- 1Department of Civil, Construction and Environmental Engineering (CCEE), University of New Mexico (UNM), Albuquerque, NM, United States
- 2CCEE, Electrical and Computer Engineering, Mechanical Engineering, UNM, Albuquerque, NM, United Sates
The 4th industrial revolution started in 2016 and referred to a new phase in the industrial revolution. One of the most significant technological evolvements during the 4th industrial revolution is Augmented Reality (AR) technology. AR superimposes interactional virtual objects/images to real environments. Because of the interaction and see-through characteristics, AR is better applied to engineering than Virtual Reality (VR). The application of AR in civil infrastructure can avoid artificial mistakes, improve efficiency, and saves budget. This article reviews AR applications in civil infrastructure, focusing on research studies in the latest five years (2016–2020) and their milestone developments. More than half of the AR research and implementation studies have focused on the construction domain in the last five years. Researchers deploy AR technologies in on-site construction to assist in discrepancy checking, collaborative communication, and safety checking. AR also uses building information models (BIMs) to produce detailed 3D structural information for visualization. Additionally, AR has been studied for structural health monitoring (SHM), routine and damage detection, energy performance assessment, crack inspection, excavation, and underground utility maintenance. Finally, AR has also been applied for architecture design, city plan, and disaster prediction as an essential part of smart city service. This article discusses the challenges of AR implementation in civil infrastructure and recommends future applications.
Introduction
The 4th industrial revolution is generally believed to start in 2016 (More Ickson and ben Dhaou, 2019). However, the exact starting point is still debatable because it is still an ongoing event. Different countries also tend to have different definitions of the starting time. For instance, German is widely accepted as one of the first countries entering the 4th industrial revolution since 2011 (Pitsis et al., 2020).
Caudell and Mizell proposed the official term “'Augmented Reality” in 1990 (Berryman, 2012). Milgram and Kishino (1994a) defined the concept of “Virtuality Continuum” and clarified the concept of Virtual Reality (VR), Augmented Reality (AR), Mixed Reality (MR), and Augmented Virtuality (AV). Figure 1 shows the classification of these four terms. The real environment is the physical surroundings that the user stands in. The virtual environment is computer-generated objects/information made up of laser/light. With VR, a user completely immerses in an entirely virtual environment and sees no more real objects in the surroundings (Burdea and Coiffet, 2003; Zyda, 2005); while with MR, a user sees both objects in the real surroundings and virtual images (Milgram and Kishino, 1994b; Ohta and Tamura, 2014; Speicher et al., 2019). MR composes of AR and AV. AR displays the real environment enhanced by interactive virtual objects (Milgram and Kishino, 1994a; Carmigniani et al., 2011). AV is to project and control real objects in a virtual world (Ternier et al., 2012; Nahon et al., 2015).
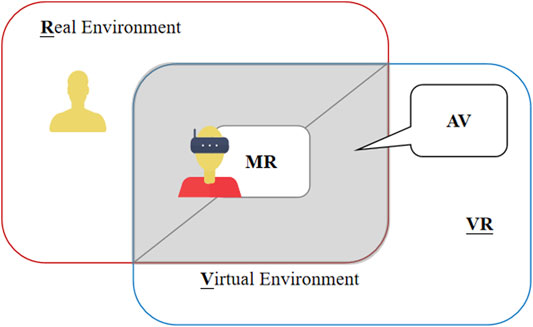
FIGURE 1. Classification of the terms Virtual Reality (VR), Mixed Reality (MR), Augmented Reality (AR), and Augmented Virtuality (AV).
Figure 2 shows the trend of AR implementation in civil infrastructure. The data in Figure 2 are from the number of research articles on the Web of Science (2020). In the last five years (2016–2020), AR has undergone rapid development and implementation in civil infrastructure, compared with the early years (Petrillo et al., 2018; Aoyama, 2019; Cattari et al., 2019; Thompson, 2019). A general and comprehensive overview of the AR implementations in civil infrastructure is needed to invoke more profound AR applications. This article provides a timely and general review of recent AR studies in civil infrastructure and a new application classification.
Figure 3 shows the distribution of the existed publications in different countries/regions (same data with Figure 2.). 40% of the studies are from the United States and China, 24 and 16%, respectively. Figure 3 shows that the United States and China are leading the 4th industrial revolution in the AR area (More Ickson and ben Dhaou, 2019; Pitsis et al., 2020). This conclusion is consistent with recent research studies (Petrillo et al., 2018; Bayode et al., 2019). Bayode et al. (2019) claimed that the United States and China had introduced initiatives albeit with different objectives to support the modernization of the industrial production sector. Petrillo et al. (2018) stated that the economic opportunities of Industry 4.0 are vast and affect the entire economies and countries, mainly including the United States, China, Germany, and the United Kingdom by 2030.
There are two kinds of classification methods for AR implementation in civil infrastructure (Wang et al., 2013): by subjects (building structures, bridges, and railways) or by application scenarios (architecture design, structural design, construction, SHM, and others) (Shin and Dunston, 2008). This article deploys the classification of different AR technologies by application scenarios. Figure 4 shows the percentage of AR applications in civil infrastructure between 2016 and 2020, divided into five significant branches: construction, building information model (BIM), structural health monitoring (SHM) and damage detection, underground utilities, and smart city management. The percentage is calculated by dividing the corresponding category's publication amount in the last five years by the same period's total publication number. The statistics ended on December 31st, 2020. The total publication number in this period is 95. Nearly half of AR implementations in civil infrastructure have focused on the on-site construction (45%), followed by SHM and damage detection (20%), BIM (19%), underground (10%), and smart city management (6%). A comprehensive review of AR studies in civil infrastructure education can be found in Diao and Shih (2019), but it was not relevant as a topic for this article.
Researchers should consider comprehensively when selecting the suitable AR platform (Julier et al., 2000; Jahn et al., 2018; Mahmood et al., 2018). There are two major categories of AR systems in general. One is the prototypes developed in labs, and the other one is the commercial AR devices in the market. The prototypes are typically designed for solving specific research problems efficiently but typically lack versatility. Unless a researcher decides to design a particular AR system for a specific research project, a suitable commercial AR device is usually a better choice (Roitman et al., 2017; Wang and Dunston, 2013). When selecting an AR device, all the parameters are classified into four major categories, general properties, sensors, computational capacities, and display capacities. The general properties include weight, price, and product durability (e.g., water resistance, working temperature range, working humidity range, and drop safe distance). Sensors include head-tracking, eye-tracking, depth sensor, GAM (gyroscope, accelerometer, and magnetometer), and GPS sensor. Researchers should also consider processing units, RAM, and storage to fulfill the computational requirements. Connectivity (e.g., Wi-Fi, Bluetooth, and USB) and display capacities (e.g., FoV and camera) are also important considerations. The choice of a suitable AR device is a complect work. Xu et al. (2020) summarized all the parameters and compared sixteen AR devices regarding these parameters. Xu and Doyle et al. (2020) also provide an empirical formula for civil engineers to select a suitable AR device. Aguero et al. (2020) applied the AR device selection procedure and connected AR with sensors in a shaking table experiment. Hossain et al. (2020) used a similar selection procedure and conducted AR modal shape analysis.
In the following sections, the authors reviewed the milestone AR implementations in construction, BIM, SHM and damage detection, underground utilities, and smart city management, respectively. Following the review, this article discusses the current progress and technical challenges of AR implementation in civil infrastructure. Finally, this article concludes and predicts the future AR development direction in civil infrastructure.
Construction
One of the salient features of AR technology is the combination of virtual and real objects. Traditionally, VR was deployed for the construction process visualization (Sacks et al., 2013; Zhao and Lucas, 2015). The pith of VR implementation in construction is the detailed 3D-CAD modeling of both the project and the surroundings (Sampaio and Martins, 2014). Detailed environment information input is also required for VR implementations. However, the requirements of detailed models become impractical with the increasing complexity of the construction procedure (Feeman et al., 2018). With AR technology, the virtual design blueprint and real construction site can be combined and illustrated to engineers and workers in real time, resulting in higher precision and efficiency (Ahmed, 2018). AR does not require detailed modeling of the surroundings or the existing project process. Applying AR in the lifecycle construction site planning can help keep the project within budget and to avoid process mistakes or complement time.
In this study, the authors classified all the AR implementations in construction nowadays into two major research areas to simplify the acknowledgment for the readers, as shown in Figure 5. The two major research areas are monitoring project progress and assisting workers. Studies of project progress monitoring can be divided into two categories, discrepancy checks and quality control. The second research area, the assistance for workers, includes collaborative visualization, telecommunication, and site safety.
Monitoring Project Progress
The significant practical construction challenges of the AR applications are the complicated and arduous outdoor environment, for example, lighting conditions and terrain variation. The monitoring project progress research has been conducted for both hardware and software development.
Discrepancy Check
A primary AR application in construction is the discrepancy check (Son et al., 2015), that is, checking the difference between on-site structures and design models. The first systematic AR implementation for discrepancy check is MARSIFT (Hammad et al., 2002). However, MARSIFT is a promising concept rather than a viable application. Since the development of MARSIFT, AR implementation in civil construction has been increasing, both in theoretic studies (pose estimation, scene understanding (Feng, 2015), and accurate 3D reconstruction of the real environment (Fathi and Brilakis, 2016)) and on-site applications. There are mainly two practical AR implementations in the discrepancy check area, SMART-ARMOR and D4AR.
SMART-ARMOR was developed based on UM-AR-GPS-ROVER (Behzadan and Kamat, 2005). UM-AR-GPS-ROVER’s ability to display virtual mechanicals in an outdoor construction site has been verified with a field experiment. In 2008, Behzadan, 2008; Behzadan et al., 2008; Behzadan and Kamat, 2009a presented ARVISCOPE-ROVER as an updated version of UM-AR-GPS-ROVER. The Global Positioning System (GPS) and orientation tracking technologies were applied in ARVISCOPE to create a dynamic simulation of the models' continuous animations. In the following years, Dong and Kamat, 2010; Kamat et al., 2011 evolved the ARVISCOPE-ROVER system to the more accurate and lighter SMART-ARMOR system. SMART-ARMOR extended the system to a more standard AR development environment by separating AR logic from the application logic part. SMART-ARMOR is a milestone holistic and applicable outdoor construction application of AR technologies.
