- 1Caesar Kleberg Wildlife Research Institute, Texas A&M University-Kingsville, Kingsville, TX, United States
- 2East Foundation, San Antonio, TX, United States
Reliable estimates of population density and size are crucial to wildlife conservation, particularly in the context of the Endangered Species Act. In the United States, ocelots (Leopardus pardalis pardalis) were listed as endangered in 1982, and to date, only one population density estimate has been reported in Texas. In this study, we integrated vegetation metrics derived from LiDAR and spatial capture-recapture models to discern factors of ocelot encounter rates and estimated localized population estimates on private ranchlands in coastal southern Texas. From September 2020 to May 2021, we conducted a camera trap study across 42 camera stations on the East Foundation’s El Sauz Ranch, which was positioned within a larger region of highly suitable woody and herbaceous cover for ocelots. We observed a high density of ocelots (17.6 ocelots/100 km2) and a population size of 36.3 ocelots (95% CI: 26.1–58.6) with the 206.25 km2 state space area of habitat. The encounter probability of ocelots increased with greater canopy cover at 1-2 m height and decreasing proximity to woody cover. These results suggest that the incorporation of LiDAR-derived vegetative canopy metrics allowed us to understand where ocelots are likely to be detected, which may aid in current and future population monitoring efforts. These population estimates reflect the first spatially explicit and most recent estimates in a portion of the northernmost population of ocelots in southern Texas. This study further demonstrates the importance of private working lands for the recovery of ocelots in Texas.
1. Introduction
In North America, primarily, in the United States, research and conservation on threatened and endangered species occur on both public and private lands. Of the two, private lands have been identified as holding immense conservation value; over 90% of listed species on the Endangered Species Act list occur on private lands (Bennett et al., 2018). Private lands equate to 71% of the known landmass in the United States (Hilty and Merenlender, 2003), with states like Texas as high as 97% (Haines et al., 2006a; Lombardi et al., 2020a). Bennett et al. (2018) reported that more than 60% of threatened and endangered species occur on private lands. Working on private lands can contribute to a better understanding of landscape resiliency (Samson et al., 2021, Donnelly et al., 2016), habitat or genetic connectivity (Hooker et al., 2021; Veals et al., 2022), and habitat preservation and restoration efforts (Burger et al., 2019; Cortez, 2021; Kobilinsky, 2022). Recovery planning for endangered species, however, requires knowledge of the best available science and if areas are not surveyed, may lead to inadequate information and strategies (Hilty and Merenlender, 2003; Burger et al., 2019).
Reliable estimates of population density and size are important objectives in the conservation and management of wildlife populations, especially in the context of the Endangered Species Act (Sharma et al., 2010; Sollmann et al., 2013; McGowan et al., 2017). Incorrect or speculative population estimates owing to incomplete survey information, limited land access, and geopolitical obstacles can lead to misinterpretations of population status, viability assessments, and extinction risk, which can impede conservation efforts (López-Bao et al., 2018). An established species recovery plan often requires that minimum population thresholds be met for a certain number of years before petitioning for delisting or changing species status (e.g., threatened, endangered, and critically endangered). Frequently, population density estimates are used as a baseline estimate of population size to inform species status in imperiled areas of their geographic range and to inform conservation strategies (Greenspan et al., 2020).
Conventional capture-recapture models have been widely used to estimate species abundance and population density (Otis et al., 1978; Sharma et al., 2010). Carnivores are difficult to sample due to their reclusive and cryptic behavior, large home ranges, and naturally occurring low densities (Sollmann et al., 2013; Hunter, 2015; Greenspan et al., 2020). Camera traps have been used since the 1990s to estimate density, and population size of cryptic and easily identifiable carnivorans with unique pelage patterns (e.g., felids) (Karanth and Nichols, 1998; Haines et al., 2006a; Brodie and Giordano, 2012; Kittle et al., 2018, Satter et al., 2018). Traditional capture-recapture (CR) frameworks required researchers to determine an ad hoc effective sampling area to produce density estimates, which often resulted in positively biased estimates (Otis et al., 1978; Lewis et al., 2015). Contrary to traditional CR models, SECR models explicitly consider the spatial nature of sampling and populations, as well as the movements of individuals off the trapping grid; consequently, SECR-based density estimates do not require an ad-hoc definition of effective sampled areas. Population density estimates from SECR frameworks have since been shown to produce lower estimates, but with higher precision than estimates conducted previously in the same regions (Lewis et al., 2015; Satter et al., 2019). These analyses allow researchers to examine the influence of environmental variables on density or encounter parameters, which can aid in informing management and recovery actions (Qi et al., 2015; Gogoi et al., 2020; Rather et al., 2021).
