- 1Centre for Advanced Spatial Analysis (CASA), University College London, London, United Kingdom
- 2Geography and Environment, The University of Southampton, Southampton, United Kingdom
- 3UWA School of Agriculture and Environment, The University of Western Australia, Crawley, WA, Australia
- 4Department of Geography and Planning, The University of Western Australia, Crawley, WA, Australia
Urban areas are expected to triple by 2030 in order to accommodate 60% of the global population. Anthropogenic landscape modifications expand coverage of impervious surfaces inducing the urban heat island (UHI) effect, a critical twenty first century challenge associated with increased economic expenditure, energy consumption, and adverse health impacts. Yet, omission of UHI measures from global climate models and metropolitan planning methodologies precludes effective sustainable development governance. We present an approach that integrates Earth observation and climate data with three-dimensional urban models to determine optimal tree placement (per square meter) within proposed urban developments to enable more effective localized UHI mitigation. Such data-driven planning decisions will enhance the future sustainability of our cities to align with current global urban development agendas.
Introduction
The United Nations (UN) New Urban Agenda aims to readdress the way in which cities are planned, designed, financed, developed, governed, and managed to sustainably accommodate 60% of the global population by 2030. As one of the twenty first century's most transformative trends, urbanization will result in a tripling of the urban land cover footprint within this time frame (2000 baseline), alongside a need to accommodate ~1.024 billion people within these urban areas (Seto et al., 2012). Anthropogenic modifications required to convert natural land into urban surfaces are inevitable as the urbanization trend continues. This most extreme form of land cover change often initiates the urban heat island (UHI) effect, where temperatures increase over human-made surfaces. Such changes have substantive implications through resultant heat-induced adverse socio-economic and environmental impacts (Hu et al., 2015). For example, in the city of Melbourne, the economic implications of the UHI exceed AUD 300 million annually, and in Hong Kong, the social repercussions of a 1°C rise in temperature above 29°C have increased mortality rates by 4.1% (Goggins et al., 2012). In terms of the environment, an increase of 0.56°C in daily minimum city temperatures for the city of Phoenix has led to continued unsustainable water usage, with monthly water consumption now exceeding 1,000 L per family household (Guhathakurta and Gober, 2007).
Sustainable urban management is of vital contemporary importance to cities for current and future planning. Under projections of a changing climate, the UHI may increase Gross Domestic Product (GDP) losses up to 0.80% by 2050 (Estrada et al., 2017). Given these potentially disruptive economic consequences, the implications of warming cities have been noted in recent international polices, including the Sendai Framework for Disaster Risk Reduction, the Sustainable Development Goals (SDG), and City Resilience Framework (CRF) (Table 1). In response to these global agendas, cities have begun implementing various UHI impact reduction initiatives (see Table 1), activities for which can be categorized under voluntary or policy themes. The voluntary theme includes demonstrative projects, education, and incentives, such as Chicago's exemplar “green” city hall roof and Baltimore County's “growing home” campaign offering vouchers for purchasing trees. The policy theme incorporates the UHI into metropolitan spatial development strategies, like in the case of The London Plan, Perth and Peel @3.5 million and Johannesburg's Spatial Development Framework 2040.
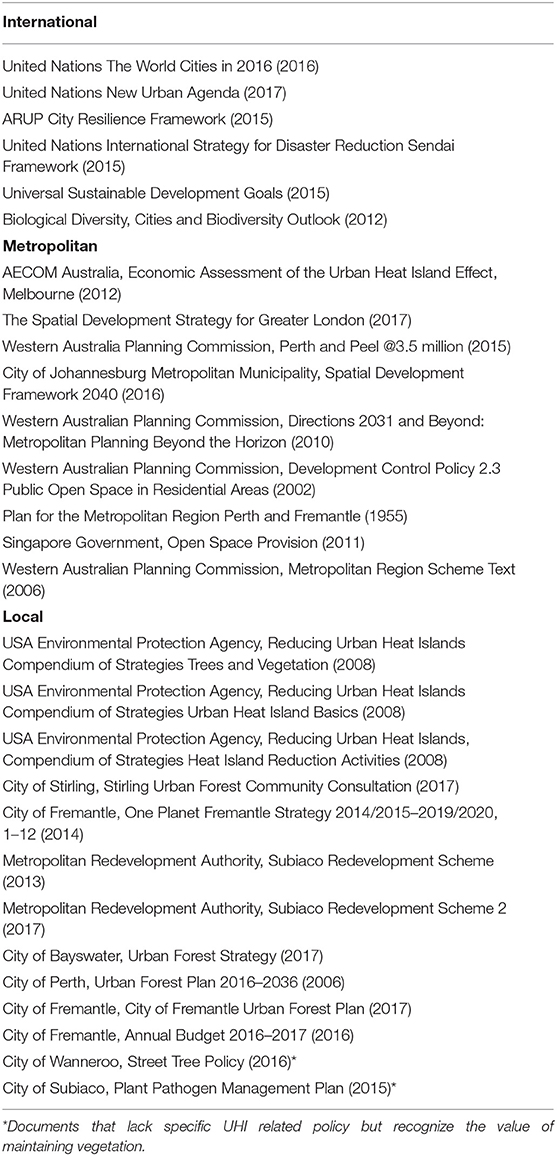
Table 1. Relevant influential international, metropolitan, and local UHI and urban expansion policies, strategies, and assessments (with publication date) referred to in this paper.
Although these policies are a step in the right direction, they generally fail in planning practicality through lacking specificities for combating adverse temperature effects at the local level (sub-city). Additionally, they provide no consistency in planning implementation methodologies or steps for assessing progress toward UHI reduction targets. Some notable exceptions do exist where UHI policy adaptations instigated by local governing authorities have successfully permitted quantifiable localized planning requirements (e.g., zoning and building codes), such as Seattle's “Green Factor,” Baton Rouge's landscape ordinance, and the City of Stirling's Amendment 9 specifying minimum vegetation requirements for developments over a certain size. However, even these urban planning designs lack empirical evidence for optimizing UHI mitigation strategies. The paucity of quantifiable measures has contributed to the global state of data-informed governance being described as underdeveloped, disparate, and marginalized (McPherson, 2016; Acuto, 2018).