D4AR was developed by Golparvar-Fard et al. (2009a); Golparvar-Fard et al. (2009b); Golparvar-Fard et al. (2011a); Golparvar-Fard et al. (2011b), applying the registration method to solve the discrepancy check problem. Later, D4AR was updated to the HD4AR system (Bae et al., 2013), enabling the application of available camera-equipped mobile devices. In 2014, bottomed on the D4AR system, manual occlusion management, and “X-ray” metaphors (Zollmann et al., 2012; Karsch et al. (2014) combined a set of AR tools to build a valuable platform, ConstructAide, to monitor project progress. ConstructAide combined unordered photo collections and 3D models to obtain construction progress.
Along with the theoretical development, researchers also conducted field experiments. For instance, by solving the alignment and plumbness problem, the discrepancy check of steel pipe modules assembly was solved by Nahangi, 2015; Nahangi et al., 2015 and Jeanclos et al., 2018; Kwiatek et al., 2018; Kwiatek et al., 2019; Li et al., 2018, capable of extending more general applications.
Quality Control
Quality control is another critical AR application in construction. Zhou et al. (2017) inspected the on-site segment displacement during tunneling construction compared with the baseline model to monitor construction quality. Aguero et al., 2020 connected AR devices and sensors, taking advantage of both the displaying function of AR technology and sensors' inspecting function. Figure 6 shows the design procedure of connecting AR devices and sensors. Figure 7 shows an illustrative experiment that Aguero et al., 2020 conducted to verify the AR-sensor connection.
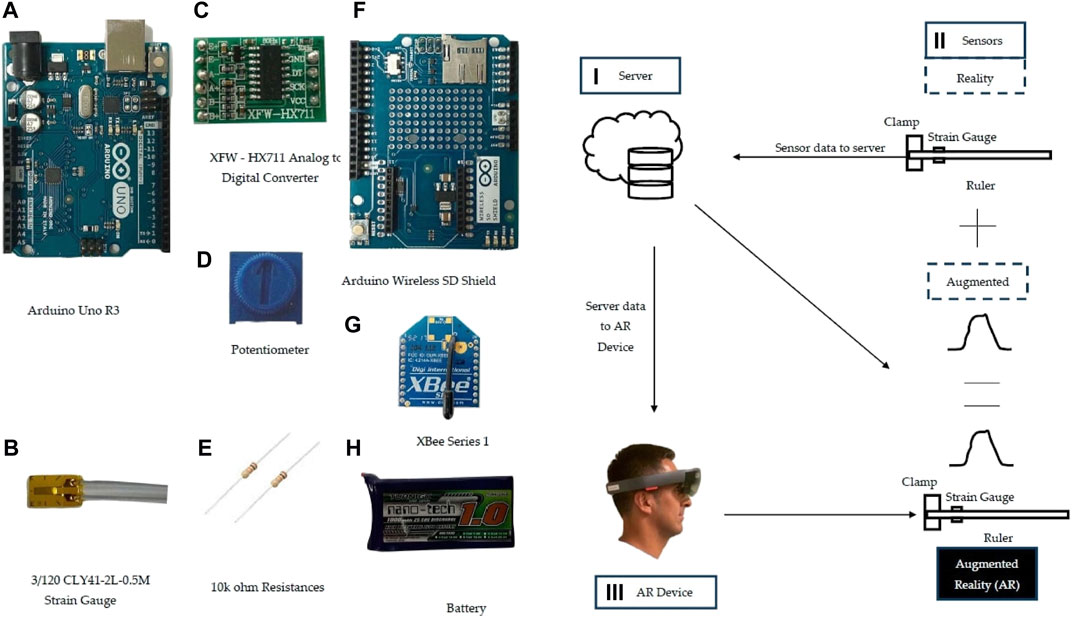
FIGURE 6. Connecting AR devices with sensors (Aguero et al., 2020).
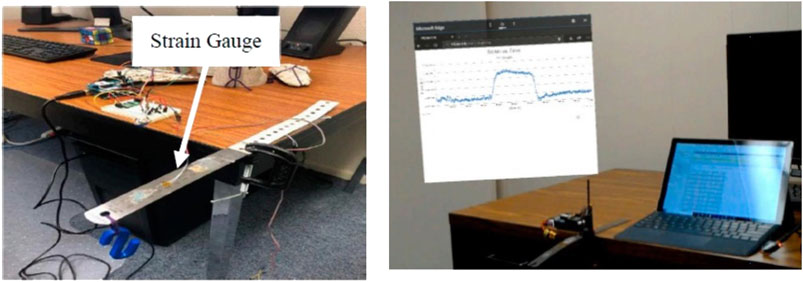
FIGURE 7. Experiments of connection between AR devices and sensors (Aguero et al., 2020).
Assisting Workers
High-efficiency collaborative visualization and communication among multiple users can be realized by applying AR technologies.
Collaborative Visualization
Dong et al. (2013) developed an AR application, ARVita, for the collaborative visualization of multiple users wearing HMDs. Using ARVita, all the uses can interact with the dynamic visuals of the real-time construction processes. Soman et al. (2017) developed an application to share AR images between designers and the field site. Hammad et al. (2009) developed a prototype distributed AR visualization collaborative system, DARCC, which was tested in a bridge deck rehabilitation project to show the effectiveness.
Telecommunication
Telecommunication can provide high-performance guidance for workers. In 2003, Aiteanu et al. (2003) invented an AR helmet to provide an online welding assistant to improve welders working conditions. Chi et al. (2012) designed an AR interface to teleoperate remote cranes by retrieving information from the field and enhancing the decision-making processes.
Site Safety
Another significant application is to guarantee site safety by visualizing complex workplace situations with AR devices. (Li et al., 2018). Kim et al. (2017) developed an AR system to provide workers safety information to avoid hazards. Liu et al., 2018 deployed AR technologies to give the workers alarms of dangers from falling.
The authors are committed to the implementation of AR to increase the safety of workers. The authors used AR to mark the bridge components for the inspectors, as shown in Figure 8. The authors are also designing an AR application using eye-tracking data to draw a cognitive map for Federal Railroad Administration (FRA) to increase railroader safety during field inspections.
In the future, AR construction research trends are real-time monitoring and the human factor. A construction site is a dynamic and fast-changing scenario. Hence, to effectively monitor real-time site information, the fast-response requirements for AR algorithms and devices are essential. Real-time monitoring has become a hot topic for AR construction. Zollmann et al. (2012) developed an application using overview and detail techniques to visualize real-time dynamic data for construction sites with AR. Behzadan and Kamat (2011) furthered the ARVISCOPE-ROVER system's study to visualize 3D AR animations of active engineering operations. Akhavian and Behzadan (2012) developed a framework driven by data to collect real-time field data and visualize dynamic 3D simulations of engineering activities. Liu et al. (2018) developed a rapid construction site information acquisition method to conduct dynamic site layout planning. Besides overcoming technical difficulties, performance-based decisions are being considered. Dunson and Wang (2005), Wang and Dunston (2006), Wang and Dunston (2008), and Dunston and Wang (2011) analyzed the feasibility of considering human factors in AR applications to develop visualization tools for construction tasks.
Most research and devices were in the experiment and prototype phase yet not applied to practical site construction. The main obstacle to adopting AR technologies during construction was the high expense and immature devices (Manuel Davila Delgado et al., 2020). Developing rapidly in recent years, AR devices suitable for construction are already commercially available. An eligible AR device can be selected by appropriate performance requirements for a different construction site. For example, the RealWear HMT-1Z1 should be chosen for high-risk (e.g., potentially explosive areas) and high-value construction for its safety and high price. At the same time, Google Glass Enterprise Edition 2 is fit for daily civil infrastructure for its lightweight. Besides HMDs and handheld displays, the combination of unmanned aerial vehicle (UAV) and AR technologies to conduct holistic site planning and large-scale terrain scanning is a significant trend. Wen and Kang (2014) enhanced and prototyped a UAV with AR technologies to provide site-level image transmission. Zollmann et al. (2014) used UAV to conduct 3D reconstruction of site information, providing on-site visualization on mobile. Yan et al. (2019) combined UAV and AR to run landscape simulations. Commercial AR-UAVs for construction could be the next important component of the AR market.
Another challenge is workforce training. Since AR technology is new to most construction workers, efficient training is necessary to implement AR technology in a real construction project. The workers will need to understand basic AR knowledge and AR device operating methods.
Other Implementations
Building Information Models
Many researchers classify BIM as an application in construction because of the extensive implementations of BIM in construction. Nonetheless, given the significance, AR-enhanced BIM is classified as a separate category in this article. Combined with AR technologies' visualization potential, BIM can perform as a functional decision support system (Chen et al., 2015; Machado and Vilela, 2020). A holistic bibliometric literature review of BIM between 2005 and 2015 can be found in Santos et al. (2017) and Abdirad (2017), concluding that AR-enhanced BIM is one of the main trends of practical BIM application. This section mainly focused on the research trend after 2015.
BIM is a functional tool for structural information management. Combined with AR technologies, visualization and intuition are enhanced for displaying information to engineers. The merging of AR and BIM maximized both the AR's visualization advantage and BIM's information management advantage (Wang et al., 2015). In 2014, Meža et al. (2014) conducted studies on information storage and regeneration and presented a prototype software system tested in a real project. In 2018, Bruno et al. (2018) conducted a diagnosis and performance assessment for existing buildings, finding that information management is crucial for AR-enhanced BIM. In 2020, Dang and Shim (2020) proposed an innovative bridge maintenance system using AR-enhanced BIM to provide bridge maintenance information for engineers.
(Hossain et al., 2020) used AR technology to overlap model shapes onto the real specimen in a shaking table experiment, as shown in Figure 9. The model shape of the specimen was changed and displayed in real time, with the real specimen moving.
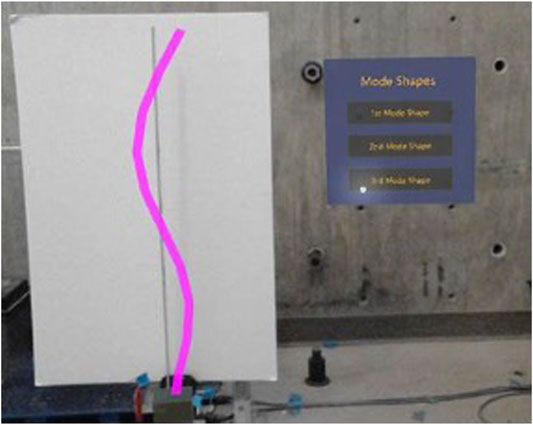
FIGURE 9. Displaying virtual model shape onto the shaking table experiments. Hossain et al. (2020).
