer lanes; some drivers – who enter the roundabout from the right entry lane – could yield to the traffic circulating in the roundabout rather than only the circulating flow on the outer lane (National Research Council and Transportation Research Board, 2010). This uncertainty about the path of circulating vehicles may be emphasized at multilane roundabouts than comparable unsignalized intersections due to the curvature of the circulatory roadway; the same gap acceptance behavior of the right entry lane could result imperfect and difficult to quantify by using a simple gap acceptance model. Thus, an inclination toward the regression models – that implicitly take into account these factors – may occur when roundabouts with two or more circulating lanes have to be examined. However, evidence showed that regression models can result insensible to entry flow distribution and opposing flow distribution at roundabouts (Silva and Vasconcelos, 2011); the same authors refer that regression models are inadequate to study new layouts, particularly, when lane-by-lane analyses is required. More complex capacity formulas based on gap acceptance may be more sensible to traffic distribution among the entry and circulatory lanes (Hagring, 1998).
Gap acceptance models provide entry capacity estimates based on constant values of the critical headway and the follow-up headway which, in turn, represent average values for all the observed drivers. Considering constant values of the critical headway and the follow-up headway, the capacity of an entry always represents average conditions that are experienced by the users. Since actually variability and heterogeneity characterize drivers’ population, the assumptions on driver behavior above introduced can produce erroneous estimates of roundabout capacity. However, the critical headway and the follow-up headway being stochastically distributed cannot be considered as constant values, but each of them should be represented by a distribution of a set of values. Moreover, when capacity models based on the gap acceptance theory are used, analyst should specify the probability distribution of headways between vehicles in each major stream (Giuffrè et al., 2012a).
The Aim of the Paper and Its Organization
Starting from these considerations and without claiming to be exhaustive, the article provides an overview of the key methodological issues in the operational analysis of the roundabouts. Focus is made on the derivation of the analytical-based models under steady-state (undersaturated) conditions at entries, the gap acceptance behavior, and the calculation of parameters included into the capacity equations, the issues of the stochastic nature of the traffic phenomena.
According to these objectives, the paper is organized as follows: Section “Operational Analysis Issues at Roundabouts” provides a brief background about data, needs, and problems concerning the operational analysis at roundabouts and then introduces several methods for estimating the parameters, which explain the driver’s psycho-technical attitudes at roundabouts. Section “Modeling Methods for Roundabout Capacity Analysis” presents modeling methods for roundabout capacity analysis, the counting distributions and the headway distributions – on which the derivation of the capacity models is based – and describes some gap acceptance capacity models. Section “Some Remarks on Steady-State and Non-Steady-State Conditions” summarizes the issues concerning the traffic steady-state and non-steady-state conditions, whereas uncertainty in roundabout capacity evaluation is commented in Section “Managing Uncertainty in Roundabout Entry Capacity Evaluation.” At last, Section “Conclusion and Future Developments” presents some future directions on the research activities of the authors in roundabouts analysis.
Operational Analysis Issues at Roundabouts
Design and performance evaluation of a new (or an existing) roundabout is the core function of an operational analysis. In order to analyze operations of planned or existing roundabouts, the methods must allow a transportation analyst to assess the operational performance with regard to the use of the intersection and the elements of geometric design. However, modeling of real-world performances can result in a complex action especially when one has to evaluate: (1) the effect of exiting vehicles on entering driver’s decision (e.g., one can be uncertain of the intentions of the exiting or turning vehicles); (2) conditions of capacity constraint for one or more entries (with the consequent circulating flow downstream of the constrained entry less than the demand); (3) origin–destination patterns, which may influence the capacity of a given entry; (4) differences in vehicle fleet mixes, and so on. An operational analysis needs two kinds of estimates: the roundabout capacity and the level of service by using measures of effectiveness such as (control and geometric) delay and queues (Rodegerdts et al., 2010). When roundabout entry capacity must be calculated over a chosen observation period, steadiness and variability in traffic demand, as well as saturated or oversaturated conditions at entries, need to be specified. This requires the analysis of roundabouts with and without statistical equilibrium; based on the traffic conditions at entries, the use of probabilistic, deterministic, or time-dependent models is also needed (Mauro, 2010; Troutbeck and Brilon, 2016).
Roundabouts normally use gap acceptance rules. Since minor street drivers have to yield the right-of-way to circulating vehicles (that pass in front of the subject entry), entry capacities, just as service times, depend on the availability of major stream gaps, which should be large enough to enter into the intersection in a safe way. Thus, the operational performance of roundabouts can be influenced by the traffic volume desiring to enter a roundabout at a given time, the vehicle flow rate on the ring and the arrival headway distributions, as well as geometric design, vehicle and environment characteristics that affect each individual gap acceptance behavior. Geometry also plays a significant role in the evaluation of the operational performance at roundabouts: the angle at which a vehicle enters can affect the speed of circulating vehicles; the entry widths can determine the number of side-by-side vehicle streams at the yield line and can affect the rate at which the circulatory roadway may accommodate the vehicles; lane alignment can determine imbalanced lane flows on an entry and thus can influence entry capacity, etc. Thus, the geometric characteristics have an impact on the gap acceptance decision-making and then the capacity.
Entry capacity estimation is based on the critical headway and follow-up headway when the analytical-based (gap-acceptance) models are used to analyze roundabout performances. Thus, the accuracy of capacity estimation at roundabouts is dependent on the accurate estimation of these two parameters. Capacity calculation always provides average values, since it is based on constant values of critical headway and follow-up headway; however, the critical headway and follow-up headway are stochastically distributed and should be represented by a distribution of values. The analysis of this problem could be the starting point for assessing and trying to measure the uncertainty in roundabout capacity estimation.
The estimation of critical headway and follow-up headway cannot be end in itself, since the gap acceptance parameters are introduced into the capacity models for unsignalized intersections and roundabouts (Brilon et al., 1999). Most of methods published around the world for estimating the gap acceptance parameters are for unsignalized intersections; however, they can be extended to roundabouts, since they focus on the simple case of two one-way streets where only two movements are allowed: one minor stream, which gives priority to the major stream before entering the intersection. Today, for estimating the critical headway and the follow-up headway, and for calibrating the existing capacity models, the analysts necessarily have to distinguish data collected at single-lane sites from those surveyed at multilane sites to assess the effect of the number of lanes, the size of the diameter and the entry width, and to consider different traffic patterns with dominant and subdominant arrival flows (Rodegerdts et al., 2007, 2010).
Before introducing the key concepts and the methods to perform the roundabout capacity analyzes, the techniques actually used to estimate the critical headways and follow-up headways will be described in the following section.
Estimation of the Critical Headway and the Follow-up Headway
The critical headway can be estimated from on-field observations by employing several techniques which, in general, fall into two classes: the first class of techniques is based on a regression analysis between the number of users, which can enter into a major stream gap and the time duration of this gap; in this case, saturated conditions are required and the queue must have at least one vehicle in it over the observation period. The second class of techniques, in turn, estimates the distribution of the critical headways and the distribution of follow-up headways independently.
Probabilistic approaches must be used to estimate the critical headway when the minor stream does not continuously queue. Thus, most of these methods require the appropriate observation of a minor street driver under unsaturated traffic conditions and his/her gap acceptance decisions at an entry of unsignalized intersections or roundabouts.
With reference to regression techniques, Siegloch (1973) proposed to observe a condition of continuous queuing on the minor street; thus, one can observe n realizations (that are always integer numbers) for the function n(τ) by counting the number of the minor stream vehicles that enter the roundabout using major stream headways of size τ: In order to represent the observed data, we can use the linear regression on the average headway size values (i.e. the dependent variable) against the number of vehicles that enter during this average headway size, , or:
in which the coefficients a and b have to be estimated. However, the average headway size from the observed τ values, for each realization n, should be computed before starting the regression; otherwise the more numerous observations for the smaller n would govern the whole result (Brilon et al., 1999).