With such limitations noted, we propose a data-driven approach to develop robust sub-city UHI measures that integrate remote sensing information. Earth Observation (EO) data—gathered using satellite and airborne imagery—can be processed to identify (un)sustainable urban development through aerial assessments of land cover change, alongside various environmental variables (e.g., temperature, elevation). EO data for measuring and monitoring urbanization provide the localized spatiotemporal scales necessary to systematically target planning policies for UHI mitigation within global cities. Specifically, EO data can produce consistent information necessary for restricting unsustainable development (MacLachlan et al., 2017a) and monitoring UHI effects based on associated land-temperature dynamics (MacLachlan et al., 2017b). To date, EO applications for UHI mitigation have been restricted to measurements from limited temporal temperature data and medium-scale spatial resolutions (e.g., Fremantle's Urban Forest summer temperature data at 30 m2). Furthermore, these measures have been aggregated to city blocks, or more generally, city-wide areas. Assessment at finer spatial scales (e.g., block subdivisions) is necessary for determining temperature dynamics across new developments and explicit targeting of localized heat remediation efforts, such as tree planting.
Consequently, in this paper, we build upon previous analyses (MacLachlan et al., 2017a,b) to outline an operational evidence-based urban planning methodology for localized UHI mitigation through the integration of EO and climate data into the planning decision-making process. Our findings demonstrate that the integrated use of remote sensing information with open-source spatial modeling can be used to (i) effectively derive temperature characteristics of different development types, and (ii) assist in the planning process through determining optimal tree placement for an urban (re)development. Our research is exemplified through applying the approach to urban development in areas of the Perth Metropolitan Region (PMR) and the City of Fremantle, the latter of which hosts a council aiming to become Western Australia's most sustainable local government within the state capital of Perth.
Study Area
The PMR has observed sustained low density outward urban expansion since the beginning of the twenty first century in response to a rapidly expanding resource sector (Kennewell and Shaw, 2008; MacLachlan et al., 2017a,d). Urban area within the PMR increased 45.3% (320.33 km2) between 1990 and 2015, with a 37.44% increase since 2000 (279.80 km2) (MacLachlan et al., 2017a,c). Between 2007 and 2014, Perth was consistently considered Australia's fastest-growing city, while only obtaining a maximum population density (2011) of 3,662 people per km2, 33.82 and 24.83% lower than Melbourne (10,827) and Sydney (14,747), respectively (ABS, 2011, 2015; MacLachlan et al., 2017a). Low population density has generated demand for dispersed housing in a non-strategic, “lot-by-lot fashion” founded upon a car-dependent lifestyle (Dhakal, 2014). Due to the large scale conversion from natural land to impervious surfaces, strategic land zonation and sustainability targets have been specified within the Western Australian Planning Commissions (WAPC) long-term (2050) development guide. UHI mitigation is listed as 1 of 16 aspirations under the city sustainability objective. In response, some local governments have devised policies, such as the City of Fremantle's and City of Perth's Urban Forest Plans, that outline greening strategies for UHI reduction targets (Table 1). Other councils recognize the value of maintaining vegetation, for example, Wanneroo's “street tree” policy and Subiaco's “plant management” plan (Table 1), but lack any specific UHI targets. Overall, all these policies are subject to the spatial and temporal limitations discussed in the previous section, and as such have potential to implement mis-informed, inconsistent, and sub-optimal UHI mitigation measures.
Materials and Methodology
A sub-city urban planning modeling approach was developed to provide a consistent evidence-based methodology for measuring and monitoring localized UHI mitigation targets. Within the methodology, temperature dynamics of low- and high-density census areas within the PMR were compared using EO data to assess the heat implications of different development types (i.e., changes in land cover composition). This part of the method can be used to compare development types and allow urban planners to select an optimal land conversation scenario that minimizes the local UHI effect. Following this, the methodology provides steps for mitigating excess temperatures within a planned area for (re)development by determining optimal placement of greening elements of the proposed plans using a data-driven model. This was demonstrated through running tree planting scenarios for a proposed redevelopment in the city of Fremantle.
Materials
The implemented methodology makes use of the open-source Urban Multi-scale Environmental Predictor (UMEP), a holistic analytical tool for urban climatology and climate-sensitive planning applications, and the first model to generate spatial temperature variations available as a plugin to the open-source Quantum Geographical Information Systems (QGIS) (Lindberg et al., 2018). To compute temperature estimates, the SOLWEIG (Solar and LongWave Environmental Irradiance Geometry) model, part of the UMEP set of modeling tools, was used. SOLWEIG creates spatial simulations of temperature through modeling shortwave and longwave radiation fluxes in six directions following the procedure developed by Höppe (1992) with full details provided in Lindberg et al. (2008). SOLWEIG required inputs of (1) elevation models, (2) land cover data, and (3) meteorological data (Figure 1A). In demonstrating a data driven-approach to mitigate excess temperatures, elevation models and land cover were manually altered to reflect proposed redevelopment plans using open access planning blueprints from the City of Fremantle. While our approach has made use of available high-resolution EO data, substitution with alternative data sources would be appropriate in locations where such imagery is unattainable; this is further explored within the discussion. The following sections describe data sources implemented here for the temperature modeling.
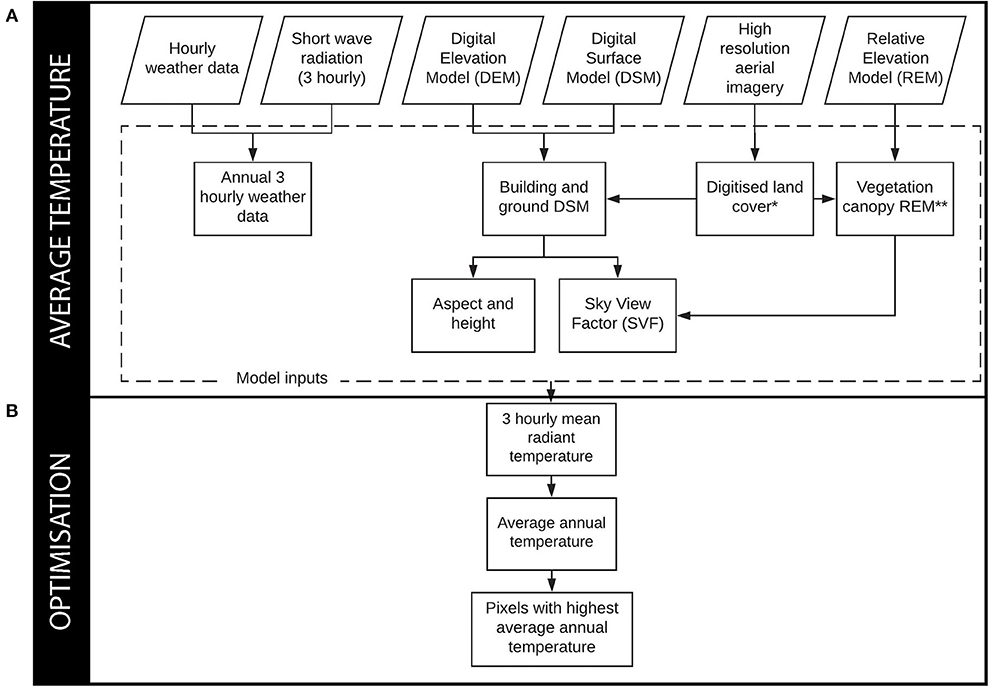
Figure 1. Summary of methodological procedures for (A) mean radiant temperature (Tmrt ) and (B) identifying optimal tree locations. *indicates existing development modified using proposed redevelopment plans. **indicates data were modified on three occasions: (1) using proposed redevelopment plans, (2) removing all trees across the proposed redevelopment site, and (3) using optimal tree locations.