Providing systematic information management, defects, and rework during the construction process can be minimized, hence guaranteeing project schedule and cost budget, verified by a quantitative analysis conducted by Hwang et al. (2019). Kim et al. (2015) combined BIM and 3D laser scanning technology to perform quality monitoring for concrete elements. Schickert et al. (2018) conducted nondestructive testing for the concrete specimen with BIM framework and AR-enhanced visualization.
Many AR-enhanced BIM applications are now available for holistic lifecycle management and specialized construction. For example, Tang et al. (2016) proposed a conceptual workflow with the BIM-GIS application for the prefabrication design to save construction time, increase efficiency, and reduce energy consumption; GhaffarianHoseini et al. (2017) suggested engagement of a BIM application, BIM-IKBMS, for the building management after construction.
For the application of standard software, Abanda et al. (2015) compared 122 BIM software applications for project management. Considering that each software has pros and cons, and different software can complete similar targets, Abanda et al. (2015) suggested selecting BIM software should consider supply chain partners and the project's lifecycle. Barazzetti and Banfi (2017) reviewed commercially available BIM software for historic buildings and suggested that the BIM applications for handheld AR devices, A360 (Autodesk (n.d.), 2016), iVisit3D (Artlantis Studio (n.d.), 2019), BIMx (Graphisoft, 2011), and Augmented 3D (2020), best fit AR technologies. Kopsida and Brilakis (2017) evaluated different software designed for AR-enhanced BIM applications, concluding that a gap still exists between AR-enhanced BIM technologies and practical on-site applications. Rahimian et al. (2020) proposed an AR-enhanced BIM framework to integrate structural elements' visualization information using Unify.
The authors are developing the interface of finite element models (FEMs) in AR for hybrid experiments (Figure 10.) The user can interface with a hologram of one virtual FEM experiment using a shaking table input created by the computer. The user can define the mass, stiffness, and height of each story in the interface. In a hybrid experiment, some structural components' movement is calculated in the computer, while others are tested using a real shaking table (Figure 11). The AR interface can superpose the virtual components onto the real ones, showing a whole structure with consistent and intuitive dynamic moving. More complicated BIM models will be developed based on this prototype.
Among all the HMD devices, Microsoft HoloLens 2 has the enormous potential to be the game-changer for the AR-enhanced BIM application. First, HoloLens 2 is embedded with a robust depth sensor. Hence, it is capable of 3D monitoring. Second, the programming platform of HoloLens 2, Unity, is relatively completed and widely accepted by engineers. Last, HoloLens 2 is compatible with most general civil infrastructural models, for example, Autodesk 3D model and Trimble SketchUp model. A detailed comparison of the available AR devices is given in Xu et al., 2020.
Life Cycle Management
AR technologies can also be used in the energy performance assessment of a building. Traditional energy performance assessment for a building is time-consuming and unwieldy. The application of AR technologies can save assessing time, reduce operation difficulty, and enable engineers to monitor real-time energy abnormity. In 2005, Malkawi and Srinivasan (2005) presented an interactive and immersive AR model showing an indoor temperature field by HMD modified in 2009 using a mobile robot to achieve robust visualization. Ham and Golparvar-Fard (2012) conducted an energy performance assessment by a new approach, EPAR, using unordered digital and thermal imagery. Experiments have verified the robustness of EPAR. Based on the EPAR models, Golparvar-Fard and Ham (2014) developed new algorithms to conduct rapid automated analysis. Wang et al. (2013) developed a prototype hybrid LIDAR system for rapid 3D thermal monitoring. Liu and Seipel (2015) provided another novel algorithm with quadrilateral features registration (e.g., windows) for building's thermal monitoring.
Structural Health Monitoring and Damage Detection
AR-based SHM is more suitable for routine inspection and monitoring than other tasks, for example, special inspection, testing, and maintenance (Peres et al., 2018). AR technologies are mainly used in the infrastructure SHM and crack inspection.
The safety of bridges is of great significance during heavy transportation today. More and more bridges are reaching the designed service year corresponding to the increasing safety requirements, leading to massive SHM and detection necessities (Moreu, 2015; Casas and Moughty, 2017). Traditional manual monitoring methods can hardly fulfill the rapidly increasing monitoring tasks. To improve monitoring efficiency, Akula et al., 2015 conducted SHM for highway bridges using a context-aware computing framework. The framework automatically identified the component of interest and retrieved relevant information from the database. Computer vision techniques are functional for crack detection during SHM. Wu (2015) developed a novel self-adaptive image-processing algorithm for the crack detection of road pavements. Lattanzi et al. (2016) conducted seismic damage detection for bridge columns. Computer vision techniques were used to estimate the peak displacement during the earthquake by damage observations from images captured by cameras.
SHM and damage detection are essential for critical infrastructure during disaster and post-disaster. In Section 2, the application of AR technologies for discrepancy check during construction has been introduced. Similar platforms can be applied for post-disaster damage detection. By comparing the post-disaster real structure and previously stored AR building information, structural damage can be observed (Kamat and El Tawil, 2007). Tedeschi and Benedetto (2017) designed an automatic recognition system to inspect pavements for potholes, longitudinal–transversal cracks, and fatigue cracks for pavements. Dang et al. (2018) developed 3D digital twin models with related damage records for typical bridge structures as a database to predict a similar structure's reliable future performance. Napolitano et al. (2019) developed a novel AR framework for data visualization during the structural inspection.
With the improvement of the display, cameras, and depth sensors of HMDs, conducting SHM and damage detection with HMDs has been studied. Yamaguchi et al. (2019) developed an AR crack inspection support system for concrete structures with HoloLens. Maharjan et al. (2019) adopted HoloLens to conduct bridge inspection in a controlled laboratory environment and found that applying AR technologies in SHM leads to higher safety and a smaller workload for field inspectors.
Napolitano et al., 2019 used AR to conduct structural health monitoring, as shown in Figure 12. AR technology helped to capture the real environment (the building information) in the field (Figure 12A) and reanchoring the image in a new position (Figure 12B).
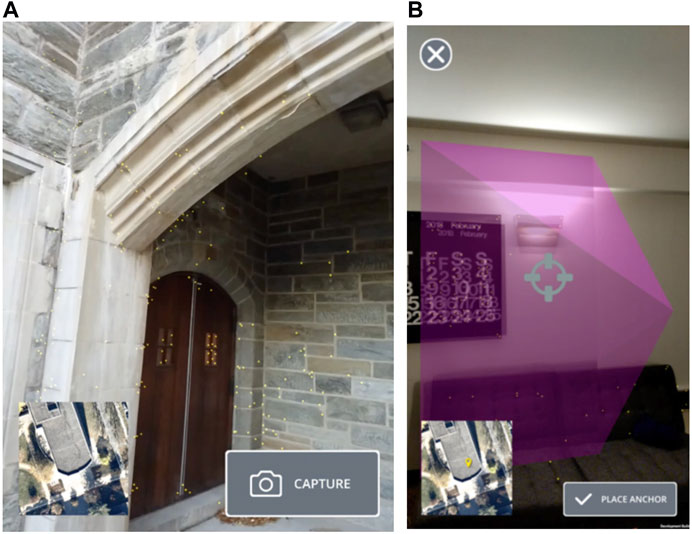
FIGURE 12. (A) Initial capture interface. (B) Reanchoring an image. The pink pyramid represents the relationship between the camera position and the image plane in terms of position and pose.
The authors developed an AR application named “Time Machine Measure (TMM)” to assist in SHM. Traditionally, researchers need to use markers to measure over time if an object's moving distance needs to be measured. TMM provides a time machine function using the real environment's spatial mapping, restoring its position at a previous timestamp. Therefore, researchers can directly measure the moving distance of an object without tedious and time-consuming marking procedures. Figure 13 shows an illustration of measurement with TMM. In Figure 13A, the green cube is the original position of the cube storage (Position-1), and the blue one is the position after a move (Position-2). The blue cube is real, while the green one is an invoked virtual image marking the original position. With this function, the moving distance can be conveniently measured as 0.91 m. Figure 13B shows that the cube is moved again to Position-3 (the cyan cube). Similarly, the cyan cube is real, while the blue and green ones are invoked virtual images. Figure 13B shows that the moving distance from Position-1 to Position-3 is 1.88 m. This measurement assistant AR application simplifies the SHM work for field inspections.
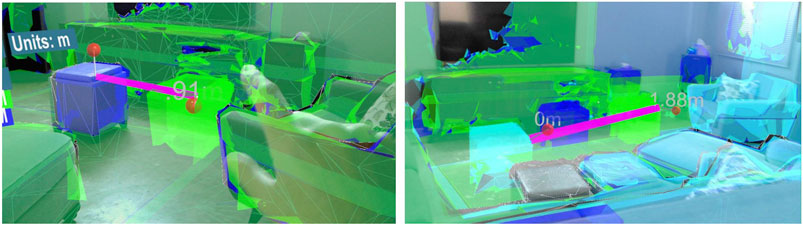
FIGURE 13. AR application–Time Machine Measure. (A) TMM measurement from Position-1 to Position-2. (B) TMM measurement from Position-1 to Position-3.
During SHM and damage detection, functional depth sensor and high-resolution cameras are requisite to HMDs. The only devices meeting both requirements are HoloLens and ThirdEye Gen X2 (Xu et al., 2020). The larger 52° FOV makes HoloLens a more popular device for researchers. However, studies of HoloLens applied in SHM are still in the experimental phase. More on-site field experiments should be conducted to verify reliability. Besides HMDs, a novel AR projector, LF2, could also be a game-changer for damage detection if the reliability under strong sunlight can be improved. As a projector, LF2 is not limited by FOV, processing, or storage capacity.
Underground Utilities
Underground utilities encompass underground geological structures (e.g., basements, foundations, garages, tunnels, and subways) and pipelines (e.g., water, sewage, gas, electricity, and telecommunications) (Bobylev, 2009). The application of AR technologies superimposed virtual underground information to the real aboveground environment, preventing accidental utility strikes during excavation and improving efficiency in maintenance (Kaddioui et al., 2019).
