How Reliable are 11- to 13-Year-Olds’ Self-Ratings of Effort in Noisy Conditions?
- Department of Engineering, University of Ferrara, Ferrara, Italy
Performing a task in noisy conditions is effortful. This is especially relevant for children in classrooms as the effort involved could impair their learning and academic achievements. Numerous studies have investigated how to use behavioral and physiological methods to measure effort, but limited data are available on how well school-aged children rate effort in their classrooms. This study examines whether and how self-ratings can be used to describe the effort children perceive while working in a noisy classroom. This is done by assessing the effect of listening condition on self-rated effort in a group of 182 children 11–13 years old. The children performed three tasks typical of daily classroom activities (speech perception, sentence comprehension, and mental calculation) in three listening conditions (quiet, traffic noise, and classroom noise). After completing each task, they rated their perceived task-related effort on a five-point scale. Their task accuracy and response times (RTs) were recorded (the latter as a behavioral measure of task-related effort). Participants scored higher (more effort) on their self-ratings in the noisy conditions than in quiet. Their self-ratings were also sensitive to the type of background noise, but only for the speech perception task, suggesting that children might not be fully aware of the disruptive effect of background noise. A repeated-measures correlation analysis was run to explore the possible relationship between the three study outcomes (accuracy, self-ratings, and RTs). Self-ratings correlated with accuracy (in all tasks) and with RTs (only in the speech perception task), suggesting that the relationship between different measures of listening effort might depend on the task. Overall, the present findings indicate that self-reports could be useful for measuring changes in school-aged children’s perceived listening effort. More research is needed to better understand, and consequently manage, the individual factors that might affect children’s self-ratings (e.g., motivation) and to devise an appropriate response format.
Introduction
Performing a listening task in adverse acoustic conditions demands a greater effort (Peelle, 2018). Speech signals can be degraded by a variety of factors, which can be categorized as listener-external (e.g., level and type of background noise, excessively long reverberation) or listener-internal (individual characteristics of auditory, linguistic, and cognitive processing) (Mattys et al., 2012; Lemke and Besser, 2016). Understanding speech in noise requires an explicit engagement of extra cognitive resources.
Effort has been defined as “the deliberate allocation of mental resources to overcome obstacles in goal pursuit when carrying out a task, with listening effort applying more specifically when tasks involve listening” (Pichora-Fuller et al., 2016). According to the Ease of Language Understanding model (ELU; Rönnberg et al., 2008; Rönnberg et al., 2013), listening to speech in ideal conditions is quick and easy, relying primarily on implicit processing. In unfavorable conditions, an explicit processing becomes necessary, posing greater cognitive demands, which the listener perceives as an increase in the effort involved. As explained in the Framework for Understanding Effortful Listening (FUEL; Pichora-Fuller et al., 2016), listening effort is also modulated by a listener’s motivation, or “the resources or energy actually used by a listener to meet the cognitive demands” (Peelle, 2018). The stronger a listener’s motivation, the more willing they will be to put effort into the task, regardless of its demands.
The concept of effort is especially relevant for children in classrooms, as greater cognitive demands related to listening could interfere with their ability to complete high-level cognitive tasks (e.g., comprehension; McGarrigle et al., 2019). Classrooms are usually far from optimal listening environments, with background noise levels that exceed the recommended normative values (Shield and Dockrell, 2008; Mealings, 2016) and with excessively long reverberation times. Research has also shown that elementary school children (from kindergarten up to grade 8) spend almost 90% of their time at school listening to speech in the presence of background noise (Crukley et al., 2011).
Previous studies found evidence of school-age children having to put more effort into listening tasks in background noise than in quiet, or when the level of background noise increased. This greater effort was revealed by slower response times (Lewis et al., 2016; McGarrigle et al., 2019; Prodi et al., 2019a; Prodi et al., 2019b; Picou et al., 2019), and by a larger task-evoked increase in pupil size (Steel et al., 2015; McGarrigle et al., 2017; Gómez-Merino et al., 2020). The results were not entirely consistent across the studies, however, presumably due to a different sensitivity of the listening effort measures (McGarrigle et al., 2017; McGarrigle et al., 2019), and to the children’s difficulty in preferentially allocating their attention during dual tasks (Choi et al., 2008; Picou et al., 2019).
While numerous studies has investigated the use of behavioral and physiological measures of effort, limited data are available regarding the reliability of school-aged children’s subjective ratings of effort in their classrooms. This seems rather odd because self-reports are the most direct and ecologically valid, non-invasive measures for tapping into the subjective experience of effortful listening. Being easy for participants to understand, and for experimenters to administer, self-reports classify as a potentially good candidate for gauging children’s listening effort.
In the literature, self-reports were obtained mainly by means of study-specific questionnaires and rating scales (Oosthuizen et al., 2021a) developed specifically for a focused assessment on the conditions adopted in a given study (Moore and Picou, 2018). Children were variously asked to rate their perceived effort (von Lochow et al., 2018), ease of listening (Picou et al., 2019; Oosthuizen et al., 2021a), listening difficulty (Prodi et al., 2010), disturbance (Klatte et al., 2010), and perceived clarity (Gustafson et al., 2014). The results indicate that children report perceiving less effort: in quiet than in noisy conditions (von Lochow et al., 2018; Picou et al., 2019); in aided versus unaided conditions (Oosthuizen et al., 2021a); following digital noise reduction in hearing aids (Gustafson et al., 2014); and after increasing the signal-to-noise ratio by 4 dB in the presence of a four-talker babble-noise (Picou et al., 2019).
On the other hand, no significant differences emerged in children’s self-ratings in noisy conditions when the type of background noise was changed (Klatte et al., 2010; von Lochow et al., 2018). Klatte et al. (2010) found no significant effect of the type of background noise (classroom noise, background speech) on first- and third-graders’ disturbance ratings for a speech perception task. In the same listening conditions, adults reported finding classroom noise more disturbing than background speech. In the same study, when the children were administered a listening comprehension task, the results indicated no correlation between their ratings of perceived disturbance and their task performance. Von Lochow et al. (2018) examined how the number of competing speakers (one or four) influenced perceived effort in a passage comprehension task. There was no significant increase in the children’s perceived effort when the number of speakers changed, despite a significant change in their accuracy.
Self-ratings rely on the assumption that listeners can accurately report the effort they experienced (Picou and Ricketts, 2018), but studies on adults indicate that self-ratings rarely correlate with behavioral or physiological measures of effort (Picou and Ricketts, 2018; Strand et al., 2018; Alhanbali et al., 2019; Lau et al., 2019; McGarrigle et al., 2020). Many variables possibly affecting different participants’ performance and self-ratings of effort might obscure any correlation measured across participants, however. On the other hand, self-ratings of effort appear to correlate inversely with self-ratings of performance (Moore and Picou, 2018). Studies in which task difficulty was manipulated (e.g., presence/absence of background noise, a change of SNR) suggest that listeners might become aware of a change in the demands of a task and/or of their own performance, and use this impression as a substitute for judging the effort involved (McGarrigle et al., 2020).
