Technology Leveraging for Infrastructure Asset Management: Challenges and Opportunities
- Department of Civil, Architectural, and Environmental Engineering, College of Engineering, Drexel University, Philadelphia, PA, United States
Transportation and other infrastructure systems, particularly in dense urban regions, are intertwined, interdependent, multi-scale, multi-domain and complex, and their behavior cannot be predicted even when element behaviors are known. Such systems should be managed just like financial assets, leveraging measurement-based, objective and reliable metrics for documenting their value, performance and condition, and based on their lifecycle and disutility risk for each distinct limit-states of performance as discussed in the following. In this paper writers attempt to offer a perspective for asset management of civil infrastructures with a focus on highway bridges and describe the tools that are considered necessary for rectifying the current shortcomings mainly arising from subjective and incomplete performance and condition evaluation practice. The adoption of sensing systems, which allows measurements of displacement, acceleration, strain, tilt and that can be collected wirelessly, has the potential of providing objective metrics needed for optimal asset management. The authors however caution that such a transition (from asset management based on visual inspection to data-driven asset management based on objective metrics) could be truly achieved only if combined with the proper training of a new generation of infrastructure inspectors and stakeholders. The paper attempts to provide a roadmap to achieve such a transition in asset management and describes the critical concepts that should be incorporated in training a new generation of civil engineers in charge of maintaining our transportation assets.
1. Infrastructures and Asset Management in the US
Infrastructures, such as transportation, water, power, fuel and communication are complex, multi-scale and multi-domain systems (with natural-human-engineered elements) providing critical services. They are key for the livability, sustainability and resilience of our communities. The need for infrastructure systems or their expansions are influenced by actual infrastructure service needs for a region, economy, financing and also politics and policy. Infrastructures in the US may fall under public, semi-public, private or hybrid (public-private partnership, PPP) ownership mainly based on history, policy and financing mechanisms. They are operated and preserved with many possible organizational constructs that are also influenced by their financing and revenue mechanisms (Figure 1).
In the last decades there has been increasing recognition that all infrastructure systems should be managed similarly to financial assets, which are invested and preserved by principles, such as diversification, time horizon and risk tolerance in addition to leveraging statistics and scenario analysis. Infrastructure asset management is the integrated, multidisciplinary set of strategies for sustaining physical assets, such as water treatment and distribution facilities, sewer lines, roads, dams, utility grids, bridges, railways, manufacturing plants and pipelines.
Figure 1 depicts some of the most critical parameters and systems that influence and therefore should be considered in infrastructure management. Given that most infrastructures in the US are many decades and in some cases centuries old—consider parts of the water distribution systems remaining from the time of the Colonies dating back to the 1700's, the railroads to the 1800's, Interstate Highway System to the 1960's and the Internet—starting as ARPANET—from the 1980's. It is natural for these infrastructure systems to remain under the influence of history, culture and the legal frameworks defining their ownership during the time of their early development.
Privately financed and operated infrastructures regulated as utilities (power, communication, internet, light rail, airlines, toll roads and bridges, clean water systems, and sewers in some regions) generally dedicate funds and adopt maintenance management policies for preservation over the long-term, as their owners stand to lose revenue in the case of service disruptions. Meanwhile, public infrastructures, such as streets, roads, parks and transit that depend on taxpayer funds from the state and especially the local governments face a different challenge. Local governments are often starved for resources (consider that the financial health of many Cities and Counties in the US remain challenged just due to their insufficiently funded pension obligations going back to many decades) and the short election cycles for elected leaders do not offer incentives for proactive long-term planning for the preservation of public assets. As a result of this, aged local infrastructures are often managed in a day-by-day triage mode and local government organizations are seldom evaluated based on the long-term performance of their infrastructure assets. In most cases, the federal government may provide the bulk of major rehabilitation and replacement costs of public infrastructures but the cost of routine preventive maintenance is considered as the responsibility of the local government. Therefore, many local governments prefer to defer maintenance and wait until assets require major rehabilitation or replacement, in which case federal funds may become available to finance most of the cost.
2. Challenges to Asset Management in the US
In the United States, there has been increasing Congressional awareness of checking the performance of federal, state and local agencies receiving federal infrastructure funds. For example, the 1993 Government Performance and Results Act (GPRA) required government agencies to pay increased attention to the outputs and outcomes that are expected from federal programs. The 1995 National Performance Review (NPR) ushered in a broader definition for performance management, which corresponds with evaluating progress toward achieving pre-defined objectives. NPR fostered an examination of the relationship between the outcomes and the investment. In 2001 the U.S. Government Accountability Office (GAO) emphasized that spending should be tied to outcomes (GAO-01-834). Shaw (2003) evaluated the performance measures of operational effectiveness for highways.
In 2010 the National Performance Management Advisory Commission (NPMAC) indicated that the relation between expenditures and predetermined outputs as organizational objectives needs to be realized. The 2012 MAP-21 Act (Moving Ahead for Progress in the 21st Century Act) of the US Congress required state agencies to focus on monitoring performance and outcomes and required that each State should be developing a risk-based transportation asset management plan (TAMP) for the National Highway System (NHS) to improve or preserve the condition of the assets and the performance of the system. MAP-21 specifically requested the Department of Transportation (DOT) secretary to ensure that all states implement performance measurement in order to adequately monitor the condition of interstate highway infrastructure and the national highway system.
MAP-21 defines asset management as a strategic and systematic process of operating, maintaining, and improving physical assets, with a focus on engineering and economic analysis based upon quality information, to identify a structured sequence of maintenance, preservation, repair, rehabilitation, and replacement actions that will achieve and sustain a desired state of good repair over the lifecycle of the assets at minimum practicable cost. According to Federal Highway Administration (FHWA), a State asset management plan shall, as a minimum, be in a form that the Secretary determines to be appropriate and include:
▪ A summary listing of the pavement and bridge assets on the National Highway System in the State, including a description of the condition of those assets;
▪ Asset management objectives and measures;
▪ Performance gap identification,
▪ Lifecycle cost and risk management analysis,
▪ A financial plan, and Investment strategies.
Although 2012 MAP-21 Act's request for each state to develop a risk-based TAMP is an excellent and desirable development, a number of challenges remain:
▪ Estimated cost of US public infrastructure renewal is in $ trillions and the estimated price we are paying as a Nation due to infrastructure disutility is also in $ trillions. Meanwhile the federal budget deficits and public animosity toward taxes imply that conventional mechanisms for infrastructure funding, such as the fuel tax and the municipal bonds funding may have become insufficient. New and innovative means of financing mechanisms for infrastructure need to be explored and incentivized. The consequences of such new financing mechanisms especially in terms of separating infrastructure management from politics, innovative organizational structures and inclusivity at urban regions also need to be carefully considered;
▪ Current condition measures for constructed assets, such as pavements and bridges are subjective, often highly simplistic and incomplete, sometimes erroneous, and they oversimplify the complex relations between condition and performance, discussed further in the following;
▪ Asset management is a complex multi-objective constrained optimization problem affected by significant epistemic uncertainty. Challenges in setting the objectives and measures are due to knowledge gaps regarding: the actual external and intrinsic loading mechanisms and resulting actions on constructed systems at various limit-states; operational demands and how capacity increase may lead to increases in demands; how site, soil, hydrology, geology, design, construction, existing conditions and changes in these impact serviceability, durability and safety performance; optimum maintenance materials, procedures and their timing; how condition changes, such as local deterioration and damage may impact capacity; and, a lack of reliable systemic and standard methods for justifying and securing adequate resource streams and distributing these to operate and preserve assets in order to assure the sustainability of system-wide services. An infrastructure manager has to incorporate significant uncertainty (both random and epistemic) in decision-making. Infrastructure performance is much more than just satisfactory operation, safety and failure modes of infrastructure elements and systems, discussed further in the following;
▪ We lack objective measurement based metrics that would reveal the Demands, Capacity, Disutility Probability, and consequences of disutility for evaluating risk in definitive and quantitative manners. A new engineering education paradigm culminating in an infrastructure engineering and management degree is urgently called for and this as well as other shortcomings of aged infrastructure management in the USA in the 21st Century has been discussed by many experts in addition to the writers (Aktan et al., 2016a,b).
3. Objectives
This paper has been written in response to a request by the Writers' colleagues for a contribution to a Frontiers collection of papers for “robust monitoring,” diagnostic methods and tools for engineered systems. Rather than directly delving into the technical specifics of “monitoring,” the writers opted to link the monitoring problem to the much broader infrastructure asset management concern. Unless monitoring of critical engineered systems is encouraged by policy, and the drivers and objectives of monitoring are crystal clear, the value of many applications where investments were made into technology leveraging have remained questionable. It is therefore important to assert that the discussions in this paper are focused on the broader problem of technology leveraging for asset management in general and highway bridge asset management in the US in particular.
A further clarification is required regarding the precise meaning of “monitoring technology.” Writers use the term “technology” to stand for “sensing,” imaging and non-destructive probing in relation to data from field experiments; information technology; modeling and simulation; and risk-based optimum decision-making for asset management.
The objectives of this paper follow from the discussions above and include the following:
▪ To introduce a contemporary definition of infrastructure performance that will serve as a foundation for infrastructure monitoring for asset management, by integrating distinct limit-states with subjective (heuristic, empirical, tacit knowledge) and objective (measured data-driven, mechanistic and explicit knowledge) elements that may help make up the objective functions for asset management;
▪ Discussion and recommendations for technology tools and integrative leveraging principles in order to make a transition from the present to objective, data-driven contemporary condition and performance metrics for risk-based asset management (including hazards risk management and resilience needs) of the highway transportation infrastructure;
▪ Describe recommended procedures for integrating and leveraging technology tools for common scenarios.
