- Department of Psychology, University of Milano-Bicocca, Milan, Italy
This Pictionary is suitable for communicating with individual unable to interact (locked-in syndrome, LIS) through the development of Brain Computer Interface (BCI) systems. It includes 60 validated, easy-to-understand illustrated plates depicting adults in various situations affecting their physiological or psychological state. The drawings are in color and represent persons of both sexes and various ethnicities. Twenty participants were interviewed to appropriately design the Pictionary. An additional group of 50 healthy adults (25 women and 25 men) aging 18–33 years was recruited to validate the pictogram corpus. Their schooling levels ranged from middle school to master's degrees. Participants were presented with five runs of randomly mixed pictograms illustrating 12 different motivational states, including primary and secondary needs, affective states, and somatosensory sensations (with five variants for each category). They had to precisely identify the motivational category illustrated (e.g., “Feeling pain” or “Being hungry”) while also providing information about its clarity and unambiguity on a Likert scale. Statistical analyses provided evidence of the strong communicative effectiveness of the illustrations (rated on average 2.7, on a 0 to 3 scale), with an accuracy of 98.4%. The PAIN set could be a valuable communication tool for individuals with LIS, as well as any clinical population lacking verbal communication skills. Its main purpose is to generate electrophysiological markers of internal mental states to be automatically classified by BCI systems.
Introduction
One of the most significant issues in dealing with individuals with LIS, who have lost the ability to communicate, is to understand their thoughts, in order to determine their condition, treat them, and address their needs. During the last decade, neuroengineers and neurophysiologists have made substantial progress in the development of sophisticated BCI systems to support human/computer communication (Naci et al., 2012). A BCI system is a device that can extract brain activity and process brain signals to enable computerized devices to accomplish specific purposes such as communicating or controlling prostheses (Wolpaw et al., 2002). The most commonly used BCI systems involve motor or kinaesthetic imagery (Jin et al., 2012; Milanés-Hermosilla et al., 2021; Mattioli et al., 2022), language (Panachakel and Ramakrishnan, 2021), face recognition (Kaufmann et al., 2013), and P300 detection (Azinfar et al., 2013; Guy et al., 2018; Mussabayeva et al., 2021). Neuroscientific studies have made significant advances in the detection of neurometabolic or electrophysiological markers of visual (Bobrov et al., 2011; Marmolejo-Ramos et al., 2015) and emotional imagery (Fan et al., 2018). For example, Proverbio et al. (2023) identified distinct Event-Related Potential (ERP) markers of visual and auditory imagery (relative to infants, human faces, animals, music, speech, and affective vocalizations), for BCI purposes, in absence of any sensory stimulation. Notwithstanding these advances, not much is known about the brain signatures of motivational imagery, such as needs or desires, because they are more difficult to be tested and evoked. According to some authors (Kavanagh et al., 2005), however, imagination can activate measurable responses to visceral desires. Imagining affectively charged memories induces measurable physiological changes such as, increased sweating, skin conductance, heart rate, and respiratory rate (Van Diest et al., 2001; Bywaters et al., 2004). Again, mental imagery of appetitive stimuli (e.g., eating lemons or drinking milk) was found to modify the salivation reflex and increase/decrease salivary pH levels (Vanhaudenhuyse et al., 2007).
The aim of the study was to investigate and validate a picture set whether it could evoke adequate P300 or N400 waves usable on a BCI. This issue belongs to the field of augmentative and alternative communication (AAC). Set of pictures or symbols can be used to communicate to non-verbal individuals after appropriate training. One of the most common aided AAC interventions to enhance functional communication skills is the Picture Exchange Communication System (Bondy and Frost, 1994), which is used for example with children affected by autistic spectrum disorder (ASD)(Pak et al., 2023). First, users learn to communicate with one picture symbol by associating the picture with desired token (e.g., some pretzels) and then learn to select between two or more pictures. Subsequently, individuals learn to combine picture symbols to make further requests. However, users have to overtly display their specific needs (for example, by reaching out for pretzels), which is not usable in individuals with LIS who are paralyzed or unable to move (e.g., in vigilant coma). Other AAC systems include speech-generating devices (SGD) (Wendt et al., 2019), i.e. portable electronic devices displaying graphic symbols or words, and producing synthesized speech output for communicating with others. SGD is also frequently used with ASD individuals (Van der Meer and Rispoli, 2010), but, again, it requires a certain degree of interaction. Up to now, available AAC systems can only be used by those who maintain some type of mobility, for example through key presses or ocular movements.
The purpose of this study was to provide a validated system of pictograms for getting insights on the motivational states and needs of individuals unable to communicate such as those affected by LIS, a condition in which a BCI could prove crucial in restoring the individual's basic communication skills (Wolpaw and Wolpaw, 2012). The present corpus is named “Pictionary-based communication tool for Assessing Individual Needs and motivational states” (the PAIN set). Pictograms (drawings, paintings, symbols, and photographs) are pictorial images used to enhance readability and comprehension of texts and concepts, and can be used to transmit information in a clear, expeditious, and simple manner. Cartoon illustrations are widely used to facilitate communication with patients about medication prescriptions and enable the communication between medical providers and stroke patients (Sarfo et al., 2016), or patients speaking foreign languages (Clawson et al., 2012).