Previous studies on school-age children generally showed no correlation between self-ratings of effort and task performance (von Lochow et al., 2018; Gustafson et al., 2014; Klatte et al., 2010). That said, Picou et al. (2019) examined the relationship between accuracy in a speech perception task, dual-task response time, and four questions related to effort (perceived performance, ease of listening, control, time) in a sample of 10- to 17-year-olds. The results suggested that changing the wording of the question and asking participants to assess attributes more readily-understandable than “listening effort” revealed significant associations between self-ratings and actual performance.
Very few studies have examined the relationship between self-ratings and objective measures of effort in children. Picou et al. (2019) found a significant association between dual-task response times and time perception ratings, but the correlation went in the opposite direction to the one hypothesized. Gustafson et al. (2014) administered a speech perception task to a group of children (7–12 years old) with normal hearing to examine the impact of digital noise reductions in hearing aids. Response times were faster and clarity ratings were higher with the noise reduction algorithm, but there was no significant correlation between the two measures.
All in all, there seems to be a paucity of information available on the reliability of school-aged children’s self-ratings of effort, and their correlation with objective measures. This information would be valuable because reliable subjective ratings of effort would facilitate the assessment of the sound environment in classrooms. A better understanding of how school-age children deal with the demands of listening in challenging acoustic conditions would enable us to promote the design of learning environments with better acoustics.
The purpose of the present study was twofold. The first aim was to explore the sensitivity of self-reports of perceived effort to changes in the demands of a task by taking action on the background noise. Three tasks were considered: 1) a speech perception task; and 2) two tasks highly representative of typical classroom activities, i.e., sentence comprehension and mental calculation. For all three tasks, we expected self-ratings of effort to be higher in noisy than in quiet conditions, reflecting the subjective perception of a greater effort being needed in more adverse listening conditions (von Lochow et al., 2018). The second aim was to examine how subjective measures of effort relate to task performance and response time (RT). In our experiments, RTs (acquired with a single-task paradigm) were used as a behavioral measure of effort. We also planned to see whether the pattern of correlations differed depending on the task.
The results of the present study will add to the current literature on listening effort in children, by:
1) Further exploring the relationship between self-ratings of effort and performance accuracy. This relationship has already been examined in adults, but few reports are currently available regarding children, and their findings are inconsistent (von Lochow et al., 2018; Picou et al., 2019);
2) Newly exploring the specific relationship between self-ratings and RTs in school-aged children.
Materials and Methods
This study is part of a broader research project conceived to investigate the effects of noisy and reverberant classrooms on children’s performance and perceived effort when engaged in linguistic and calculation tasks. The research also considered the potential role of mediating factors (age, gender, noise source, task difficulty, and room acoustics). The material and methods used in this study were consequently the same as those adopted in other, related studies (Prodi et al., 2019a; Caviola et al., 2021; Prodi et al., 2021; Prodi and Visentin, in press). The focus of the present study is very different, however, in that it investigates the effects of listening conditions and age on self-ratings of effort, whereas the above-mentioned studies only concerned behavioral measures of performance and listening effort.
Participants
A total of 182 children between the ages of 11 and 13 years took part in the study. They were from two schools in Ferrara (Italy), and the study involved three classes for each grade (6–8). Six children were excluded from the data analysis due to intellectual disabilities or a diagnosis of hearing impairment. Other children were also excluded because of their maths fluency assessment (n = 14) or their score in the reading comprehension task (n = 6). The final sample included 159 participants for the speech perception and sentence comprehension tasks, and 162 children for the mental calculation task.
The study was approved by the Ethics Committee at the University of Padova (Italy) and by the school management.
Reading Comprehension and Maths Fluency Tests
In addition to the experimental tasks, children were administered a standardized reading comprehension test [derived from Cornoldi et al. (2017)] and a standardized maths fluency test (Caviola et al., 2016). Both tests were administered collectively to the students in their classrooms, in quiet conditions, one week after the experimental tasks. These individual measures were used for data screening purposes: participants obtaining a standardized score lower than −2.5 (reading comprehension) and −3 (maths fluency) were excluded from our analysis. Their individual scores were included in the statistical model as a covariate, to control for the effects of comprehension abilities and maths fluency on children’s perceived effort.
Listening Tasks
Speech Perception Task
The Italian version of the Matrix Sentence Test (Puglisi et al., 2015) was used to measure speech perception. The test consists of sentences with a fixed syntactic structure but no semantic predictability [e.g., Chiara manda sette porte azzurre (Chiara sends seven blue doors)], obtained from a base matrix of 50 words. After listening to a sentence, the children selected the words they had heard from the base-word matrix shown on a tablet. Sixteen trials (sentences) were presented in each listening condition. After the last sentence, the children rated their perceived effort in performing the task.
Sentence Comprehension Task
Sentence comprehension in the auditory modality was measured using the COMPRENDO test (Cecchetto et al., 2012), which consists of sentences of varying syntactic complexity [e.g., La mamma sta inseguendo il bambino (The mother is chasing the child)]. For each trial, children listened to the playback of a sentence. Then, at the audio offset, four images appeared on the tablet and they had to select the image that best matched the sentence content. Sixteen trials were presented in each listening condition. After the last sentence, the children rated their perceived effort.
Mental Calculation Task
The mental calculation task consisted in solving two-digit additions and subtractions, with or without borrowing and carrying procedures (Caviola et al., 2021). For each problem, the children listened to the playback of a female voice posing the problem. Then, they were asked to select the right answer from among the three options presented on the tablet. Twenty-eight trials (problems) were presented in each listening condition. After the last problem, the children rated their perceived effort.
Listening Conditions
Background Noises
Participants completed each task and provided self-ratings for the three background noise conditions (quiet, traffic noise, classroom noise).
In the quiet condition, no noise was played back, and children completed the tasks in the actual ambient noise of their classrooms, mainly consisting of noises coming from other classrooms and corridors. Traffic noise was obtained by obtaining recordings alongside a busy road (with cars and trucks passing by), then applying spectral filtering to correct for the sound insulation properties of a typical building façade. Classroom noise included sound events typical of a working classroom (e.g., pens rolling onto the floor, chairs scraping) which were mixed with a standard noise signal (ICRA noise; Dreschler et al., 2001). The ICRA noise was constructed from Italian sentences, processed to make them unintelligible but still retaining the long-term average spectrum and temporal envelope fluctuations of speech.
Test Environments
The experiments were carried out in two classrooms, one at each school. The two classrooms had a similar volume (152 and 155 m3), size (7.3 x 7.0 x 3.1 and 8.3 x 6.0 x 3.1 m), and reverberation time (after one of the classrooms had been temporarily treated by installing sound-absorbing polyester fiber blankets).
During the tests, speech was presented from a Gras 44AB mouth simulator positioned in front of the teacher’s desk (height: 1.50 m). The background noises were played back with a Look Line D303 omnidirectional source placed on the floor near a corner of the classroom. Audio playback, testing and data collection were managed with a laptop PC running a wireless test bench (Prodi et al., 2012).