4. Infrastructure Performance
Infrastructure performance may be defined as the analysis of a multi-dimensional Capacity/Demand relationship as illustrated in Table 1. The probability of critical Demands exceeding the corresponding Capacities of an infrastructure system should be the basis for evaluating infrastructure performance at each of the four Distinct Performance Limit-States–Utility and Functionality; Serviceability and Durability; Life Safety and Stability of Failure; and Resilience. The Return Periods for peak demands for most infrastructure components typically vary as shown in the first row and the Performance Criteria for each limit-state of performance are narrated in the last row. Today, engineers and infrastructure owners often do not incorporate the interdependencies between each of these four limit-states and often assume that safety and resilience are separate problems from functionality and durability.
If a common facility is designed and constructed by adhering to the building codes, such as those issued by the International Building Code (IBC) in the USA (or by Eurocodes in the EU) for buildings, the probability of collapse is assumed to be ~10-6 for a 475 years seismic event or other demands governing the safety limit-state. However, we lack the data to confirm or dispute the actual performance. In Japan, a country with the most stringent seismic codes and enforcement, the 1995 Kobe earthquake is reported to have destroyed about 50,000 buildings. In the case of highway bridges designed by American Association of State Highway and Transportation Officials (AASHTO) Standards, there is data implying that the probability of failure is 1/10,000 based on an assumed lifecycle of 75 years (Bektas and Albughdadi, 2018).
Aside from the safety limit-state, most public infrastructures especially in the older urban regions in the USA fail to perform in the Utility and Functionality as well as the Serviceability and Durability limit-states much earlier than anticipated in their service-lives. The Second Row of Table 1 implies that asset management should actually integrate the management of operations, maintenance and preservation, structural safety and stability and resilience on the same platform. However, most engineers, public infrastructure owners and managers have fragmented the lifecycle asset management challenge by delegating operations, preservation, safety, and resilience to different and disconnected jurisdictions, bureaus, and organizations.
In some cases, such as the tall building stock in San Francisco, doubts may arise about potential performance at the safety and resilience limit-states after several decades of a building boom (Fueller et al., 2018). In most cases, however, it takes an actual natural or man-caused hazard to occur to reveal the actual performance of systems at the safety and resilience limit-states. Our current civil and structural engineering practice based on code prescriptions and subjective visual inspections (Moore et al., 2001) is grossly insufficient given the increasing nature and frequency of hazards and associated risks due to infrastructurefailures and disutility. Further, many civil engineers are not even aware of the uncertainty in predicting the performance of a constructed system which they design by code provisions.
The desirable approach to managing public infrastructures, especially at dense urban regions, such as the NE Corridor in USA is to demand quantitative descriptions and objective and measurement-based metrics for Loads, Demands, and Capacities from infrastructures and data for evaluating whether the disutility probability is acceptable at each and every limit-state of performance. The acceptable disutility probability would naturally depend on the affected human population and the economic consequences. For example, we may tolerate less than perfect roughness indices and congestion at some highways at certain times of the day and/or the week; and a bridge deck to require maintenance in just 5 years after construction due to wear and deterioration. On the other hand, we cannot continue to practice infrastructure management with the subjective pseudo-reality of how constructed systems perform and how engineered, social and natural elements of complex infrastructure systems interact. We have to bring infrastructure management to be based on a rational, objective set of metrics defining the performance expected of them and their organizations. This requires the ability to measure and monitor performance as per Edwards Deming's teachings since we cannot manage what we cannot measure.
Fortunately, the information technology revolution of the last several decades offer the tools needed for measuring and monitoring performance.
5. The Information Technology Revolution
Information Technology (IT) has been defined as the study or use of systems (especially computers and telecommunications) for storing, retrieving, and sending information. Intel 4004 is considered as the first commercial 4-bit IC micro-processor which has advanced continuously since its invention in 1971. The 4004 was only capable of 60,000 instructions per second, but its successors including the Intel 8086/8088 family brought ever-growing speed and power to computers. Today, thanks to smartphones and tablets we enjoy ubiquitous computing, data and image capture and communication. Cloud computing and storage has become a significant industry, removing many of the limits to computing, archiving and retrieval of data and information. Software is available to the public at a very low cost for almost any conceivable purpose, including all levels of games, K-12 education, productivity, finance, engineering, arts, architecture, and sciences amongst others.
Along with the advances in IT, parallel advances in experimental technology (sensors, actuators, data acquisition systems, controllers, and pumps; wide-area high-definition digital imaging; a variety of NDT probes; and more recently, wireless sensor networks and SCADA (supervisory control and data acquisition) Systems have become available. Most of these hardware and associated data acquisition and control software (e.g., NI's LabVIEW) have been used in laboratories and in some cases in the field on actual infrastructures. Naturally, a variety of sensors have been and are being used in the airplane, auto, HVAC, and elevator systems and in defense applications. More recently, coupled multi-level and broad area real-time imaging, sensing, computing communication and actuation-control systems have been demonstrated in association with homeland security purposes and for infrastructure management.
Explosion of IT has created immense amounts of data—to put things in perspective, the size of the Internet doubles about every 2 years. For the beginning of 2016, the Counter expects around 7.7 Zettabyte (ZB) on to data that is distributed worldwide to Internet servers (1 ZB = 1021 bytes or 1 million petabytes = 1 billion terabytes = 1 trillion gigabytes). Along with this data explosion, privacy, and cyber-security have become significant societal concerns. A Wall Street Journal article describes the major role “big data” is playing in the US economy (Stoll, 2018).
It follows that in spite of the great abundance of data, the challenge of organizing, synchronizing, visualizing and interpreting this data into information, followed by knowledge and wisdom for transforming infrastructure management, is pending. However, we do not yet clearly know the scope of useful data on organizations and assets that we need for infrastructure management, and how we can collect this data. What constitutes useful data (and images) for objective measurement of infrastructure performance at various limit-states, especially at the service and safety limit-states, and how we may capture, fuse and interpret this data will be discussed later. One thing is certain—IT explosion, if properly leveraged, offers a great opportunity for rationalizing and optimizing infrastructure management! However, the path to improving infrastructure management requires being able to manage data and understanding the path from data to wisdom.
5.1. Data-Information-Knowledge-Wisdom
In order to identify the most critical data that we need and how to capture this data for prudent management decisions, first we need to understand the distinction and hierarchy of data, information, knowledge and wisdom.
Figure 2 describes the stages of identifying and understanding complex systems behavior by transforming data to information by understanding any existing relationships between data (e.g., by correlation analyses), followed by understanding the patterns embedded in information to lead to knowledge. Finally, by understanding the physical, chemical and mathematical principles embedded in knowledge we may acquire the wisdom that is essential for generalizing knowledge and developing prudent decision-making tools, such as scenario generation and simulation. While data collection is only the first step of this process, we also have to appreciate that there are additional manners of acquiring knowledge discussed further in relation to Figure 3.
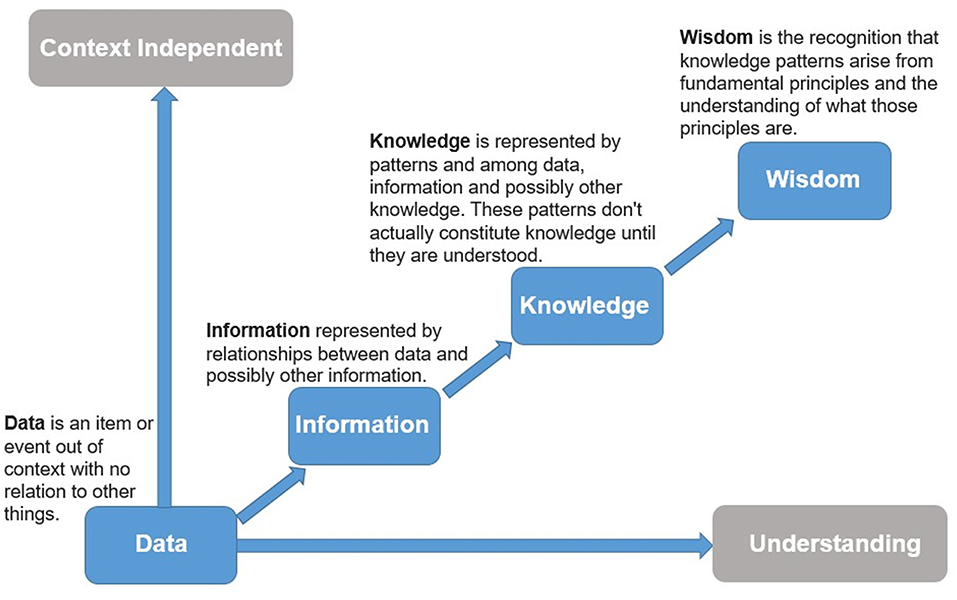
Figure 2. The process of acquiring knowledge from data (Equity, 2018).
5.2. Knowledge Classification and Acquisition
Figure 3 (Knowledge-Management-Tools, 2017) illustrates a commonly accepted knowledge classification: Tacit vs. Explicit (or mechanistic). Tacit knowledge on a system needs to be accumulated before the fundamental principles, critical parameters and the mechanisms that describe and help model the system for simulation may be established as “explicit or mechanistic knowledge” about the system.
The attributes of tacit knowledge are described in Figure 3, indicating that data and IT are often not sufficient for its accumulation especially when we deal with complex systems, such as infrastructures. Some additional sources include: (a) collection and verification of existing heuristics, (b) reason and logic, (c) mathematical proof, (d) trial and error, (e) intuition, (f) experience gained through apprenticeship under the mentorship of an expert, (g) observation and empiricism, and most importantly, (h) the scientific method. Once tacit knowledge about infrastructures which are complex and large natural-human-engineered socio-technical and interdependent system-of-systems is accumulated, this may then be transformed into explicit knowledge through standards, codes, algorithms and numerical-statistical models.