The major challenge is the localization problem (Pereira et al., 2020), that is, how to guarantee the accuracy of the superimposition to deploy AR technologies. Traditionally, abstaining location information required merging data from blueprints, historical records, dead reckoning, and aboveground sensors, which are time-consuming and lack accuracy (Li et al., 2015). The leading solutions today to solve the localization problem consist of utilizing the GPS and ground penetrating radar (GPR) (Kaddioui et al., 2019; Ortega et al., 2019). Dai et al. (2011) proposed an analytical approach compiled with GPS to incorporate virtual underground infrastructure into site photos to provide more invisible information. Pereira et al. (2018); Pereira et al. (2019) provided a novel GPR system integrated with AR to solve the localization problem. The proposed GPR system was capable of arbitrary-route scanning with high fidelity.
With the development of HMDs and handheld devices, the information traditionally stored in office-based desktop computers can be shown directly to engineers in the field. Webster et al. (1996) first developed an AR HMD system for architectural construction. The system had the see-through characteristic and could show the columns occluded by walls and rebars inside the columns. Behzadan and Kamat (2009b) combined the HMD device with GPS and CAD models to superimpose real-time virtual underground utilities over the real environment on an excavation site. Schall et al. (2010) designed a novel mobile AR device, VIDENTE, to maintain, plan, and survey underground infrastructure using spatial interaction and visualization techniques. Improved from Vesp'R (Schall et al., 2009), VIDENTE was a relatively complete and functional AR device, getting positive feedback from utility companies. Roberts et al. (2002) developed an AR see-through system compatible with HMDs and handheld laptops, solving the Tracker Technology positioning problem.
Bentley Systems developed a relatively mature and available prototype application for the AR-enhanced underground utility visualization software. The application combined AR, GPR, and BIM and can measure the distance between underground objectives.
Smart City
The official definition of “smart city” has not been universally accepted. However, a common understanding is conducting city management by collecting information from residents and the IoT (Kyriazis et al., 2013; Poslad et al., 2015; Giang et al., 2016). AR is playing a big part in smart city service, for example, public transport (Novi Sad, Serbia) based on the IoT devices installed on buses (Pokric et al., 2014). Golparvar-Fard et al. (2015) and Balali (2015) proposed a novel algorithm to monitor and preserve the high-quantity and low-cost assets on highways (e.g., traffic signals and light poles). Besides transportation, AR technologies have also been deployed in the city plan and architecture design.
Early in 1999, Thomas et al. (1999) designed an AR platform, TINMITH2, to visualize the architecture design in real surroundings. Due to computation limits, the architecture was depicted with single lines. Wang (2009) considered using AR technologies for architecture design in 2009. However, due to the technology limit, a practical 3D rendering prototype application was not completed. The primary technology limit is the transition from legally approved 2D design drawing to well-structured integrated 3D models readily for AR systems. The maturity of 3D BIM design promoted the application of AR in architecture design. For example, in 2013, Côté et al. (2013) interpreted 2D engineering drawings to the 3D real environment directly with an animated sliding plane and model clipping technique. The mobile AR application developed by VTT Technical Research Center of Finland (VTT, 2020) was the first practical application, helping plan a hotel in Billnäs ironworks site at Raseborg, Kämp Tower in Jätkäsaari, Helsinki, and a wind generator farm in Pörtom, Närpes (Woodward, 2015).
Traditionally, existing structures and natural objects need to be modeled in 3D CAD during city planning to illustrate the relative position and height relation, which is time-consuming and money-costing. AR is a powerful tool for both landscape and height design during a city plan. In 2011, to preserve historic buildings’ good landscapes and provide a reasonable basis for city height regulations, Yabuki et al. (2011) developed a feasible and effective system deploying ARToolKit to measure the maximum invisible height of each building. A typical example illustrating the AR city plan is conducted in Christchurch, New Zealand. A mobile AR application, CityViewAR (no longer available), was used to assist the post-quake city plan and reconstruction of destroyed buildings for the first time (Lee et al., 2012).
Satellites are widely implemented to obtain data for building smart cities. For example, DSCOVR satellite (Deep Space Climate Observatory satellite), launched on February 11th, 2015, can monitor the real-time solar wind in the Unites States, hence to the accuracy forecast weather alerts (NOAA, 2015). The authors developed an AR application, SMAC, to monitor and control the satellites, including the position (latitude and altitude), velocity, and other data collected by the satellites, as shown in Figure 14. Until now, SMAC can access three satellites, SHARC, DSCOVR, and the International Space Station. SMAC can assist smart city management with access to more satellite data, such as traffic monitoring data and communication network data.
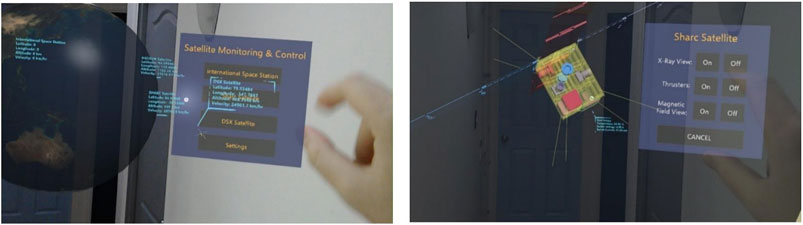
FIGURE 14. Satellite monitoring and control with AR technology. (A) Satellite monitoring and control interface. (B) Control the SHARC satellite.
HoloLens and Unity platform were further investigated among all the available AR devices. Gang et al. (2016) developed a toolkit by Unity to conduct water hazard prediction by monitoring real-time precipitation and hydrological information. Ergün et al. (2019) tested HoloLens by 21 participants in an in-house workshop, showing the potential of using HoloLens in AR-assisted architecture design. As an essential part of the smart city, the rapid development of applying HoloLens in city plan and architecture design is foreseeable.
Discussion
The authors generate a synthesis comparison table to summarize the current progress and technical challenges for each AR implementation category in civil infrastructure. As discussed in Sections Construction and Other Implementations, AR implementations in civil infrastructure can be divided into construction, BIM, SHM, underground utilities, and smart city management. Implementations in construction are further divided into monitoring project progress and assisting workers. With this comparison table, readers can generate a thorough understanding of AR implementations in civil infrastructure today and compare their study to the frontiers in the corresponding area. References in Table 1 are the typical studies in the correlated category. Comparably, AR technologies in discrepancy check, quality control, BIM, and SHM are more mature. Multiple prototypes have been designed, and small-scale field experiments have been conducted.
There are three fundamental technologies in AR implementations, display technology, tracking registration technology, and human–computer interaction technology (Zhang et al., 2020). In the three fundamental AR technologies, five significant technical challenges hinder the AR development in civil infrastructure today are as follows:
1)Occlusion problem. Occlusion problem is one of the two major fundamental technical issues in the various AR implementations in civil infrastructure. Occlusion problem exists in every mobile AR application and HMD, limiting the augmented range and accuracy. The standard solution for the occlusion problem is to pre-map the surroundings to avoid solving the real-time occlusion problem. However, researchers have proposed more acceptable methods for the real-time occlusion problem (Holynski and Kopf, 2018). Applying recent theories to AR devices can motivate more AR implementations, although the occlusion problem still has a long way to the perfect solution.
2)Latency problem. Another major fundamental challenge for AR technology is the latency problem, that is, the time-delay problem. The time delay of the virtual environment calculation can cause a displacement between the real and virtual environments. Two display methods exist when merging the real and virtual environments, optical see-through and video see-through. The optical see-through method displays the real environment through a glass with virtual objects showing on the glass (e.g., Microsoft HoloLens
3)The see-through video method captures the real environment by a camera and shows both the images in the real environment and the virtual objects on the same monitor screen. The synchronization problem of the video see-through display method can be solved by adding a time delay for the real environment on purpose to offset the virtual environment (Kanbara et al., 2000). However, time delay cannot be eliminated entirely for the optical see-through method but can only be minimized by accelerating the virtual environment's calculation (Kiyokawa et al., 2003).
4)Outdoor environments. Today, most AR devices, including both prototypes and commercial devices, use lasers to overlap virtual images onto the real environment. Lasers work well and stable in indoor environments. However, the temperature and luminance changes in outdoor environments can be a challenge to most AR devices. For instance, Microsoft HoloLens 2, as a powerful and comprehensive AR device in the market, still inherits the intrinsic disadvantages of the working environment.
a)HoloLens uses visible light to track the user's location and the surroundings, hence requiring a steady 500–1000 lux luminance for best performance. If the environment is too bright, the cameras can get saturated, and nothing can be seen. If the environment is too dark, the cameras cannot pick up enough information, and nothing is seen (Microsoft, 2019). 500 lux luminance is the light of typical indoor environments, for example, offices, groceries, and laboratories. 1000 lux luminance is the light of bright indoor environments, for example, detailed mechanical workshops and operation theaters.
b)HoloLens operates with the best performance in the environments within the temperature range of 10–27°C (50–81°F) and the humidity range of 8–90% relative humidity. In the authors' experiments, HoloLens can operate in environments out of these ranges. However, implementations out of the recommended range may lead to shorter battery life or device damage.
c)Localization problem is also a challenge for an outdoor environment. AR devices need to scan the surroundings using a depth sensor to generate a detailed spatial map so that virtual images can be displayed in an accurate position. However, in an outdoor environment, the surroundings often lack adequate references like an indoor wall. Some AR devices choose to deploy a GPS sensor to solve the localization problem.
5)Accuracy requirement. To generate realistic virtual images, the positions of the virtual images have to be very accurate. A relatively large position error will lead users to doubt the reality of the AR images and cause motion sickness, dizziness, and nausea.
6)Communication stability and efficiency requirement. AR devices are designed to be able to communicate with each other. This function is beneficial for assisting workers and providing remote directions. In this scenario, the stability and efficiency of the communication are vital. Unstable communication and large latency can cause danger to workers. The speed and quality of remote communications typically depend on the wireless network. Therefore, a sound and reliable local wireless network is necessary for AR communication.