In North America, there has been a contraction in the geographical range of carnivores, of which, over 40% have suffered range contractions of over 20% (Laliberte and Ripple, 2004). Within the United States, Texas historically supported a robust carnivore guild of more than 20 carnivores with five felid species: bobcats (Lynx rufus), puma (Puma concolor), jaguar (Panthera onca), jaguarundi (Puma yagouaroundi), and ocelot (Lombardi et al., 2020a). Habitat loss and fragmentation due to agriculture, energy, and urban development coupled with historic large-scale predator control, contributed to the extirpation of many species (Laliberte and Ripple, 2004; Leslie, 2016; Lombardi et al., 2020a). Today, the only felids remaining in Texas are pumas, bobcats, and ocelots (Leslie, 2016; Lombardi et al., 2020a).
Ocelots are medium-sized felids with an extensive geographic range that stretches from northern Uruguay to Texas, with populations in Texas separated from populations in northeastern Mexico by >150 km (Lombardi et al., 2022). According to the IUCN Red List, ocelots are classified as Least Concern across their geographic range, but regional population statuses are highly variable. In South America, ocelots are listed as Vulnerable in Brasil, Colombia, and Argentina, and in North America, they are listed as Endangered in Mexico and the United States (Hunter, 2015; International Union for the Conservation of Nature 2022). Ocelots occur in heterogenous forested landscapes and often select for areas of mixed and dense forest and vegetation (Hunter, 2015; Wang et al., 2019; Lombardi et al., 2021; Lombardi et al., 2022; Veals et al., 2022). Further, their niche space has been debated with some referring to them as generalists (Paolino et al., 2018, Moreno-Sosa et al., 2022) and others as specialists (Shindle and Tewes, 1998; Haines et al., 2006a; Horne et al., 2009). Common vegetation communities they occur in include tropical broadleaf (Wang et al., 2019; Satter et al., 2019), semi-arid thorn and dry forest (Lombardi et al., 2022), pine (Pinus spp.) – Oak (Quercus spp.) woodlands (Gomez-Ramirez et al., 2017), and dense herbaceous cover (Paolino et al., 2018, Pasa et al., 2021). Ocelot population densities range from 0.51 to 25 ocelots/100 km2 across their geographic range (Pérez-Irineo and Santos-Moreno, 2014; Martínez-Hernández et al., 2015; Penido et al., 2016; Satter et al., 2019; Lombardi et al., 2022). To date, only Haines et al. (2006b) estimated population density (0.30 ocelots/km2) in Texas, however, estimates were based on traditional CR approaches and did not include population size.