High-Resolution Aerial Imagery
High-resolution aerial and elevation data captured by the March 2009 Australian Commonwealth Scientific and Industrial Research Organization (CSIRO) Perth and Peel Urban Monitoring Programme were used within this analysis. Land cover was established from the aerial data (described within the methodology). The elevation data were pre-processed to produce four elevation models: a Digital Surface Model (DSM), a Digital Terrain model (DTM), and two Relative Elevation Models (REM). The DSM represents a model of the protrusions from Earth's surface, the DTM is a model of Earth's bare surface, and the REM represents the difference between the surface (DSM) and the bare surface (DTM) elevation models providing relative heights above ground level. A vegetation canopy REM layer was also provided that established trees as vegetation >2 m, and grass as vegetation below 2 m. Due to vegetation senescence, some minor manual modifications (e.g., addition of trees to the layer) were subsequently made to the vegetation canopy REM. From this processing of the high-resolution data, the input datasets for the SOLWEIG model were acquired: land cover, a building DSM, a ground DTM, and a vegetation canopy REM. The methodology provides more details on extraction of the preprocessed data into the model format datasets where appropriate. Information on the acquisition of the high-resolution imagery can be found within Caccetta et al. (2012).
Meteorological Data
Meteorological data required for temperature modeling using SOLWEIG included air temperature, relative humidity, barometric pressure, wind speed, wind direction, and downward shortwave radiation. Owing to these data frequently being unavailable in a spatially continuous form (e.g., raster data), the input required for SOLWEIG is often limited to point data, acquired from weather stations as suggested by Lindberg et al. (2018). The Australian Bureau of Meteorology (BOM) provide continuous hourly meteorological measurements that can be freely accessed through the online Weather Underground portal. Here, data were obtained from the Perth International Airport weather station (station YPPH), the most complete temporal record within the Perth Metropolitan Region (PMR). While wind speed and direction are classed as “not needed” by the temperature model, lack of wind has been identified as a contributing factor to increased UHI intensity (Gaffin et al., 2008; Lindberg et al., 2017). Wind is particularly pertinent to Perth during the summer months due to major land and sea temperature differences that induce an onshore wind of up to 40 km h−1 termed the “Fremantle Doctor,” cooling the metropolitan region (Eliot et al., 2006).
Downward shortwave radiation is not commonly captured by meteorological stations. However, the European Water and Global Change (WATCH) initiative has generated free global continuous 3-hourly estimates within the dataset “WATCH Forcing Data methodology applied to ERA-interim” (WFDEI) being generated from extensive satellite, atmospheric, and surface observations. In order to produce a coherent complete (3-hourly) meteorological dataset, the hourly meteorological variables were temporally aggregated to the 3-hourly shortwave radiation data. All meteorological data were obtained for the years 2008–2010, with modeled temperature data averaged over this period to reduce inter-annual variation (Imhoff et al., 2010; Zhang et al., 2012; Hu et al., 2015; MacLachlan et al., 2017b). Full details of WFDEI data can be found within Weedon et al. (2014).
Open Street Map Data
Infrastructure data were necessary for the first part of the methodology to assess temperature dynamics within the two PMR areal census tracts. Open street map road data, with minor manual digitization editing to reflect past 2009 road layouts identified through use of high-resolution aerial imagery, guided the three-dimensional modeling of the low-density (Currambine) and high-density (Subiaco) Australian Bureau of Statistics (ABS)-defined Level 1 Statistical Areas (SA1), as presented in Figure 2.
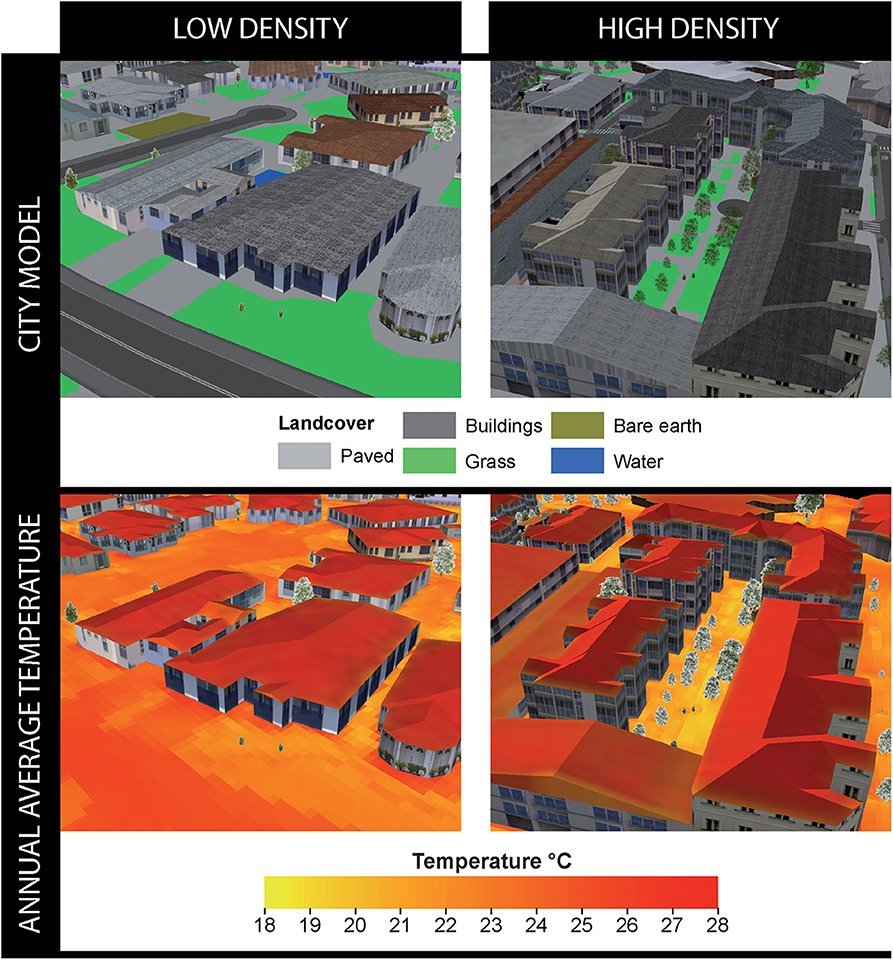
Figure 2. Three-dimensional city models (top row) and average annual mean radiant temperature (Tmrt ) overlays (bottom row) for subsets of two SA1 locations representing low-density (cul-de-sac in Currambine) and high-density (infill site in Subiaco) developments. Two-dimensional renditions for complete SA1 coverage are presented in Figure 3.