Frontier computer vision and graphics research provide more satisfying solutions for the five previously mentioned AR technical challenges. Today, frontier computer vision research includes four aspects: data collection, low-/mid-level processing, high-level processing, and application (Xu et al., 2020). The frontier data collection technologies try to design a good data collection strategy, for example, layout plan to adverse environmental noise. The frontier low-/mid-level processing technologies exploit GPUs and parallel computing to reduce computational cost and improve accuracy. The frontier high-level processing technologies are combined with BIM to solve the problem of lacking prior models. Finally, all the frontier computer vision technologies lack implementations and tests in real projects, which have become one of the most considerable weaknesses of computer vision and graphics research.
In another article, the authors classified sixteen AR devices available in the market in the last five years regarding civil infrastructure implementation capabilities. The authors compared AR devices’ performances in weight, price, product durability, sensors, connectivity, computational capacities, and display capacities, including the parameters that civil engineers are most interested in, for example, camera resolution, processing unit, and FoV. According to the classification and ranking of that study, Google Glass Enterprise Edition 2, Microsoft HoloLens 2, and Everysight Raptor have the highest combined capabilities to become popular in civil infrastructure. Google Glass Enterprise Edition 2 has the largest FoV, which is essential in construction. Everysight Raptor has the second largest FoV among all the AR devices and the second largest camera resolution. Microsoft HoloLens 2 has all-sided sensors (including depth sensor), a high-resolution display, the third largest FoV, and relatively balanced calculation and storage performances. All the three AR devices have significant potential in the future civil infrastructure implementations.
Conclusion
This article reviewed the research field of AR applications in civil infrastructure, mainly focused on research studies in the latest five years and milestone developments. While several other introductory articles have been written on AR applications in civil engineering, this article is more comprehensive and up-to-date. The most extensive research branch is construction with AR, followed by BIM, SHM, underground utilities, and smart city management. Given that quite a few prototype devices and applications have been designed and tested today, few devices are commercially practical for massive on-site applications. Fit devices are recommended for each application branch. Generally, HoloLens has become the most promising AR HMD for civil infrastructure because of its all-sided sensors (including depth sensor), high-resolution display, larger FOV, and balanced calculation and storage performance.
There are three central research tendencies in the future. First, theoretical breakthroughs in AR technology research are vital, including further studies of the occlusion and latency problems. Second, the weight and price of updated version AR devices should be reduced and improve performance. Finally, a unified AR function “toolbox” platform and benchmark models should be established and well accepted in both the academic and engineering community. The complicated applications and applications can be compared and combined to display the respective superiority. Unity has become a more general platform for AR application development. In the future, researchers should conduct more field experiments to verify the prototypes. With the help from the industry, the verified AR applications will then be implemented more extensively. For a civil engineer who is a novice in AR, the authors suggested the engineer starting the AR studies with relatively more mature platforms, for example, Unity 3D. Besides the references discussed in this article, the authors also recommend reading the Unity manual (Unity, 2021) and starting with the programmed MRTK SDKs (The Mixed Reality Toolkit (MRTK), 2020).
Ethics statement
Written informed consent was obtained from the individuals for the publication of any potentially identifiable images or data included in this article.
Author Contributions
JX collected the data, organized the citations, and drafted the manuscript. FM is the leader of the project and coordinated the access to data, resources, and analysis. FM reviewed the manuscript composition, sources, and the final version. FM secured the funding to conduct this research, access to AR resources, codes, and experiments.
Funding
This research’s financial support is provided in part by the Air Force Research Laboratory (AFRL, Grant number FA9453-18-2-0022) and the New Mexico Consortium (NMC, Grant number 2RNA6.) The conclusions of this research solely represent those of the authors.
Conflict of Interest
The authors declare that the research was conducted in the absence of any commercial or financial relationships that could be construed as a potential conflict of interest.
References
Abanda, F. H., Vidalakis, C., Oti, A. H., and Tah, J. H. M. (2015). A critical analysis of building information modelling systems used in construction projects. Adv. Eng. Softw. 90, 183–201. doi:10.1016/j.advengsoft.2015.08.009
Abdirad, H. (2017). Metric-based BIM implementation assessment: a review of research and practice. Architectural Eng. Des. Manage. 13 (1), 52–78. doi:10.1080/17452007.2016.1183474
Aguero, M., Maharjan, D., del Pilar Rodriguez, M., Mascarenas, D. D. L., and Moreu, F. (2020). Design and implementation of a connection between augmented reality and sensors. Robotics 9 (3), 9010003. doi:10.3390/robotics9010003
Ahmed, S. (2018). A review on using opportunities of augmented reality and virtual reality in construction project management. Organization, technology and management in construction. Int. J. 10 (1), 1839–1852. doi:10.2478/otmcj-2018-0012
Aiteanu, D., Hillers, B., and Graser, A. (2003). “A step forward in manual welding: demonstration of augmented reality helmet,” in The second IEEE and ACM international symposium on mixed and augmented reality, Tokyo, TKY, 27 October 2003 (IEEE), 309–310. doi:10.1109/ISMAR.2003.1240734
Akhavian, R., and Behzadan, A. H. (2012). An integrated data collection and analysis framework for remote monitoring and planning of construction operations. Adv. Eng. Inform. 26 (4), 749–761. doi:10.1016/j.aei.2012.04.004
Akula, M., Sandur, A., Kamat, V. R., and Prakash, A. (2015). Context-aware framework for highway bridge inspections. J. Comput. Civ. Eng. 29 (1), 04014027. doi:10.1061/(ASCE)CP.1943-5487.0000292
Aoyama, K. (2019). “Novel display using percutaneous electrical stimulation for virtual reality,” in Human interface and the management of information (international conference on human-computer interaction), Orlando, FL, July 26–31, 2019 (New York, NY: Springer), 3–14. doi:10.1007/978-3-030-22649-7_1
Artlantis Studio (n.d.) (2019). iVisit3D. Available at: https://atl.artlantis.com/en/ivisit3d.
Augmented 3D (2020). Available at: https://rb.gy/wbc6vp.
Autodesk (n.d.) (2016). A360. Artlantis Studio. Available at: https://www.autodesk.com/products/a360/overview.
Bae, H., Golparvar-Fard, M., and White, J. (2013). High-precision vision-based mobile augmented reality system for context-aware architectural, engineering, construction and facility management (AEC/FM) applications. Visual. Eng. 1 (1), 3. doi:10.1186/2213-7459-1-3
Balali, V. (2015). Image-based recognition, 3D localization, and retro-reflectivity evaluation of high-quantity low-cost roadway assets for enhanced condition assessment. Champaign, IL: University of Illinois at Urbana-Champaign.
Barazzetti, L., and Banfi, F. (2017). “Historic BIM for mobile VR/AR applications,” in Mixed reality and gamification for cultural heritage. New York, NY: Springer International Publishing, 271–290. doi:10.1007/978-3-319-49607-8_10
Bayode, A., van der Poll, J. A., and Ramphal, R. R. (2019). “4th industrial revolution: challenges and opportunities in the south African context,” in 17th JOHANNESBURG Int’l conference on science, engineering, technology and waste management (SETWM-19), Johannesburg, South Africa, November 18–19, 2019 (Potchefstroom, South Africa: North West University), 341–347. doi:10.17758/EARES8.EAP1119285
Behzadan, A. H. (2008). ARVISCOPE: georeferenced visualization of dynamic construction processes in three-dimensional outdoor Augmented Reality. PhD thesis. Ann Arbor (MI): University of Michigan.
Behzadan, A. H., and Kamat, V. R. (2009a). Automated generation of operations level construction animations in outdoor Augmented Reality. J. Comput. Civ. Eng. 23 (6), 405–417. doi:10.1061/(ASCE)0887-3801200923
Behzadan, A. H., and Kamat, V. R. (2009b). Interactive augmented reality visualization for improved damage prevention and maintenance of underground infrastructure. Construct. Res. Cong. Build. a Sustain. Fut., 1214–1222. doi:10.1061/41020(339)123
Behzadan, A. H., and Kamat, V. R. (2011). Integrated information modeling and visual simulation of engineering operations using dynamic augmented reality scene graphs. J. Inf. Tech. Construction (ITcon) 16 (17), 259–278.
Behzadan, A. H., and Kamat, V. R. (2005). “Visualization of construction graphics in outdoor augmented reality,” in Proceedings of the winter simulation conference 2005. Orlando, FL, 23 January 2006 (IEEE), 1914–1920. doi:10.1109/WSC.2005.1574469
Behzadan, A. H., Timm, B. W., and Kamat, V. R. (2008). General-purpose modular hardware and software framework for mobile outdoor augmented reality applications in engineering. Adv. Eng. Inform. 22 (1), 90–105. doi:10.1016/j.aei.2007.08.005
Berryman, D. R. (2012). Augmented reality: a review. Med. Ref. Serv. Q. 31 (2), 212–218. doi:10.1080/02763869.2012.670604
Bobylev, N. (2009). Mainstreaming sustainable development into a city's Master plan: a case of Urban Underground Space use. Land Use Policy 26 (4), 1128–1137. doi:10.1016/j.landusepol.2009.02.003
Bruno, S., De Fino, M., and Fatiguso, F. (2018). Historic building information modelling: performance assessment for diagnosis-aided information modelling and management. Autom.Constr. 86, 256–276. doi:10.1016/j.autcon.2017.11.009
Carmigniani, J., Furht, B., Anisetti, M., Ceravolo, P., Damiani, E., and Ivkovic, M. (2011). Augmented reality technologies, systems and applications. Multimed Tools Appl. 51 (1), 341–377. doi:10.1007/s11042-010-0660-6
Casas, J. R., and Moughty, J. J. (2017). Bridge damage detection based on vibration data: past and new developments. Front. Built Environ. 3, 4. doi:10.3389/fbuil.2017.00004
Cattari, N., Cutolo, F., D’amato, R., Fontana, U., and Ferrari, V. (2019). “Toed-in vs parallel displays in video see-through head-mounted displays for close-up view,” in IEEE Access, Pisa, PI, October 31, 2019 (IEEE), 159698–159711.
Chen, K., Lu, W., Peng, Y., Rowlinson, S., and Huang, G. Q. (2015). Bridging BIM and building: from a literature review to an integrated conceptual framework. Int. J. Project Manage. 33 (6), 1405–1416. doi:10.1016/j.ijproman.2015.03.006
Chi, H.-L., Chen, Y.-C., Kang, S.-C., and Hsieh, S.-H. (2012). Development of user interface for tele-operated cranes. Adv. Eng. Inform. 26 (3), 641–652. doi:10.1016/j.aei.2012.05.001
Côté, S., Trudel, P., Snyder, R., and Gervais, R. (2013). “An augmented reality tool for facilitating on-site interpretation of 2D construction drawings,” in Proceedings of the 13th international conference on construction applications of virtual reality. London, United Kingdom, October 30–31, 2013 (CONVR), 316–323.