The linear regression function in Eq. 1 would be correct if the critical headway and the follow-up headway were constant values; then it can be written as follows:
where τ0 = τc − τf/2 represents the intercept of the headway size axis and τf the slope of the linear regression above introduced. In this way, the critical headway τc can be calculated from the regression technique directly.
Under unsaturated traffic conditions, the regression techniques cannot be applied (see above); thus, the critical headway can be calculated through probabilistic approaches. On this regard, the most commonly used methods – but not limited to these – are Raff’s method (Raff and Hart, 1950), Ashworth’ method (Ashworth, 1970), the maximum likelihood technique (Troutbeck, 1992); subsequently, a brief description will be given.
According to Raff and Hart (1950), the critical headway (τc) represents that value of τ at which 1 − Fr(τ) = Fa(τ), that is the cross point of the cumulative distribution function Fr(τ) of the rejected headways (>τ) and the cumulative distribution function Fa(τ) of the accepted headways (<τ). The critical headway represents the median value (not the mean value) of the distribution.
Differently from Raff and Hart (1950); Ashworth (1970) found that the critical headway (τc) can be estimated from the mean (μa) of the accepted headways (τa) and the SD of the accepted headways (σa): τc = μa − qc ⋅ σa2, where qc stands for the major stream traffic volume (in volume per second). It is noteworthy that the equation above is valid under the assumption of exponentially distributed major stream headways (with statistical independence between consecutive headways) and normal distribution for τc and τa. Thus, for the critical headway estimation, Ashworth’s method uses only the accepted headways, neglecting the rejected headways. In turn, the maximum likelihood method requires information about the accepted gap and the largest rejected gap for each driver.
Troutbeck (1992) described in detail this method based on the assumption that a driver’s critical headway is larger than the largest rejected headway (τr) and smaller than the accepted headway (τa). Thus the method calculates the probability of the critical headway being between the largest rejected headway (τr) and the accepted headway (τa). In order to estimate this probability, the driver’s behavior is assumed to be consistent. The likelihood that the driver’s critical headway τc will be between τr and τa is given by the difference between the two corresponding cumulative distribution functions Fa(τa) − Fr(τr).
Based on the two vectors of the observed {τr} and {τa}, the likelihood L* for a sample of n observed entering drivers is given by:
whereas the logarithm L of the likelihood L* is given by:
The probabilistic distribution for the critical headways is usually assumed to be log-normal. The likelihood estimators μ and σ2 (the mean and the variance of the critical headway distribution), which maximize L are the solutions to the two equations and . This leads to a set of two equations, which are depending on the vectors of the observed {τr} and {τa} and must be solved iteratively by using numerical methods. Troutbeck (1992) proposed a solution by using iterative numerical solution techniques; thus the mean critical headway and its variance could be computed by: and . According to Tian et al. (1999), the mean critical headway could be calculated and used in various gap acceptance capacity and delay models, since it was an acceptable quantity for representing the average driver behavior.
Other methods for estimating critical headways have been also recommended for practical applications: Harder’s method (Harders, 1968) discussed in more detail by Brilon et al. (1999), the logit procedures, which provide many similarities to the classical logit models of transportation planning [see, e.g., Polus et al. (2005)], the probit procedures, having formulations similar to the logit models, and used to estimate the probability that a gap will be accepted; however, in the last flows should be managed a lot more carefully (Solberg and Oppenlander, 1964). Wu (2012) proposed a method for estimating the distribution function of critical headways at unsignalized intersections based on equilibrium of probabilities; in turn, Hewitt’s method (Hewitt, 1983) enabled the calculation of the probability distribution of the critical headways for entering drivers, which reject the initial lag; the method is based on observations of the time duration of the headways refused and eventually accepted by drivers. Hewitt (1985) also performed a comparison between some methods for measuring critical headway.
More recently, microscopic approaches have been also used to estimate the critical headway at roundabouts. For instance, Vasconcelos et al. (2012) proposed an alternative gap-acceptance model that described the interactions on a microscopic level between the entry and opposing vehicles. The model explored the complex interactions between the driver/vehicle dynamics and the intersection geometry; it was calibrated based on a video recording of a Portuguese roundabout, and then validated by using geometric and traffic characteristics of other roundabouts. Since the estimates were close to the results of traditional estimation methods, the proposed model was considered a promising alternative to field observations, particularly for non-standard intersections. In the field of roundabouts, just as for the at-grade intersections, several studies and researches developed worldwide provide measurements of driver’s critical headway [see, e.g., Rodegerdts et al. (2007), Guo (2010), Vasconcelos et al. (2013), Ahmad et al. (2015), and so on]. In most cases, the maximum likelihood procedure resulted the most promising method for estimating the critical headway at single-lane and multilane roundabouts.
Differently from the critical headway, the follow-up headway can be estimated directly from on-field observations by measuring the difference between the entry departure times of the minor-street queued vehicles using the same gap in the major stream. Rodegerdts et al. (2007) observed that vehicles using the same gap usually have the same opposing vehicle time, which may be calculated based on the accepted lag or the accepted gap. By using the accepted lag, the opposing vehicle time can be calculated by adding the entry arrival time to the accepted lag; by using the accepted gap, in turn, the opposing vehicle time can be calculated by adding the entry arrival time to the total rejected gaps and lag. It is noteworthy that, at multilane sites, the follow-up headway may be also influenced by the dominant and subdominant arrival flows. However, further sites with dominant left-lane arrival flows should be examined to validate the concept; what is more, interdependencies between entering and circulating vehicles at multilane roundabouts can be observed, because of the priority reversal between entering and circulating vehicles. In these cases, specific analytical capacity models should be derived from observations of the driver behavior [see, e.g., Giuffrè et al. (2012b)]. The complexity of these models may lie behind the difficulty of observing the behavioral parameters, which are required to implement the model. Giuffrè et al. (2014) proposed a procedure to get the unknown behavioral parameters from traffic surveys; these parameters concerned the saturation headways, which often elude the direct observations, since traffic conditions in which they can be observed rarely occur. Thus, the unknown parameters were estimated through a regression model based on-field data surveyed at a multilane roundabout in Palermo, Italy; for further details, the reader is referred to the original sources abovementioned.
Based on a systematic literature review of empirical studies and researches developed in different countries with the objective to measure the major gap-acceptance parameters at existing roundabouts, Giuffrè et al. (2016) noted that the effect size – i.e., the statistical mean values of the critical headway and the follow-up headway, which each (primary) study presents – varied from study to study; hence, they performed the meta-analysis of effect sizes as part of the literature review through the random-effects model. The meta-analysis, indeed, was developed in order to work directly with the effect size from each study; thus, a summary effect was computed (and tested) for each gap-acceptance parameter – at different types of roundabouts – by synthesizing the site-specific estimates from prior studies. Compared to the results of each study, the single quantitative meta-analytic estimate, both for the critical headway and the follow-up headway, represented an appropriate and reliable quantity for describing an average driver behavior. At last, the meta-analytic estimate gave, with greater power than each effect size, a comprehensive measure for the parameters of interest; it could be used to recalibrate existing capacity models for single-lane roundabouts and double-lane roundabouts.
Modeling Methods for Roundabout Capacity Analysis
Starting from the simple queuing model – in which a single minor traffic stream crosses a single major traffic stream – the capacity calculation for a roundabout in steady-state condition can be addressed by specifying the arrival headway distribution in the major stream of volume Qc (veh/h) and the gap-acceptance function which expresses the number of minor stream vehicles that can depart during an acceptable headway of size τ (Siegloch, 1973). However, an understanding of the interaction of two traffic streams can represent the basic sources of knowledge about capacity estimation for unsignalized intersections and roundabouts with more than two traffic streams.