6. Technology Integration
We now return to the challenge of objective data-driven asset management of infrastructures. What type of data is needed; how it may be collected; and, how this data would help complement the available tacit knowledge and help culminate in explicit knowledge are some of the fundamental questions. In addition to IT, a technology leveraging toolbox would include:
1. Technology tools for field observations and experiments, i.e., ability to study by observation (similar to telescopes and microscopes), as well as design and execute field experiments by leveraging sensing and imaging, along with leveraging controlled or uncontrolled loading and/or excitation. Field experimental technology also requires an ability to capture the environmental conditions and changes in these during an experiment, such as wind, temperatures, radiation, humidity, etc. Additional tools are needed for:
2. Data management, quality assurance, processing, synchronization, visualization, and correlations; statistical modeling;
3. Analytical modeling and simulation, including parameter identification and an Avatar—or calibration of a digital twin;
4. Decision-making based on lifecycle cost, Pareto optimization, and risk analysis technologies.
These technology tools are naturally integrated by following the scientific method or structural system identification. Figure 4 offers a schematic of the structural system-identification method which was formalized and reported in a book by an ASCE Committee Catbas et al. (2013), and also discussed by Aktan and Brownjohn (2013). However, the roots of system-identification as well as innovations leading to the field experimental tools including sensing go back to many decades. We need to acknowledge many contemporary colleagues and especially giants from earlier generations who have contributed to the concepts and tools that have made system-identification of operating buildings, bridges and other constructed systems in the field possible. Many of these colleagues are acknowledged in the Summary and Acknowledgments (section 8) and the writers are aware that they are inevitably failing to include many worthy contributors for which they apologize.
A successful culmination of the process (more than one cycle may be required) in Figure 4, may lead to a digital twin of the structural-foundation-soil system within the resolution of a mixed macro-micro level representation of a system. The digital twin may potentially serve as a birth-certificate for a new system, and as a basis for condition evaluation and NDT and SHM applications for long-term condition monitoring. These are critical for preventive maintenance as well as evaluating the condition of a system following a hazard such an accident leading to damage.
A closer scrutiny of Figure 4 reveals the range of disciplines and specializations that are needed for an application to an operating infrastructure component or system. The process is successful only if each of the Six Steps are overseen by the same “project manager” with experience and domain knowledge associated with each and every one of the Steps, preferably a structural engineer who would also possess domain knowledge and heuristics that has been accumulated about the structural system being identified.
Many researchers specialize in only analytical modeling, or only experiment, or only computation and parameter identification, or only risk and reliability theory. They may simply obtain the products from each Step executed by others and then try to integrate these. Such a disconnected approach often fails to produce a reliable, high-fidelity digital twin that will distribute its loads through the same paths as the actual system, or one that does not deform and displace like the actual system, i.e., share the kinematics of the actual system. The maximum demands computed under different loading schemes during the simulations may not match reality, and most importantly, the corresponding actual capacity distribution may be inaccurate. It is therefore critical to have the same experienced structural engineer participate in, oversee and integrate each Step of the process in Figure 4 to help properly integrate the contributions by different experts as depicted in Figure 4.
7. Sensing Technologies
Having introduced the fundamentals or infrastructure performance; why prudent asset management requires objective metrics and measurement data on performance; and the challenges in integrating and leveraging technology; we now need to review the current practice in sensing and imaging. We will first focus on sensing as the most fundamental element in field experimentation and monitoring. For a crash course on sensors (sensorwiki, 2018) is an excellent resource.
A National Academy Report (National Research Council, 1995) offered definitions and issues related to sensing in manufacturing and for structural monitoring and control in aerospace, space, defense and homeland security applications. The definitions and glossary in this book may be used for sensors for civil engineering applications. Table 2 extracted from this book lists some of the critical sensor characteristics for static and dynamic applications.
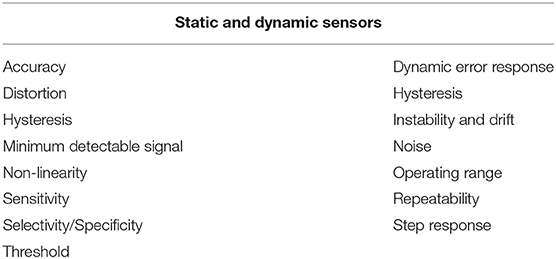
Table 2. Sensor characteristics (National Research Council, 1995).
Electronic sensing in civil engineering goes back to 1930's (Treatise on Photoelasticity, published in 1930 by Cambridge Press). The bonded wire resistance strain gage for aluminum or steel was invented at MIT in 1938. This was the predecessor of current foil gages manufactured and sold by Vishay, Micro Measurements (recently purchased by Vishay), or HBM which also offer strain-gages for concrete. Weldable versions of these gages are manufactured by and available from HITEC. Tokyo Sokki Kenkyujo (TML) is a sensor manufacturer in Japan that also offers specialized strain gages, such as for post-yield measurements. TML products may be purchased in the USA from Texas Measurements. Vibrating wire versions of strain transducers, with various gage-lengths and installation methods, have been commercially available for decades and distributed by several companies, such as Telemac in France, Geokon in the US and Roctest in Canada to name a few. Similarly, fiber optic sensors were commercially available since 1990's with pioneers, such as FISO in Canada, Smartec in Switzerland, Omnisens in Switzerland, Blue Road Research and Micron-Optics in the US, and Ando in Japan. Obviously the availability of sensors is no longer a concern, but the art is in designing a field experiment by selecting, calibrating, positioning, installation and integrating the outputs of the best sensors for each measurement needs and constraints in an optimum manner.
The invention of the strain gage enabled the design and development of many transducers capable of measuring deformations, displacements and forces by leveraging strain gages. Vishay, HBM, and TML offer many of these. For example, a clip-gage is a raised arch wired to accommodate a full strain-gage bridge which amplifies and measures the strains as the arch is extended or contracted at the base. By using such a micro-structural system, the strains of which are measured by strain gages, we may correlate the strains to the elongation or contraction between two points on a member. A TML clip gage is illustrated in Figure 5. The PI displacement transducer has a simple structure: a combination of strain gauges and an arch-shaped spring plate, the former attached to the latter. Six models designed for gauge lengths of 50–300 mm are available. This transducer is used to measure the crack opening displacement occurring within each gauge length on the surface of concrete or to measure local deformations between elements of various structures. Many other types of clip gages have been used in research as long as strain gages have become available.
Linear Variable Differential Transformers (LVDT's) were invented in the 1940's for displacement measurements requiring higher resolution and sensitivity than what is offered by strain-gage based transducers, such as the clip gage. LVDT's operate by leveraging inductance change and are used in the aerospace industry. LVDT's of various sizes and sensitivity are manufactured by Honeywell, Intertechnology (Celesco), TML, and others. Some LVDT's use a spring-loaded thin steel wire which enables them to measure displacement between distant points.
The vibrating wire strain gage and other vibrating-wire transducers for temperature, tilt, displacement and pressure or force have been used for geotechnical measurements. Geokon Inc. is one of the oldest and most extensive US manufacturer of vibrating wire transducers for geotechnical and structural applications. Roctest is another sensor manufacturer based in Canada and established in 1967, offering a variety of vibrating wire as well as fiber-optic based transducers.
Another class of transducers that is used in field experimentation is the accelerometer. PCB (recently purchased by MTS) offers a wide-range of products suitable for testing constructed systems. Brüel and Kjær, Kistler Group, and TOYO Corporation also offer various accelerometers [A listing of accelerometer manufacturers is available at sens2b-sensors (2018)] with a wide range of specifications. Geophones which measure velocity have also been adapted from mining and used in some bridge tests.
Tiltmeters are designed to measure very small changes from the vertical level, either on the ground or in structures. Tiltmeters are used for monitoring dams, the small movements of potential landslides, the orientation and volume of hydraulic fractures, and the response of structures to various influences, such as loading and foundation settlement. Tiltmeters may be purely mechanical or incorporate vibrating-wire or electrolytic sensors for electronic measurement. A sensitive instrument can detect changes of as little as one arc second. Tuff tiltmeters from Jewell and vibrating wire tiltmeters from Geokon were used by the writer in the past for monitoring bridge superstructure and substructure rotations.
The writers started to explore sensors that may be suitable for monitoring bridges following the Big Bayou Canot rail accident (2018) on September 22, 1993. FHWA and Ohio DOT supported an investigation of available sensors that could be used for bridge monitoring, which were purchased and extensively studied both in the laboratory and in the field over several years. These experiences accumulated and have led to a 2002 FHWA Report “Development of a model health monitoring guide for major bridges” (Aktan et al., 2003)1.
7.1. Sensor Selection and Calibration Fundamentals
There are many criteria in selecting a sensor for measuring a physical, chemical or electrical quantity in the field. First we must understand that the reading of any sensor in the field will be impacted by many phenomena, and sometimes the sensor reading may prove more sensitive to a cause or mechanism that is unknown as opposed to what the designer of an experiment may think is being measured. In general, we may classify common transducer measurement errors as:
1. Transducer installation assembly error, which is due to the self-response of the transducer and the attachment assembly as a mini-structure. The kinematics of a sensor together with its installation assembly is what is measured. So if the reference point of a displacement sensor rotates as well as displaces its reading would be affected by both of these vectors. Resonating due to a dynamic disturbance, or responding to changes in environmental conditions, such as the effects of wind or temperature on reference wires, and other environmental effects on transducers cannot be corrected by a single calibration constant or even a calibration curve.