Dai, F., Lu, M., and Kamat, V. R. (2011). Analytical approach to augmenting site photos with 3D graphics of underground infrastructure in construction engineering applications. J. Comput. Civ. Eng. 25 (1), 66–74. doi:10.1061/(ASCE)CP.1943-5487.0000072
Dang, N.-S., Kang, H.-R., Lon, S., and Shim, C.-S. (2018). “3D digital twin models for bridge maintenance,” in Proceedings of 10th international conference on short and medium span bridges, Quebec,QC, July 13–August 3, 2003 73, 1–9.
Dang, N., and Shim, C. (2020). BIM-based innovative bridge maintenance system using augmented reality technology. CIGOS 2019 Innov. Sustain. Infrastruct. 54, 1217–1222. doi:10.1007/978-981-15-0802-8_195
Diao, P.-H., and Shih, N.-J. (2019). Trends and research issues of augmented reality studies in architectural and civil engineering education-A review of academic journal publications. Appl. Sci. 9 (9), 1840. doi:10.3390/app9091840
Dong, S., Behzadan, A. H., Chen, F., and Kamat, V. R. (2013). Collaborative visualization of engineering processes using tabletop augmented reality. Adv. Eng. Softw. 55, 45–55. doi:10.1016/j.advengsoft.2012.09.001
Dong, S., and Kamat, V. R. (2010). “Robust mobile computing framework for visualization of simulated processes in augmented reality,” in Proceedings of the 2010 winter simulation conference, Baltimore, MD, December 5–8, 2010 (IEEE), 3111–3122. doi:10.1109/WSC.2010.5679004
Dunston, P. S., and Wang, X. (2011). An iterative methodology for mapping mixed reality technologies to AEC operations. J. Inf. Tech. Constr. (ITcon) 16 (30), 509–528.
Dunston, P. S., and Wang, X. (2005). Mixed reality-based visualization interfaces for architecture, engineering, and construction industry. J. Constr. Eng. Manage. 131 (12), 1301–1309. doi:10.1061/(asce)0733-9364(2005)131:12(1301)
Ergün, O., Akın, Ş., Dino, İ. G., and Surer, E. (2019). “Architectural design in virtual reality and mixed reality environments: a comparative analysis,” in IEEE Conference on Virtual Reality and 3D User Interfaces (VR), Osaka, OX, March 23–27, 2019 (IEEE), doi:10.1109/VR.2019.8798180
Fathi, H., and Brilakis, I. (2016). Multistep explicit stereo camera calibration approach to improve euclidean accuracy of large-scale 3D reconstruction. J. Comput. Civ. Eng. 30 (1), 04014120. doi:10.1061/(asce)cp.1943-5487.0000454
Feeman, S. M., Wright, L. B., and Salmon, J. L. (2018). Exploration and evaluation of CAD modeling in virtual reality. Computer-Aided Des. Appl. 15 (6), 892–904. doi:10.1080/16864360.2018.1462570
Feng, C. (2015). Camera marker networks for pose estimation and scene understanding in construction automation and robotics. PhD thesis. Ann Arbor (MI): University of Michigan.
Gang, S.-M., Choi, H.-W., Kim, D.-R., and Choung, Y.-J. (2016). A study on the construction of the unity 3D engine based on the webGIS system for the hydrological and water hazard information display. Proced. Eng. 154, 138–145. doi:10.1016/j.proeng.2016.07.431
GhaffarianHoseini, A., Zhang, T., Nwadigo, O., GhaffarianHoseini, A., Naismith, N., Tookey, J., et al. (2017). Application of nD BIM integrated knowledge-based building management system (BIM-IKBMS) for inspecting postconstruction energy efficiency. Renew. Sustain. Energ. Rev. 72, 935–949. doi:10.1016/j.rser.2016.12.061
Giang, N. K., Lea, R., Blackstock, M., and Leung, V. C. M. (2016). “On building smart city IoT applications: a coordination-based perspective,” in Proceedings of the 2nd international workshop on smart, Vancouver,BC, December 2016 (ACM), 1–6. doi:10.1145/3009912.3009919
Golparvar-Fard, M., Balali, V., and De La Garza, J. M. (2015). Segmentation and recognition of highway assets using image-based 3D point clouds and semantic texton forests. J. Comput. Civil Eng. 29 (1), 04014023. doi:10.1061/(ASCE)CP.1943-5487.0000283
Golparvar-Fard, M., Bohn, J., Teizer, J., Savarese, S., and Peña-Mora, F. (2011a). Evaluation of image-based modeling and laser scanning accuracy for emerging automated performance monitoring techniques. Autom. Constr. 20 (8), 1143–1155. doi:10.1016/j.autcon.2011.04.016
Golparvar-Fard, M., Peña-Mora, F., and Savarese, S. (2011b). Integrated sequential as-built and as-planned representation with D4AR tools in support of decision-making tasks in the AEC/FM industry. J. Constr. Eng. Manage. 137 (12), 1099–1116. doi:10.1061/(ASCE)CO.1943-7862.0000371
Golparvar-Fard, M., and Ham, Y. (2014). Automated diagnostics and visualization of potential energy performance problems in existing buildings using energy performance augmented reality models. J. Comput. Civ. Eng. 28 (1), 17–29. doi:10.1061/(asce)cp.1943-5487.0000311
Golparvar-Fard, M., Peña-Mora, F., Arboleda, C. A., and Lee, S. (2009a). Visualization of construction progress monitoring with 4D simulation model overlaid on time-lapsed photographs. J. Comput. Civ. Eng. 23 (6), 391–404. doi:10.1061/(asce)0887-3801(2009)23:6(391)
Golparvar-Fard, M., Peña-Mora, F., and Savarese, S. (2009b). D4AR–a 4-dimensional augmented reality model for automating construction progress monitoring data collection, processing and communication. J. Inf. Tech. Constr. (ITcon) 14 (13), 129–153.
Graphisoft (2011). BIMx. Available at: https://en.wikipedia.org/wiki/BIMx.
Ham, Y., and Golparvar-Fard, M. (2012). “Rapid 3D energy performance modeling of existing buildings using thermal and digital imagery,” in Construction challenges in a flat world:construction research congress proceedings of the 2012 construction Research congress, West Lafayette, IN, May 21–23, 2012 (ASCE), 991–1000. doi:10.1061/9780784412329.100
Hammad, A., Garrett, J. H., and Karimi, H. A. (2002). “Potential of mobile augmented reality for infrastructure field tasks,” in Applications of advanced technologies in transportation, Cambridge, MA, August 5–7, 2002 (ASCE), 425–432. doi:10.1061/40632(245)54
Hammad, A., Wang, H., and Mudur, S. P. (2009). Distributed augmented reality for visualizing collaborative construction tasks. J. Comput. Civ. Eng. 23 (6), 418–427. doi:10.1061/(ASCE)0887-3801200923
Holynski, A., and Kopf, J. (2018). Fast depth densification for occlusion-aware augmented reality. ACM Trans. Graphics 37, 1–11. doi:10.1145/3272127.3275083
Hossain, M., Hanson, J.-W., and Moreu, F. (2020). Real-time theoretical and experimental dynamic mode shapes for structural analysis using augmented reality. Top. Modal Anal. Test., 351–356. doi:10.1007/978-3-030-47717-2_36
Hwang, B.-G., Zhao, X., and Yang, K. W. (2019). Effect of BIM on rework in construction projects in Singapore: status quo, magnitude, impact, and strategies. J. Constr. Eng. Manage. 145 (2), 04018125. doi:10.1061/(ASCE)CO.1943-7862.0001600
Jahn, G., Newnham, C., van den Berg, N., and Beanland, M. (2018). “Making in mixed reality,” in ACADIA 2018 Proceedings of the 38th Annual Conference of the Association for Computer Aided Design in Architecture (ACADIA), Mexico, MX, October 18–20, 2018, 88–97. Available at: http://papers.cumincad.org/cgi-bin/works/BrowseTreefield=seriesorder=AZ/Show?acadia18_88.
Jeanclos, N., Sharif, M.-M., Li, S. K., Kwiatek, C., and Haas, C. (2018). “Derivation of minimum required model for augmented reality based stepwise construction assembly control,” in Workshop of the european group for intelligent computing in engineering, Waterloo,ON, May19, 2018 (Springer), 336–358. doi:10.1007/978-3-319-91635-4_18
Julier, S., Baillot, Y., Lanzagorta, M., Brown, D., and Rosenblum, L. (2000). “Bars: battlefield augmented reality system,” in NATO symposium on information processing techniques for military systems. Washington, DC, Available at: http://citeseerx.ist.psu.edu/viewdoc/summary?doi=10.1.1.1.5064
Kaddioui, A., Shahrour, I., and El Oirrak, A. (2019). “Uses of augmented reality for urban utilities management,” in MATEC Web of Conference, Lille,NO, January 2019, 295, 02009. doi:10.1051/matecconf/201929502009
Kamat, V. R., and El-Tawil, S. (2007). Evaluation of augmented reality for rapid assessment of earthquake-induced building damage. J. Comput. Civ. Eng. 21 (5), 303–310 . doi:10.1061/(ASCE)0887-3801200721
Kamat, V. R., Martinez, J. C., Fischer, M., Golparvar-Fard, M., Peña-Mora, F., and Savarese, S. (2011). Research in visualization techniques for field construction. J. Constr. Eng. Manage. 137 (10), 853–862. doi:10.1061/(ASCE)CO.1943-7862.0000262
Kanbara, M., Okuma, T., Takemura, H., and Yokoya, N. (2000). “A stereoscopic video see-through augmented reality system based on real-time vision-based registration,” in Proceedings IEEE Virtual Reality, 255–262. doi:10.1109/vr.2000.840506
Karsch, K., Golparvar-Fard, M., and Forsyth, D. (2014). ConstructAide: analyzing and visualizing construction sites through photographs and building models. ACM Trans. Graph. 33 (6), 1–11. doi:10.1145/2661229.2661256
Kim, K., Kim, H., and Kim, H. (2017). Image-based construction hazard avoidance system using augmented reality in wearable device. Autom. Constr. 83, 390–403. doi:10.1016/j.autcon.2017.06.014
Kim, M.-K., Cheng, J. C. P., Sohn, H., and Chang, C.-C. (2015). A framework for dimensional and surface quality assessment of precast concrete elements using BIM and 3D laser scanning. Autom. Const. 49, 225–238. doi:10.1016/j.autcon.2014.07.010
Kiyokawa, K., Billinghurst, M., Campbell, B., and Woods, E. (2003). “An occlusion capable optical see-through head mount display for supporting co-located collaboration,” in The Second IEEE and ACM International Symposium on Mixed and Augmented Reality (Institute of Electrical and Electronics Engineers Inc.), 133–141. doi:10.1109/ISMAR.2003.1240696
Kopsida, M., and Brilakis, I. (2017). “BIM registration methods for mobile augmented reality-based inspection,” in EWork and EBusiness in architecture, engineering and construction: ECPPM 2016: proceedings of the 11th European conference on product and process modelling (ECPPM 2016), Cambridge, United Kingdom, September 21, 2016, 7–9.