Proposed Planning Application Data
The SOLWEIG modeling required detailed planning documentation for information on proposed land cover change during a planned redevelopment. Blueprints from City of Fremantle's planning portal for the Woolstore Shopping Center and Carpark redevelopment proposal (28 Cantonment Street, Fremantle) enabled manual modification of elevation models and land cover in determining proposed land cover change. This also identified the proposed green infrastructure for the planned redevelopment, which was used to inform subsequent tree placement within the modeling framework.
Methodology
The following subsections describe the temperature modeling approach implemented, and the modification of existing data (e.g., elevation models and land cover) to reflect the proposed redevelopment to allow for optimum tree placement to be determined using the SOLWEIG model outputs.
Temperature Modeling
Temperature was modeled per square meter every 3 h between 2008 and 2010 using the SOLWEIG model. The model has already undergone validation through comparison to field measurements in global cities including Shanghai (Chen et al., 2016), Hong Kong (Lau et al., 2016), and Gothenburg (Lindberg and Grimmond, 2011). All studies produced strong correlations (R2 > 0.90), with the latter city validation explicitly noted as statistically significant. This demonstrates the model's ability to accurately reflect city temperature dynamics and warrants model suitability for application in this paper to the PMR. The model was used to (i) compare temperature dynamics within low- and high-density urban areas, and (ii) use as a basis for assessing a planned redevelopment and how optimizing tree placement relative to modeled temperature values could assist in mitigating localized UHI impacts.
Inputs required for the model were generated from previously described data, and these inputs were meteorological data, land cover, a building DSM, a ground DTM, and a vegetation canopy REM. All inputs were in a raster (grid-based) format and generated within a geographic information system (e.g., QGIS). The complete meteorological data and vegetation canopy REM described within the materials section needed no further processing for inclusion within the model. Land cover was determined from manual digitization of the high resolution aerial imagery into the classes of paved, building, grass, bare soil, and water, with conversion to raster format. In computing the building DSM and ground DTM, buildings established within the land cover dataset were used to extract height values from the DSM, with all other values assigned to the DTM.
In order to appropriately model temperature, the Sky View Factor (SVF) was required. The SVF is defined by the ratio of radiation received (or emitted) by a planar surface to the radiation emitted (or received) by the entire hemispheric environment, with both radiation components being essential components to human comfort, solar access, and solar energy (Holst and Mayer, 2011; Lau et al., 2015). This variable was computed from the vegetation canopy REM, the building DSM, and the ground DTM, processed with tools provided within the UMEP plugin. Additionally, building wall heights and aspect were requisites of the model, generated from the building DSM and ground DTM using the UMEP plugin. Full details on the algorithms used for SVF alongside building height/aspect computation can be found in Lindberg and Grimmond (2010) and Lindberg et al. (2015), respectively.
Data-Driven Tree Placement
Following the generation of modeled temperature outputs to compare low- and high-density urban areas, a site was selected for modeling temperature to investigate the impact of a proposed redevelopment within the City of Fremantle. For this site, three scenarios were processed following the temperature modeling methodology, as specified in the previous section, to assess temperatures for (1) the current urban footprint, (2) proposed changes to the urban footprint based on the redevelopment plans (blueprints), and (3) the proposed redevelopment with no trees, with results used to redesign tree placement and then re-run the model. The proposed redevelopment with all trees removed model run permitted identification of the highest average annual temperatures per square meter. Based on these temperature predictions, the locations (square meter blocks) with the highest temperatures were used to replace the planned tree locations for the redevelopment complex in order to maximize the temperature reduction potential across the redevelopment (Figure 1B). Fifteen trees, as specified in the original redevelopment plans, were distributed while preserving original design aspects found within the blueprints including crown radius (2.82 m), spacing (>7 m), and height (with street level trees assigned a height of 16.41 m and trees on buildings a height of 3.54 m). The new tree locations were reflected in an updated vegetation canopy REM, and the analysis was re-run to compare temperature across the redevelopment site, relative to the original urban footprint and the proposed redevelopment plan (Figure 1). The proposed site and surrounding city blocks were modeled in all scenarios accounting for the influence of neighboring landscape features (e.g., shadowing).
Results and Discussion
The influence of localized urban development types on temperature dynamics was established through comparison of two census areas within the city of Perth. The two ABS SA1s selected for the comparative analysis represent areas that have undergone (i) low-density urban fringe green field development (Currambine) and (ii) high-density Brownfield infill development (Subiaco). Commencing with similar population density (per 0.1 km2) values in 2011 (Subiaco: 325, Currambine: 310), by 2015, Subiaco more than doubled in density compared to Currambine (Subiaco: 712, Currambine: 324) (Table 2).
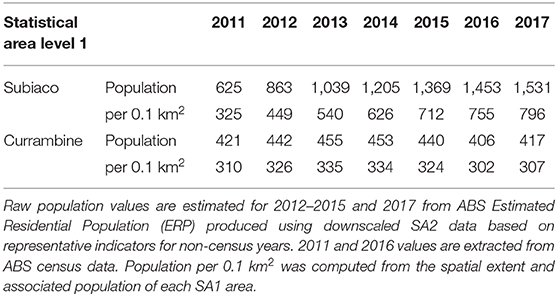
Table 2. Total population and population density per 0.1 km2 between 2011 and 2016 for Subiaco and Currambine SA1s as defined by the ABS.
The urban design characteristics commonly associated with low-density development, such as lower building heights, less dense vegetation, and more sprawled layout, produced higher average temperatures of 1.00°C (paved), 0.90°C (grass), and 0.60°C (buildings) compared to that of the high-density infill site, as illustrated in the spatial extent of the three-dimensional view area (Figure 2). The complete SA1 of Currambine experienced higher temperatures of 0.54°C (paved), 0.39°C (grass), and 0.14°C (buildings) compared to that of Subiaco (Figure 3). The Western Australia Planning Commission (WAPC) recognize sustainability concerns within the PMR due to the majority of new development occurring as low-density, outward suburban sprawl encroaching into the wildland–urban interface. To combat such apprehensions, the WAPC have detailed infill development strategies alongside UHI reduction aspirations for the long-term (2050) development of the city. While local governments, such as the City of Bayswater and Stirling, have observed population increase with infill development, the tendency toward larger houses on subdivided blocks has significantly declined the level of tree canopy coverage. Consequently, low-density development and associated canopy reduction seem to be adversely impacting the localized UHI effect (Table 1).