Usually, n(τ) denotes the number of the minor stream vehicles, which can enter the roundabout using a time headway of size τ and f(τ) denotes the probability function of all headways in the major circulating stream. Based on the assumptions about user behavior at unsignalized intersections and roundabouts as introduced in the previous sections, a biunique correspondence exists between n(τ) and τ. This is why f(τ) can be also viewed as the probability distribution of n(τ) (Mauro, 2010); then the mean value can be calculated as follows:
When one divides this mean value to the average size of headways , clearly equal to , one can get:
Equation 6 gives the mean number of minor vehicles, which perform their maneuvering in the time unit, i.e., the entry capacity. This equation for the entry capacity of unsignalized intersections and roundabouts forms the foundation of the gap acceptance theory; indeed, most of the analytical capacity models found in literature are based on this concept [see, e.g., Brilon et al. (1999) and Mauro (2010)]. The capacity provided by τ headways per hour is then Qc ⋅ f(τ) ⋅ n(τ); thus, the capacity is a function of the circulating flow (Qc, veh/h), which is synthesized by f(τ); in turn, n(τ) takes into account the users’ psycho-technical attitudes, which are synthesized by the critical headway and the follow-up headway (Mauro, 2010).
As a consequence of the equation above, the capacity of the simple two-stream situation can be calculated by methods based on the elementary probability theory when the following assumptions are met: (1) constant values for the critical headway and the follow-up headway; (2) exponential distribution for major stream headways (see “Counting Distributions”); (3) each traffic stream is characterized by constant values of the traffic volumes.
Considering constant values for the critical headway and the follow-up headway, two different types of capacity equations can be distinguished based on two different formulations for n(τ): the first type of capacity equations assumes a stepwise (constant) function for n(τ) (Harders, 1968), whereas the second type of capacity equations assumes a continuous linear function for n(τ) (Siegloch, 1973; Rodegerdts et al., 2010). It is noteworthy that when one models the capacity of entries conflicted by two (or more) circulating lanes, the conflicting flow rate is the total of all major streams (National Research Council and Transportation Research Board, 2010); thus, all major streams are combined as one traffic stream and examined by using proper multilane stream parameters. The headway distribution in the major traffic stream can be dealt by using appropriate values of the minimum headway and the bunching parameters.
In general, modeling arrivals of vehicles at a road cross-section is a fundamental step in traffic flow theory. An important application concerns traffic flow simulation in which vehicle generation has to represent vehicles arrivals. However, the vehicle arrival is a random process since several vehicles can come together, or vehicle arrivals can be rare events. Modeling vehicle arrivals means modeling how many vehicles arrive in a given interval of time, or modeling what is the time interval between two arrivals of successive vehicles. In the first process, the random variable is the number of vehicle arrivals observed in a given interval of time; it takes some integer values. Thus, the process can be modeled by a discrete distribution. In the second process, the random variable is represented by the time interval between successive arrival of vehicles and it can be any positive real values; thus, some continuous distributions can be considered to model the vehicle arrivals. It is noteworthy that, being these processes correlated, the distributions that describe them should be also inter-related for better explaining this traffic phenomenon (Kadiyali, 1987; May, 1990).
Bearing in mind the objective to estimate entry capacity at roundabouts, in the following, we will refer about some discrete distributions, which account for traffic counts and are used to model the vehicle arrivals; then, we will present some continuous distributions used for (time) headway modeling.
Counting Distributions
According to Mannering and Washburn (2013), the derivation of models that take into account the non-uniformity in traffic flow is based on the assumption that vehicle arrivals, at a specified cross-section, correspond to some random process. Thus, one should select a probability distribution suitable to represent the observed patterns of traffic arrivals. Among the counting distributions, we can remember the Poisson distribution; the corresponding probability mass function is given as follows:
where P(n) is the probability of having n vehicle arrivals in the time interval t, λ is the average vehicle flow (i.e., the arrival rate in vehicles per unit time), and t stands for the duration of the time interval over which vehicles are counted. The Poisson distribution (also known as the law of rare events) was introduced by Kinzer (1933); until now, several applications have been carried out in transportation engineering.
Based on the statistical assumptions concerning the derivation of Poisson distribution, the model lends itself well as arrival model in a single lane (or two or more adjacent lanes) when steady-state conditions persist over the analysis time period, and the arrival of one vehicle is independent of the arrival of another vehicle (i.e., no interaction is experienced between the arrivals of two successive vehicles). Empirical observations have shown that the assumption of Poisson-distributed traffic arrivals is most realistic in lightly congested traffic conditions; thus, the model can be consistent with experimental data when the flow is rare and, hence, it can be used when flow rates up to 400–500 veh/h are accommodated. The Poisson distribution cannot be used without a steady-state condition or when traffic flows reach heavily congested conditions; in these cases, other traffic flow distributions can be considered more appropriate (Mauro, 2010). Another limitation of Poisson model is that the mean of the observations equals the variance (Mannering and Washburn, 2013). However, many real count data do not adhere to the assumption that the mean and the variance are equal, and another distribution should be used. When the variance exceeds the mean of the counts, the negative binomial distribution can be used; it captures overdispersion, which can take place in various contexts [see, e.g., Hilbe (2008)]. When, in turn, the mean of the counts exceeds the variance the choice of the probability distribution can fall on the binomial distribution; however, it should be particularized with the measured data [see, e.g., Devroye (1986)]. Such distributions are discussed in more specialized sources [such as, for instance, May (1990), Lord and Mannering (2010), and Mauro and Branco (2012)]. The criterion for choosing alternative traffic counting models has been exposed by Mauro and Branco (2013); the same source shows the theoretical probability distributions of arrivals (namely, the binomial, Poisson, and negative binomial distribution) and their expressions as a function of the sample statistics.
Headway Distributions
Models of random arrivals are widely discussed in the technical literature and used since they are fundamental to the gap acceptance modeling. Besides counting distributions, suitable for describing counts of discrete units, such as cars, under various conditions of occurrence, another class of distributions is that of interval distributions, which describe the probability of intervals (headways) of different sizes between events and need to be characterized statistically. However, counts of cars deal with discrete events, whereas headways can be measured on a continuous scale. For purely random events, arrival headways are described by the negative exponential distribution; when drivers are forced into non-random behavior as during congested traffic conditions, other distributions can result more appropriate.
In detail, for populations whose counts are described by the Poisson distribution, the headways between counts can be described by the negative exponential distribution (M1). This distribution has been extensively used in literature; it is based on the assumption that each vehicle arrives at random without dependence between successive vehicle arrivals (Troutbeck and Brilon, 2016).
The headway h must be then greater than t and the probability density function may be stated as:
where h stands for the headway between events and λ = Qc/3600 is the average arrival rate in the opposing stream (in vehicle per second). The cumulative probability function of headways is given by
However, the M1 distribution allows unrealistic short headways and does not describe platooning. When traffic volume is so high that each car tends to follow the car ahead, M1 distribution may be unsuitable to describe the headways between cars and can be considered realistic for a very low traffic flow rate (about less than 150 veh/h). Thus, the shifted negative exponential distribution (M2) can result more suitable. Indeed, M2 distribution represents the probability that the headway h is less than t with a prohibition of headways less than Δ, that is the shifted exponential distribution assumes that there is a minimum headway between vehicles. The cumulative probability distribution of headways may be stated:
where Δ is the amount of the shift since short headways are prohibited, τ ≥ Δ and λ is a model parameter calculated as . However, the M2 distribution is used for single-lane traffic only. Although, the negative and the shifted negative exponential distribution (M1 and M2) are widely used as headway distribution models, the bunched exponential distribution of arrival headways (M3) improves the representation of inter-vehicular time intervals in the (major) circulating stream, and gives a more accurate prediction of arrival headways about up to 12 s, that is particularly useful for analyzing urban roads and streets. The M3 distribution was proposed by Cowan (1975, 1987) and then extensively used for estimating entry capacity of unsignalized intersections and roundabouts [see, e.g., Akçelik and Chung (1994)]. Cowan (1987) discussed the value of the above models for their use as arrival processes in stochastic model building and described some traffic situations where the models could be appropriate. The cumulative distribution function for the bunched exponential distribution (M3 distribution) represents the probability of a headway less than t seconds and may be stated as follows:
where Δ in this case is the average intrabunch (minimum) arrival headway, is the proportion of unbunched (free) vehicles and λ is a model parameter calculated as with q ≤ 0.98/Δ (note that the arrival flow rate is q in vehicle per second). The intrabunch headway (or the headway within each bunch equal to the minimum arrival headway Δ) and the proportion of unbunched (free) vehicles (with randomly distributed headways) are related to the distribution of the circulating stream headways. The average intrabunch headway corresponds to the average headway at capacity (Δ = 3600/C, where C is the capacity in veh/h).