Kwiatek, C., Li, S. K., Sharif, M. M., Jeanclos, N., Haas, C. T., and Walbridge, S. (2018). “Facilitating the communication of rework information to craft workers using an augmented reality process,” in ISARC 2018-35th international symposium on automation and robotics in construction and international AEC/FM hackathon: the future of building things, Waterloo, ON, July 12, 2018. 1–8. doi:10.22260/isarc2018/0071
Kwiatek, C., Sharif, M., Li, S., Haas, C., and Walbridge, S. (2019). Impact of augmented reality and spatial cognition on assembly in construction. Autom.in Constr. 108, 102935. doi:10.1016/j.autcon.2019.102935
Kyriazis, D., Varvarigou, T., White, D., Rossi, A., and Cooper, J. (2013). “Sustainable smart city IoT applications: heat and electricity management & eco-conscious cruise control for public transportation,” in 2013 IEEE 14th International Symposium on" A World of Wireless, Mobile and Multimedia Networks (WoWMoM), Madird. MA, June 4–7, 2013 (IEEE),
Lattanzi, D., Miller, G. R., Eberhard, M. O., and Haraldsson, O. S. (2016). Bridge column maximum drift estimation via computer vision. J. Comput. Civ. Eng. 30 (4), 04015051. doi:10.1061/(ASCE)CP.1943-5487.0000527
Lee, G. A., Dunser, A., Kim, S., and Billinghurst, M. (2012). “CityViewAR: a mobile outdoor AR application for city visualization,” in 2012 IEEE international symposium on mixed and augmented reality-arts. Media, and Humanities (ISMAR-AMH), Atlanta, GA, November 5–8 2012 (IEEE), 57–64. doi:10.1109/ISMAR-AMH.2012.6483989
Li, S., Cai, H., and Kamat, V. R. (2015). Uncertainty-aware geospatial system for mapping and visualizing underground utilities. Autom. Constr. 53, 105–119. doi:10.1016/j.autcon.2015.03.011
Li, X., Yi, W., Chi, H.-L., Wang, X., and Chan, A. P. C. (2018). A critical review of virtual and augmented reality (VR/AR) applications in construction safety. Autom. Constr. 86, 150–162. doi:10.1016/j.autcon.2017.11.003
Liu, C.-W., Wu, T.-H., Tsai, M.-H., and Kang, S.-C. (2018). Image-based semantic construction reconstruction. Autom. Constr. 90, 67–78. doi:10.1016/j.autcon.2018.02.016
Liu, F., and Seipel, S. (2015). Infrared-visible image registration for augmented reality-based thermographic building diagnostics. Visualization Eng. 3 (1), 16. doi:10.1186/s40327-015-0028-0
Machado, R. L., and Vilela, C. (2020). Conceptual framework for integrating BIM and augmented reality in construction management. J. Civil Eng. Manage. 26 (1), 83–94. doi:10.3846/jcem.2020.11803
Maharjan, D., Aguero, M., Lippitt, C., and Moreu, F. (2019). Infrastructure stakeholders’ perspective in development and implementation of new structural health monitoring (SHM) technologies for maintenance and management of transportation infrastructure. MATEC web of conferences 271, 01010. doi:10.1051/matecconf/201927101010
Mahmood, A., Butler, B., Zen, H., and Jennings, B. (2018). “Key early verticals, challenges and limitations in implementation of augmented reality,” in Encyclopedia of computer graphics and games (Berlin, Germany: Springer).
Malkawi, A. M., and Srinivasan, R. S. (2005). A new paradigm for human-building interaction: the use of CFD and augmented reality. Autom. Constr. 14 (1), 71–84. doi:10.1016/j.autcon.2004.08.001
Manuel Davila Delgado, J., Oyedele, L., Beach, T., and Demian, P. (2020). Augmented and virtual reality in construction: drivers and limitations for industry adoption. Loughborough, United: American Society of Civil Engineers.
Meža, S., Turk, Ž., and Dolenc, M. (2014). Component based engineering of a mobile BIM-based augmented reality system. Autom. Constr. 42, 1–12. doi:10.1016/j.autcon.2014.02.011
Microsoft (2019). Environment considerations for HoloLens. Available at: https://rb.gy/kb5xay.
Milgram, P., and Kishino, F. (1994a). “A taxonomy of mixed reality visual displays. IEICE Transact. Inform. Sys. E77-D (12), 1–15.
Milgram, P., and Kishino, F. (1994b). “A taxonomy of mixed reality visual displays. IEICE Transact. Inform. Sys. 77 (12), 1321–1329.
More Ickson, M., and ben Dhaou, S. (2019). “Responding to the challenges and opportunities in the 4 th Industrial revolution in developing countries,” in Proceedings of the 12th international conference on theory and practice of electronic governance, Melbourne, Australia, Feburary 22, 2019, 244–253. doi:10.1145/3326365.3326398
Moreu, F. (2015). Framework for risk-based management and safety of railroad bridge infrastructure using wireless smart sensors (WSS). Champaign, IL: University of Illinois at Urbana-Champaign.
Nahangi, M. (2015). Development of transformations between designed and built structural systems and pipe assemblies. Waterloo, ON: University of Waterloo.
Nahangi, M., Yeung, J., Haas, C. T., Walbridge, S., and West, J. (2015). Automated assembly discrepancy feedback using 3D imaging and forward kinematics. Autom. Const. 56, 36–46. doi:10.1016/j.autcon.2015.04.005
Nahon, D., Subileau, G., and Capel, B. (2015). “Never Blind VR” enhancing the virtual reality headset experience with augmented virtuality,” in 2015 IEEE Virtual RealityVR, Arles, FC, March 23–27 2015, 347–348.
Napolitano, R., Liu, Z., Sun, C., and Glisic, B. (2019). Combination of image-based documentation and augmented reality for structural health monitoring and building pathology. Front. Built Environ. 5, 1–14. doi:10.3389/fbuil.2019.00050
NOAA (2015). NOAA satellite and information service deep space climate observatory (DSCOVR), Silver Spring, MD: NOAA
Ohta, Y., and Tamura, H. (2014). Mixed reality: merging real and virtual worlds. Berlin, Germany: Springer Publishing Company
Ortega, S., Wendel, J., Santana, J., Murshed, S., Boates, I., Trujillo, A., et al. (2019). Making the invisible visible-strategies for visualizing underground infrastructures in immersive environments. IJGI 8 (3), 152–173. doi:10.3390/ijgi8030152
Pereira, M., Burns, D., Orfeo, D., Farrel, R., Huston, D. R., and Xia, T. (2018). “New GPR system integration with augmented reality based positioning,” in Proceedings of the 2018 on great lakes symposium on VLSI, Boston,MA, May 2018 (ACM), 341–346. doi:10.1145/3194554.3194623
Pereira, M., Burns, D., Orfeo, D., Zhang, Y., Jiao, L., Huston, D., et al. (2020). “3-D multistatic ground penetrating radar imaging for augmented reality visualization,” in IEEE transactions on geoscience and remote sensing, Princeton, PU, February 6, 2020, 5666–5675.
Pereira, M., Orfeo, D., Ezequelle, W., Burns, D., Xia, T., and Huston, D. R. (2019). “Photogrammetry and augmented reality for underground infrastructure sensing, mapping and assessment,” in International conference on smart infrastructure and construction 2019 (ICSIC) driving data-informed decision-making, London, United Kingdom, July 5, 2019 (ICE), 169–175. doi:10.1680/icsic.64669.169
Peres, F. F. F., Scheer, S., and Faria, É. F. d. (2018). A taxonomy of tasks in dam cracks surveillance for augmented reality application. IJAERS 5 (10), 179–184. doi:10.22161/ijaers.5.10.24
Petrillo, A., De Felice, F., Cioffi, R., and Zomparelli, F. (2018). “Fourth industrial revolution: current practices, challenges, and opportunities,” in Digital transformation in smart manufacturing. Editors R. Cioffi, F. De Felice, and A. PetrilloSoskice (London, United Kingdom: IntechOpen), 1–20.
Pitsis, T. S., Beckman, S. L., Steinert, M., Oviedo, L., and Maisch, B. (2020). Designing the future: strategy, design, and the 4th industrial revolution-An introduction to the special issue. Calif. Manage. Rev. 62 (2), 5–11. doi:10.1177/0008125620907163
Pokric, B., Krco, S., and Pokric, M. (2014). “Augmented reality based smart city services using secure IoT infrastructure,” in 2014 28th international conference on advanced information networking and applications workshops. Victoria, BC, May 13–16, 2014 (IEEE), 803–808. doi:10.1109/WAINA.2014.127
Poslad, S., Ma, A., Wang, Z., and Mei, H. (2015). Using a smart city IoT to incentivise and target shifts in mobility behaviour-Is it a piece of pie? Sensors 15 (6), 13069–13096. doi:10.3390/s150613069
Rahimian, F. P., Seyedzadeh, S., Oliver, S., Rodriguez, S., and Dawood, N. (2020). On-demand monitoring of construction projects through a game-like hybrid application of BIM and machine learning. Autom. Constr. 110, 103012. doi:10.1016/j.autcon.2019.103012
Roberts, G. W., Evans, A., Dodson, A. H., Denby, B., Cooper, S., and Hollands, R. (2002). “The use of augmented reality, GPS and INS for subsurface data visualization,” in FIG XXII international congress, Washington, DC, April 19–26, 2002, 1–12.