Methods
Participants
Participants were 50 healthy adults (24 men and 26 women), aged 18–33 years (mean age = 23.22, SD = 2.85), with a mean schooling of 15.45 years. 48% of participants had a BA degree, 39% a high school diploma, 7% a middle school diploma and 6% a Master degree. Written informed consent was obtained from each participant. They had normal vision and no history of neurological/psychiatric disorders. The experiment was conducted in accordance with international ethical standards and approved by the Research Assessment Committee of the Department of Psychology for minimal risk projects under the aegis of the Ethical Committee of the University of Milano-Bicocca (protocol n: RM-2020-242).
Stimuli
Hundreds of images were processed with Photoshop and Paint graphic tools to generate novel colored cartoon-type drawings. The stimuli were created using CC BY-NC-SA 3.0 images fragments downloaded from Wikihow.com and appropriately modified for our purposes, as permitted. All images were adapted and modified. The images were 26 cm × 18 cm in size and represented an adult person in a specific emotional and motivational state. Sixty pictorial tables were finalized, in which 60 different characters (half male and half female) were depicted. Twelve different motivational, emotional, and visceral states were displayed, with 5 variants for each micro-category. For example, the “feeling hot” state was illustrated in 5 different ways: as the desire to be sprayed with ice water, to turn on the air conditioning, to put an icy cloth on the forehead, to eat a popsicle, and to stand in front of a fan. Furthermore, these subjective states were organized into four macro-categories (detailed in Table 1) based on their common properties.
Macro-categories were:
Primary needs (visceral sensations): reflecting the necessity of maintaining the body's homeostasis, based on sensations of hunger, thirst, or sleep. These visceral sensations would enable physiological regulation to maintain adaptive set points required for organismic integrity (Tucker and Luu, 2021) and would be neurally based on the limbic system and Papetz circuits identified by MacLean as regulating primary survival needs.
Somatosensory sensations: including cold, hot, and pain, mediated by peripheral or internal nociceptors and processed by the somatosensory system, signaling harmful thermal or physiological conditions (Gracheva and Bagriantsev, 2021).
Affective states: of fear, sadness, and cheerfulness, represented at both cortical and subcortical level (Phillips et al., 2003). Each emotion would rely on partly dedicated neural circuitry. In general, cheerfulness would be associated with an activation of the ventromedial mesolimbic dopaminergic system, sadness with an activation of habenular nuclei and cingulate cortex, and fear of the amygdalaloid nuclei (e.g., Gu et al., 2019), among other areas.
Secondary needs or desires: desire for listening to music, moving (running, jumping, dancing), and playing video games with friends. They can be assimilated to wishes and were selected by taking into account some of the sought-after recreational activities among adults. They are reward-related hedonic experiences, but unlike other needs, sensations, and emotions (Paulus, 2007), they can be, in part, inhibited and voluntarily suppressed or postponed, which implies a neocortical representation.
The vignettes clearly expressed needs such as “I'm hungry,” “I'm thirsty,” “I'm sad,” etc., by means of contextual information and in absence any linguistic information. A little cloud contained a representation of the character's wishes or desires. For the more tangible sensations (“I'm hungry,” “I'm hot,” “I'm sleepy,” etc.) unambiguous representations were inserted in the cloud, to make them easily understandable (food, fresh air, a sleeping person, etc.).
To determine how to effectively depict emotional sensations without relying solely on facial expressions, twenty healthy university students aged 19–24 years (10 men and 10 women) were preliminarily interviewed. Stimuli were equiluminant, as assessed by a repeated-measures ANOVAs applied to stimulus luminance values [F (11.44) = 0.41, p = 0.94]. Mean values were: 71.40 cd/m2 for Primary needs, 74.50 cd/m2 for Secondary needs, 70.25 cd/m2 for Somatosensory sensations, and 70.27 cd/m2 for Affective states.
Procedure
To validate the pictogram set, a Google Forms questionnaire was administered to participants, featuring 60 randomly presented illustrations subdivided into six distinct sections. Instructions were: Please indicate to which extent you believe that the illustration efficaciously depicts the person's state. Participants should indicate the motivational state that they deemed most appropriate while also using a 3-point Likert scale (1 = not much, 2 = fairly, 3 = very much) to establish the strength of the association between a pictogram and the defined motivational state. If participants selected the incorrect category, a score of 0 was given (see Figure 1 for some examples of pictograms).