The M3 distribution explicitly takes into account the number of bunched vehicles through the φ parameter representing the proportion of free vehicles. Application of the M3 parameters to each circulating lane of the roundabouts allows to use capacity formulas for n-lanes, each having different Cowan M3 parameters. The M1 and the M2 distributions can be derived from the M3 distribution by assuming Δ = 0 and φ = 1 (and therefore λ = q) for the M1 distribution and φ = 1 and therefore λ = q/(1 − Δq), with q ≤ 0.98/Δ, for the M2 distribution. One can observe that both distributions assume no bunching, whereas the M3 distribution model can be applied by estimating φ or using a bunching model, which estimates φ as a function of the opposing flow. In practical application, indeed, only the traffic flow is known, not the headway distribution; thus, it is necessary to relate φ or Δ with the opposing flow. Further discussions on the M3 model and gap acceptance models can be found in more specialized sources [see, e.g., Luttinen (1999) and Akçelik (2007)].
Gap Acceptance Capacity Models
The arrival headway distribution models can be used together with gap acceptance parameters to derive the capacity models. As above introduced, gap acceptance models are (macroscopic) analytical models, which express the capacity in an exponential function of the circulating flow; thus the rate of reduction in capacity decreases as the circulating flow increases.
Based on the gap acceptance process, for the simple two-stream situation entry capacity can be estimated by elementary probability theory methods if the assumptions introduced in Section “Modeling Methods for Roundabout Capacity Analysis” are met. Harders (1968), for instance, used Eq. 6 and combined a stepwise constant function for the number of minor stream vehicles n(τ) (which can enter the roundabout using a major stream headway of size τ) and the M1 distribution for headways in the major circulating stream. Thus, he obtained the well-known capacity model as follows:
where λ = Qc/3600 and the other symbols have the meaning already explained. This model was also adopted in the National Research Council and Transportation Research Board (2000). Siegloch (1973) first derived another capacity formula resulting in a relation of capacity versus conflicting flow Qc = λ⋅3600, veh/h, by applying a continuous linear function for n(τ) as follows:
where τ0 = τc − τf/2, s. More recently, this capacity model was revised in the National Research Council, and Transportation Research Board (2010) as follows:
The above capacity model is an exponential regression model based on a gap acceptance theory (Akçelik, 2011); this model can be calibrated by using site-specific values for the critical headway and the follow-up headway. Geometry is classified in terms of the numbers of circulating lanes and entry lanes. In this model, shorter critical headways were used for a multilane roundabout than a single-lane roundabout.
Troutbeck (1986), as reported by Brilon et al. (1999), highlighted that the assumptions introduced above (see “Modeling Methods for Roundabout Capacity Analysis”) for the capacity of the simple two-stream situation, could lead to an unrealistic representation of the phenomenon under examination and different efforts to drop one or the other assumption were made also by using traffic simulations technique [see, e.g., among the first researches, Grossmann (1988)]. However, Troutbeck and Brilon (2016), also based on the ongoing experience, observed that Harder’s model (Harders, 1968) and Siegloch’s model (Siegloch, 1973) can give quite realistic results in practice.
More general solutions for the capacity models have been obtained by replacing the M1 distribution with the more realistic M2 and M3 distributions. For instance, a more general capacity formula is derived by using a dichotomized distribution as follows:
where λ = (φ⋅Qc)/(3600 − Δ⋅Qc). If φ = 1 and Δ = 0, then Harders’ equation is obtained. If φ = 1 − λ⋅Δ the capacity equation derived by Tanner (1962) is obtained with a step function for n(τ), whereas, by using the linear relationship for n(τ), the capacity equation derived by Jacobs (1979) is given:
Fisk (1989) extended Tanner’s model to multilane roundabouts by assuming that drivers had a different critical gap when crossing different streams, whereas Hagring (1998) presented a generalization of the earlier gap acceptance model by extending Troutbeck’s model (Troutbeck, 1986). Hagring (1998) indeed derived the capacity of a minor stream crossing or merging n (independent) major streams, by using for each stream a Cowan’s M3 headway distribution. This is why Cowan’s M3 headway distribution explicitly accounts for the number of bunched vehicles through the proportion of free vehicles expressed by the φ parameter. Some bunching models to estimate φ formulated by several authors have been collected by Giuffrè et al. (2012a) and Akçelik (2007).
The Hagring model (Hagring, 1998) has been rewritten and applied several times. For instance, Giuffrè et al. (2012a) used this model to compare performances of turbo roundabouts and double-lane roundabouts; so they specified the model in relation to values of the conflicting traffic flow (moving on the inner circulating lane Qc,i and/or the outer circulating lane Qc,e), and τc, τf, and Δvalues and obtained:
when the subject entry drivers face one antagonist traffic flow; this is the case of right-lane capacity estimation. They also estimated left-lane capacity when the subject entry drivers face both the circulating traffic flow in the outer lane (Qc,e) and the circulating traffic flow in the inner lane (Qc,i) on the circulatory roadway as follows:
It should be noted that the capacity models above were built for unsignalized intersections. Since their understanding is based on the operation of the interacting streams, these models can be extended to the roundabout operation with one circulating stream or more circulating streams. Table 1 shows, in turn, a summary of gap acceptance capacity models specifically developed for roundabouts at steady-state conditions. However, the reader is invited to consult Rodegerdts et al. (2007) for a wider summary of roundabout operational models, also including details about linear and exponential regression models for capacity calculation. Figure 1 shows, in turn, the comparison among some capacity models for single-lane roundabouts; two models (Hagring, 1998; National Research Council and Transportation Research Board, 2010, recalibrated model) were also calibrated on the values of the critical headway and the follow-up headway as calculated by the meta-analysis previously performed by the authors (Giuffrè et al., 2016).
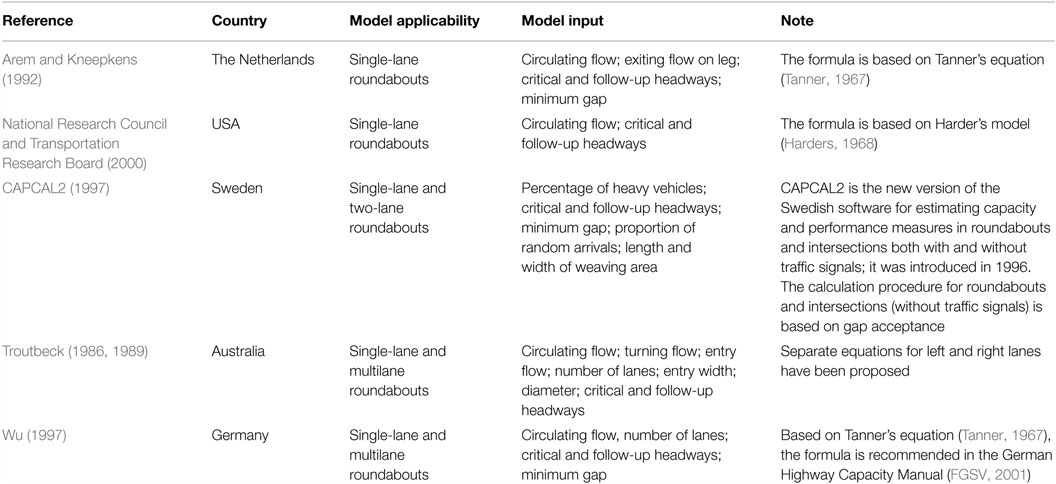
Table 1. A summary of gap acceptance capacity models for roundabouts (references in the first column).