For all conditions, the level of the speech signal was set to 63 dB(A), measured at 1 m in front of the speech source. The background noises (traffic noise, classroom noise) were played back at a level of 60 dB(A), measured as the spatial average of four receivers (positioned in the area where children were seated).
Acoustic Measurements
The reverberation time (T20), i.e., the time it takes, after a source of sound in an enclosure has stopped, for the sound to decrease by 60 dB, and the A-weighted equivalent sound pressure levels (LA,eq) were measured in the two classrooms in occupied conditions (Geneva: International Organization for Standardization, 2009). The measurements were obtained at four positions in the part of the classroom where children were seated, using an omnidirectional B&K4189 ½ inch microphone (height: 1.20 m). As the classrooms were small and the distance between the speech source and the listeners was short, equivalent listening conditions were ensured for the various seating positions. Differences between the acoustic parameters measured in the two classrooms were always below the minimum perceivable threshold, so the two classrooms could be considered equivalent in terms of acoustic perception. Table 1 shows the listening conditions in the classrooms during the experiments; for more details, see Prodi et al. (2019a).
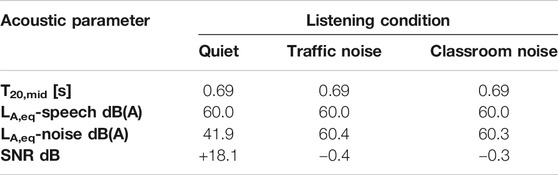
TABLE 1. Listening conditions in the classrooms during the experiments, in terms of reverberation times (T20,mid, averaged over the 0.5–2 kHz frequency bands) and sound pressure levels (LA,eq). The reported values are the spatial averages across four positions in the audience and across repetitions over the classes.
Procedures
We used a within-subject study design, with all children performing each task in the three listening conditions. The order of the listening conditions was balanced across the classes in each school year. Children took part in the experiment as a whole class, and the tasks were administered collectively. The three tasks were completed in two sessions, one week apart. The children completed the mental calculation task in the first session, then the speech perception and sentence comprehension tasks in the second. Both sessions lasted about 1 h (including the acoustic measurements, repeated for each class after completing the experimental tasks). To avoid order and fatigue effects, the order of the speech perception and sentence comprehension tasks was counterbalanced across the classes in each school year. The children completed the standardized reading comprehension and maths fluency assessments in their classrooms nearly a week after the second experimental session.
For each task, the children were given instructions and practiced with three or four trials in quiet conditions. Then they were administered three tasks, one for each listening condition. The order of the listening conditions was balanced across the classes for each school year. During the tests, the background noise started approximately 1 s before the speech signal and ended simultaneously with it. In the quiet condition, an acoustic signal (a brief pure tone at 500 Hz) was played back 1 s before the spoken sentence. The next trial was automatically played back only after all participants had answered or reached the time limit (12, 15, or 20 s, depending on the task). Participants were instructed to pay attention to the task and to respond as accurately as possible.
Effort Ratings
Following the completion of each task in a given listening condition, the children were asked to report how much effortful the task had been (“How much effort did doing this task require?”). Their answers were given using on a categorical rating scale from one (“minimum effort”) to five (“maximum effort”). The question and the scale were presented to the children on the tablet and the numbers they used for their answers were visible on the screen.
Data Analysis
Linear mixed-effects models (LMMs) were used for the statistical analysis, using the R software (R Core Team, 2017), and the lme4 package (Bates et al., 2015). The outcome variable was the self-rated listening effort. The fixed effects included listening condition (quiet, traffic noise, classroom noise), age (categorical variable: 11, 12, 13 years), and their interaction. One model was set up for each task. Individual scores in the reading comprehension test were included as a covariate in the models for the speech perception and sentence comprehension tasks. Individual scores in the maths fluency test were included as a covariate in the models for the mental calculation task. The participant variable was included in all models as a random intercept. In LMMs, the fixed effects represent average trends in the data. Including participants as random effects in the model overcomes the problem of non-independence of the data, and accounts for the fact that each participant may react differently from the average trend. When analyzing RTs, for instance, this approach accounts for the fact that some participants respond more slowly than others.
The p-values and χ2 values for the LMMs were obtained with the afex package (Singmann et al., 2021). The normality of the random effects and residuals was checked for each model to identify potential violations of statistical assumptions (Everitt and Hothorn, 2010). Post-hoc tests were run and standardized effect sizes (corresponding to Cohen’s d) were calculated with the emmeans package (Lenth, 2020). In the case of multiple comparisons, the p-values were adjusted using the false discovery rate procedure (Benjamini and Hochberg, 1995).
Finally, a correlation analysis was run between self-ratings, task performance accuracy, and RTs. The correlations were examined using a repeated-measures correlation statistical technique, which examines the overall intra-individual association between two measures (Bakdash and Marusich, 2017). This method was chosen to account for the repeated-measures design of the experiment, yielding non-independent observations across listening conditions. The main advantages of this regression technique over standard approaches are: 1) the chance to analyze paired repeated measures without any averaging, and without violating independence assumptions (Bakdash and Marusich, 2017); and 2) the high statistical power, enabling within-subject associations between measures to be tested without any need for large samples of participants (McGarrigle et al., 2020). In the present study, the repeated-measures correlation was used to examine to what extent two measures (RT and self-ratings, or accuracy and self-ratings) show a corresponding variance as a function of changes in the within-subject factor (listening condition). The analysis was implemented using the rmcorr package in R (Bakdash and Marusich, 2017). The Bonferroni method was applied to adjust the p-values for multiple comparisons.
Results
The findings are reported as the scores participants gave to the amount of effort they felt they had put into the tasks, on a scale from one to five (from less to more effort). Figure 1 shows the average perceived effort ratings, by listening condition and age: it suggests that children found the tasks more effortful in background noise than in quiet conditions. The pattern varied, however, depending on the task. Age-related changes in perceived effort appeared to be quite small. A detailed statistical analysis of the effort ratings is reported in Effort Ratings.
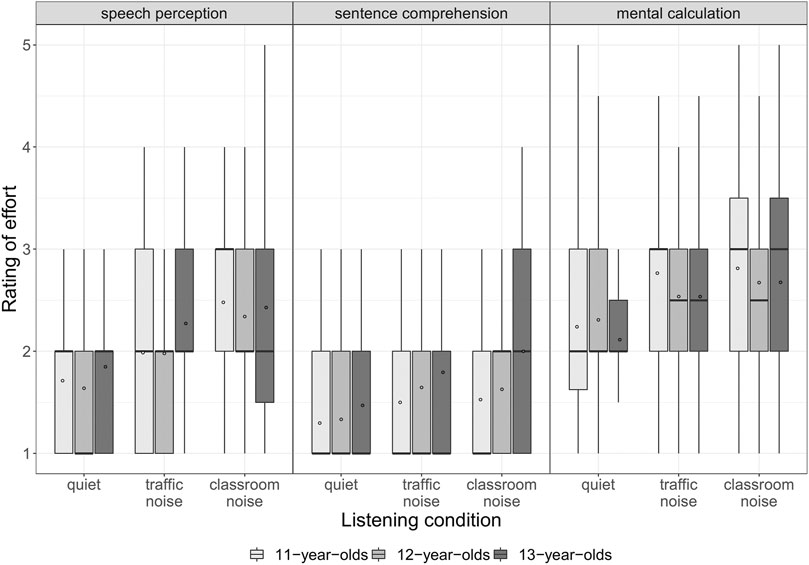
FIGURE 1. Self-ratings of effort for each listening condition and age. In the boxplots the bottom and top boxes are the first and third quartiles of the data distributions; the central, bold line is the median, and the white circle is the mean; 99% of the data fall within the whiskers.