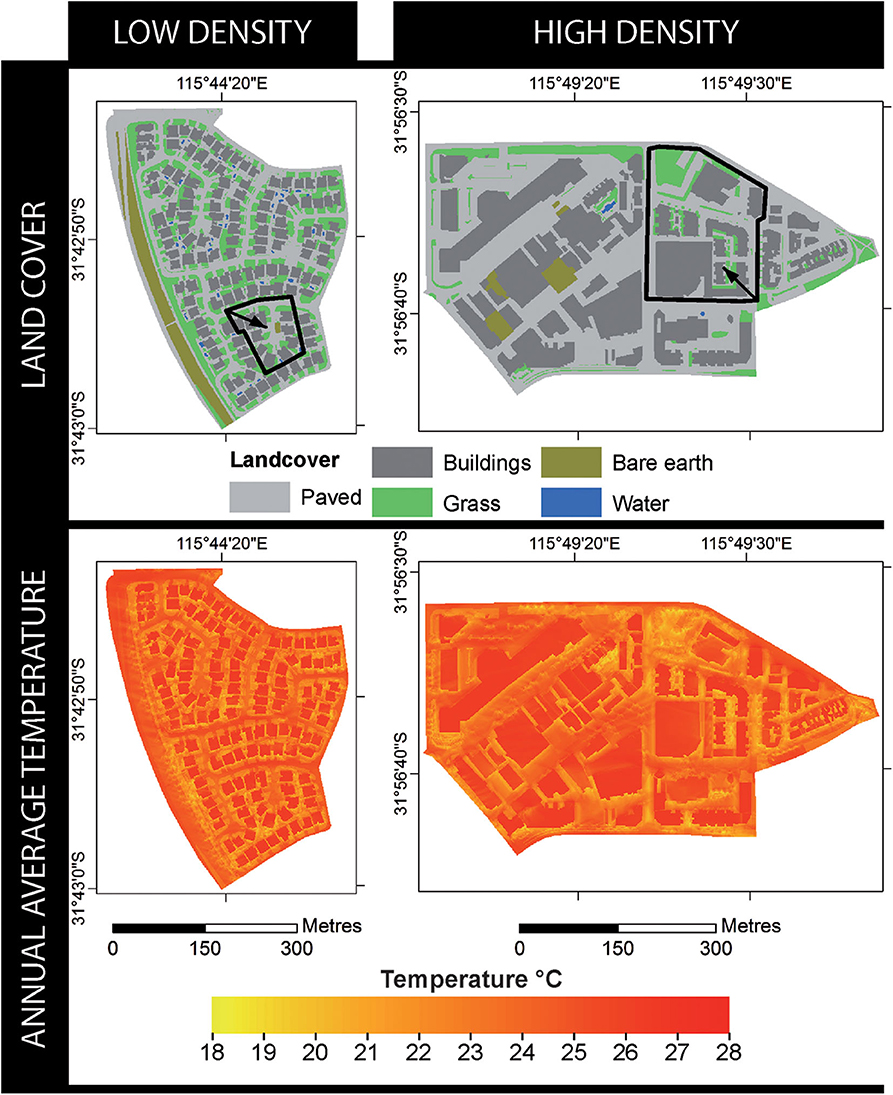
Figure 3. Land cover (top row) and average annual mean radiant temperature (Tmrt ) overlays (bottom row) for two SA1s as defined by the ABS representing low (Currambine, SA Level 1 ID: 5107102) and high (Subiaco, SA Level 1 ID: 5104203) density developments. Bold black outline extents with directional arrows denote three-dimensional subsets presented in Figure 2.
The proposed Woolstores shopping center redevelopment in the City of Fremantle will alter land use from retail to a mixed-use development comprising of accommodation and commercial property. Correspondingly, land cover will change from high to low urban reflectance (albedo). Based on our previous analyses (MacLachlan et al., 2017a,b), such a change represents an optimum urban land cover conversion. However, our temperature modeling indicates that large vegetation features (e.g., trees) could be more effectively planned for the new landscape layout to better mitigate excess temperatures. Modeled temperature changes for the proposed redevelopment plans (as per the blueprints) indicate a potential 1.2°C reduction in average annual temperature across the site, in comparison to the original retail complex. Yet, further to this, while maintaining proposed building layout and vegetation design characteristics, placing trees in optimal locations relative to modeled temperature values indicates that temperatures could be further abated by 0.8°C across the site, with a maximum average temperature reduction of 0.9°C (Figure 4). This analysis must be contextualized within the aim to maximize temperature reduction for UHI mitigation, yet other competing design factors such as the view from windows could influence placement. Regardless, with spatial quantification of such design factors, analysis could be recomputed to further refine tree placement and maintain optimal temperature reduction.
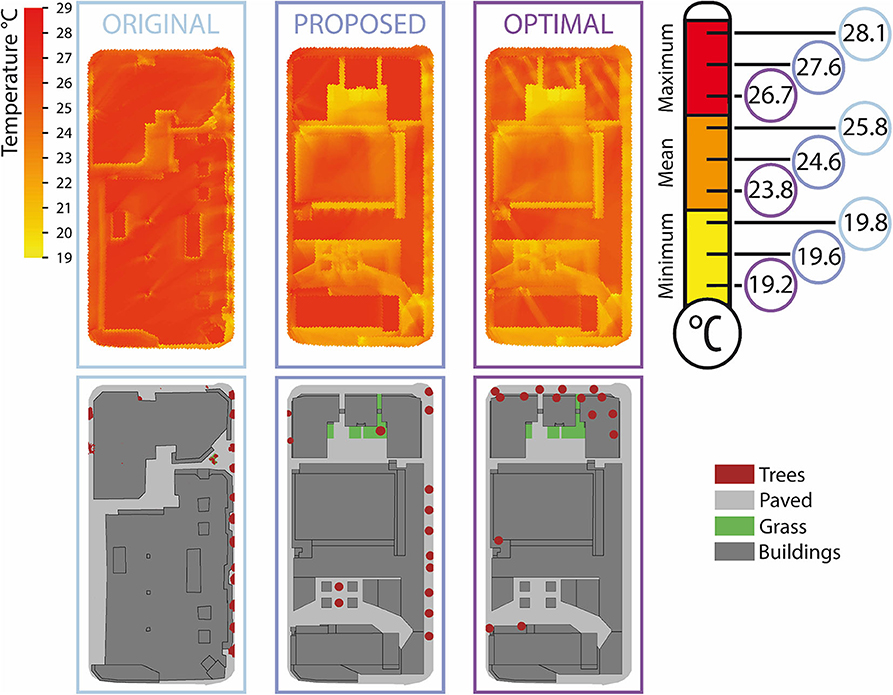
Figure 4. Average annual temperature using the original urban footprint, proposed redevelopment plans, and proposed plans with optimal tree placement. Also indicated are the average annual minimum, mean, and maximum mean radiant temperature with color-coded circles for each urban footprint scenario. The proposed redevelopment constitutes increased building height and variation across the site enlarging shading potential, reducing site mean radiant temperature compared to the original urban area.