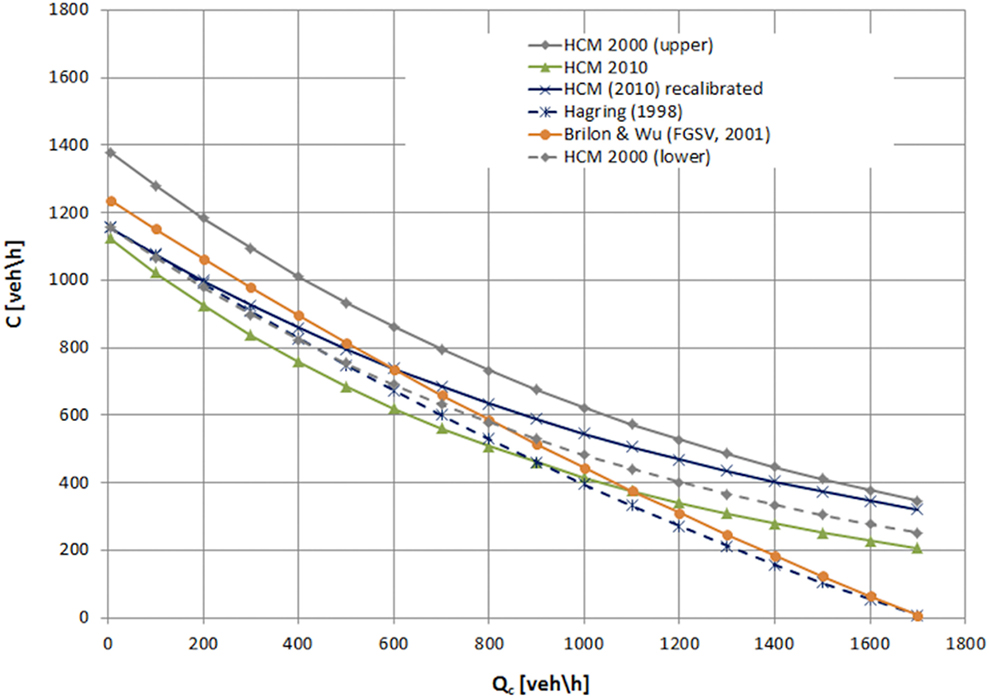
Figure 1. Comparison of capacity models for single-lane roundabouts [note that C stands for capacity and Qc for circulating flow; the Brilon and Wu formula is recommended in the German Highway Capacity Manual (FGSV, 2001)].
It should be noted that, for calculating roundabout capacity, as well as queue lengths and waiting times, steadiness and variability of traffic demand must be specified for the time period chosen; the presence of one or more saturated (or oversaturated) entries must be also highlighted. This requires the analysis of the roundabout with and without statistical equilibrium; moreover, based on the state at entries, probabilistic and/or deterministic models not only will be applied but also the time-dependent models. Statistical equilibrium and steady-state conditions will be briefly discussed in the following section. However, capacity calculation at saturation or oversaturation conditions of entries have been widely described by Mauro (2010) to which the interested reader is referred.
Some Remarks on Steady-State and Non-Steady-State Conditions
In addition to capacity, the indices that are taken into account for the assessment of traffic flow performance at roundabouts are the queue lengths, measured by the number of vehicles in terms of means and percentiles, the waiting times due to the queuing up, and the average delay for vehicles entering the intersection. To evaluate these indices, two tools can be used to solve the problems of gap acceptance: queuing theory and simulation.
Each solution based on the conventional queuing theory is a steady-state solution. Indeed, this kind of solutions are usually expected for non-time dependent traffic volumes, which are subsequent to infinitely long time, and when the demand that is experiencing compared to capacity gives a degree of saturation less than 1.
It should be noted that, calculation of roundabout capacity, queue lengths, and waiting times require that steadiness and variability of traffic demand are specified for the time period chosen; the presence of one or more saturated (or oversaturated) entries must be also highlighted. According to Mauro (2010), the analysis of the roundabout with and without statistical equilibrium is required; moreover, based on the state at entries, probabilistic and/or deterministic models not only can be applied but also the time-dependent models can be used (Troutbeck and Brilon, 2016).
In general, the operational conditions of a roundabout may be studied through the succession of states, whose evolution requires that the probability associated with each state of the system is known. However, this probability for the same state may vary any time. Thus, the system exists in a transient condition. In turn, the system reaches a statistical equilibrium (i.e., the system is in a steady-state condition) when the probabilities of the states remain constant over time. According to Mauro (2010), rather than evaluating the time-invariant state probability distribution, the finding that a steady-state condition exists, relies on the evaluation of the time invariance of some appropriate statistical values for one or more variables, that evolve randomly, and which are deemed to be related to the operating conditions of the system. Thus, a roundabout can be considered at a steady-state condition when entering traffic demand does not change over time and the roundabout entries are characterized by undersaturated conditions. In practical terms, a steady-state condition is reached, being the entries undersaturated, when the traffic demand is constant for a finite time interval T which must be:
known as Morse’s inequality (Morse, 1982). This condition can only be applied if Ci (capacity of entry i) and Qei (demand volume of entry i) – both expressed in hourly volumes – may be assumed constant during T, the entries are undersaturated in T and the degree of saturation is suitably smaller than unity. Morse’s inequality (Morse, 1982), indeed, should allow to stabilize the traffic conditions of the roundabout around constant mean values of the state variables (as queue lengths and waiting times). If Morse’s inequality (Morse, 1982) is not fulfilled, time-dependent solutions should be used. In other words, the non-steady-state situations (characterized by the variability of Qei and/or the oversaturation of the entry under examination having the degree of saturation not sufficiently greater than one) cannot be evaluated through probabilistic and deterministic approaches. Mauro (2010) reviewed some results of stochastic and deterministic theory about waiting phenomena. He also presented a general heuristic criterion for evaluating the system state variables at any operational conditions at roundabout entries, namely undersaturated, saturated, and oversaturated conditions, as well as the methods for the study of roundabouts with time-dependent traffic demands (especially roundabouts with traffic peaks, which occur between two steady-state periods of flows). The coordinate transformation method is one technique, which provides estimates between these two states (Troutbeck and Brilon, 2016). Newell (1982) developed the first mathematical solutions for the time-dependent problem, which now needs to be made more accessible to practitioners. However, a heuristic (approximate) solution for the case of the peak hour effect was proposed by Kimber and Hollis (1979).
In general, the derivation of time-dependent relationships is based on the assumption that the statistical equilibrium solutions (which allow to reach deterministic solutions) are relative to Poissonian arrivals and exponential service time. Also time-dependent solutions for traffic peaks, which occur between two periods at steady-state conditions were based on the assumption that statistical equilibrium conditions, both before and after the peaks, are the same. Mauro (2010) again highlighted that the result obtained by using time-dependent formulas (of heuristic nature) does not match the results given by the queuing theory without statistical equilibrium; however, differences can be neglected for practical interest. The approximate solutions for studying the transient states of waiting phenomena are now thoroughly explored [see, e.g., Troutbeck and Brilon (2016)]. Indeed, the modern tools of stochastic simulation allow to overcome almost all problems easily, increasing (to any desired level) the same degree of reality of the model. However, restrictions are due to the efforts to be done and the available computer time.