Figure 2 shows the frequency distribution of the self-ratings by task and listening condition, over the five scores on the scale. The scores were generally low (from one to three). It was only in the mental calculation task, and in the classroom noise condition in particular, that the ratings were more evenly distributed over the whole scale.
Table 2 provides descriptive statistics on performance accuracy and RTs in the three tasks, by age and listening condition; these data are relevant to the correlation analyses. The RTs were defined as the time elapsing between the audio stimulus offset and the moment an answer was chosen. A detailed analysis of these results is reported elsewhere (Prodi et al., 2019a; Caviola et al., 2021).

TABLE 2. Mean correct answers (in %) and response times (in ms) in each task, by listening condition. Standard deviations are given in parentheses.
Effort Ratings
Table 3 shows the statistical results for the three linear mixed-effects models (one for each task).

TABLE 3. Linear mixed-effects models for predictors of effort ratings in the three tasks (Reference levels: Listening condition–quiet; Age–11 years).
For the speech perception task, there was a significant main effect of listening condition (χ2(2) = 63.83, p < 0.001), with effort ratings higher for noisy than for quiet conditions (Table 3). The main effects of age (p = 0.41), reading comprehension score (p = 0.84), and the listening condition x age interaction (p = 0.49) were not significant. Pairwise comparisons indicated that perceived effort was significantly lower in quiet than in noisy conditions (quiet < traffic noise: t = −4.25, p < 0.01, d = 0.51, difference = 0.35; quiet < classroom noise: t = −8.33, p < 0.01, d = 1.00, difference = 0.67), and in traffic noise than in classroom noise (traffic noise < classroom noise: t = -4.07, p < 0.01, d = 0.49, difference = 0.33).
Regarding the sentence comprehension task, the analysis again revealed a significant main effect of listening condition (χ2(2) = 35.33, p < 0.001), with effort ratings higher for noisy than for quiet conditions (Table 3). The main effects of age (p = 0.06), reading comprehension score (p = 0.36), and the listening condition x age interaction (p = 0.37) were not significant. Pairwise comparisons indicated that perceived effort was significantly lower in quiet than in noisy conditions (quiet < traffic noise: t = −4.47, p < 0.01, d = 0.54, difference = 0.28; quiet < classroom noise: t = −5.77, p < 0.01, d = 0.70, difference = 0.37). There was no difference in the effort ratings between the two noisy conditions (p = 0.55).
As for the mental calculation task, there was a significant main effect of listening condition in this case too (χ2(2) = 44.42, p < 0.001), with effort ratings higher for noisy than for quiet conditions (Table 3). The main effect of the maths fluency score was significant too (χ2(1) = 9.89, p = 0.002): examining the summary output (Table 3) showed that, when all other predictors were set to the reference level, an increase of one standard deviation in the maths fluency score coincided with an estimated 0.22 lower perceived effort. The main effect of age (p = 0.63) and the listening condition x age interaction (p = 0.20) were not significant. Pairwise comparisons indicated that perceived effort was rated significantly lower in quiet than in noisy conditions (quiet < traffic noise: t = −5.28, p < 0.01, d = 0.59, difference = 0.40; quiet < classroom noise: t = -6.41, p < 0.01, d = 0.72, difference = 0.49). There was no difference between the effort ratings in the two types of noise (p = 0.76).
Correlation Analysis
Repeated-measures correlation tests were run to explore the association between perceived effort, task performance accuracy and RTs, as a function of changes in the listening condition. The results showed that the correlation between the measures depended on the task.
In particular, for the speech perception task, there was an inverse relationship between perceived effort and performance accuracy, higher effort ratings being associated with a worse performance [r = −0.47; p < 0.001; 95%CI (−0.55–−0.38)]. There was a positive relationship instead between perceived effort and RTs, i.e., higher scores for effort were associated with longer RTs [r = 0.16; p = 0.004; 95%CI (0.03–0.27)].
No significant correlation emerged between effort ratings and RTs in the sentence comprehension task (r = −0.006, p = 0.91). No correlation analysis was run between effort ratings and accuracy due to the ceiling effect in task performance accuracy.
Finally, there was a significant relationship between effort ratings and performance accuracy in the mental calculation task [r = −0.14; p = 0.011; 95%CI (−0.026–−0.063)], but not between effort ratings and RTs (r = 0.001, p = 0.98).
Discussion
The two aims of this study were: 1) to assess the effect of listening condition on children’s self-ratings of the effort needed to perform a task; and 2) to investigate the relationship between children’s effort ratings and their task performance accuracy, and a behavioral measure of effort (RTs). The two aims are discussed separately below.
Effects of Listening Condition on Effort Ratings
We used three tasks typical of daily classroom activities (speech perception, sentence comprehension, and mental calculation tasks) to examine the effect of listening condition in the classroom (quiet, traffic noise, classroom noise) on the effort that 11- to 13-year-olds reported it costing them to perform such tasks. Our analyses showed that it was more effortful to work in the two noisy conditions than in quiet, for all tasks. The children found classroom noise more disturbing than traffic noise, but only in the speech perception task.
Our results are in line with previous research showing that school-age children found it more effortful to work in adverse (noisy) conditions than in quiet (von Lochow et al., 2018; Picou et al., 2019). These findings support the idea that performing a task in the presence of background noise (whatever its spectral characteristics or informational content) requires the allocation of more cognitive resources, and this is perceived by children as demanding a greater effort. It is worth noting that, when performance in quiet is already near-ceiling (as in our sentence comprehension task), adding background noise will increase perceived effort without affecting performance (Krueger et al., 2017).