Historically, ocelots occupied much of Texas, with potentially larger population sizes, however, populations declined in the 20th century due to loss of habitat and predator trapping efforts (Frye and Lay 1942, U. S. Fish and Wildlife Service, 2016). Ocelots were initially listed on the Texas Endangered Species List in 1973 and listed as federally endangered in 1982 (U. S. Fish and Wildlife Service, 2016) with the first field studies undertaken in 1982 (Tewes, 1986). In the mid-1980s, it was estimated that fewer than 120 ocelots remained in Texas, but no formal population size estimates were made at the time. Over the last 40 years, subjective estimates of population size have fluctuated from 80 to 120, less than 100, and more recently less than 80 (Tewes, 1986; Haines et al., 2006b; U. S. Fish and Wildlife Service, 2016; Lombardi et al., 2021; Veals et al., 2022). Today, ocelots exist in two isolated breeding populations in coastal southern Texas with no known genetic exchange between the two populations (Janecka et al., 2016). The Ranch Ocelot Population (35-50; based on known individuals) occurs on at least three privately-owned ranchlands of extensive thorn and oak forests (Lombardi et al., 2021). The smaller, Refuge Ocelot Population (16-20 based on known individuals; H. Swarts, USFS pers. com), is located 25 km south of Laguna Atascosa National Wildlife Refuge and surrounded by extensive agriculture and urban development (Veals et al., 2022). Inbreeding depression and loss of genetic diversity (Janecka et al., 2016), land conversion (Lombardi et al., 2020a; Veals et al., 2022), and road mortality (Blackburn et al., 2021) have continued to negatively affect ocelot populations in Texas. Currently, there has yet to be an updated formal analysis using SECR models to estimate local population density and population size in any part of the ocelot’s geographic range in Texas.
In this study, we used camera trap data in a SECR framework to estimate population density and provide an estimate of population size in a portion of the northernmost population of ocelots on private working ranchlands. Additionally, we extracted environmental data using remotely sensed light detection and ranging (LiDAR) imagery to determine their potential impact on ocelot encounter probability. Results of this analysis will aid ongoing population monitoring efforts and inform concurrent reintroduction habitat suitability and population viability studies to support ocelot recovery in the United States.
2. Material and methods
2.1. Study system
We conducted this study within a portion of the Ranch Ocelot Population on the East Foundation’s El Sauz Ranch (113 km2), which was managed for cattle, wildlife conservation, and land stewardship in southeastern Kenedy and northeastern Willacy counties in southern Texas. The ranch is located on the confluence of two major eco-regions; the Coastal Sand Plain and Lower Rio Grande Valley Eco-regions with a regional semi-arid and subtropical climate that ranges from as low as 10° C in winter to highs greater than 40° C in the summer (Norwine and Kuruvilla, 2007; Lombardi et al., 2021). The ranch has been managed for cattle for over 150 years and functions as a living laboratory with multiple concurrent ecological studies occurring in different areas of the ranch. Anthropogenic infrastructure on the ranch includes a network of caliche and dirt ranch roads, an outdoor education center, and ranch headquarters.
The ranch has a diverse composition of land cover types including woody and herbaceous vegetation communities, large parabolic inland and coastal dunes, expansive cordgrass (Spartina alterniflora), and coastal prairie palustrine emergent and woody wetlands (Lombardi et al., 2020b). Woody vegetation dominates the western third of the ranch and transitions from live oak (Quercus virginiana) forest with thornshrub and guinea grass (Megathyrus maximus) understories in the northwest to thornshrub-cordgrass-wetland playas in the southwest. The transition zone between the two areas contains a mosaic of playas surrounded by cordgrass, guinea grass, mesquite (Prosopis glandulosa), and many thornshrub species. Woody communities include extensive isolated mottes and large patches of live oak forest (including remnant old-growth stands), old-growth palm (Sabal spp.) forest, and diverse low to mid-story shrub communities. Low- to mid-story shrub communities included lime prickly ash (Zanthoxylum fagara), white brush (Aloysia gratissma), huisache (Acacia smaliii), catclaw acacia (Acacia greggi), ebony (Ebenopsis ebano), brasil (Condalia hookeri), desert olive (Forestiera angustifolia), crucifixion thorn (Castela emoryi), and American beautyberry (Callicarpa americana). Cactuses include cholla (Opuntia imbricata), prickly pear (Oputia spp.), and tasajillo (Opuntia leptocaulis) (Lombardi et al., 2021).