Managing Uncertainty in Roundabout Entry Capacity Evaluation
Most of the technical literature agrees that, in gap acceptance process, the critical headway and the follow-up headway have a significant role in determining the roundabout entry capacity as a function of the major stream flow rate with a specified arrival headway distribution. In the calculation process, current practices replace these random variables by single mean values, neglecting their changes, and providing a single-value of entry capacity. In order to manage uncertainty in capacity estimation at roundabouts, entry capacity distributions should be estimated, once the probability distributions of the critical headways and follow-up headways have been assumed. Thus, the results of the calculation should be expressed probabilistically, meaning that the probability distributions of entry capacity rather than the simple point estimates of the performance measure have to be obtained. In this view, random variables are not just the flows of the various legs (or the traffic demand), but also the entry capacities that are depending on them; furthermore, it must be said that these variables are non-statistically independent. Since traffic demand and entry capacity are random variables, they should be characterized by their probability functions. This is necessary for the evaluation of reliability in each leg, that is to say the probability that the system does not fail and, in the specific case of the roundabouts, that traffic demand does not exceed the single entry capacity.
Mauro (2010) presented general criteria for the evaluation of reliability at roundabout legs based on the study of the performance function represented by the reserve capacity (C − Qe) or the reciprocal of the rate of capacity (C/Qe); he provided the analytical relations when capacity and demand result normally distributed (having means and variances known) and he also proved that the two indices to which we usually resort (i.e., the reserve capacity or the rate of capacity) are not enough to say that the system does not fail. It is noteworthy, however, that when the mean values of the two indices in question are constant, the reliability is depending on the dispersion around the mean values of the flows, i.e., the level of uncertainty that affects the values above introduced.
Based on these considerations, in the operational analysis of roundabouts, one of the primary objectives should be to derive an entry capacity distribution, which accounts for the variations in the contributing random variables. In order to match this goal, Monte Carlo simulation can be useful to obtain the entry capacity distribution at roundabouts. Thus, one has to perform the random sampling from the probability density functions, which have to be chosen for the contributing parameters, according to an adopted capacity formulation. Here, for a first exploration, Crystal Ball software was used for performing many runs based on the Hagring model; the values of the critical headways and follow-up headways were randomly drawn from their corresponding (selected) distributions in each run. By way of example, for single-lane roundabouts, Figure 2 shows the comparison between the capacity model based on the mean values of the critical headway and the follow-up headway, which were derived from a meta-analysis (Giuffrè et al., 2016), and the capacity functions based on the probability distributions of the model parameters. It should be noted that the capacity function based on the Hagring model matches the median function; however, we observe more dispersion and uncertainty in the capacity estimation when low values of the circulating flow are considered. Moreover, the actual capacity of the roundabout may be (with a probability of about 50 percent) higher than the capacity estimated in a deterministic way, whereas, with the same probability, the capacity calculated through the deterministic model may overestimate the actual capacity (so that, for a given traffic demand, oversaturation at entries is not highlighted). It notes, therefore, that the deterministic estimation of capacity can result not cautionary, and poor performances can happen especially for low values of the circulating flow. However, the results of this first exploration could be affected by several reasons and further analysis is needed with reference to multilane and turbo roundabouts. At last, the same threshold value to attribute to reliability, it cannot be established in general terms, but it should be chosen every time in relation to the damage caused by the system failure.
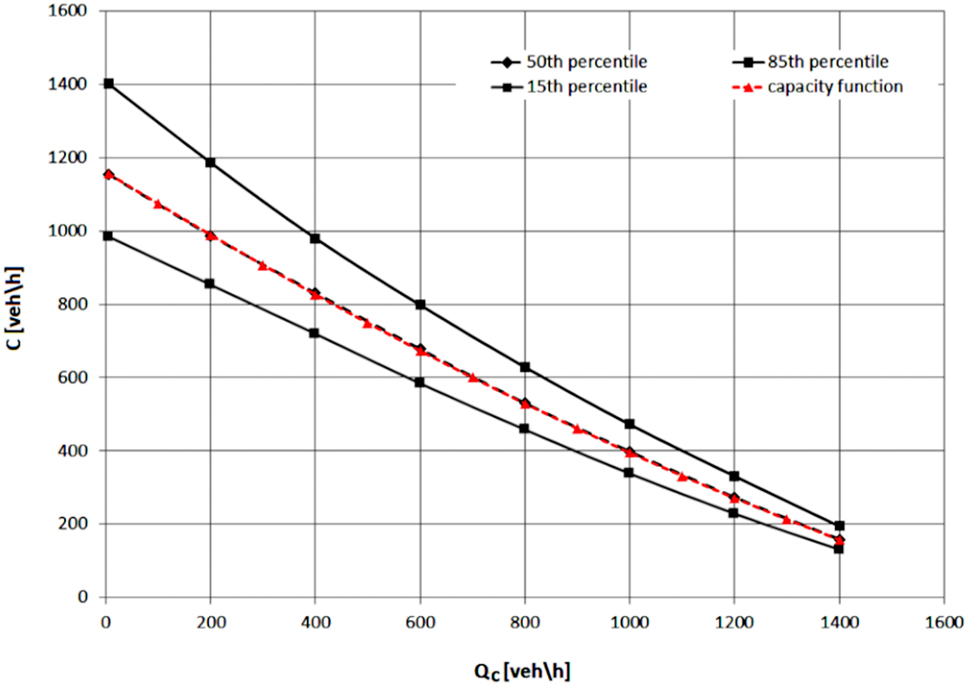
Figure 2. Uncertainty in capacity estimation at single-lane roundabouts {note that the capacity equation in figure is calculated by using the mean critical and follow-up headways provided by the meta-analysis [see Giuffrè et al. (2016)]}.
Conclusion and Future Developments
In some countries (and among these in Italy), a specific formula for the determination of the roundabout capacity has not yet been defined with any precision. Thus, several positive experiences made worldwide can be considered the starting point for the prediction of the roundabout capacity. It should be noted, first of all, that it is essential to compare the types of roundabouts recommended in each national standard (if available) with the roundabout schemes proposed by the foreign standards that address the same types of intersections. Indeed, different countries around the world use different classifications, and their standards can result not fully interchangeable with respect to the conformity between the geometric standards and the context of the roundabout installation. The same consideration may interest the calculation procedure especially regarding the entry capacity. We have introduced that, in capacity calculation, the general situation is (even today) characterized by the discussion between gap acceptance and empirical regression models. We also have focused attention on the capacity analysis procedures, which are based on gap acceptance theory to some extent and/or have understood that this theory can be considered the basis for the roundabout operations even if they have not used the same theory explicitly. Thus, attention must be placed on the precise evaluation of critical headways and follow-up headways from traffic observation, since these behavioral parameters have an important role in determining the entry capacity at roundabouts. The behavioral parameters, indeed, are random variables and they should be characterized by their probability distributions; thus, the resulting capacity value based on the mean values of the major gap acceptance parameters may underestimate or overestimate the real value of entry capacity. Moreover, based on a systematic literature review of studies and researches developed worldwide to measure critical headways and follow-up headways at existing roundabouts, the authors noted that the effect size for each parameter varied from study to study. Thus the meta-analysis of effect sizes performed as part of the literature review through the random-effects model can represent a useful approach to provide a single quantitative meta-analytic estimate, both for the critical headway and the follow-up headway; in turn, the quantitative meta-analytic estimate can be considered more appropriate for representing the gap acceptance behavior of drivers on roundabouts. It was also pointed out that traffic demand and entry capacity are also random variables and they should be characterized by their probability functions. This could increase the uncertainty in estimation procedures. In order to detect these kinds of effects, the managing of uncertainty in roundabout entry capacity evaluation, as well as the evaluation of the roundabout reliability could become fields requiring increasing attention in the next future.