Judging from our findings for noisy conditions, a difference in the effort required for different types of background noise only emerged in the speech perception task, in which case the children found classroom noise as more disturbing than traffic noise. The greater perceived effort required may be attributable more to the characteristics of the noise than to its level, since the SNR was the same in the two noisy conditions. Unlike the traffic noise (which had a stationary temporal envelope), the classroom noise used in our experiment had speech-like temporal fluctuations and included salient events. Given the mechanisms of auditory distraction (Hughes, 2014), the particular combination of the noise’s changing state with its salient embedded events might have taxed the children’s cognitive resources by competing with the speech material they needed to process, and by prompting them to become disengaged from the task. A greater use of cognitive resources would be needed to deal with the noise, thereby causing an increase in the experience of perceived effort. Our findings add to the limited literature on school-aged children’s self-reported effort ratings in a speech perception task. Klatte et al. (2010) reported that a significant effect of the type of background noise on how adults rated the disturbance, but the same did not apply to children in first and third grade. Similarly, Prodi et al. (2010) found that task difficulty ratings by children 8–10 years old were less sensitive to listening condition than those of adults. On the other hand, Picou et al. (2019) showed that 10- to 17-year-olds’ self-rated ease of listening was sensitive to changes in the SNR.
No significant difference in the effort ratings emerged between the two noisy conditions in the sentence comprehension task, while the RTs were significantly longer in classroom noise than in traffic noise (Prodi et al., 2019a; Caviola et al., 2021). This result is in line with reports from von Lochow et al. (2018), and Klatte et al. (2010). The former found no significant increase in the perceived effort to complete a passage comprehension task when the number of background speakers was increased from one to four. The latter found that children’s ratings of perceived disturbance did not discriminate between the effects of classroom noise and background speech. Both studies concluded that, although children are aware of the disruption caused by background noise, they do not notice any change in the cognitive load of completing a task with different types of background noise. As concerns the sentence comprehension task used in the present study, an alternative explanation might lie in the fact that children’s accuracy was at ceiling for all listening conditions. The presence of a four-option, forced-choice paradigm, and the contextual cues provided by the sentences might have made the task too easy for any changes in background noise to affect their accuracy or perceived effort (though it did influence their RTs).
The type of background noise also failed to affect the children’s perceived effort in the mental calculation task. This was the only task in which there was a significant effect of individual proficiency (i.e., maths fluency score) on the self-ratings, however. Each child’s maths fluency score significantly predicted their effort ratings, the children with higher scores perceiving the task as less effortful. For this task, the perceived effort seems to relate to the processing load involved: children more proficient in maths adopted more efficient strategies to complete the mental calculations, and this cost them less effort. It could also be that mastering mental calculation is associated with a more domain-general attentional control, which would be responsible for a better control over the distracting effect of noise. Unfortunately, no specific data were obtained in this study to test such a hypothesis.
Finally, it is worth noting that our children’s effort ratings were rather low, mainly ranging from one to three (on a scale from one to five; Figure 2). Their tendency to use scores indicating a limited perceived effort might stem from subjective differences in effort “threshold” (McGarrigle et al., 2014), such that conditions rated as effortful by one sample of listeners might not be rated in the same way by another. Judging from the literature older adults tend to underestimate their perceived listening effort by comparison with young adults (Larsby et al., 2005); non-native listeners report less perceived effort than native listeners, despite the former having a worse performance (Visentin et al., 2018); and children 8–10 years old tend to rate their listening difficulty more favorably than adults (Prodi et al., 2010). We need to bear in mind that children’s self-ratings could be affected by a social desirability response bias (King and Bruner, 2000); in other words, children may give researchers the answers they think researches would like to hear. For children’s self-ratings to be usable, it is crucial to assess this issue, and try to control it experimentally. This will be the object of a specific study.
Alternatively, the children’s reasons for using only the lower scores on the rating scale could have to do with their motivation towards the experiment and the tasks. According to the FUEL model (Pichora-Fuller et al., 2016), perceived effort depends not only on the demands of a task, but also on respondents’ motivation, which mediates the cognitive demands of the task when listeners prioritize the allocation of their resources (Peelle, 2018). In the present case, the children were probably strongly motivated: the unconventional classroom experience involved in the listening tests was able to keep them engaged throughout the experiment. This was also noted by the experimenters, who could be considered as qualitative witnesses of the children’s motivation. Unfortunately, no specific quantitative data were retrieved on this aspect, and our understanding of what motivates children to listen and engage in academic tasks in the classroom is rather limited (Rudner et al., 2018). More research is needed in this area, including motivation as a mediator in the analysis of self-rated effort.
Relations Between Effort Ratings, Accuracy, and RTs
A second goal of the present study was to examine within-subject associations between effort ratings, RTs, and performance accuracy in different tasks, as a function of the changes in listening condition.
An inverse within-subject association was found between effort ratings and accuracy (in the speech perception and mental calculation tasks), a better performance in the task being associated with lower effort ratings. This result is in line with previous findings referring mainly to speech reception tasks. For instance, Morimoto et al. (2004) found that a very high task performance (but still not at the ceiling) showed a strong negative correlation with the subjective judgement of listening difficulty and intelligibility–and self-rated listening difficulty was a more accurate indicator of performance than intelligibility. We did not find self-reported effort a more sensitive performance indicator than accuracy, but our results might be limited by the fact that our children only used the lower scores on the rating scale, so the variation in their effort ratings was limited.
A significant relationship between the two measures of effort considered here (self-rated effort and RT) only emerged for the speech reception task, but the two measures did not show a corresponding change with listening condition in the sentence comprehension and mental calculation tasks. These findings give the impression that the relationship between different measures of effort might depend on the type of task (e.g., characteristics, difficulty), potentially reinforcing the claim that they tap into different underlying cognitive dimensions (Alhanbali et al., 2019). For instance, recent research indicates a dissociation between ratings of “ease” or “effort” and behavioral measures of effort, in both adults (Lemke and Besser, 2016; Visentin et al., 2019) and children (Picou et al., 2019; Oosthuizen et al., 2021b). Studies exploring this relationship in school-age children are scarce, however, and future studies need to address this research gap.
Study Limitations and Future Directions
Several aspects of this study may limit the generalizability of our findings.
First, we assessed perceived effort based on a single question (“How much effort did doing this task require?”), which might be too difficult for the children to understand. According to Kahneman and Frederick (2002), people faced with a difficult question tend to answer a different, easier question. Formulating the question in a way that is easier for children to understand might generate results that are more reliable, and less biased toward positive responses (Picou et al., 2019).
Second, we used a 5-point rating scale for the self-ratings. It may be better to use a visual analogue scale to obtain a finer measure of the amount of effort perceived. This might give us more useful information on the construct assessed, and avoid systematic upward or downward bias deriving from the limited number of scores on the scale (Kuhlmann et al., 2017).
Third, none of the variables included in the statistical analysis (listening condition, age, individual proficiency in the baseline task) accounted for much of the substantial inter-individual variance in self-ratings of effort, as indicated by the conditional R-squared coefficients in Table 3. This suggests that other (intrinsic or extrinsic) factors might influence school-age children’s effort ratings. For instance, the FUEL model indicates that listening effort depends both on the demands of a task and on the listener’s motivation. The latter governs how hard listeners try to understand what is being said and governs how well their perceptual and cognitive abilities are used (Lidestam and Beskow, 2006). Future studies should include questions to assess children’s motivation in order to shed light on how much they focus their attention on completing the task.