Strategic Planning for Localized UHI Mitigation
Reactively, planning initiatives by the City of Fremantle and City of Perth have initiated novel EO data-driven urban forest plans for directing tree planting, whereby data obtained every 16 days with values per 30 m2 of land surface area have been used to produce static summer average land surface temperatures aggregated up to city block. Such decentralized initiatives are advancing other local schemes, including Pennsylvania's “Treevitalize,” and in Arizona and California where state urban forestry programs aim to increase tree coverage. The process of urban greening assists in cooling environments through shading and evapotranspiration, reducing the amount of solar radiation reaching the ground and providing ambient heat dissipation, respectively (evidence drawn from assessments in Table 1). Increased neighborhood green [and blue (Gascon et al., 2017)] space has also been positively associated with better mental health outcomes, such as in Wisconsin (Beyer et al., 2014) and London (Taylor et al., 2016). In terms of relevant enforceable landscape ordinance in Perth, 10% of any gross subdivisible area is required for public open space, normally as a green recreation reserve, a policy that has remained unaltered since the first (Stephenson–Hepburn) metropolitan regional plan was legislated in 1955. These stipulations for open space were based on population density values, with an assumed number of persons likely to be housed across various residential codes (dwellings per hectare), which ensued to be a gross underestimation for required open space (Grose, 2007). Comparatively, Singapore's 2011 open space provisioning is defined as 4.05 m2 for every 56.0 m2 of gross floor area with detailed landscaping requirements such as grass coverage, tree girth, and minimum branches, yet excludes landscape arrangement conditions (Table 1).
Despite such regulations for promoting city open spaces, global policies lack an effective data-driven approach in deriving optimal vegetative placement—particularly for reducing the ever-increasing socio-economic and environmental impacts associated with UHI effects—with current methods restricted by a lack of spatial data and analysis or coarse resolution (e.g., 30 m2), block aggregation, limited temporal data collection (e.g., every 16 days), and outdated open space legislation. Additionally, recently published and updated international policies express the importance of UHI reduction due to current exclusion from global climate models, and as a result, metropolitan spatial development strategies are having to evolve to reflect these model omissions. Nonetheless, modern city development and restorative urban schemes are hampered by a lack of consistent and scientifically valid decisions that can unnecessarily exacerbate the UHI effect (Table 1).
While it is accepted throughout literature that UHIs are the result of additional dark (often impermeable) urban surfaces and the removal or lack of vegetation additional factors such as cloud cover, cyclic solar radiation, building material type, and anthropogenic energy sources can exacerbate the effect (Frumkin, 2002; Voogt and Oke, 2003; Rizwan et al., 2008). Nevertheless, such considerations are challenging to incorporate into localized temperature modeling and often beyond the scope and budgetary constraints of planning departments (e.g., Fremantle's 2017 Urban Forest Plan). Consequently, here we have presented a simplistic and achievable data-driven modeling approach that can be universally integrated into urban planning regulation frameworks. Specifically, planning departments could mandate optimum placement of vegetation based on data-driven analysis using proposed development plans to mitigate excess temperature, while allowing reasonable concessions for building design features or requirements where appropriate. Such a straightforward unified approach is exemplified as especially important due to the range of health, energy, water, and economic savings attributable to a 1°C increase in temperature, for example, an associated 7.5% electricity demand rise in Bangkok (Santamouris et al., 2015) and a 2.1% increase in respiratory admissions in six Mediterranean cities in relation to maximum apparent temperature (Michelozzi et al., 2009). Application of our approach will permit expansion and redevelopment of other global cities while mitigating the localized UHI effect and associated negative environmental, social, and economic consequences. Not only will this provide structured means for city councils to conform to UHI reduction requirements locally, it will also ensure long-term city sustainability, aligning future development with global policies, for example, contributions to meeting the UN Sustainable Development Goal 11, target 3 that specifies sustainable human settlement planning and management alongside the New Urban Agenda call for action 54 that commits member states to reducing the financial, environmental, and public health costs of the UHI.
While elevation and land cover inputs used within this analysis were determined from aerial EO, required layers could be substituted with data from alternative sources. This could include digitization from publicly available planning applications such as those provided by the City of Fremantle's planning portal, or alternative freely available data sources such as Google Earth, Open Topography (elevation), GlobeLand 30 (land cover), national Light Detection and Ranging (LiDAR) surface model composites (e.g., England and Australia), and high-resolution datasets (e.g., the State of Indiana's aerial, LiDAR and elevation data) (Intergovernmental Committee on Surveying Mapping, 2008; Chen et al., 2015; Environment Agency, 2016; The State of Indiana, 2017). Consequently, our accessible approach summarized in Figure 5 can easily be replicated within planning procedures to aid the sustainable development and restorative urban design of our cities for localized UHI reduction.
Recommendations
Urbanized areas are expected to triple by 2030 (2,000 baseline of 652,825 km2) with the Cities and Biodiversity Outlook estimating that 60% of land projected to be urban by 2030 is yet to be built. Current voluntary and policy UHI mitigation policies that aim to increase vegetation coverage frequently fail in determining effective distribution owing to a lack of spatial data, broad aggregation, and selective temporal sampling (Table 1). Integration of an UHI mitigation approach into international and metropolitan spatial development strategies would overcome the current inconsistent and varied urban planning methodologies exhibited through frequent devolution to local governments and the incomparability of their results. Upon receipt of development applications, our approach could be replicated by planning agencies to ensure strategic tree placement in reducing overall temperature, a consideration that should be an essential pre-requisite in planning approval for enhancing localized UHI mitigation (Figure 5). Inclusion at the proposal stage would mitigate aforementioned implications and diminish the requirement for extensive urban forest plans and associated financial costs, e.g., estimated at AUD 2.57 million for the complete City of Fremantle strategy (Table 1). Similarly, application to existing metropolitan regions could maximize often limited resource investment in mitigating locations found to obtain maximum temperatures, overcoming the limitations of current methods (e.g., block aggregation). Through scientifically rigorous data-informed governance, metropolitan cities and federations will be able to effectively interact with, and numerically quantify, mitigation actions for meeting international sustainable development policy requirements such as the reduction of environmental costs (e.g., excess temperature) of the UHI specified in the New Urban Agenda (McPherson, 2016). We advocate that the transformation of multi-level policy to incorporate data-driven frameworks, as we have proposed here, is an essential requirement for ensuring the future socio-economic and environmental sustainability of our cities.