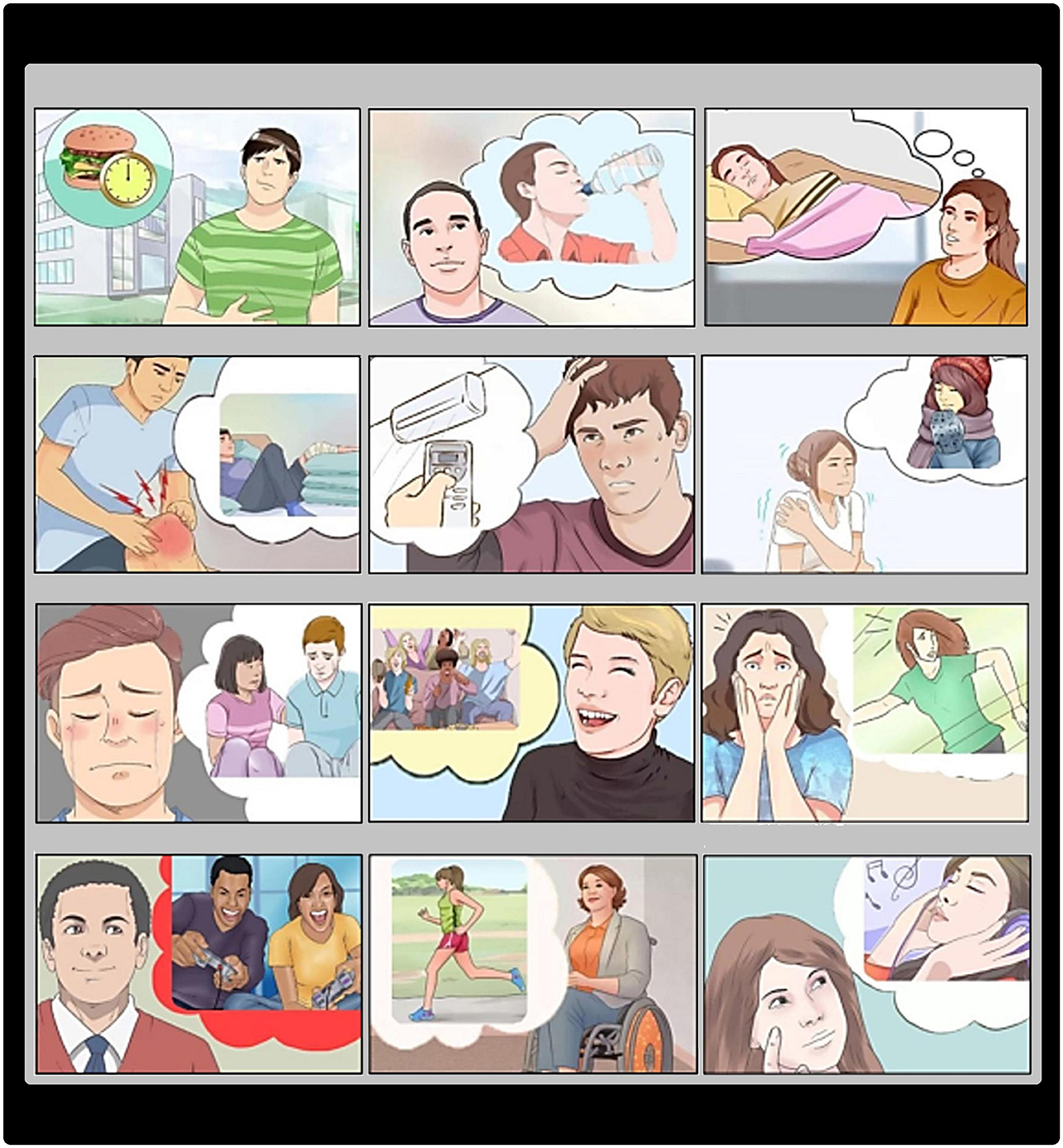
Figure 1. Example of pictograms for the 12 physiological and motivational states (from the top: primary needs, somatosensory sensations, affective states, and secondary needs).
Data analysis
The number of correct responses and Likert scores were processed separately. Individual scores were calculated for each illustration, participant, micro-category, and macro-category. Two 2-way repeated-measures ANOVAs were applied to the individual Likert scores considering the following factors of variability: the between-group factor “sex” (male, female) and the within-group factor “category.” The first ANOVA was performed considering the individual scores obtained for each of the 12 micro-categories, and the second ANOVA was performed considering the mean values obtained for the four macro-categories of motivational states. Statistica10 software (StatSoft.inc) was used for statistical analyses; homoscedasticity was assessed. Post-hoc comparisons between means were performed using Tukey's test.
Results
All stimulus categories were recognized very clearly, reporting averages above “fairly” and close to “very much” ranging from a minimum of 2.55 (SD = 0.57) for “I am afraid,” to a maximum of 2.87 (SD = 0.41) for “I am cold” and “I am in pain.” The mean scores obtained as a function of macro-categories were: 2.69 (SD = 0.43) for “Primary needs”; 2.70, SD = 0.40 for “Secondary Needs”; 2.83 (SD = 0.30) for “Somatosensory sensations”; 2.61 (SD = 0.40) for “Affective states.” The percentage of incorrect categorizations was 1.63%. The percentage of “1” responses indicating not much association (but within the correct category) was 3.73%. The percentage of “2” responses indicating a good association with the correct category was 17.77%. The percentage of “3” responses indicating an extremely good association was 76.8%. The motivational categories showing the relatively lower number of “3” responses were “I am thirsty” and “I am scared,” as can be observed in Figure 2.