Another aspect of effort that future research could explore concerns confidence ratings, or how much guesswork respondents feel they have used in completing a task. This would give us an idea of their meta-cognitive monitoring abilities, i.e., the degree to which respondents are capable of monitoring whether they have understood the message correctly or not (Giovanelli et al., 2021). This aspect is especially relevant in the case of children working in classrooms in inadequate acoustic conditions, as an adequate meta-cognitive monitoring of their teacher’s verbal communication would trigger compensatory strategies (e.g., the children would ask the teacher to repeat a sentence, or speak more slowly or louder) to help them cope with the adverse listening conditions.
Conclusion
This study investigated the effect of background noise conditions on self-ratings of listening effort in children aged 11–13 years completing three academic tasks (speech perception, sentence comprehension, and mental calculation). In all three tasks, the children’s perceived effort was greater in background noise than in quiet conditions, but it was only in the speech perception task that the type of background noise (traffic versus classroom noise) influenced their effort ratings.
Our results indicate a significant within-subject association between children’s effort ratings and their accuracy in all tasks. On the other hand, a significant link between their effort ratings and a behavioral measure of listening effort (response times) only emerged for the speech perception task.
Overall, the present findings go to show that self-ratings could be useful for measuring changes in school-aged children’s perceived listening effort. More research is needed to clarify, and thus control the individual factors that influence children’s effort ratings (such as motivation) and to devise an appropriate response format.
Data Availability Statement
The raw data supporting the conclusions of this article will be made available by the authors, without undue reservation.
Ethics Statement
The studies involving human participants were reviewed and approved by the Ethics Committee at the University of Padova (Italy) and by the school managements. Written informed consent to participate in this study was provided by the participants’ legal guardian/next of kin.
Authors Contributions
CV and NP conceived and designed the study and took care of data collection. CV performed the statistical analysis and wrote the first draft of the paper, and both authors were actively involved in preparing the final draft.
Funding
This work was funded partly by the Emilia-Romagna Regional Authority’s COMPRENDO project (Action POR FESR 2014-20–Axis 1 Action 1.2.2 (2019)), and partly by the BRIC 2019 project-topic ID 14 of the National Institute for Insurance against Accidents at Work (INAIL).
Conflict of Interest
The authors declare that the research was conducted in the absence of any commercial or financial relationships that could be construed as a potential conflict of interest.
Acknowledgments
The authors would like to thank Erika Borella and Irene Mammarella, who contributed advice, ideas and opinions on the selection and administration of the tasks, and Alberto Di Domenico and Sara Caviola, who provided the materials for the sentence comprehension and mental calculation tasks. The authors also thank the children and teachers who took part in the study. Finally, the authors acknowledge Hörtech GmbH, Oldenburg, Germany, for providing the speech recordings of the Matrix Sentence Test in the Italian language.
References
Alhanbali, S., Dawes, P., Millman, R. E., and Munro, K. J. (2019). Measures of Listening Effort Are Multidimensional. Ear and Hearing 40 (5), 1084–1097. doi:10.1097/AUD.0000000000000697
Bakdash, J. Z., and Marusich, L. R. (2017). Repeated Measures Correlation. Front. Psychol. 8, 456. doi:10.3389/fpsyg.2017.00456
Bates, D., Mächler, M., Bolker, B., and Walker, S. (2015). Fitting Linear Mixed-Effects Models Usinglme4. J. Stat. Soft. 67, 1–48. doi:10.18637/jss.v067.i01
Benjamini, Y., and Hochberg, Y. (1995). Controlling the False Discovery Rate: a Practical and Powerful Approach to Multiple Testing. J. R. Stat. Soc. Ser. B (Methodological). 57, 289–300. doi:10.1111/j.2517-6161.1995.tb02031.x
Caviola, S., Gerotto, G., Lucangeli, D., and Mammarella, I. C. (2016). Test AC-FL: Prove di fluenza per le abilità di calcolo [AC-FL Test: Maths fluency abilities test]. Trento: Edizioni Centro Studi Erickson.
Caviola, S., Visentin, C., Borella, E., Mammarella, I., and Prodi, N. (2021). Out of the Noise: Effects of Sound Environment on Maths Performance in Middle-School Students. J. Environ. Psychol. 73, 101552. doi:10.1016/j.jenvp.2021.101552
Cecchetto, C., Di Domenico, A., Garraffa, M., and Papagno, C. (2012). Comprendo. Batteria per la Comprensione di frasi negli adulti [Comprendo. Test battery for sentence comprehension in adults]. Milano: Raffaello Cortina Editore.
Choi, S., Lotto, A., Lewis, D., Hoover, B., and Stelmachowicz, P. (2008). Attentional Modulation of Word Recognition by Children in a Dual-Task Paradigm. J. Speech Lang. Hear. Res. 51 (4), 1042–1054. doi:10.1044/1092-4388(2008/07610.1044/1092-4388(2008/076)
Cornoldi, C., Carretti, B., and Colpo, C. (2017). Prove MT-Kit Scuola. Dalla valutazione degli apprendimenti di lettura e comprensione al potenziamento [MT Tests - School Kit. From the assessment of reading and comprehension to its improvement]. Firenze: Organizzazioni Speciali Editore.
Crukley, J., Scollie, S., and Parsa, V. (2011). An Exploration of Non-quiet Listening at School. J. Educ. Audiol. 17 (1), 23–35.
Dreschler, W. A., Verschuure, H., Ludvigsen, C., and Westermann, S. (2001). ICRA Noises: Artificial Noise Signals with Speech-like Spectral and Temporal Properties for Hearing Instrument Assessment: Ruidos ICRA: Señates de ruido artificial con espectro similar al habla y propiedades temporales para pruebas de instrumentos auditivos. Int. J. Audiol. 40, 148–157. doi:10.3109/00206090109073110
Everitt, B. S., and Hothorn, T. (2010). A Handbook of Statistical Analyses Using R. New York: Chapman & Hall.
Giovanelli, E., Valzolgher, C., Gessa, E., Todeschini, M., and Pavani, F. (2021). Unmasking the Difficulty of Listening to Talkers with Masks: Lessons from the COVID-19 Pandemic. i-Perception. 12 (2), 204166952199839. doi:10.1177/2041669521998393
Gómez-Merino, N., Gheller, F., Spicciarelli, G., and Trevisi, P. (2020). Pupillometry as a Measure for Listening Effort in Children: a Review. Hearing, Balance Commun. 18 (3), 152–158. doi:10.1080/21695717.2020.1807256
Gustafson, S., McCreery, R., Hoover, B., Kopun, J. G., and Stelmachowicz, P. (2014). Listening Effort and Perceived Clarity for normal-hearing Children with the Use of Digital Noise Reduction. Ear Hear. 35 (2), 183–194. doi:10.1097/01.aud.0000440715.85844.b8
Hughes, R. W. (2014). Auditory Distraction: a Duplex-Mechanism Account. PsyCh J. 3 (1), 30–41. doi:10.1002/pchj.44
Geneva: International Organization for Standardization (2009). Acoustics – Measurement of Room Acoustic Parameters – Part 1: Performance Spaces. Geneva, Switzerland, ISO3382–1.:2009.