2. Instrument/data acquisition variance errors. Spurious readings and noise due to electromagnetic interference on data acquisition is the most critical one to detect and correct. Variance errors may be controlled by appropriate data acquisition and processing strategies, such as filtering, common-mode rejection, and averaging of the data. Long time-windows of data rather than just a single reading is required.
3. Instrument/data acquisition bias errors. Drift or changes in calibration due to an impact or temperature effects on an instrument are the most critical ones to detect and correct. Due to bias errors, one has to design in-situ calibration check strategies;
4. Apparent structural response, such as unrestrained temperature strains and rigid-body displacements as well as rotations caused by settlements, temperature, creep or shrinkage;
5. Structural response associated with stress and force, including self-equilibrating stresses; since the intrinsic stresses and other responses change with environmental conditions or transient loading, sensors inevitably will include these responses as readings.
The Five most common sources of measurement error are shown in the following expression:
ϵ1: Errors due to transducer mechanical assembly (epoxy slip, support offsets, etc.)
ϵ2: Instrument/data acq. errors (variance errors, such as noise, etc.)
ϵ3: Instrument/data acq. errors (bias errors, such as temp. effects on sensors)
ϵ4: Apparent structural response (Temp. strains, etc.)
ϵ5: Response associated with stress and force
We should recognize that understanding the magnitudes of these errors and mitigating them is not trivial. In fact, the errors will depend on the sensor, its transduction, the structural-mechanical system of the transducer together with its installation assembly, power, A/D conversion, environmental conditions and their changes, bandwidth and duration of data acquisition and most importantly, whether the experiment with sensor types and distribution or density is designed properly. It follows that performing field measurements and checking and validating data reliability and quality requires significant Tacit Knowledge.
The single most effective manner for assuring data quality is to perform calibration of individual sensors, followed by the system of sensors and DAQ in the laboratory (Figure 6). Individual sensor and sensor-DAQ system calibrations are discussed in the FHWA Report on Model Health Monitoring Guide (2002) referenced earlier.
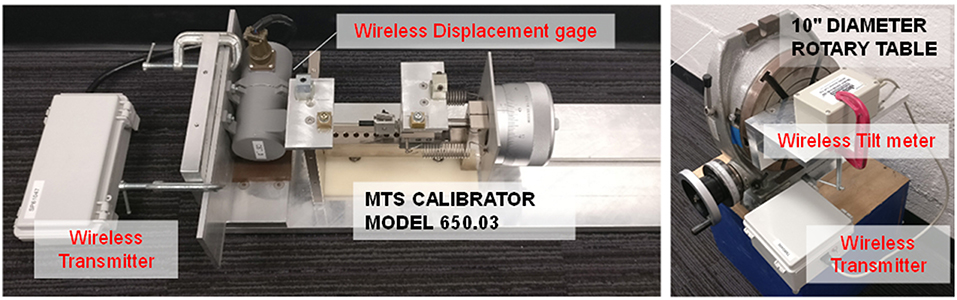
Figure 6. Jigs for ground truth calibration of sensors before systems-level calibration in the field.
A valid question becomes: “How can we leverage field experiments for measuring the behavior and performance of constructed systems given the challenges in obtaining reliable measurements in the field?” This is a very important question and requires federal and state governments to invest into training at field measurement laboratories (e.g., instrumented monitoring of operating bridges) to demonstrate sensors, calibration, installation, measurement system design fundamentals and data quality assurance. These laboratories (Virtual Non-destructive Evaluation Library for Highway Structures, 2018)2 may serve as best practices for training motivated engineers to be able to obtain reliable field measurements and obtain certificates and even professional degrees.
7.2. Wireless Sensing
One of the purposes of this paper has been to introduce the products of a recent research project performed under FHWA Exploratory Advanced Research Program (EARP) funding. In this project, given the challenges in developing new reliable wireless sensors for field measurements, the objective was to explore whether it is possible to transform the most reliable and proven sensors into wireless operation.
The sensors that were selected were: (a) Resistive strain gages or rosettes; (b) Clip Gage—as a 4-arm strain gage transducer; (c) Vibrating wire gage and other vibrating-wire transducers; (d) Displacement transducers employing wire potentiometers; (e) Electrolytic tilt-meters; (f) Seismic accelerometers (see Figure 7). Each type of sensor was successfully untethered from power, data acquisition, and communication cables by locating in-situ power, conditioning, data acquisition and communication IC boards in a small box, and the sensor readings were transmitted wirelessly to any computer. All the sensors previously described have been extensively tested in the laboratory and tested in the field and have been presented to a committee of experts (representatives from NASA, Volpe Center, PennDOT, FHWA, Minnesota DOT) as part of a Technology Readiness Level (TRL) assessment on June 6th, 2017.
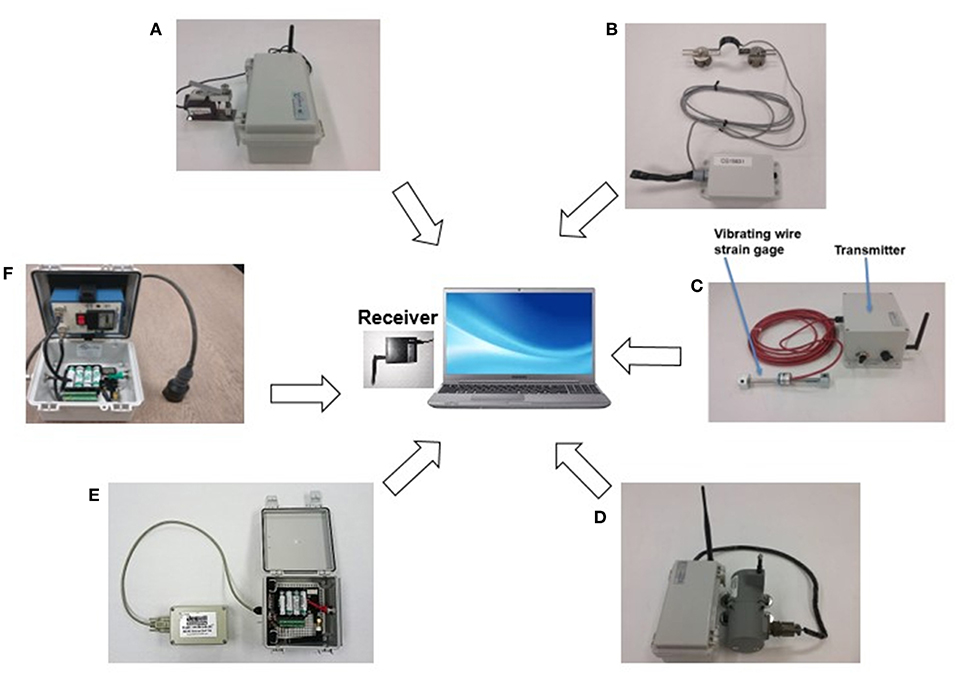
Figure 7. Suite of traditional proven sensors transformed for wireless operation, (A) Wireless magnetic strain gage, (B) Wireless clip gage (crack meter, strain), (C) Wireless vibrating wire strain gage, (D) Wireless displacement gage, (E) Wireless tiltmeter, (F) PCB wireless accelerometer.
The TRL panel agreed that the technology had reached a TRL between 4—“Components validated in laboratory environment”—and 5 “Integrated components demonstrated in a laboratory environment.” Since then, the researchers have continued the development of the wireless sensing platform and followed the TRL feedback received to identify scenarios and use case development, and to develop a set of requirements for the identified use cases based on stakeholder needs.
With the above set of sensors or transducers, it is possible to measure: strains, deformations or distortions, displacements, rotations, and accelerations along with a wide frequency bandwidth. For instance, the vibrating wire gages offer a highly robust measurement of temperature, and distortion that is stress-related (not temperature related) measured at a 1 Hz frequency. These sensors (in conjunction with imaging) offer an excellent capability for measuring structural and environmental responses of any highway structure, at an appropriate frequency. Typically, for operational monitoring, acceleration data is obtained at <500 Hz; strain, displacement and rotation response data is collected at <200 Hz and data on environmental conditions and their effects on bridge responses is obtained with vibrating-wire sensors at 1 Hz.
Untethered sensors offer further opportunities for rapid deployment (minutes/hours) and field monitoring of structures for weeks since power sources that are standard batteries last for weeks, depending on the bandwidth and data capture time-window. Installation assemblies by leveraging industrial magnets are being designed to enable rapid and easy field installation even for structures with challenging access constraints. This capability promises quantitative measurements of structural strains, distortions, displacements, rotations and accelerations of a bridge during operations, augmenting the current visual inspection and subjective assessments to include objective “pulse and blood-pressure” measurements under traffic and even special loads. Such measurements may be conducted for several hours as the inspectors prepare for the inspection of a bridge. Normally, operational monitoring would be recommended for 24 h for some critical bridges to understand and include the impact of daily environmental changes on the structure's responses.
As an example, the traffic video in Figure 8 that is stored during operational monitoring is synchronized with displacement time histories (or any other response recorded) of sensors installed on selected girders of the spans 3 and 4 of a viaduct structure supporting. A screenshot of one significant displacement event example (peak displacement near 0.6 in) is shown in Figure 8. In the video, the sensor layout and locations are displayed on the left hand side on a 3D model of the bridge. The right hand side shows the video of traffic and the corresponding real-time displacement recordings. This image-data integration helps users comprehend the effect of the traffic (for instance a large truck traveling westbound that just crossed Span 3 at 6:50:05am and that caused displacement responses recorded at critical locations by the wireless sensors 1, and 5 at the mid-span of Spans 3 and 4). Such synchronized image and data combinations are useful for objectively documenting vehicles that may cause significant demands from a viaduct.