2.2. Camera surveys
We conducted camera trap surveys for ocelots as part of an ongoing long-term population monitoring effort on the El Sauz Ranch (Figure 1). From September 2020 to May 2021, we monitored an established camera trap array of 42 camera stations across the western half of the ranch. This large camera trap array represents an expansion of an initial 28-camera station array originally used from 2011 to early 2020 (Lombardi et al., 2020b; Lombardi et al., 2022). The camera array used in this study extended into isolated patches of forested cover and the habitat transition zones to include areas of marginal landscape suitability for ocelots (Lombardi et al., 2021). Habitats surveyed in this expanded camera trap array included open to mixed canopies of live oak forest with thornshrub and grassy understories, palm-thornshrub, mesquite-thornshrub woodlands, cordgrass prairie with live oak mottes, and woody wetlands adjacent to nearby patches of thornshrub. This camera array was designed using a systematic 1x1 km grid-based sampling with a randomized point within each grid cell (Lombardi et al., 2020b); we established 1 camera trap station at each randomized point and maintained at least 1 km distance between each camera station (Lombardi et al., 2020b).
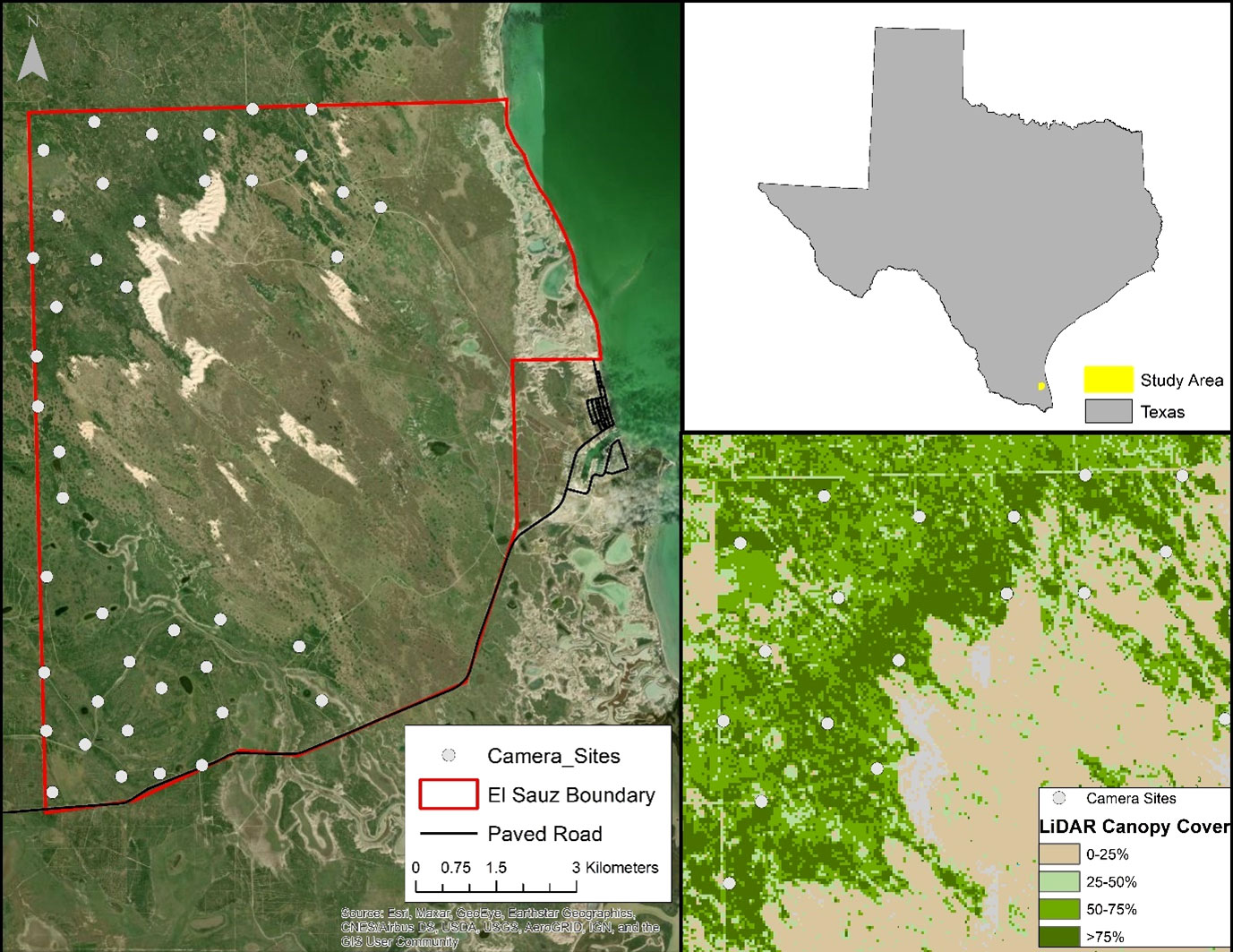
Figure 1 Map of camera trap array (n = 42 stations; left panel) to determine population density and population size of ocelots Leopardus pardalis pardalis) on the East Foundation’s El Sauz Ranch in Willacy and Kenedy counties in southern Texas (upper-right panel) from September 2020 to May 2021. The lower-right panel depicts an example of the zoomed-in view of the LiDAR-derived woody and herbaceous canopy cover surrounding the camera trap array.