Data Availability Statement
The datasets generated for this study are available on request to the corresponding author.
Author Contributions
All authors assisted in conceiving and designing the experiments lead by AM. AM performed the experiments and analyzed the data. EB, GR, and BB contributed materials/analysis tools. AM wrote the paper with input and revisions from EB, GR, and BB.
Funding
This work was supported by the Economic and Social Research Council (grant number ES/J500161/1) with funding support from the University of Southampton via the World University Network (WUN) researcher mobility programme.
Conflict of Interest
The authors declare that the research was conducted in the absence of any commercial or financial relationships that could be construed as a potential conflict of interest.
Acknowledgments
We would like to thank Mayor Brad Pettitt, Paul Garbett, Marcel Maron, and Gavin Giles from the City of Fremantle for operational planning insights. Access to Urban Monitor high-resolution data was provided in part through the National Environmental Science Program, Clean Air, and Urban Landscapes Hub.
References
ABS (2015). Australian National Accounts 1988-2015. Belconnen, ACT: Australian Bureau of Statistics.
Acuto, M. (2018). Global science for city policy. Sci. Policy Forum 359, 165–166. doi: 10.1126/science.aao2728
Beyer, K. M. M., Kaltenbach, A., Szabo, A., Bogar, S., Javier Nieto, F., and Malecki, K. M. (2014). Exposure to neighborhood green space and mental health: evidence from the survey of the health of wisconsin. Int. J. Environ. Res. Public Health 11, 3453–3472. doi: 10.3390/ijerph110303453
Caccetta, P., Collings, S., Devereux, A., Hingee, K., Mcfarlane, D., Traylen, A., et al. (2012). Urban Monitor: Enabling Effective Monitoring and Management of Urban and Coastal Environments Using Digital Aerial Photography Final Report – Transformation of Aerial Photography Into Digital Raster Information Products. CSIRO.
Chen, J., Chen, J., Liao, A., Cao, X., Chen, L., Chen, X., et al. (2015). Global land cover mapping at 30m resolution: a POK-based operational approach. ISPRS J. Photogramm. Remote Sens. 103, 7–27. doi: 10.1016/j.isprsjprs.2014.09.002
Chen, L., Yu, B., Yang, F., and Mayer, H. (2016). Intra-urban differences of mean radiant temperature in different urban settings in Shanghai and implications for heat stress under heat waves: A GIS-based approach. Energy Build. 130, 829–842. doi: 10.1016/j.enbuild.2016.09.014
Dhakal, S. P. (2014). Glimpses of sustainability in Perth, Western Australia : capturing and communicating the adaptive capacity of an activist group. Cons. J. Sustain. Dev. 11, 167–182. Available online at: http://www.jstor.org/stable/26188736
Eliot, M. J., Travers, A., and Eliot, I. (2006). Morphology of a low-energy beach, Como beach, Western Australia. J. Coast. Res. 221, 63–77. doi: 10.2112/05A-0006.1
Estrada, F., Botzen, W. J. W., and Tol, R. S. J. (2017). A global economic assessment of city policies to reduce climate change impacts. Nat. Clim. Chang. 7, 403–406. doi: 10.1038/nclimate3301
Frumkin, H. (2002). Urban sprawl and public health. Public Health Rep. 117, 201–217. doi: 10.1016/S0033-3549(04)50155-3
Gaffin, S. R., Rosenzweig, C., Khanbilvardi, R., Parshall, L., Mahani, S., Glickman, H., et al. (2008). Variations in New York city's urban heat island strength over time and space. Theor. Appl. Climatol. 94, 1–11. doi: 10.1007/s00704-007-0368-3
Gascon, M., Zijlema, W., Vert, C., White, M. P., and Nieuwenhuijsen, M. J. (2017). Outdoor blue spaces, human health and well-being: A systematic review of quantitative studies. Int. J. Hyg. Environ. Health 220, 1207–1221. doi: 10.1016/j.ijheh.2017.08.004
Goggins, W. B., Chan, E. Y. Y., Ng, E., Ren, C., and Chen, L. (2012). Effect modification of the association between short-term meteorological factors and mortality by urban heat islands in Hong Kong. PLoS ONE 7:e38551. doi: 10.1371/journal.pone.0038551
Grose, M. J. (2007). Perth's Stephenson-Hepburn Plan of 1955. Aust. Plan. 44, 20–21. doi: 10.1080/07293682.2007.9982608
Guhathakurta, S., and Gober, P. (2007). The impact of the Phoenix Urban Heat Island on residential water use. J. Am. Plan. Assoc. 73, 317–329. doi: 10.1080/01944360708977980
Holst, J., and Mayer, H. (2011). Impacts of street design parameters on human-biometeorological variables. Meteorol. Zeitschr. 20, 541–552. doi: 10.1127/0941-2948/2011/0254
Höppe, P. (1992). A New Procedure to Determine the Mean Radiant Temperature Outdoors. Wetter und Leb.
Hu, Y., Jia, G., Hou, M., Zhang, X., Zheng, F., and Liu, Y. (2015). The cumulative effects of urban expansion on land surface temperatures in metropolitan Jingjintang, China Yonghong. J. Geophys. Res. Atmos. Res. 9932–9943. doi: 10.1002/2015JD023653
Imhoff, M. L., Zhang, P., Wolfe, R. E., and Bounoua, L. (2010). Remote sensing of the urban heat island effect across biomes in the continental USA. Remote Sens. Environ. 114, 504–513. doi: 10.1016/j.rse.2009.10.008
Intergovernmental Committee on Surveying and Mapping (2008). ICSM Guidelines for Digital Elevation Data. Symonston, ACT: Geoscience Australia.