Since many random factors affect roundabout operations (e.g., vehicles arriving and gap acceptance), and the application of the statistical methods can result expensive and time consuming (since reliable results can require lots of well-chosen field observations on different geometric and traffic conditions), microscopic traffic simulation can result the favorite method for the study of the roundabout operations. Recent advances in research and applications to road and highway engineering outline the great potential for useful application of microscopic traffic simulation models to accurately account for road operations and performances, since they capture the interactions of road traffic through a series of complex algorithms describing car-following, lane-changing, gap-acceptance as they happen in the real world. Differently to traditional analytical models and techniques that provide a simplified (aggregated) representation of road traffic, microscopic traffic simulation models represent for researchers and practitioners the favorite methods for analyzing operations of road networks or single road infrastructures, and taking decisions on their geometric design development, since they allow the accurate modeling of some road planning and design problems. Microsimulation, indeed, enables to create increasing levels of complexity and uncertainty in the operations of road traffic networks and single road installations, but concerns are often expressed by practitioners regarding its possible misuse. In simulation studies, moreover, model calibration is a crucial task when reliable results have to be obtained from the analysis that we made.
In the recent past, the authors have published the first results of a research in which the calibration of the microscopic traffic simulation model was formulated as an optimization problem based on a genetic algorithm; the objective function was defined in order to minimize the differences between the simulated and real data set in the speed–density graphs for a freeway segment. The application of the proposed methodology to roundabouts can represent an interesting starting point for future research activities. A comparison could be performed between the capacity functions based on the critical headways and the follow-up headways derived from meta-analysis and simulation outputs for a roundabout built in Aimsun microscopic simulator.
Author Contributions
OG is full professor in Road, Railway, and Airport Construction at Department of Civil, Environmental, Aerospace, and Materials Engineering – University of Palermo, Palermo, Italy. He has led several researches about different aspect of road construction, particularly pavement building materials, road safety, and traffic engineering. He has been present at a lot of international and national congress, and he has published several scientific works up to now. He has coordinated and coordinates many scientific local and national team. He took part in Research Committee on standards relative to road materials and project, construction, and maintenance, established at National Research Council (CNR), which is interested in fixing rules predicted by Nuovo Codice della Strada (The New Highway Code); within the Committee, he coordinates Road and Territory team. In July 2000, on behalf of the Ministry of Public Works (Circulation and Traffic Inspectorate), he carried out a specialist consultancy for the preparation of guidelines for the implementation of a system of prior control of road safety; these guidelines were incorporated into the Circular of the Ministry of Public Works in June 2001 (Circular on the guidelines for the analysis of road safety). In the years 2003–2004, he designed and later coordinated the Master course in Engineering Road Safety, financed with funds PON 2000/2006 (Axis III “Development of human capital of excellence;” Measure III.4 – training higher and further education). In years 2009–2010 and 2010–2011, he designed and later coordinated the Master course in Environmental sustainability of transport infrastructures, University of Palermo. From the academic year 2011/2012 (XXV cycle), he is the coordinator of the Ph.D. in Civil and Environmental Engineering. From the academic year 2013/2014 (XXIX cycle), he is the vice-coordinator of the Ph.D. in Civil, Environmental, and Materials Engineering at Department of Civil, Environmental, Aerospace, and Materials Engineering (University of Palermo, Italy). AG, Ph.D. in Road Infrastructure Engineering, is Associate Professor for the Scientific Sector ICAR/04 (Road, Railways, and Airport) at Department of Civil, Environmental, Aerospace, and Materials Engineering – University of Palermo, Palermo, Italy. Research activity is shown by printed articles on journals or proceedings of national and international conferences. AG has participated in several congresses; in many cases, she was called for presentation of her own papers. She won the SIIV Research award for the best paper presented at the 2008 SIIV annual meeting. Since 2012, she is editor-in-chief for the Journal of Sustainable Development. Her areas of research and expertise include functional design of road geometry, with particular reference to unsignalized intersections, modern roundabouts and turbo roundabouts, safety and reliability of transport infrastructure (roads and intersections); crash analysis and modeling; capacity modeling and transport simulation. MT is a civil engineering and a Ph.D. student in Civil and Environmental Engineering at Department of Civil, Environmental, Aerospace, and Materials Engineering – University of Palermo, Palermo, Italy. The main branches of transport engineering that she is studying are traffic and infrastructure engineering. Her research project includes meta-analysis, uncertainty in capacity estimation for roundabouts, and traffic microsimulation by using Aimsun.
Conflict of Interest Statement
The authors declare that the research was conducted in the absence of any commercial or financial relationships that could be construed as a potential conflict of interest.
References
Ahmad, A., Rastogi, R., and Chandra, S. (2015). Estimation of critical gap on a roundabout by minimizing the sum of absolute difference in accepted gap data. Can. J. Civil Eng. 42, 1011–1018. doi:10.1139/cjce-2014-0450
Akçelik, R. (2007). “A review of gap acceptance capacity models,” in 29th Conference of Australian Institutes of South Australia, Adelaide, Australia, 5–7 December 2007.
Akçelik, R. (2011). “An assessment of the highway capacity manual 2010 roundabout capacity model,” in International TRB Roundabout Conference, Carmel, Indiana, USA, May 2011.
Akçelik, R., and Chung, E. (1994). Calibration of the bunched exponential distribution of arrival headway. Road Transp. Res. 3, 42–59.
Arem, B., and Kneepkens, W. E. (1992). “Capacities and delays at roundabouts in the Netherlands,” in Proc. of the 20th PTRC Summer Annual Meeting, September 14–18, 1992. Manchester: University of Manchester.
Ashworth, R. (1970). The analysis and interpretation of gap acceptance data. Transp. Sci. 4, 270–280. doi:10.1287/trsc.4.3.270
Brilon, W., Troutbeck, R., and König, R. (1999). Useful estimation procedures for critical gaps. Transp. Res. Part A Policy Pract. 33, 161–186. doi:10.1016/S0965-8564(98)00048-2
Brilon, W., and Vandehey, M. (1998). Roundabouts – the state of the art in Germany. ITE J. 68, 48–54.
CAPCAL2. (1997). “A new version of the SNRA capacity, delay, and VOC software,” in Proc. of the 3rd International Symposium on Intersections without Traffic Signals, 21–23 July 1997 (Portland, OR).
Cowan, R. J. (1987). An extension of Tanner’s results on uncontrolled intersections. Queuing Syst. 1, 249–263. doi:10.1007/BF01149537
FGSV. (2001). Handbuch fuer die bemessung von Straβen [HBS: German Highway Capacity Manual, Edition 2001]. Cologne: Forschungsgesellschaft für Straßenund Verkehrswesen – FGSV. Available at: www.fgsv.de
Fisk, C. S. (1989). Priority intersection capacity: a generalization of Tanner’s formula. Transp. Res. Part B Methodol. 23, 281–286. doi:10.1016/0191-2615(89)90030-1
Fortuijn, L. G. H. (2009). Turbo roundabouts. Estimation of capacity. Transp. Res. Rec. 2130, 83–92. doi:10.3141/2130-11
Giuffrè, O., Granà, A., Giuffrè, T., and Marino, R. (2014). Estimating traffic operations at multi-lane roundabouts: a case study. J. Eng. Appl. Sci. 9, 90–97.
Giuffrè, O., Granà, A., and Marino, S. (2012a). Turbo-roundabouts vs roundabouts performance level. Procedia 53, 590–600. doi:10.1016/j.sbspro.2012.09.909
Giuffrè, O., Granà, A., Giuffrè, T., and Marino, R. (2012b). Researching a capacity model for multilane roundabouts with negotiation of the right-of-way between antagonist traffic flows. Mod. Appl. Sci. 6, 2–12. doi:10.5539/mas.v6n5p2
Giuffrè, O., Granà, A., and Tumminello, M. L. (2016). Gap-accepteance parameters for roundabouts: a systematic review. Eur. Transp. Res. Rev. 8, 1–20. doi:10.1007/s12544-015-0190-4
Grossmann, M. (1988). “Knosimo – a practicable simulation model for unsignalized intersections,” in Intersections without Traffic Signals, ed. W. Brilon (Berlin: Springer Publications), 263–273.