Kahneman, D., and Frederick, S. (2002). “Representativeness Revisited: Attribute Substitution in Intuitive Judgment,” in Heuristics and Biases: The Psychology of Intuitive Judgment. Editors T. Gilovich, D. Griffin, and D. Kahneman (Cambridge: Cambridge University Press), 49–81. doi:10.1017/CBO9780511808098.004
King, M. F., and Bruner, G. C. (2000). Social Desirability Bias: a Neglected Aspect of Validity Testing. Psychol. Mark. 17 (2), 79–103. doi:10.1002/(SICI)1520-6793(200002)17:2<79::AID-MAR2>3.0.CO;2-0
Klatte, M., Lachmann, T., and Meis, M. (2010). Effects of Noise and Reverberation on Speech Perception and Listening Comprehension of Children and Adults in a Classroom-like Setting. Noise Health 12, 270–282. doi:10.4103/1463-1741.70506
Krueger, M., Schulte, M., Zokoll, M. A., Wagener, K. C., Meis, M., Brand, T., et al. (2017). Relation between Listening Effort and Speech Intelligibility in Noise. Am. J. Audiol. 26 (3S), 378–392. doi:10.1044/2017_AJA-16-0136
Kuhlmann, T., Dantlgraber, M., and Reips, U. D. (2017). Investigating Measurement Equivalence of Visual Analogue Scales and Likert-type Scales in Internet-Based Personality Questionnaires. Behav. Res. 49 (6), 2173–2181. doi:10.3758/s13428-016-0850-x
Larsby, B., Hällgren, M., Lyxell, B., and Arlinger, S. (2005). Cognitive performance and perceived effort in speech processing tasks: effects of different noise backgrounds in normal-hearing and hearing-impaired subjects Desempeño cognitivo y percepción del esfuerzo en tareas de procesamiento del lenguaje: Efectos de las diferentes condiciones de fondo en sujetos normales e hipoacúsicos. Int. J. Audiol. 44 (3), 131–143. doi:10.1080/14992020500057244
Lau, M. K., Hicks, C., Kroll, T., and Zupancic, S. (2019). Effect of Auditory Task Type on Physiological and Subjective Measures of Listening Effort in Individuals with normal Hearing. J. Speech Lang. Hear. Res. 62 (5), 1549–1560. doi:10.1044/2018_JSLHR-H-17-0473
Lemke, U., and Besser, J. (2016). Cognitive Load and Listening Effort: Concepts and Age-Related Considerations. Ear Hear. 37, 77S–84S. doi:10.1097/AUD.0000000000000304
Lenth, R. V. (2020). Data From: Emmeans: Estimated Marginal Means, Aka Least-Squares Means. R package version 1.4.4. https://CRAN.R-project.org/package=emmeans.
Lewis, D., Schmid, K., O'Leary, S., Spalding, J., Heinrichs-Graham, E., and High, R. (2016). Effects of Noise on Speech Recognition and Listening Effort in Children with normal Hearing and Children with Mild Bilateral or Unilateral Hearing Loss. J. Speech Lang. Hear. Res. 59 (5), 1218–1232. doi:10.1044/2016_JSLHR-H-15-0207
Lidestam, B., and Beskow, J. (2006). Motivation and Appraisal in Perception of Poorly Specified Speech. Scand. J. Psychol. 47 (2), 93–101. doi:10.1111/j.1467-9450.2006.00496.x
Mattys, S. L., Davis, M. H., Bradlow, A. R., and Scott, S. K. (2012). Speech Recognition in Adverse Conditions: A Review. Lang. Cogn. Process. 27, 953–978. doi:10.1080/01690965.2012.705006
McGarrigle, R., Dawes, P., Stewart, A. J., Kuchinsky, S. E., and Munro, K. J. (2017). Measuring Listening-Related Effort and Fatigue in School-Aged Children Using Pupillometry. J. Exp. Child Psychol. 161, 95–112. doi:10.1016/j.jecp.2017.04.006
McGarrigle, R., Gustafson, S. J., Hornsby, B. W. Y., and Bess, F. H. (2019). Behavioral Measures of Listening Effort in School-Age Children: Examining the Effects of Signal-To-Noise Ratio, Hearing Loss, and Amplification. Ear Hear. 40, 381–392. doi:10.1097/AUD.0000000000000623
McGarrigle, R., Munro, K. J., Dawes, P., Stewart, A. J., Moore, D. R., Barry, J. G., et al. (2014). Listening Effort and Fatigue: What Exactly Are We Measuring? A British Society of Audiology Cognition in Hearing Special Interest Group 'white Paper'. Int. J. Audiol. 53 (7), 433–445. doi:10.3109/14992027.2014.890296
McGarrigle, R., Rakusen, L., and Mattys, S. (2020). Effortful Listening under the Microscope: Examining Relations between Pupillometric and Subjective Markers of Effort and Tiredness from Listening. Psychophysiology 58 (1), e13703. doi:10.1111/psyp.13703
Mealings, K. (2016). “Classroom Acoustic Conditions: Understanding what Is Suitable through a Review of National and International Standards, Recommendations, and Live Classroom Measurements,” in Proceedings of Acoustics 2016 – The Second Australasian Acoustical Societies Conference. Editors I. Hillock, and D. Mee (Brisbane, Australia: The Australian Acoustical Society), 145–172.
Moore, T. M., and Picou, E. M. (2018). A Potential Bias in Subjective Ratings of Mental Effort. J. Speech Lang. Hear. Res. 61 (9), 2405–2421. doi:10.1044/2018_JSLHR-H-17-0451
Morimoto, M., Sato, H., and Kobayashi, M. (2004). Listening Difficulty as a Subjective Measure for Evaluation of Speech Transmission Performance in Public Spaces. The J. Acoust. Soc. America 116 (3), 1607–1613. doi:10.1121/1.1775276
Oosthuizen, I., Picou, E. M., Pottas, L., Myburgh, H. C., and Swanepoel, D. W. (2021b). Listening Effort in School-Age Children with normal Hearing Compared to Children with Limited Useable Hearing Unilaterally. Am. J. Audiol. 30, 309–324. doi:10.1044/2021_AJA-20-00082
Oosthuizen, I., Picou, E. M., Pottas, L., Myburgh, H. C., and Swanepoel, D. W. (2021a). Listening Effort in School-Aged Children with Limited Useable Hearing Unilaterally: Examining the Effects of a Personal, Digital Remote Microphone System and a Contralateral Routing of Signal System. Trends Hearing 25, 233121652098470. doi:10.1177/2331216520984700
Peelle, J. E. (2018). Listening Effort: How the Cognitive Consequences of Acoustic challenge Are Reflected in Brain and Behavior. Ear Hear. 39 (2), 204–214. doi:10.1097/AUD.0000000000000494
Pichora-Fuller, M. K., Kramer, S. E., Eckert, M. A., Edwards, B., Hornsby, B. W. Y., Humes, L. E., et al. (2016). Hearing Impairment and Cognitive Energy: the Framework for Understanding Effortful Listening (FUEL). Ear Hear. 37, 5S–27S. doi:10.1097/AUD.0000000000000312
Picou, E. M., Bean, B., Marcrum, S. C., Ricketts, T. A., and Hornsby, B. W. Y. (2019). Moderate Reverberation Does Not Increase Subjective Fatigue, Subjective Listening Effort, or Behavioral Listening Effort in School-Aged Children. Front. Psychol. 10, 1749. doi:10.3389/fpsyg.2019.01749
Picou, E. M., and Ricketts, T. A. (2018). The Relationship between Speech Recognition, Behavioural Listening Effort, and Subjective Ratings. Int. J. Audiol. 57 (6), 457–467. doi:10.1080/14992027.2018.1431696
Prodi, N., and Visentin, C. (in press). A Slight Increase in Reverberation Time in the Classroom Affects Performance and Behavioral Listening Effort. Manuscript Accepted for Publication in Ear Hear. Ear Hear.