Responses from wireless sensors are consistent with the responses measured by traditional wired displacement gages (sensor 3 and 13) that require significantly longer installation time (and cost) for routing several hundred feet of power and communication cables to a data acquisition system and a portable generator. Cables and especially their connections are also a major source of vulnerability and errors in measurements and their elimination augments the performance of the wireless sensing system caused by the traffic loading as well as caused by temperature changes during the period of testing.
7.3. Future Opportunities Afforded by Rapidly Deployed Wireless Sensing
Sensors may serve to provide quantitative information, situational awareness and insight on structural behavior during many types of bridge inspection listed in the following (Hearn, 2007). While each type of inspection in Table 3 would greatly benefit from practically deployed wireless sensing, the value of sensing in the case of Special and Damage Inspections would be especially important and critical for reducing the uncertainty of visual inspection. Special and/or damage inspections are used for bridge reconstruction especially in the case of accelerated construction; bridges that exhibit unexpected damage and/or tilting and deformations; fatigue crack monitoring; load testing for load capacity rating, etc. There is no question that wireless technology offers significant advantages in feasibility over the standard wired sensors in these applications. We anticipate that wireless sensing, especially the ones obtained by transforming off-the-shelf sensors that have been proven robust and reliable over decades of use can impact the state of practice in these applications immediately as opposed to more long-term, routine measurements and structural-identification applications which are typically longer term investments for the owner agencies.
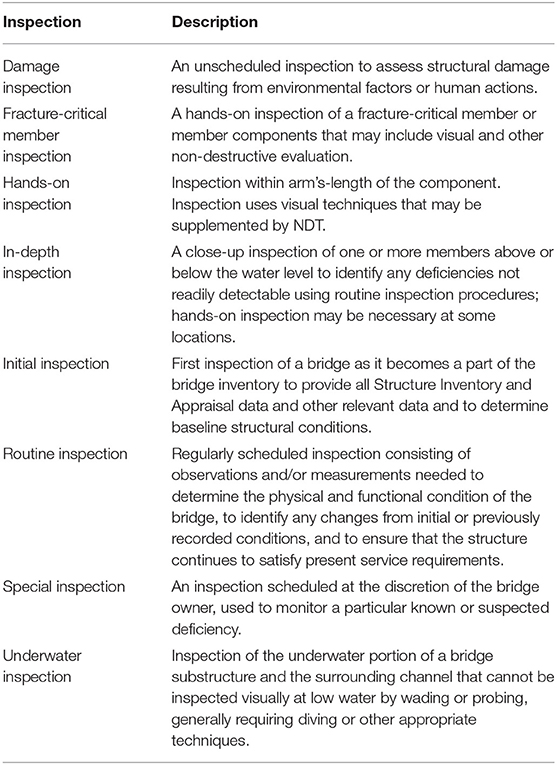
Table 3. U.S. Federally mandated bridge inspection types (Bektas and Albughdadi, 2018).
To reiterate, wireless sensing may offer great value to a bridge owner in the case of:
▪ Damage inspections
▪ Special inspections
▪ Complementing routine visual inspection of a bridge by measuring “objective performance and health indicators,” such as operating deflections, strains, accelerations which are necessary for transitioning to asset management based on objective data
And more specifically, especially if baseline measurements at commissioning are available:
▪ Measurement of displacement and rotation due to foundation settlements caused by soil instability, impact, scour
▪ Bridge replacement while reusing the foundations
▪ Monitoring to assure safety during Accelerated Bridge Construction
▪ Post-event safety assessment (Accidental hits, scour, fire, flood, earthquake, etc.)
▪ Monitoring bridges under permit and super-loads to ensure that no permanent local damage occurs
▪ Weigh-in-motion (WIM) for load enforcement or truck-load characterization
▪ Dynamic characterization of a bridge by operational modal analysis
▪ Research, such as the long term bridge and pavement performance programs
Untethered sensors offer opportunities for rapid deployment (installation in minutes/hours) unlike tethered sensors and allow field monitoring of structures for weeks (since batteries were verified to last for weeks and up to several months depending on the bandwidth and data capture time-windows). Installation assemblies by leveraging industrial magnets were designed to enable even faster and easier field installation especially for structures with challenging access constraints. Elimination of long cables and connections with these help reduce significant sources of error and uncertainty in measurements under field conditions.
Rapid deployment capability promises quantitative measurements of structural strains, distortions, displacements, rotations and accelerations of a bridge during operations, to augment visual inspection and subjective condition ratings by including objective deformation measurements under normal traffic and even special proof-level loads.
Enhancing visual inspection with rapid wireless sensing measurements appear as a most promising application. A follow-up question may regard the merits of recording bridge “pulses” during operations every 2 years. A structural system-identification of a bridge type may offer foundational knowledge for the interpretations of measured responses over long-term. This idea was in fact suggested two decades ago (Hunt et al., 1998).
The Long-Term Bridge Performance (LTBP) research program administered by the FHWA was based on a similar concept. The insight and knowledge from the structural system-identification of reference structures (field laboratories serving as benchmarks and also as training facilities) and the bridge population represented by the reference structure, would enable an excellent understanding of the health of a bridge population.
Naturally, rapidly deployable wireless sensors offer many other uses. Load testing for bridge load rating, construction monitoring especially in the case of accelerated bridge construction, transport and erection, foundation reuse, permit loading, and, structural health monitoring all require reliable field measurements for short and long durations. The single most important prerequisite is to train and educate a sufficient number of technicians and engineers who will have an understanding of what the measurements physically mean, and exactly what load or other environmental event is causing the measurements.
When we stop thinking about bridges as simple beams, and understand the complexity of the behavior of site-soil-foundations-substructures-approaches-bearings-and-superstructures as an integrated system, we will be able to better understand bridge safety and reliability - much better than what is implicit in the code and incorporating fabrication and construction quality and maintenance.
7.4. Potential of High Resolution Imaging
While companies, such as Google are completing the mapping of a large portion of the built environment and users can access street view images of locations across the globe, bridge inspection often still relies on traditional means of documenting the condition of infrastructures by photographs, technical drawings and other information considered relevant by an inspector. Images collected by bridge inspectors are very powerful in documenting local regions of concern but they represent spatially isolated data points and often miss the relationship of these to the global system. The limits in the field of view of human vision in understanding the broader patterns indicating possible condition changes along a large system are well-established by photographers.
Technology become recently available to achieve high resolution maps of bridge decks by stitching high resolution images collected by cameras installed on road vehicles moving at traffic speed (Hiasa et al., 2016). Such rapid data collection, is not only useful because it keeps the inspector protected from the risks posed by moving traffic and reduce the need for traffic control, but it is particularly valuable because it provides a visual overall documentation of the actual condition of the entire deck surface. Further, high-resolution RGB images can be integrated with information which can be extracted from properly timed infrared imaging and reveal possible existence and range of delamination hidden under the surface (Hiasa et al., 2016).
An example of the product extracted from a rapid high speed survey is shown in Figure 9 where a complete HD imaging of a bridge surface is shown together with zoomed views of the HD images at locations of interest and the location of possible delaminations detected by high speed IR imaging. The study of a full-bridge deck surface image could reveal patterns that even an inspector walking on a bridge cannot easily discern. Such an image would also help design a more in-depth NDT application if needed. Periodically collected images complemented with crack mapping could be really powerful ways to document the progressive deterioration of the deck.
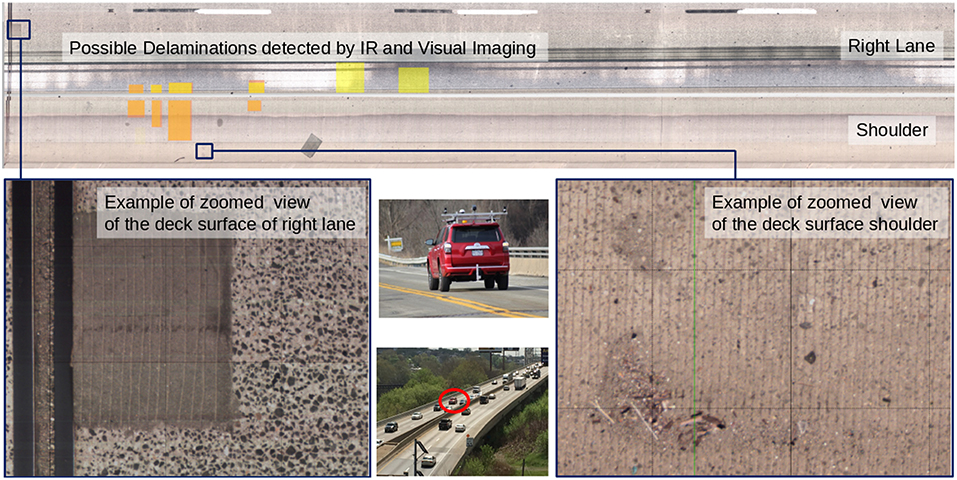
Figure 9. High speed high resolution visual and infrared imaging of bridge deck (courtesy of NEXCO).
7.5. A Holistic Technology Integration and Leveraging Strategy for Bridge Asset Management
Given that this paper was written in response to a call for “robust monitoring, diagnostic methods and tools for engineered systems,” writers believe that it is important to consider how all of the critical experimental, information, modeling and simulation, and, decision tools may come together in relation to typical highway bridge assets. Table 4 presents a logical hierarchy for field technology applications—starting from (a) Inspection; (b) Measurement of Geometry and Material Properties; (c) Evaluation of Condition and Performance; (d) Diagnosis, Prognosis, Risk Evaluation and Options for Intervention Designs; and, (e) Health and Performance Monitoring based Asset Management. Each Column of Table 4 would be applied from Left to Right, and from Cell 1 to Cell 4 in sequence as needed and justified.