Guo, R. (2010). Estimating critical gap of roundabouts by different methods. Sixth Adv. Forum Transp. China 84, 89. doi:10.1049/cp.2010.1107
Hagring, O. (1998). A further generalization of Tanner’s formula. Transp. Res. Part B Methodol. 32, 423–429. doi:10.1016/S0191-2615(98)00010-1
Harders, J. (1968). Die Leistungsfähigkeit Nicht Signalregelter Städtischer Verkshrsknoten [Capacity of Unsignalized Urban Intersections]. Bonn: Strassenbau und Strassenverkehrstechnik 76 [Road Construction and Traffic Technology, 76], Bundesminister für Verkehr [Federal Department of Transport].
Hewitt, R. H. (1985). A comparison between some methods for measuring critical gap. Traff. Eng. Control 26, 13–22.
Jacobs, F. (1979). Capacity Calculations for Unsignalized Intersections (Technical Report in German). Institute fur StarBenBau und Verkehrswesen, University Stuttgart.
Kadiyali, L. R. (1987). Traffic Engineering and Transportation Planning. New Delhi: Khanna Publishers, 1987.
Kimber, R., and Coombe, R. (1980). The Traffic Capacity of Some Major Priority Junctions. Crowthorne, Berkshire: Transport and Road Research Laboratory. Supplementary Report 582.
Kimber, R. M., and Hollis, E. M. (1979). Traffic Queues and Delays at Road Junctions. Crowthorne, Berkshire: TRRL Laboratory. Report LR909.
Kinzer, J. P. (1933). Application of the theory of probability to problems of highway traffic. Inst. Traff. Eng. 5, 118–124.
Lord, D., and Mannering, F. (2010). The statistical analysis of crash-frequency data: A review and assessment of methodological alternatives. Transportation Research Part A: Policy and Practice 44, 291–305. doi:10.1016/j.tra.2010.02.001
Luttinen, R. T. (1999). Properties of Cowan’s M3 headway distribution. Transp. Res. Rec. 1678, 189–196. doi:10.3141/1678-23
Mannering, F. L., and Washburn, S. S. (2013). Principles of Highway Engineering and Traffic Analysis, 5th Edn. Singapore: John Wiley & Sons.
Mauro, R., and Branco, F. (2012). Two vehicular headways time dichotomic models. Mod. Appl. Sci. 6, 1–12. doi:10.5539/mas.v6n12p1
Mauro, R., and Branco, F. (2013). Update on the statistical analysis of traffic countings on two-lane rural highways. Mod. Appl. Sci. 7, 67. doi:10.5539/mas.v7n6p67
National Research Council, and Transportation Research Board. (2000). Highway Capacity Manual. Washington, DC: Transportation Research Board, National Research Council.
National Research Council, and Transportation Research Board. (2010). HCM 2010: Highway Capacity Manual. Washington, DC: Transportation Research Board.
Polus, A., Shmueli, S., and Shiftan, Y. (2005). Evaluation of the waiting time effect on critical gaps at roundabouts by a Logit model. Eur. J. Transp. Infrastruct. Res. 5, 1–12.
Raff, M. S., and Hart, J. W. (1950). A Volume Warrant for Urban Stop Signs. Saugatuck, CT: The Eno Foundation for Highway Traffic Control.
Rodegerdts, L., Bansen, J., Tiesler, C., Knudsen, J., Myers, E., Johnson, M., et al. (2010). National Cooperative Highway Research Program Report 672: Roundabouts: An Informational Guide, 2nd Edn. Washington DC: Transportation Research Board of the National Academic.
Rodegerdts, L., Blogg, M., Wemple, E., Myers, E., Kyte, M., Dixon, M., et al. (2007). Roundabouts in the United States. National Cooperative Highway Research Program Report 572. Washington DC: Transportation Research Board of the National Academic.
Siegloch, W. (1973). Die Leistungsermittlung an Knotenpunkten Ohne Lichsignalsteuerung (Capacity Unsignalized Intersections. Calculations for Unsignalized Intersections). Bonn: Schriftenreihe Strassenbau and Strassenverkehrstechnik [Road Construction and Traffic Technology, 154].
Silva, A. B., and Vasconcelos, L. (2011). “Roundabouts in Portugal-state of the art,” in International Roundabout Design and Capacity Seminar, 1st July 2011 (Stockholm, Sweden).
Solberg, P., and Oppenlander, J. (1964). Lag and gap acceptances at stop-controlled intersections. Highway Capacity Rec. 118, 48–67.
Tanner, J. C. (1962). A theoretical analysis of delays at an uncontrolled intersection. Biometrica 49, 163–170. doi:10.1093/biomet/49.1-2.163
Tanner, J. C. (1967). The capacity of an uncontrolled intersection. Biometrica 54, 657–658. doi:10.1093/biomet/54.3-4.657
Tian, Z., Vandeheya, M., Robinsona, B. W., Kittelsona, W., Kyteb, M., Troutbeckc, R., et al. (1999). Implementing the maximum likelihood methodology to measure driver’s critical gap. Transp. Res. Part A 33, 187–197.
Tollazzi, T. (2015). “Alternative types of roundabouts: an informational guide,” in Springer Tracts on Transportation and Traffic 6 ed. R. P. Roess (New York: Springer-Verlag, Springer International Publishing), 1–214.
Troutbeck, R. J. (1986). Average delay at an unsignalized intersection with two major streams each having a dichotomized headway distribution. Transp. Sci. 20, 272–286. doi:10.1287/trsc.20.4.272
Troutbeck, R. J. (1989). Evaluating the Performance of a Roundabout. Special Report 45. Vermont South: Australian Road Research Board.
Troutbeck, R. J. (1992). “Estimating the critical acceptance gap from traffic movements,” in Physical Infrastructure Center Report (Australia: Queensland University of Technology).
Troutbeck, R. J., and Brilon, W. (2016). “Chapter 8 revised monograph on traffic flow theory,” in Unsignalized Intersection Theory (Federal Highway Administration). Available at: https://www.fhwa.dot.gov
Vasconcelos, L., Seco, Á, and Silva, A. B. (2013). Comparison of procedures to estimate critical headways at roundabouts. Promet 25, 43–53. doi:10.7307/ptt.v25i1.1246
Vasconcelos, L., Silva, A. B., Seco, Á, and Rouxinol, G. (2012). Estimation of critical headways at unsignalized intersections – a microscopic approach. Adv. Transp. Stud. 29, 59–72.
Wu, N. (1997). An Universal Formula for Calculating Capacity at Roundabouts. Research Report of Institute for Traffic Engineering No.13, Ruhr-University Bochum, March 1997, 1–49.
Keywords: single-lane roundabout, multilane roundabout, gap acceptance behavior, capacity, critical headway, follow-up headway
Citation: Giuffrè O, Granà A and Tumminello ML (2016) Methodological Frontier in Operational Analysis for Roundabouts: A Review. Front. Built Environ. 2:28. doi: 10.3389/fbuil.2016.00028
Received: 17 May 2016; Accepted: 26 October 2016;
Published: 16 November 2016
Edited by:
Cholachat Rujikiatkamjorn, University of Wollongong, AustraliaReviewed by:
Pabitra Rajbongshi, National Institute of Technology Silchar, IndiaRasa Ušpalytė-Vitkūnienė, Vilnius Gediminas Technical University, Lithuania
Copyright: © 2016 Giuffrè, Granà and Tumminello. This is an open-access article distributed under the terms of the Creative Commons Attribution License (CC BY). The use, distribution or reproduction in other forums is permitted, provided the original author(s) or licensor are credited and that the original publication in this journal is cited, in accordance with accepted academic practice. No use, distribution or reproduction is permitted which does not comply with these terms.
*Correspondence: Anna Granà, YW5uYS5ncmFuYUB1bmlwYS5pdA==