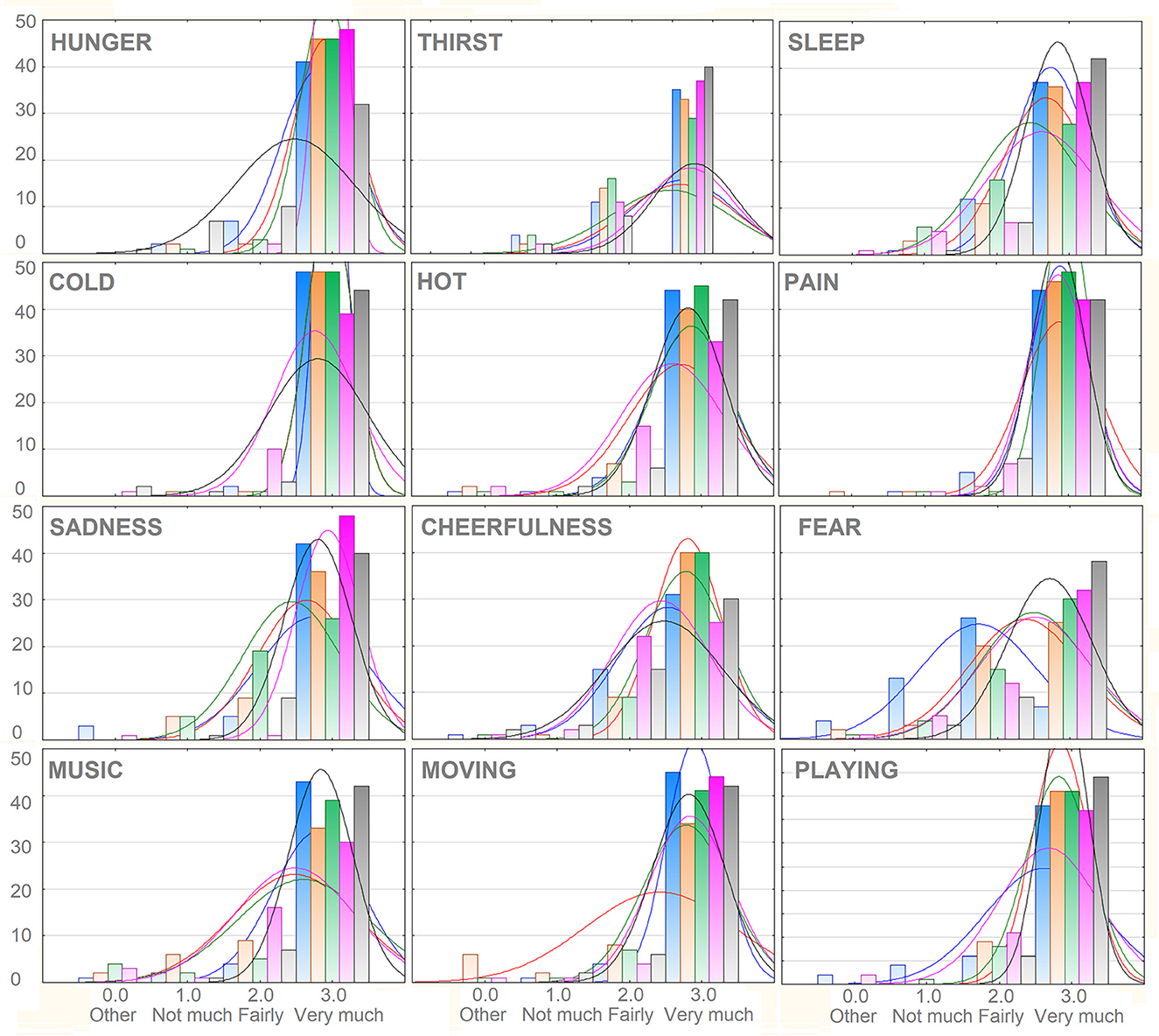
Figure 2. Frequency distribution of responses attributed to the various categories of motivational states. Note that 1, 2, and 3 responses were all assigned to the correct categories, but varied in the strength of the association (1 = not much, 2 = fairly, 3 = very much). The extremely rare 0 response indicated the selection of an incorrect category. On the y-axis is displayed the number of observations.
ANOVA on motivational micro-categories
The ANOVA did not show a significant effect of “Gender” factor [F (1.48) = 3.63, p = 0.06], but a tendency for female participants to recognize affective or motivational states (especially fear and sadness) more clearly than males. In contrast, the “micro-category” was a significant factor [F (7.336) = 5.75, p < 0.001, partial square Eta = 0.10]. Post-hoc comparisons showed higher (p < 0.05) scores for “feeling pain,” “feeling cold” and “feeling hungry” than “feeling fear,” and “thirsty” (see Figure 3).