Prodi, N., Visentin, C., and Bellettini, C. (2012). “Listening Efficiency Testing,” in Audio Engineering Society Conference: 45th International Conference: Applications of Time-Frequency Processing in Audio, Helsinki, Finland, March 1–4, 2012 (Audio Engineering Society).
Prodi, N., Visentin, C., Borella, E., Mammarella, I. C., and Di Domenico, A. (2019a). Noise, Age, and Gender Effects on Speech Intelligibility and Sentence Comprehension for 11- to 13-Year-Old Children in Real Classrooms. Front. Psychol. 10, 2166. doi:10.3389/fpsyg.2019.02166
Prodi, N., Visentin, C., Borella, E., Mammarella, I. C., and Di Domenico, A. (2021). Using Speech Comprehension to Qualify Communication in Classrooms: Influence of Listening Condition, Task Complexity and Students' Age and Linguistic Abilities. Appl. Acoust. 182, 108239. doi:10.1016/j.apacoust.2021.108239
Prodi, N., Visentin, C., and Farnetani, A. (2010). Intelligibility, Listening Difficulty and Listening Efficiency in Auralized Classrooms. J. Acoust. Soc. America 128, 172–181. doi:10.1121/1.3436563
Prodi, N., Visentin, C., Peretti, A., Griguolo, J., and Bartolucci, G. B. (2019b). Investigating Listening Effort in Classrooms for 5- to 7-Year-Old Children. Lshss 50 (2), 196–210. doi:10.1044/2018_LSHSS-18-0039
Puglisi, G. E., Warzybok, A., Hochmuth, S., Visentin, C., Astolfi, A., Prodi, N., et al. (2015). An Italian Matrix Sentence Test for the Evaluation of Speech Intelligibility in Noise. Int. J. Audiol. 54, 44–50. doi:10.3109/14992027.2015.1061709
Rönnberg, J., Lunner, T., Zekveld, A., Sörqvist, P., Danielsson, H., Lyxell, B., et al. (2013). The Ease of Language Understanding (ELU) Model: Theoretical, Empirical, and Clinical Advances. Front. Syst. Neurosci. 7, 31. doi:10.3389/fnsys.2013.00031
Rönnberg, J., Rudner, M., Foo, C., and Lunner, T. (2008). Cognition Counts: a Working Memory System for Ease of Language Understanding (ELU). Int. J. Audiol. 47, S99–S105. doi:10.1080/14992020802301167
Rudner, M., Lyberg-Åhlander, V., Brännström, J., Nirme, J., Pichora-Fuller, M. K., and Sahlén, B. (2018). Listening Comprehension and Listening Effort in the Primary School Classroom. Front. Psychol. 9, 1193. doi:10.3389/fpsyg.2018.01193
Shield, B. M., and Dockrell, J. E. (2008). The Effects of Environmental and Classroom Noise on the Academic Attainments of Primary School Children. J. Acoust. Soc. America 123, 133–144. doi:10.1121/1.2812596
Singmann, H., Bolker, B., Westfall, J., Aust, F., and Ben-Shachar, M. S. (2021). Data From: Afex: Analysis of Factorial Experiments. R package version 0.28-1. https://CRAN.R-project.org/package=afex.
Steel, M. M., Papsin, B. C., and Gordon, K. A. (2015). Binaural Fusion and Listening Effort in Children Who Use Bilateral Cochlear Implants: a Psychoacoustic and Pupillometric Study. PLOS ONE 10 (2), e0117611. doi:10.1371/journal.pone.0117611
Strand, J. F., Brown, V. A., Merchant, M. B., Brown, H. E., and Smith, J. (2018). Measuring Listening Effort: Convergent Validity, Sensitivity, and Links with Cognitive and Personality Measures. J. Speech Lang. Hear. Res. 61 (6), 1463–1486. doi:10.1044/2018_JSLHR-H-17-0257
Visentin, C., Prodi, N., Cappelletti, F., Torresin, S., and Gasparella, A. (2019). Speech Intelligibility and Listening Effort in university Classrooms for Native and Non-native Italian Listeners. Building Acoust. 26 (4), 275–291. doi:10.1177/1351010X19882314
Visentin, C., Prodi, N., Cappelletti, F., Torresin, S., and Gasparella, A. (2018). Using Listening Effort Assessment in the Acoustical Design of Rooms for Speech. Building Environ. 136, 38–53. doi:10.1016/j.buildenv.2018.03.020
von Lochow, H., Lyberg-Åhlander, V., Sahlén, B., Kastberg, T., and Brännström, K. J. (2018). The Effect of Voice Quality and Competing Speakers in a Passage Comprehension Task: Perceived Effort in Relation to Cognitive Functioning and Performance in Children with normal Hearing. Logop. Phoniatr. Vocology 43 (1), 32–41. doi:10.1080/14015439.2017.1307446
Keywords: children, classroom acoustics, listening effort, self-ratings, speech perception, comprehension, maths, noise
Citation: Visentin C and Prodi N (2021) How Reliable are 11- to 13-Year-Olds’ Self-Ratings of Effort in Noisy Conditions?. Front. Built Environ. 7:688016. doi: 10.3389/fbuil.2021.688016
Received: 30 March 2021; Accepted: 09 July 2021;
Published: 20 July 2021.
Edited by:
Hasim Altan, Arkin University of Creative Arts and Design (ARUCAD), CyprusReviewed by:
Harvey Dillon, Macquarie University, AustraliaShabana Urooj, Princess Nourah bint Abdulrahman University, Saudi Arabia
Copyright © 2021 Visentin and Prodi. This is an open-access article distributed under the terms of the Creative Commons Attribution License (CC BY). The use, distribution or reproduction in other forums is permitted, provided the original author(s) and the copyright owner(s) are credited and that the original publication in this journal is cited, in accordance with accepted academic practice. No use, distribution or reproduction is permitted which does not comply with these terms.
*Correspondence: Chiara Visentin, chiara.visentin@unife.it