Kennewell, C., and Shaw, B. J. (2008). Perth, Western Australia. Cities 25, 243–255. doi: 10.1016/j.cities.2008.01.002
Lau, K. K.-L., Lindberg, F., Rayner, D., and Thorsson, S. (2015). The effect of urban geometry on mean radiant temperature under future climate change: a study of three European cities. Int. J. Biometeorol. 59, 799–814. doi: 10.1007/s00484-014-0898-1
Lau, K. K. L., Ren, C., Ho, J., and Ng, E. (2016). Numerical modelling of mean radiant temperature in high-density sub-tropical urban environment. Energy Build. 114, 80–86. doi: 10.1016/j.enbuild.2015.06.035
Lindberg, F., and Grimmond, C. S. B. (2010). Continuous sky view factor maps from high resolution urban digital elevation models. Clim. Res. 42, 177–183. doi: 10.3354/cr00882
Lindberg, F., and Grimmond, C. S. B. (2011). Nature of vegetation and building morphology characteristics across a city: Influence on shadow patterns and mean radiant temperatures in London. Urban Ecosyst. 14, 617–634. doi: 10.1007/s11252-011-0184-5
Lindberg, F., Grimmond, C. S. B., Gabey, A., Huang, B., Kent, C. W., Sun, T., et al. (2018). Urban Multi-scale Environmental Predictor (UMEP): an integrated tool for city-based climate services. Environ. Model. Softw. 99, 70–87. doi: 10.1016/j.envsoft.2017.09.020
Lindberg, F., Grimmond, C. S. B., Gabey, A., Jarvi, L., Kent, C., Krave, N., et al. (2017). Urban Multi-scale Environmental Predictor (UMEP) Manual [WWW Document]. Available online at: https://umep-docs.readthedocs.io/ (accessed September 5, 2018).
Lindberg, F., Holmer, B., and Thorsson, S. (2008). SOLWEIG 1.0 - modelling spatial variations of 3D radiant fluxes and mean radiant temperature in complex urban settings. Int. J. Biometeorol. 52, 697–713. doi: 10.1007/s00484-008-0162-7
Lindberg, F., Jonsson, P., Honjo, T., and Wästberg, D. (2015). Solar energy on building envelopes - 3D modelling in a 2D environment. Sol. Energy 115, 369–378. doi: 10.1016/j.solener.2015.03.001
MacLachlan, A., Biggs, E., Roberts, G., and Boruff, B. (2017a). Urban growth dynamics in Perth, Western Australia: using applied remote sensing for sustainable future planning. Land 1, 1–14. doi: 10.3390/land6010009
MacLachlan, A., Biggs, E., Roberts, G., and Boruff, B. (2017b). Urbanisation-induced land cover temperature dynamics for sustainable future urban heat Island mitigation. Urban Sci. 1, 1–21. doi: 10.3390/urbansci1040038
MacLachlan, A., Biggs, E., Roberts, G., and Boruff, B. (2017c). Classified Earth Observation Data Between 1990 and 2015 for the Perth Metropolitan Region, Western Australia Using the Import Vector Machine Algorithm. Pangaea. doi: 10.1594/PANGAEA.871017
MacLachlan, A., Roberts, G., Biggs, E., and Boruff, B. (2017d). Subpixel land-cover classification for improved urban area estimates using Landsat. Int. J. Remote Sens. 38, 5763–5792. doi: 10.1080/01431161.2017.1346403
McPherson, T. (2016). Scientists must have a say in the future of cities. Nature 538, 165–166. doi: 10.1038/538165a
Michelozzi, P., Accetta, G., De Sario, M., D'Ippoliti, D., Marino, C., Baccini, M., et al. (2009). High temperature and hospitalizations for cardiovascular and respiratory causes in 12 European cities. Am. J. Respir. Crit. Care Med. 179, 383–389. doi: 10.1164/rccm.200802-217OC
Rizwan, A. M., Dennis, L. Y. C., and Liu, C. (2008). A review on the generation, determination and mitigation of Urban Heat Island. J. Environ. Sci. 20, 120–128. doi: 10.1016/S1001-0742(08)60019-4
Santamouris, M., Cartalis, C., Synnefa, A., and Kolokotsa, D. (2015). On the impact of urban heat island and global warming on the power demand and electricity consumption of buildings - a review. Energy Build. 98, 119–124. doi: 10.1016/j.enbuild.2014.09.052
Seto, K. C., Güneralp, B., and Hutyra, L. R. (2012). Global forecasts of urban expansion to 2030 and direct impacts on biodiversity and carbon pools. Proc. Natl. Acad. Sci. U.S.A. 109, 16083–16088. doi: 10.1073/pnas.1211658109
Taylor, M. S., Wheeler, B. W., White, M. P., Economou, T., and Osborne, N. J. (2016). Urban street tree density and antidepressant prescription rates; A cross - sectional study in London, UK. Landsc. Urban Plan. 136, 174–179. doi: 10.1016/j.landurbplan.2014.12.005
The State of Indiana (2017). 2016-2017 Strategic Plan Update for the Indiana Geographic Information Office. IN: Indiana Geographic Information Office.
Voogt, J. A., and Oke, T. R. (2003). Thermal remote sensing of urban climates. Remote Sens. Environ. 86, 370–384. doi: 10.1016/S0034-4257(03)00079-8
Weedon, G. P., Balsamo, G., Bellouin, N., Gomes, S., Best, M. J., and Viterbo, P. (2014). Data methodology applied to ERA-interim reanalysis data. Water Resour. Res. 50, 7505–7514. doi: 10.1002/2014WR015638
Keywords: sustainable development, urban heat island, earth observation, smart cities, applied spatial analysis, data visualization, city science, urban planning
Citation: MacLachlan A, Biggs E, Roberts G and Boruff B (2021) Sustainable City Planning: A Data-Driven Approach for Mitigating Urban Heat. Front. Built Environ. 6:519599. doi: 10.3389/fbuil.2020.519599
Received: 12 December 2019; Accepted: 03 November 2020;
Published: 26 January 2021.
Edited by:
Nahid Mohajeri, University of Oxford, United KingdomReviewed by:
Juan Pablo Rodríguez Sánchez, University of Los Andes, ColombiaKavan Javanroodi, Chalmers University of Technology, Sweden
Copyright © 2021 MacLachlan, Biggs, Roberts and Boruff. This is an open-access article distributed under the terms of the Creative Commons Attribution License (CC BY). The use, distribution or reproduction in other forums is permitted, provided the original author(s) and the copyright owner(s) are credited and that the original publication in this journal is cited, in accordance with accepted academic practice. No use, distribution or reproduction is permitted which does not comply with these terms.
*Correspondence: Andrew MacLachlan, YS5tYWNsYWNobGFuQHVjbC5hYy51aw==