ANOVA on motivational macro-categories
The repeated-measures ANOVA applied to macro-categories did not show a significant effect for the between-subjects factor “Gender” [F (1.48) = 3.63, p = 0.06]. In contrast, the within-subjects “Macro-categories” factor was significant [F (2.63, 126.5) = 8.60, p < 0.001, Partial Square Eta =0.15]. Post-hoc comparisons showed relatively higher scores for “Somatosensory sensations” than other macro-categories.
Discussion
The results of this study demonstrate the validity of this Pictionary, which was tested here on a group of healthy adult individuals. Behavioral data analysis showed an extremely high rate of correct categorizations (98.4% accuracy) for the 12 motivational states considered. Perceived relatedness with the chosen category was scored, on average, 2.7 on a 0-to-3 scale. The best comprehended set of pictograms included somatosensory sensations (such as “Being in pain” or “Being cold”), while slightly lower scores were attributed to the affective state of “Being afraid” and the primary need of “Being thirsty.” Overall, all stimulus categories were recognized very clearly, reporting scores above “fairly” comprehensible and close to “much” comprehensible for all 50 tested participants.
These data refer to a group of healthy people with intact brains, but are extremely encouraging in indicating the realistic possibility that PAIN set could be used for communicating with adult individuals with LIS incapable of speaking, moving, or understanding verbal commands (Mak et al., 2012). It could be particularly useful for BCI systems based on P300 or N400 detection (McCane et al., 2015) or eye-tracker systems (Poletti et al., 2017). A substantial number of patients who survive severe brain injury enter a non-responsive state of wakeful unawareness, referred to as a vegetative state. They appear to be awake but show no signs of awareness of themselves or their environment according to clinical examinations. Recent neuroimaging research has demonstrated that some vegetative state patients can respond to commands by willfully modulating their brain activity according to instructions (Owen et al., 2006; Monti et al., 2010; Sun and Zhou, 2010). For example, Kujawa et al. (2021) assessed the linguistic capabilities of a group of patients with unresponsive wakefulness syndrome caused by sudden circulatory arrest. They were asked to point to the picture they heard called (for example, “thermometer”) and to choose the related pictogram among other objects. Patients were able to perform approximately 70% of the linguistics tasks administered.
The advantage of using pictograms over verbal language is that pictograms do not require knowledge of a specialized language (e.g., ASL), specialized equipment (e.g., a digital glove), or human translator (e.g., virtual reality systems) (Wołk et al., 2017). They can be used to communicate with adults using artificial airways in intensive care (Manrique-Anaya et al., 2021) or patients with non-standard linguistic competence (Pahisa-Solé and Herrera-Joancomartí, 2019). This was shown in an interesting investigation, where a set of six pictures of familiar objects was correctly classified with an AI classificator based on the P300 response in a small group of healthy subjects and three post-stroke people with an accuracy of 91.79% and 89.68% for healthy and disabled subjects, respectively (Cortez et al., 2021). Higher levels of accuracy were obtained in healthy controls by Proverbio and coworkers by automatically classifying electrical signals relative to 12 perceptual categories by means of statistical analyses (Proverbio et al., 2023), and supervised machine-learning systems (Leoni et al., 2021, 2022) applied to ERPs (Leoni et al., 2021) and EEG single-trials (Leoni et al., 2022). Apart from this literature, we are not aware of BCI studies currently using pictorial communication systems to investigate sensations, volitional and affective states. This Pictionary might be usefully tested on individuals with LIS to assess their ability to process information, and to have a direct insight into their mental states. It would be a great technological advance to understand their otherwise unexpressed needs (e.g., for pain relief, comfort, etc.). Very recently, Proverbio and Pischedda (2023) have successfully used the PAIN set to record ERP signals during imagination of the 12 microstates in healthy adults. They found that frontal N400 component acted as a clear marker of subjects' imagined physiological needs and motivational states, especially for cold, pain, and fear states, but also sadness and the urgency to move, which could signal life-threatening conditions. The data suggests that EEG/ERP markers might potentially allow the reconstruction of mental representations related to various motivational states through BCI systems. Automatic machine-learning classification systems are now being applied to the PAIN-generated EEG data set.
A possible limitation of the PAIN set, as an AAC tool, is that it might be too complex to understand for patients with neurological disorders. Indeed, people who lose their speech might not able to work with too complicated picture systems, containing too many details, and/or needing a high level of skills in abstraction, combination and association. However, the Pictionary was primarily aimed at developing BCI systems for detecting EEG signatures of inner motivational states, not requiring picture processing, at a later stage of experimentation.
In conclusion, the present study provides the validation of a totally unprecedented 60-table Pictionary for assessing 12 sub-categories of physiological or psychological needs (primary visceral needs, somatosensory homeostatic needs, affective states and secondary needs). It allows the generation of category-specific imagery for the development of EEG/ERP-based BCI systems for communicating with individuals with LIS.