It is possible to argue that the expertise and cost requirements of integrated technology leveraging in Table 4 may be currently overwhelming for many infrastructure owners. However, in the case of major infrastructure components, such as long-span bridges, viaducts and tunnels that cost $ billions, and that are critical for the economic vitality of major urban regions, it is difficult not to justify moving toward a technology-based asset-management suggested in Table 4. In the case of typical highway bridges, Table 4 may be applied to a selected population which may provide an excellent asset management procedure for the entire population.
8. Summary and Acknowledgments
Critical infrastructure systems like water, power, communication and transportation are key for the livability, sustainability, and resilience of urban regions. Together with the natural and the built environments, society, economy and the government services, infrastructure services serve as foundation of our cities. Meanwhile, stresses due to urbanization and climate change are challenging the performance of urban infrastructure systems and their services.
Infrastructure systems are intertwined, interdependent, multi-scale, multi-domain and complex, where system behavior cannot be predicted even when element behaviors are known. The complexity is compounded in dense urban regions where infrastructures are bundled in close proximity, where the failure of one impacts all others.
There is now recognition that infrastructure systems need to be managed just like financial assets, yet we are a long way from measurement-based, objective and reliable metrics for documenting their value, performance and condition; as well as how changes in their condition may impact their performance. In this paper writers strive to offer a perspective for meaningful asset management of infrastructures and describe the tools that are needed for rectifying the current shortcomings mainly arising from subjective and incomplete performance and condition evaluation.
By defining “Infrastructure Performance” and “Technology Leveraging” for performance and condition evaluation in terms of rational indices, the paper describes how asset management can be based on objective data in addition to tacit knowledge and how data may then be transformed into explicit knowledge. It would take decades before such a transformation may be completed, however there has to be foundation for proper technology leveraging. In this section writers wish to acknowledge a few of their colleagues who have made significant contributions to the state of the art in the structural system-identification (St-Id) concept, experimental, analytical and computational arts, reliability and decision theory, lifecycle cost analysis and asset management concepts.
In the case of structural system-identification concept as applied to constructed systems, we recognize Agbabian et al. (1991), Shinozuka and Ghanem (1995), Ibáez (1973), Hart and Yao (1977), Beck and Jennings (1980), Yun and Shinozuka (1980), Shinozuka et al. (1982), Yao and Natke (1994), and as significant contributions. Actual field experiments on full-scale constructed systems were pioneered by late Professors Hudson (1964) from CALTECH and Clough from UC Berkeley who collaborated in developing rotary-weight shakers for dynamic testing of buildings and dams in the 1960's. Such devices were also used for destructive tests of a decommissioned structure (Galambos and Mayes, 1978). Proper Implementation of St-Id requires careful modeling of constructed systems (Catbas et al., 2013), adequate field testing capabilities (Aktan et al., 2016b), data interpretation (Law et al., 2014; Smith, 2016) and robust parameter identification strategies (Rafael and Smith, 2003; Posenato et al., 2008, Goulet et al., 2010).
During the 1970's, mechanical engineers interested in experimental structural dynamics developed the art of modal analysis and civil engineers started exploring how modal analysis theory may be applied to structural identification of constructed systems (First IMAC conference held at 1982 in Orlando, FL). Brown and Allemang at the University of Cincinnati offered a review of the history of modal analysis at IMAC in 2007 (Brown and Allemang, 2007)3.
In the 1990's, engineers from different disciplines have embarked on an exploration of health monitoring as a research area. The First International Workshop on Structural Health Monitoring (IWSHM) was held in 1997 at Stanford University, organized by Professor Fu-Kuo Chang following the first Non-Destructive Testing in Civil Engineering Conference held at Berlin in 1995 and organized by BAM (Schickert, 1997). These milestones of technology applications for constructed systems were followed by remarkable research efforts and summarized in reports which captured the goals and the potential of SHM for civil and other structures (Farrar, 2001; Chang et al., 2003; Brownjohn, 2006; Farrar and Worden, 2006). Sensing systems capable of being deployed for long periods of time were demonstrated on constructed systems (Glisic et al., 2013; Sigurdardottir and Glisic, 2013; Leung et al., 2015).
While in-service or decommissioned constructed systems have been tested by perturbing with many methods (shakers, loaded trucks, pull-release by cranes, rock-anchors and actuators, implosion, etc.), in the case of long-span bridges and high-rise buildings perhaps the only practical approach is measuring their ambient vibrations caused by operations and ambient environmental loads, such as by wind. This approach is also referred to as operational modal analysis (Abdel-Ghaffar and Housner, 1977, 1978; Peeters and Roeck, 2001; Ko et al., 2002; Grimmelsman et al., 2007; Conte et al., 2008; Siringoringo and Fujino, 2008; Pakzad and Fenves, 2009; Brownjohn et al., 2010). In the case of ambient monitoring of buildings, exemplary research by Kareem et al. (1999) should be mentioned.
In the last 20 years, exploring structural health monitoring applications by leveraging wireless accelerometers became a trend. Among the many research groups who have advanced SHM for bridges leveraging untethered sensing systems we recognize Straser et al. (2001), Lynch et al. (2004), Lynch et al. (2005), Lynch and Koh (2006), Lynch (2006), Kim et al. (2007), Pakzad et al. (2008), Jang et al. (2010), Jo et al. (2010), Meyer et al. (2010), Feltrin et al. (2011), Spencer et al. (2016), Zhang et al. (2016), O'Connor et al. (2017), Dragos and Smarsly (2017), Moreu et al. (2017), Noel et al. (2017).
Finally, we should acknowledge the significant body of research dedicated to structural reliability, lifecycle cost analysis and management of bridges championed by many researchers, such as Yanev (2001), Frangopol and Liu (2007), Thoft-Christensen (2012) and Yuan et al. (2017) amongst many others. Without these major contributions it would not have been possible for the writers to make their contributions to structural identification for health monitoring and asset management of bridges.
9. Conclusions and Recommendations
The discussions offered in this white paper are intended to start a conversation on making infrastructure asset management decisions in general and for bridges and other highway structures in particular, based on objective data on structural performance and condition based on objective indices measured in the field. We cannot eliminate visual bridge inspections that are conducted based on NBIS (National Bridge Inspection Standards) every 2 years, but we may make them more effective by leveraging technology and even extend the inspection interval to 5 years as it is in EU and the Far East for many common bridges.
One possible way to augment current bridge inspection and condition rating practice is by making objective measurements that would describe the “pulses or health-signs” of a bridge during or before an inspection. Sensors for making measurements of strains, displacements, rotations and accelerations at any point of a bridge have been available for decades. These sensors have now been transformed to wireless and practical deployment. It should be possible to train a sufficient number of expert bridge inspectors who are capable of making such measurements. On the other hand we have to caution that sensing and imaging is not as simple as buying and installing a sensor. There are too many examples of failed technology applications that did not lead to any meaningful and actionable information. This has become a major impediment for infrastructure owners and managers buying in to sensing and measurements.
This paper detailed the theoretical background and the training that is essential for educating a new breed of civil engineers who can properly leverage technology. The challenges that have to be overcome for completing a transformation of infrastructure asset management are:
1. Understanding the complex nature of infrastructure systems made up of engineered, human and natural elements; Understanding the definition of infrastructure performance and each of the distinct but interrelated and interdependent limit-states that govern performance; Understanding that infrastructure asset management should be managed by recognizing performance in a holistic manner, with the interdependency between condition and performance at each and every one of the limit-states; Understanding how mechanistic structural engineering and the reliability theory need to be integrated and applied in an engineering design-thinking approach for asset management;
2. Making measurements in the field that can lead to data and interpretation to rationalize bridge asset management decisions require much more than just mounting sensors and collecting data. The training and education of inspectors and engineers need to be considerably more extensive than current NHI (National Highway Institute) courses to lead to the following outcomes:
a. Comprehend the elements and mechanisms through which bridges actually carry their intrinsic and live loads;
b. 3D FEM modeling and analysis of typical bridges;
c. What are the pulses of a bridge (critical locations and strains, displacements, tilts, and accelerations at these locations along with the causes of these responses);
d. What measurements mean in relation to the serviceability, durability and safety performances of the bridge in terms of its live load and intrinsic stresses and the changes in these due to changes in environmental and live load effects.
3. The principles of leveraging FEM analysis and Tacit Knowledge for field instrumentation design, obtaining and managing data, and interpretation toward information and knowledge;
4. Evaluating data quality and reliability, followed by revisiting a-priori FEM for completing and calibrating this into a digital twin;
5. How to leverage a digital twin for identifying critical elements, regions, and mechanisms for load rating, permits, and preservation decisions;
6. How to improve inspections by leveraging operational monitoring results and their history;
In spite of the challenges in the training of a new generation of bridge inspectors and engineers, infrastructure capital needs and increasing backlog in infrastructure renewal are forcing us to bring infrastructure asset management to a rational objective platform.
There is a lot that FHWA can do to facilitate objective data-driven asset management. For example, by leveraging the reference bridges that will serve for the LTBP program research, we may also take advantage of these also to serve as field laboratories for demonstrations and training of the DOT engineers and bridge inspectors. By instrumenting these bridges using wireless sensors, FHWA may greatly simplify taking measurements for LTBP data collection, gaining considerable advantage and cost reduction. FHWA can also support best-practice demonstrations and model standards for technology leveraging that can be adopted by AASHTO as Standards or Recommendations.