At each location, we established a camera trap station of two Reconyx passive infrared PC900 camera traps (Reconyx, Holmen, WI, USA) (Lombardi et al., 2020b). We programmed each camera trap on a 1-minute delay between trigger events and RAPIDFIRE© burst of two photographs per detection event with high sensitivity. Camera traps were attached to trees or wooden stakes and positioned 20-30 cm above the ground. We offset each pair of cameras 1-2 m to allow for individual identification of pelage patterns (Lombardi et al., 2020b; Lombardi et al., 2022). Paired camera traps were used to aid in obtaining the right and left side photographs of ocelots and were offset to avoid infrared flash interference during the night (Lombardi et al., 2020b).
2.3. Individual identification of ocelots
Individual adult and subadult ocelots were identified based on their unique pelage rosette patterns on their flanks, tails, face, and heads (Gomez-Ramirez et al., 2017; Satter et al., 2019; Lombardi et al., 2022). Ocelots are born with unique pelage patterns that do not change throughout their life (Hunter, 2015). We initially identified ocelots visually by cross-referencing each photograph to a large extensive 10-year ocelot identification database of over >50 previously known individuals from prior live-capture photographs, camera trap photographs, and camera trap video stills from the study area. To confirm the identification of a previously known or new individual, we identified at least three unique spots or rosettes on the ocelot (Figure 2). Due to this extensive photographic historic identification library, we had multiple photographs of previously identified ocelots from multiple angles (i.e., right and left flanks, head, tail, overhead views) that allowed for the identification of individuals (new and old). As an added layer of security, we used HotSpotter (Crall et al., 2013; Nipko et al., 2020), to double-check and confirm our ocelot identities (Lombardi et al., 2022), especially for new individual ocelots. Photographs of kittens or ocelots that could not be identified due to obstructed angle and granular or distorted photographic resolution were removed from further analyses.