The PAIN set should also be validated, by further studies, as an AAC tool in different populations. For example: on patients suffering from consciousness or motor disorders such as children with cerebral palsy (e.g., O'Neill and Wilkinson, 2020), individuals with amyotrophic lateral sclerosis (ALS), multiple sclerosis, muscular dystrophy, brain or spinal cord injury, and brainstem stroke patients, to assess their ability to process information, and to have a direct insight into their physiological and motivational state. For this reason, we are making the PAIN set freely available to scholars, colleagues and scientists interested in validating it on clinical populations.
Data availability statement
The original contributions presented in the study are included in the article/supplementary material, further inquiries can be directed to the corresponding author.
Ethics statement
The studies involving human participants were reviewed and approved by Ethic Committee of University of Milano-Bicocca. The patients/participants provided their written informed consent to participate in this study.
Author contributions
AP designed the study and wrote the paper. FP created the stimuli and gathered the data. All authors performed data analysis and contributed to data interpretation.
Acknowledgments
The authors are very grateful to Alessandra Brusa for her technical assistance.
Conflict of interest
The authors declare that the research was conducted in the absence of any commercial or financial relationships that could be construed as a potential conflict of interest.
Publisher's note
All claims expressed in this article are solely those of the authors and do not necessarily represent those of their affiliated organizations, or those of the publisher, the editors and the reviewers. Any product that may be evaluated in this article, or claim that may be made by its manufacturer, is not guaranteed or endorsed by the publisher.
References
Azinfar, L., Amiri, S., Rabbi, A., and Fazel-Rezai, R. (2013). “A review of P300, SSVEP, and hybrid P300/SSVEP brain- computer interface systems,” in Brain-Computer Interface Systems—Recent Progress and Future Prospects, ed R. Fazel-Rezai (InTech Open books). doi: 10.1155/2013/187024
Bobrov, P., Frolov, A., Cantor, C., Fedulova, I., Bakhnyan, M., and Zhavoronkov, A. (2011). Brain-computer interface based on generation of visual images. PLoS ONE 6, e20674. doi: 10.1371/journal.pone.0020674
Bondy, A., and Frost, L. (1994). The picture exchange communication system. Focus Aut. Other Develop. Disabil. 9, 1–19. doi: 10.1177/108835769400900301
Bywaters, M., Andrade, J., and Turpin, G. (2004). Intrusive and non-intrusive memories in a non-clinical sample: The effects of mood and affect on imagery vividness. Memory 12, 467–478. doi: 10.1080/09658210444000089
Clawson, T. H., Leafman, J., Nehrenz, G. M., and Kimmer, S. (2012). Using pictograms for communication. Mil. Med. 177, 291–295. doi: 10.7205/MILMED-D-11-00279
Cortez, S. A., Flores, C., and Andreu-Perez, J. (2021). “A smart home control prototype using a P300-based brain–computer interface for post-stroke patients,” in eds Y. Iano and R. Arthur. Proc. 5th Brazilian Technology Symposium. (Cham: Springer). doi: 10.1007/978-3-030-57566-3_13
Fan, J., Wade, J. W., Key, A. P., Warren, Z. E., and Sarkar, N. (2018). EEG-based affect and workload recognition in a virtual driving environment for ASD intervention. IEEE Transact. Bio-Med. Engin. 65, 43–51. doi: 10.1109/TBME.2017.2693157
Gracheva, E. O., and Bagriantsev, S. N. (2021). Sensational channels. Cell 184, 6213–6216. doi: 10.1016/j.cell.2021.11.034
Gu, S., Wang, F., Cao, C., Wu, E., Tang, Y. Y., and Huang, J. H. (2019). An integrative way for studying neural basis of basic emotions with fMRI. Front Neurosci. 19, 13:628. doi: 10.3389/fnins.2019.00628
Guy, V., Soriani, M. H., Bruno, M., Papadopoulo, T., Desnuelle, C., and Clerc, M. (2018). Brain computer interface with the P300 speller: usability for disabled people with amyotrophic lateral sclerosis. Ann. Phys. Rehabil. Med. 61, 5–11. doi: 10.1016/j.rehab.2017.09.004
Jin, J., Allison, B. Z., Kaufmann, T., Kübler, A., Zhang, Y., Wang, X., et al. (2012). The changing face of P300 BCIs: a comparison of stimulus changes in a P300 BCI involving faces, emotion, and movement. PLoS ONE 7, e49688. doi: 10.1371/journal.pone.0049688
Kaufmann, T., Schulz, S. M., Köblitz, A., Renner, G., Wessig, C., and Kübler, A. (2013). Face stimuli effectively prevent brain—computer interface inefficiency in patients with neurodegenerative disease. Clin. Neurophysiol. 124, 893–900. doi: 10.1016/j.clinph.2012.11.006