Roitman, L., Shrager, J., and Winograd, T. (2017). A comparative analysis of Augmented Reality technologies and their marketability in the consumer electronics segment. J. Biosens. Bioelectron. 08 (1), 1000236. doi:10.4172/2155-6210.1000236
Sacks, R., Perlman, A., and Barak, R. (2013). Construction safety training using immersive virtual reality. Constr. Manage. Econ. 31 (9), 1005–1017. doi:10.1080/01446193.2013.828844
Sampaio, A. Z., and Martins, O. P. (2014). The application of virtual reality technology in the construction of bridge: the cantilever and incremental launching methods. Autom. Constr. 37, 58–67. doi:10.1016/j.autcon.2013.10.015
Santos, R., Costa, A. A., and Grilo, A. (2017). Bibliometric analysis and review of building information modelling literature published between 2005 and 2015. Autom. Constr. 80, 118–136. doi:10.1016/j.autcon.2017.03.005
Schall, G., Mendez, E., Kruijff, E., Veas, E., Junghanns, S., Reitinger, B., et al. (2009). Handheld augmented reality for underground infrastructure visualization. Pers Ubiquit Comput. 13 (4), 281–291. doi:10.1007/s00779-008-02045
Schall, G., Schmalstieg, D., and Junghanns, S. (2010). “VIDENTE-3D visualization of underground infrastructure using handheld augmented reality,” in Geohydroinformatics: integrating GIS and water engineering (Berlin, Germany: Springer).
Schickert, M., Koch, C., and Bonitz, F. (2018). “Prospects for integrating augmented reality visualization of nondestructive testing results into model-based infrastructure inspection,” in NDE/NDT for Highways and Bridges SMT 2018, Brazil, August 27, 2018.
Shin, D. H., and Dunston, P. S. (2008). Identification of application areas for augmented reality in industrial construction based on technology suitability. Autom. Const. 17 (7), 882–894. doi:10.1016/j.autcon.2008.02.012
Soman, R. K., Birch, D., and Whyte, J. K. (2017). “Framework for shared visualization and real-time information flow to the construction site,” in 24th International Workshop on Intelligent Computing in Engineering, London, United Kingdom, July 12, 2017. doi:10.24928/jc3-2017/0273
Son, H., Bosché, F., and Kim, C. (2015). As-built data acquisition and its use in production monitoring and automated layout of civil infrastructure: a survey. Adv. Eng. Inform. 29 (2), 172–183. doi:10.1016/j.aei.2015.01.009
Speicher, M., Hall, B. D., and Nebeling, M. (2019). “What is mixed reality?,” in Proceedings of the 2019 CHI conference on human factors in computing systems, Ann Arbor, MI, May 17, 2019, 15, 1–15. doi:10.1145/3290605.3300767
Tang, S., Tang, L., Wu, Z., Zheng, W., and Chen, C. (2016). “A conceptual workflow for BIM based prefabrication design visualization with augmented reality,” in Proceedings of the 16th international conference on construction applications of virtual reality, Hong Kong,HK, December 2016, 376–384.
Tedeschi, A., and Benedetto, F. (2017). A real-time automatic pavement crack and pothole recognition system for mobile Android-based devices. Adv. Eng. Inform. 32, 11–25. doi:10.1016/j.aei.2016.12.004
Ternier, S., Klemke, R., Kalz, M., van Ulzen, P., and Specht, M. (2012). ARLearn: augmented reality meets augmented virtuality. J. Univ. Comput. Sci. 18 (15), 2143–2164.
The Mixed Reality Toolkit (2020). Available at: https://rb.gy/93r4nf.
Thomas, B., Piekarski, W., and Gunther, B. (1999). “Using augmented reality to visualise architecture designs in an outdoor environment,” in International Journal of Design Computing Special Issue on Design Computing on the Net (DCNet), Mawson Lakes, SA 1 (4), 2.
Thompson, C. (2019). Inventor Alex Kipman’s grand vision for how holograms will change our lives. Washington, DC: Smithsonian MagazineAvailable at: https://www.smithsonianmag.com/innovation/alex-kipman-grand-visions-how-holograms-change-lives-180973489/.
Unity (2021). Unity user manual (2019.4 LTS). Available at: https://docs.unity3d.com/Manual/index.html
VTT (2020). Building and infrastructure. Available at: https://rb.gy/2itv2g.
Wang, C., Cho, Y. K., and Gai, M. (2013a). As-is 3D thermal modeling for existing building envelopes using a hybrid LIDAR system. J. Comput. Civil Eng. 27 (6), 645–656. doi:10.1061/(ASCE)CP.1943-5487.0000273
Wang, J., Hou, L., Wang, Y., Wang, X., and Simpson, I. (2015). “Integrating augmented reality into building information modeling for facility management case studies,” in Building information modeling: applications and practices (Reston, Virginia: American Society of Civil Engineers (ASCE)). 279–304. doi:10.1061/9780784413982
Wang, X. (2009). Augmented reality in architecture and design: potentials and challenges for application. Int. J. Archit. Comput. 7 (2), 309–326. doi:10.1260/147807709788921985
Wang, X., and Dunston, P. S. (2006). Compatibility issues in augmented reality systems for AEC: an experimental prototype study. Autom. Constr. 15 (3), 314–326. doi:10.1016/j.autcon.2005.06.002
Wang, X., and Dunston, P. S. (2013). Tangible mixed reality for remote design review: a study understanding user perception and acceptance. Visual. Eng. 1 (1), 8. doi:10.1186/2213-7459-1-8
Wang, X., and Dunston, P. S. (2008). User perspectives on mixed reality tabletop visualization for face-to-face collaborative design review. Autom. Constr. 17 (4), 399–412. doi:10.1016/j.autcon.2007.07.002
Wang, X., Kim, M. J., Love, P. E. D., and Kang, S. C. (2013b). Augmented reality in built environment: classification and implications for future research. Autom.Constr. 32, 1–13. doi:10.1016/j.autcon.2012.11.021
Web of Science (2020). Available at: https://rb.gy/va0yqz.
Webster, A., Feiner, S., MacIntyre, B., Massie, W., and Krueger, T. (1996). Augmented reality in architectural construction, inspection, and renovation. Proc. ASCE Third Congress on Computing in Civil Engineering (New York, NY), 913–919.
Wen, M. C., and Kang, S. C. (2014). Augmented reality and unmanned aerial vehicle assist in construction management. In Proc. ASCE Third Congress on Computing in Civil Engineering 1, 1570–1577.
Wu, L. (2015). Applications of computer vision technologies of automated crack detection and quantification for the inspection of civil infrastructure systems. Orlando, FL: University of Central Florida.
Xu, J., Doyle, D., and Moreu, F. (2020). State of the art of augmented reality (AR) capabilities for civil infrastructure applications (in submission). Autom. Const. 1 (3), 17,
Xu, S., Wang, J., Shou, W., Ngo, T., Sadick, A. M., and Wang, X. (2020). Computer vision techniques in construction: a critical review. Arch. Comput. Methods Eng. 1, 3. doi:10.1007/s11831-020-09504-3
Yabuki, N., Miyashita, K., and Fukuda, T. (2011). An invisible height evaluation system for building height regulation to preserve good landscapes using augmented reality. Autom. Constr. 20 (3), 228–235. doi:10.1016/j.autcon.2010.08.003
Yamaguchi, T., Kanda, M., Shibuya, T., and Yasojima, A. (2019). “Crack inspection support system for concrete structures using head mounted display in mixed reality space,” in 2019 58th Annual Conference of the Society of Instrument and Control Engineers of Japan (SICE). Hiroshima, OA, September 10–13, 2019 (IEEE). 791–796. doi:10.23919/SICE.2019.8859876
Yan, L., Fukuda, T., and Yabuki, N. (2019). Integrating UAV development technology with augmented reality toward landscape tele-simulation.
Zhang, Y., Zhang, H., and Chen, X. (2020). Augmented reality technology: research situation and key technologies. Lecture Notes Electr. Eng. 588, 986–1004. doi:10.1007/978-981-32-9437-0_102
Zhao, D., and Lucas, J. (2015). “Virtual reality simulation for construction safety promotion,” in Int. J. Inj. Cont. Saf. Promot. 22 (1), 57–67. doi:10.1080/17457300.2013.861853
Zhou, Y., Luo, H., and Yang, Y. (2017). Implementation of augmented reality for segment displacement inspection during tunneling construction. Autom. Constr. 82, 112–121. doi:10.1016/j.autcon.2017.02.007
Zollmann, S., Hoppe, C., Kluckner, S., Poglitsch, C., and Bischof, H. (2014). “Augmented reality for construction site monitoring and documentation,” in Proceedings of the IEEE, Graz, AT, January20, 2014 (IEEE), 137–154.
Zollmann, S., Kalkofen, D., Hoppe, C., Kluckner, S., Bischof, H., and Reitmayr, G. (2012). “Interactive 4D overview and detail visualization in augmented reality,” in 2012 IEEE international symposium on mixed and augmented reality (ISMAR), Atlanta, GA, November 5–8, 2012 (IEEE).
Keywords: augmented reality, civil infrastructure, construction, building information models, structural health monitoring
Citation: Xu J and Moreu F (2021) A Review of Augmented Reality Applications in Civil Infrastructure During the 4th Industrial Revolution. Front. Built Environ. 7:640732. doi: 10.3389/fbuil.2021.640732
Received: 12 December 2020; Accepted: 17 February 2021;
Published: 02 June 2021.
Edited by:
Branko Glisic, Princeton University, United StatesReviewed by:
David Lattanzi, George Mason University, United StatesRebecca Napolitano, Pennsylvania State University (PSU), United States
Dryver R Huston, University of Vermont, United States
Copyright © 2021 Xu and Moreu. This is an open-access article distributed under the terms of the Creative Commons Attribution License (CC BY). The use, distribution or reproduction in other forums is permitted, provided the original author(s) and the copyright owner(s) are credited and that the original publication in this journal is cited, in accordance with accepted academic practice. No use, distribution or reproduction is permitted which does not comply with these terms.
*Correspondence: Fernando Moreu, Zm1vcmV1QHVubS5lZHU=