Writers fully recognize that it may take many years if not decades until civil engineering education and practice is reformed and civil engineers can take an effective lead role in guiding government, infrastructure owners, regulators and stakeholders in cost-effective and reliable asset management of infrastructures as complex systems. However, we cannot delay the reform if we are interested in livability, sustainability, and resilience of our urban regions.
Author Contributions
AA drafted the entire paper and is the primary contributor to the manuscript. IB has led a Research Project on the development of wireless sensing units, the results of which are presented in the paper and contributed in drafting several Figures. SK was a key individual in the improvement of the wireless sensors. He has been critical in performing the quality control of the paper and in formatting the document following the guidelines of Frontiers.
Conflict of Interest Statement
The authors declare that the research was conducted in the absence of any commercial or financial relationships that could be construed as a potential conflict of interest.
Acknowledgments
Writers are grateful to the National Science Foundation; Dr. Steven Chase (former FHWA Chief Science Officer), Dr. Hamid Ghasemi (Former Program Manager of the LTBP Program) and current FHWA EARP Manager David Kuehn, and Program Managers Dr. Jalinoos, Mr. Faridazar, and Dr. Azari for their support and guidance of their past and ongoing research projects involving technology leveraging for infrastructure asset management. Writers would also like to acknowledge their research partners Dr. Moon, Dr. Sumitro, and Mr. Matsumoto as well as graduate students Shi Ye, Qiang Mao, Mustafa Furkan, and Xiangang Lai who contributed to their ongoing research projects (NSF project 0855023 and FHWA project DTFH61-13-C-00021-Drexel Univ-SF26-1).
Footnotes
1. ^http://www.di3.drexel.edu/w2/files/FHWA_Report_7_18_03.pdf
2. ^A virtual version of such a laboratory is available at www.di3.drexel.edu/view_project.php?p=907
References
Abdel-Ghaffar, A. M., and Housner, G. W. (1977). An Analysis of the Dynamic Characteristics of a Suspension Bridge by Ambient Vibration Measurements, Report EERL 77-01. Earthquake Engineering Research Laboratory, California Institute of Technology.
Abdel-Ghaffar, A. M., and Housner, G. W. (1978). Ambient vibration tests of suspension bridge. J. Eng. Mech. Div. 104, 983–999.
Agbabian, M. S., Masri, S. F., Miller, R. K., and Caughey, T. K. (1991). System identification approach to detection of structural changes. J. Eng. Mech. 117, 370–390.
Aktan, A. E., and Brownjohn, J. M. W. (2013). Structural identification: opportunities and challenges. J. Struct. Eng. 139, 1639–1647. doi: 10.1061/(ASCE)ST.1943-541X.0000723
Aktan, A. E., Catbas, F. N., Grimmelsman, K. A., and Pervizpour, M. (2003). Development of a Model Health Monitoring Guide for Major Bridges. FHWA Research and Development Report.
Aktan, A. E., Moon, F. L., Bartoli, I., and Sjoblom, K. (2016a). Identification of infrastructure systems. J. Infrastruct. Syst. 22:02516002. doi: 10.1061/(ASCE)IS.1943-555X.0000294
Aktan, A. E., Moon, F. L., and Weidner, J. S. (2016b). Leveraging technology for infrastructure condition and performance assessment. Front. Built Environ. 2:36. doi: 10.3389/fbuil.2016.00036
Beck, J. L., and Jennings, P. C. (1980). Structural identification using linear models and earthquake records. Earthq. Eng. Struct. Dyn. 8, 145–160.
Bektas, B. A., and Albughdadi, A. J. (2018). NCHRP Project 20-07, Task 397, Characteristics of Decomissioned Bridges. Ames, IA.
Big Bayou Canot Rail Accident (2018). Available online at: https://en.wikipedia.org/wiki/Big_Bayou_Canot_rail_accident (accessed August 01, 2018).
Brown, D. L., and Allemang, R. J. (2007). “The modern era of experimental modal analysis,” in Sound and Vibration/January 2007, 40th Anniversary Issue, 16–25.
Brownjohn, J. M. (2006). Structural health monitoring of civil infrastructure. Philos. Trans. R. Soc. A Math. Phys. Eng. Sci. 365, 589–622. doi: 10.1098/rsta.2006.1925
Brownjohn, J. M. W., Magalhaes, F., Caetano, E., and Cunha, A. (2010). Ambient vibration re-testing and operational modal analysis of the humber bridge. Eng. Struct. 32, 2003–2018. doi: 10.1016/j.engstruct.2010.02.034
Catbas, F. N., Kijewski-Correa, T., and Aktan, A. E. (2013). Structural Identification of Constructed Systems. Reston, VA: ASCE.
Chang, P. C., Flatau, A., and Liu, S. (2003). Review paper: health monitoring of civil infrastructure. Struct. Health Monit. 2, 257–267. doi: 10.1177/1475921703036169
Conte, J. P., He, X., Moaveni, B., Masri, S. F., Caffrey, J. P., Wahbeh, M., et al. (2008). Dynamic testing of alfred zampa memorial bridge. J. Struct. Eng. 134, 1006–1015. doi: 10.1061/(ASCE)0733-9445(2008)134:6(1006)
Dragos, K., and Smarsly, K. (2017). “Decentralized infrastructure health monitoring using embedded computing in wireless sensor networks,” in Dynamic Response of Infrastructure to Environmentally Induced Loads, eds A. Sextos and G. Manolis (Milan: Springer), 183–201.
Equity (2018). Available online at: https://www.theenginuity.com/blog/the-business-edge-of-converting-information-into-wisdom/ (accessed August 01, 2018).
Farrar, C. R. (2001). Historical Overview of Structural Health Monitoring, Lecture Notes on Structural Health Monitoring Using Statistical Pattern Recognition, (Bethesta, MD: US National Library of Medicine, NIH).
Farrar, C. R., and Worden, K. (2006). An introduction to structural health monitoring. Philos. Trans. R. Soc. Lond. A Math. Phys. Eng. Sci. 365, 303–315. doi: 10.1098/rsta.2006.1928
Feltrin, G., Soukh, O., Bischoff, R., Meyer, J., and Motavalli, M. (2011). “Structural monitoring with wireless sensor networks: Experiences from field deployments,” in Proceedings of First Middle East Conference on Smart Monitoring, Assessment and Rehabilitation of Civil Structures (Dubai).
Frangopol, D. M., and Liu, M. (2007). Maintenance and management of civil infrastructure based on condition, safety, optimization, and life-cycle cost. Struct. Infrastruct. Eng. 3, 29–41. doi: 10.1080/15732470500253164
Fueller, T., Singhvi, A., and Williams, J. (2018). San Francisco's Big Seismic Gamble. Available online at: https://www.nytimes.com/interactive/2018/04/17/us/san-francisco-earthquake-seismic-gamble.html (accessed April 17, 2018).
Galambos, T. V., and Mayes, R. L. (1978). Dynamic Tests of a Reinforced Concrete Building. St. Louis, MO: Washington University, School of Engineering and Applied Science, Dept. of Civil Engineering.
Glisic, B., Inaudi, D., Lau, J. M., and Fong, C. C. (2013). Ten-year monitoring of high-rise building columns using long-gauge fiber optic sensors. Smart Mater. Struct. 22:055030. doi: 10.1088/0964-1726/22/5/055030
Goulet, J. A., Kripakaran, P., and Smith, I. F. (2010). Multimodel structural performance monitoring. J. Struct. Eng. 136, 1309–1318. doi: 10.1061/(ASCE)ST.1943-541X.0000232
Grimmelsman, K. A., Pan, Q., and Aktan, A. E. (2007). Analysis of data quality for ambient vibration testing of the Henry Hudson bridge. J. Intell. Mater. Syst. Struct. 18, 765–775. doi: 10.1177/1045389X06074774
Hart, G. C., and Yao, J. T. P. (1977). System identification in structural dynamics. J. Eng. Mech. Div. 103, 1089–1104.
Hearn, G. (2007). NCHRP Synthesis 375, Bridge Inspection Practices, A Synthesis of Highway Practice. Washington, DC: The National Academies Press.
Hiasa, S., Catbas, F. N., Matsumoto, M., and Mitani, K. (2016). Monitoring concrete bridge decks using infrared thermography with high speed vehicles. Struct. Monit. Maint. 3, 277–296. doi: 10.12989/smm.2016.3.3.277
Hudson, D. E. (1964). Resonance testing of full scale structures. J. Engrg. Mech. Div. ASCE 90, 1–19.
Hunt, V. J., Turer, A., Gao, Y., Levi, A., Helmicki, A. J., Barrish, R. A., et al. (1998). “Instrumented monitoring and nondestructive evaluation of highway bridges,” in Proceedings of Structural Materials Technology III - An NDT Conference (San Antonio, TX: International Society for Optics and Photonics), 472–481.
Ibáez, P. (1973). Identification of dynamic parameters of linear and non-linear structural models from experimental data. Nuclear Eng. Des. 25, 30–41.
Jang, S., Jo, H., Cho, S., Mechitov, K., Rice, J. A., Sim, S. H., et al. (2010). Structural health monitoring of a cable-stayed bridge using smart sensor technology: deployment and evaluation. Smart Struct. Syst. 6, 439–459. doi: 10.12989/sss.2010.6.5_6.461
Jo, H., Rice, J. A., Spencer, B. F., and Nagayama, T. (2010). “Development of high-sensitivity accelerometer board for structural health monitoring,” in Sensors and Smart Structures Technologies for Civil, Mechanical, Aerospace Systems, (Bellington, WA), 764706.
Kareem, A., Kijewski, T., and Tamura, Y. (1999). Mitigation of motions of tall buildings with specific examples of recent applications. Wind Struct. 2, 201–251.