Kavanagh, D. J., Andrade, J., and May, J. (2005). Imaginary relish and exquisite torture: the elaborated intrusion theory of desire. Psychol. Revi. 112, 446–467. doi: 10.1037/0033-295X.112.2.446
Kujawa, K., Zurek, G., Kwiatkowska, A., Olejniczak, R., and Zurek, A. (2021). Assessment of language functions in patients with disorders of consciousness using an alternative communication tool. Front Neurol. 12, 684362. doi: 10.3389/fneur.2021.684362
Leoni, J., Strada, S., Tanelli, M., Jiang, K., Brusa, A., and Proverbio, A. (2021). Automatic stimuli classification from ERP data for augmented communication via brain-computer interfaces. Expert Sys. Appl. 184, 5572. doi: 10.1016/j.eswa.2021.115572
Leoni, J., Tanelli, M., Strada, S., Brusa, A., and Proverbio, A. M. (2022). Single-trial stimuli classification from detected P300 for augmented brain-computer interface: a deep learning approach. Mach. Learn. Appl. 9, 100393. doi: 10.1016/j.mlwa.2022.100393
Mak, J. N., McFarland, D. J., Vaughan, T. M., McCane, L. M., Tsui, P. Z., Zeitlin, D. J., et al. (2012). EEG correlates of P300-based brain-computer interface (BCI) performance in people with amyotrophic lateral sclerosis. J. Neural. Eng. 9, 026014. doi: 10.1088/1741-2560/9/2/026014
Manrique-Anaya, Y., Cogollo Milanés, Z., and Simancas Pallares, M. (2021). Transcultural adaptation and validity of a pictogram to assess communication needs in adults with artificial airway in intensive care. Enferm. Intensiva. 32, 198–206. doi: 10.1016/j.enfie.2021.01.002
Marmolejo-Ramos, F., Hellemans, K., Comeau, A., Heenan, A., Faulkner, A., Abizaid, A., et al. (2015). Event-related potential signatures of perceived and imagined emotional and food real-life photos. Neurosci. Bullet. 31, 317–330. doi: 10.1007/s12264-014-1520-6
Mattioli, F., Porcaro, C., and Baldassarre, G. A. (2022). 1D CNN for high accuracy classification and transfer learning in motor imagery EEG-based brain-computer interface. J Neural Eng. 18, 4430. doi: 10.1088/1741-2552/ac4430
McCane, L. M., Heckman, S. M., McFarland, D. J., Townsend, G., Mak, J. N., Sellers, E. W., et al. (2015). P300-based brain-computer interface (BCI) event-related potentials (ERPs): People with amyotrophic lateral sclerosis (ALS) vs. age-matched controls. Clin. Neurophysiol.126, 2124–2131. doi: 10.1016/j.clinph.2015.01.013
Milanés-Hermosilla, D., Trujillo Codorniú, R., López-Baracaldo, R., Sagaró-Zamora, R., Delisle-Rodriguez, D., Villarejo-Mayor, J. J., et al. (2021). Monte Carlo dropout for uncertainty estimation and motor imagery classification. Sensors. 21, 7241. doi: 10.3390/s21217241
Monti, M. M., Vanhaudenhuyse, A., Coleman, M. R., Boly, M., Pickard, J. D., Tshibanda, L., et al. (2010). Willful modulation of brain activity in disorders of consciousness. N. Engl. J. Med. 36, 2579–89. doi: 10.1056/NEJMoa0905370
Mussabayeva, A., Jamwal, P. K., Tahir, Akhtar, M. (2021). Ensemble learning approach for subject-independent P300 speller. Annu. Int. Conf. IEEE Eng. Med. Biol. Soc. 2021, 5893–5896. doi: 10.1109/EMBC46164.2021.9629679
Naci, L., Monti, M. M., Cruse, D., Kübler, A., Sorger, B., Goebel, R., et al. (2012). Brain-computer interfaces for communication with nonresponsive patients. Ann. Neurol. 72, 312–323. doi: 10.1002/ana.23656
O'Neill, T., and Wilkinson, K. M. (2020). Preliminary investigation of the perspectives of parents of children with cerebral palsy on the supports, challenges, and realities of integrating augmentative and alternative communication into everyday life. Am. J. Speech Lang. Pathol. 29, 238–254. doi: 10.1044/2019_AJSLP-19-00103
Owen, A. M., Coleman, M. R., Boly, M., Davis, M. H., Laureys, S., and Pickard, J. D. (2006). Detecting awareness in the vegetative state. Science 313, 1402. doi: 10.1126/science.1130197
Pahisa-Solé, J., and Herrera-Joancomartí, J. (2019). Testing an AAC system that transforms pictograms into natural language with persons with cerebral palsy. Assist. Technol. 31, 117–125. doi: 10.1080/10400435.2017.1393844
Pak, N. S., Bailey, K. M., Ledford, J. R., and Kaiser, A. P. (2023). Comparing interventions with speech-generating devices and other augmentative and alternative communication modes: a meta-analysis. Am. J. Speech Lang. Pathol. 32, 786–802. doi: 10.1044/2022_AJSLP-22-00220
Panachakel, J. T., and Ramakrishnan, A. G. (2021). Classification of phonological categories in imagined speech using phase synchronization measure. Annu. Int. Conf. IEEE Eng. Med. Biol. Soc. 2021, 2226–2229. doi: 10.1109/EMBC46164.2021.9630699
Paulus, M. P. (2007). Neural basis of reward and craving—A homeostatic point of view. Dialog. Clin. Neurosci. 9, 379–387. doi: 10.31887/DCNS.2007.9.4/mpaulus
Phillips, M. L., Drevets, W. C., Rauch, S. L., and Lane, R. (2003). Neurobiology of emotion perception I: the neural basis of normal emotion perception. Biol. Psychiatry 54, 504–514. doi: 10.1016/S0006-3223(03)00168-9
Poletti, B., Carelli, L., Solca, F., Lafronza, A., Pedroli, E., Zago, S., et al. (2017). An eye-tracking controlled neuropsychological battery for cognitive assessment in neurological diseases. Neurol Sci. 38, 595–603. doi: 10.1007/s10072-016-2807-3
Proverbio, A. M., and Pischedda, F. (2023). Measuring brain potentials of imagination linked to physiological needs and motivational states. Front. Hum. Neurosci. 17:1146789. doi: 10.3389/fnhum.2023.1146789
Proverbio, A. M., Tacchini, M., and Jiang, K. (2023). What do you have in mind? ERP markers of visual and auditory imagery. Brain Cogn. 166, 105954. doi: 10.1016/j.bandc.2023.105954
Sarfo, F. S., Gebregziabher, M., Ovbiagele, B., Akinyemi, R., Owolabi, L., Obiako, R., et al. (2016). Validation of the 8-item questionnaire for verifying stroke free status with and without pictograms in three West African languages. eNeurologicalSci 3, 75–79. doi: 10.1016/j.ensci.2016.03.004
Sun, F., and Zhou, G. (2010). Willful modulation of brain activity in disorders of consciousness. N. Engl. J. Med. 362, 1937. doi: 10.1056/NEJMc1003229
Tucker, D. M., and Luu, P. (2021). Motive control of unconscious inference: the limbic base of adaptive Bayes. Neurosci. Biobehav. Rev. 128, 328–345. doi: 10.1016/j.neubiorev.2021.05.029
Van der Meer, L. A., and Rispoli, M. (2010). Communication interventions involving speech-generating devices for children with autism: a review of the literature. Dev. Neurorehabil. 13, 294–306. doi: 10.3109/17518421003671494
Van Diest, I., Winters, W., Devriese, S., Vercamst, E., Han, J. N., Van de Woestijne, K. P., et al. (2001). Hyperventilation beyond fight/flight: respiratory responses during emotional imagery. Psychophysiology 38, 961–968. doi: 10.1111/1469-8986.3860961
Vanhaudenhuyse, A., Bruno, M., Brédart, S., Plenevaux, A., and Laureys, S. (2007). The challenge of disentangling reportability and phenomenal consciousness in post-comatose states. Behav. Brain Sci. 30, 529–530. doi: 10.1017/S0140525X0700310X
Wendt, O., Hsu, N., Simon, K., Dienhart, A., and Cain, L. (2019). Effects of an iPad-based speech-generating device infused into instruction with the picture exchange communication system for adolescents and young adults with severe autism spectrum disorder. Behav. Modif. 43, 898–932. doi: 10.1177/0145445519870552
Wołk, K., Wołk, A., and Glinkowski, W. (2017). A cross-lingual mobile medical communication system prototype for foreigners and subjects with speech, hearing, and mental disabilities based on pictograms. Computat. Math. Methods Med. 2017, 4306416. doi: 10.1155/2017/4306416
Wolpaw, J. R., Birbaumer, N., McFarland, D. J., Pfurtscheller, G., and Vaughan, T. M. (2002). Brain-computer interfaces for communication and control. Clin. Neurophysiol. 113, 767–791. doi: 10.1016/S1388-2457(02)00057-3
Keywords: communication, locked-in patients, mental imagery, brain computer interface, LIS (locked-in state)
Citation: Proverbio AM and Pischedda F (2023) Validation of a pictionary-based communication tool for assessing physiological needs and motivational states: the PAIN set. Front. Cognit. 2:1112877. doi: 10.3389/fcogn.2023.1112877
Received: 30 November 2022; Accepted: 03 April 2023;
Published: 20 April 2023.
Edited by:
István Czigler, Hungarian Academy of Sciences (MTA), HungaryReviewed by:
Sophia Kalman, Hungarian Bliss Foundation, HungaryMariana P. Branco, University Medical Center Utrecht, Netherlands
Copyright © 2023 Proverbio and Pischedda. This is an open-access article distributed under the terms of the Creative Commons Attribution License (CC BY). The use, distribution or reproduction in other forums is permitted, provided the original author(s) and the copyright owner(s) are credited and that the original publication in this journal is cited, in accordance with accepted academic practice. No use, distribution or reproduction is permitted which does not comply with these terms.
*Correspondence: Alice Mado Proverbio, bWFkby5wcm92ZXJiaW9AdW5pbWliLml0