Kim, S., Pakzad, S., Culler, D., Demmel, J., Fenves, G., Glaser, S., et al. (2007). “Health monitoring of civil infrastructures using wireless sensor networks,” in Proceedings of the 6th International Conference on Information Processing in Sensor Networks (Cambridge, MA), 254–263.
Knowledge-Management-Tools (2017). Available online at: https://www.knowledge-management-tools.net/ (accessed August 01, 2018).
Ko, J. M., Sun, Z. G., and Ni, Y. Q. (2002). Multi-stage identification scheme for detecting damage in cable-stayed kap shui mun bridge. Eng. Struct. 24, 857–868. doi: 10.1016/S0141-0296(02)00024-X
Law, K. H., Smarsly, K., and Wang, Y. (2014). Sensor data management technologies for infrastructure asset management. Sensor Technol. Civil Infrastruct. 2, 3–32. doi: 10.1533/9781782422433.1.3
Leung, C. K., Wang, K. T., Inaudi, D., Bao, X., Habel, W., Zhou, Z., et al. (2015). Optical fiber sensors for civil engineering applications. Mater. Struct. 48, 871–906. doi: 10.1617/s11527-013-0201-7
Lynch, J. P. (2006). An overview of wireless structural health monitoring for civil structures. Philos. Trans. R. Soc. Lond. A Math. Phys. Eng. Sci. 365, 345–372. doi: 10.1098/rsta.2006.1932
Lynch, J. P., and Koh, K. J. (2006). A summary review of wireless sensors and sensor networks for structural health monitoring. Shock Vib. Digest. 38, 91–130. doi: 10.1177/0583102406061499
Lynch, J. P., Law, K. H., Kiremidjian, A. S., Carryer, E., Farrar, C. R., Sohn, H., et al. (2004). Design and performance validation of a wireless sensing unit for structural monitoring applications. Struct. Eng. Mech. 17, 393–408. doi: 10.12989/sem.2004.17.3_4.393
Lynch, J. P., Wang, Y., Law, K. H., Yi, J. H., Lee, C. G., and Yun, C. B. (2005). “Validation of a large-scale wireless structural monitoring system on the geumdang bridge,” in Proceedings of 9th International Conference on Structural Safety and Reliability (Rome), 19–23.
Meyer, J., Bischoff, R., Feltrin, G., and Motavalli, M. (2010). Wireless sensor networks for long-term structural health monitoring. Smart Struct. Syst. 6, 263–275. doi: 10.12989/sss.2010.6.3.263
Moore, M., Phares, B. M., Graybeal, B., Rolander, D., and Washer, G. (2001). Reliability of Visual Inspection for Highway Bridges, Volume I (McLean, VA: FHWA), FHWA-RD-01-020.
Moreu, F., Kim, R., and Spencer, B. (2017). Railroad bridge monitoring using wireless smart sensors. Struct. Control Health Monit. 24:e1863. doi: 10.1002/stc.1863
National Research Council (1995). Expanding the Vision of Sensor Materials. Washington, DC: National Academies Press.
Noel, A. B., Abdaoui, A., Elfouly, T., Ahmed, M. H., Badawy, A., and Shehata, M. S. (2017). Structural health monitoring using wireless sensor networks: A comprehensive survey. IEEE Commun. Surveys Tutor. 19, 1403–1423. doi: 10.1109/COMST.2017.2691551
O'Connor, S. M., Zhang, Y., Lynch, J. P., Ettouney, M. M., and Jansson, P. O. (2017). Long-term performance assessment of the telegraph road bridge using a permanent wireless monitoring system and automated statistical process control analytics. Struct. Infrastruct. Eng. 13, 604–624. doi: 10.1080/15732479.2016.1171883
Pakzad, S. N., and Fenves, G. L. (2009). Statistical analysis of vibration modes of a suspension bridge using spatially dense wireless sensor network. J. Struct. Eng. 135, 863–872. doi: 10.1061/(ASCE)ST.1943-541X.0000033
Pakzad, S. N., Fenves, G. L., Kim, S., and Culler, D. E. (2008). Design and implementation of scalable wireless sensor network for structural monitoring. J. Infrastruct. Syst. 14, 89–101. doi: 10.1061/(ASCE)1076-0342(2008)14:1(89)
Peeters, B., and Roeck, G. D. (2001). One-year monitoring of the z24-bridge: environmental effects versus damage events. Earthq. Eng. Struct. Dyn. 30, 149–171. doi: 10.1002/1096-9845(200102)30:2<149::AID-EQE1>3.0.CO;2-Z
Posenato, D., Lanata, F., Inaudi, D., and Smith, I. F. (2008). Model-free data interpretation for continuous monitoring of complex structures. Adv. Eng. Inform. 22, 135–144. doi: 10.1016/j.aei.2007.02.002
Rafael, B., and Smith, I. F. (2003). A direct stochastic algorithm for global search. Appl. Math. Comput. 146, 729–758. doi: 10.1016/S0096-3003(02)00629-X
Schickert, G. (1997). “Ndt-ce symposium berlin 1995: a concluding review,” in International Symposium Non-Destructive Testing in Civil Engineering (NDT-CE) (Berlin).
sens2b-sensors (2018). Available online at: http://www.sens2b-sensors.com/directory/mechanical-dynamical/accelerometers-acceleration-sensors (accessed August 01, 2018).
sensorwiki (2018). Available online at: http://www.sensorwiki.org/doku.php/sensors/introduction (accessed August 01, 2018).
Shaw, T. (2003). NCHRP Synthesis 311, Performance Measures of Operational Effectiveness for Highway Segments and Systems. Hoboken, NJ: Transportation Research Board.
Shinozuka, M., and Ghanem, R. (1995). Structural system identification. II: Experimental verification. J. Eng. Mech. 121, 265–273.
Shinozuka, M., Yun, C. B., and Imai, H. (1982). Identification of linear structural dynamic systems. J. Eng. Mech. Div. 108, 1371–1390.
Sigurdardottir, D., and Glisic, B. (2013). Neutral axis as damage sensitive feature. Smart Mater. Struct. 22:075030. doi: 10.1088/0964-1726/22/7/075030
Siringoringo, D. M., and Fujino, Y. (2008). System identification of suspension bridge from ambient vibration response. Eng. Struct. 30, 462–477. doi: 10.1016/j.engstruct.2007.03.004
Smith, I. F. (2016). Studies of sensor data interpretation for asset management of the built environment. Front. Built Environ. 2:8. doi: 10.3389/fbuil.2016.00008
Spencer, B. F., Jo, H., Mechitov, K. A., Li, J., Sim, S. H., Kim, R. E., et al. (2016). Recent advances in wireless smart sensors for multi-scale monitoring and control of civil infrastructure. J. Civil Struct. Health Monit. 6, 17–41. doi: 10.1007/s13349-015-0111-1
Stoll, J. D. (2018). Facebook's Hard Fall Shows the Pitfalls of Big Data. Available online at: https://www.wsj.com/articles/facebooks-hard-fall-shows-the-pitfalls-of-big-data-1532750496?mod=hp_lead_pos3 (accessed July 18, 2018).
Straser, E. G., Kiremidjian, A. S., and Meng, T. H. (2001). Modular, Wireless Damage Monitoring System for Structures. Patent No: 6,292,108, U.S. Patent.
Thoft-Christensen, P. (2012). Infrastructures and life-cycle cost-benefit analysis. Struct. Infrastruct. Eng. 8, 507–516. doi: 10.1080/15732479.2010.539070
Virtual Non-destructive Evaluation Library for Highway Structures (2018). Available online at: www.di3.drexel.edu/view_project.php?p=907 (accessed August 01, 2018).
Yao, J. T. P., and Natke, E. L. G. (1994). Damage detection and reliability evaluation of existing structures. Struct. Saf. 15, 3–16.
Yuan, C., McClure, T., Cai, H., and Dunston, P. S. (2017). Life-cycle approach to collecting, managing, and sharing transportation infrastructure asset data. J. Constr. Eng. Manag. 143:04017001. doi: 10.1061/(ASCE)CO.1943-7862.0001288
Yun, C. B., and Shinozuka, M. (1980). Identification of nonlinear structural dynamic systems. J. Struct. Mech. 8, 187–203.
Zhang, Y., Kurata, M., and Lynch, J. P. (2016). Long-term modal analysis of wireless structural monitoring data from a suspension bridge under varying environmental and operational conditions: System design and automated modal analysis. J. Eng. Mech. 143:04016124. doi: 10.1061/(ASCE)EM.1943-7889.0001198
Keywords: bridge asset management, bridge inspection, sensing technologies, sensor selection, high resolution imaging, wireless sensing
Citation: Aktan AE, Bartoli I and Karaman SG (2019) Technology Leveraging for Infrastructure Asset Management: Challenges and Opportunities. Front. Built Environ. 5:61. doi: 10.3389/fbuil.2019.00061
Received: 16 November 2018; Accepted: 29 April 2019;
Published: 16 May 2019.
Edited by:
Eleni N. Chatzi, ETH Zürich, SwitzerlandReviewed by:
Branko Glisic, Princeton University, United StatesKe Chen, The University of Hong Kong, Hong Kong
Fernando Moreu, University of New Mexico, United States
Copyright © 2019 Aktan, Bartoli and Karaman. This is an open-access article distributed under the terms of the Creative Commons Attribution License (CC BY). The use, distribution or reproduction in other forums is permitted, provided the original author(s) and the copyright owner(s) are credited and that the original publication in this journal is cited, in accordance with accepted academic practice. No use, distribution or reproduction is permitted which does not comply with these terms.
*Correspondence: S. Gokhan Karaman, ks3285@drexel.edu