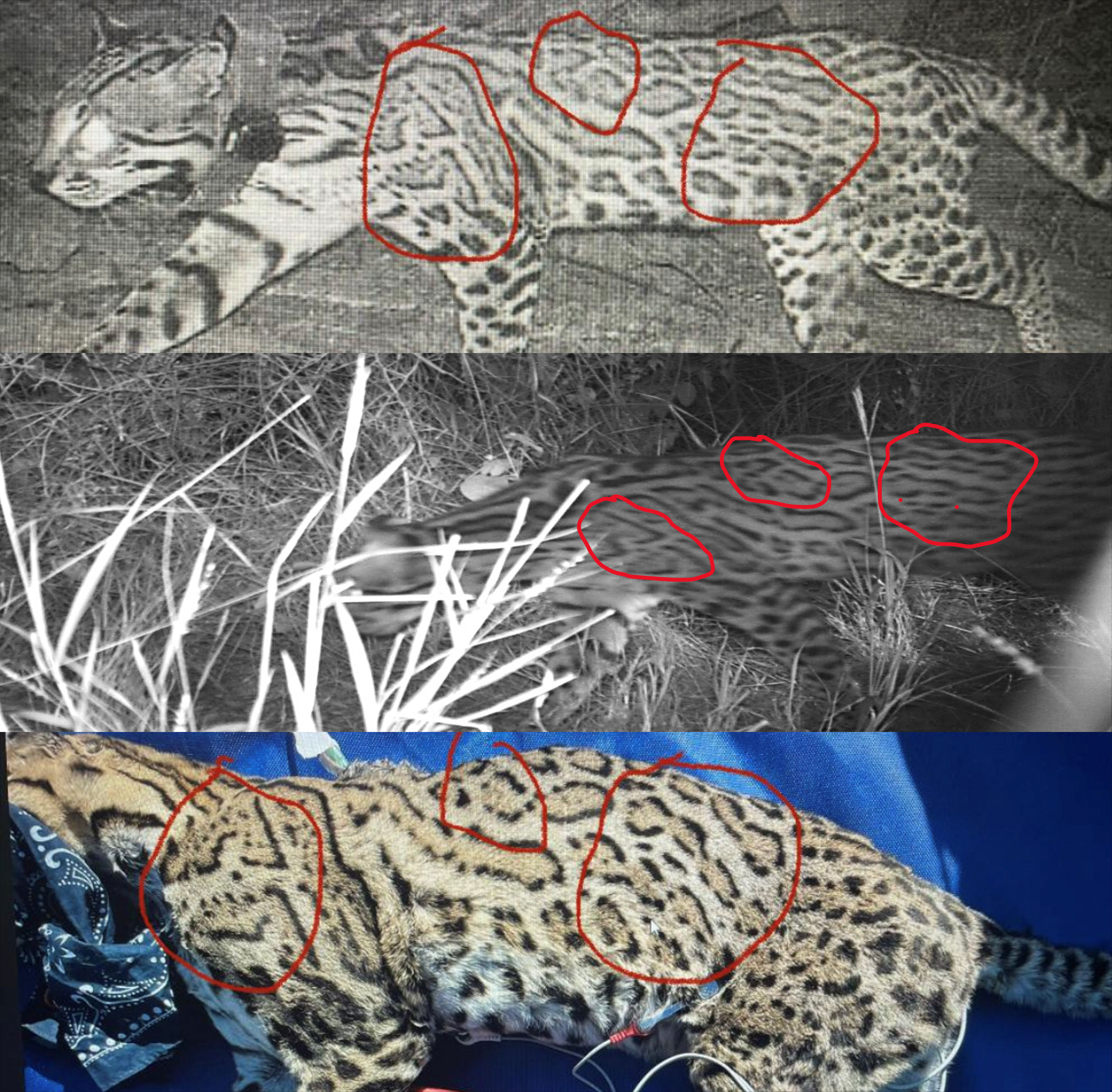
Figure 2 Example of individual ocelot identification using unique pelage patterns of an adult female ocelot (Leopardus pardalis pardalis) based on photographs from ocelot camera trap identification library (top), this study (middle), and live capture (this past year) on the East Foundation’s El Sauz Ranch, Texas in Willacy and Kenedy counties, Texas. Red circles indicate each unique pelage pattern (rosettes and spots) on the side of the upper shoulder, upper back, and hind torso.
2.4. Remote sensing methods
To understand the landscape-level mechanisms that influenced ocelot encounter probabilities, we examined the low-shrub cover (<3 m), and woody canopy height (m). Previous research in southern Texas has indicated ocelots use a larger proportion and area of woody patches, with low shape-index values (Lombardi et al., 2020b), and farther from roads (Veals et al., 2022). Ocelots in areas of southern Texas have shown a preference for dense shrub cover (>75% canopy cover) and low canopy heights (<2 m) (Shindle and Tewes, 1998; Horne et al., 2009) but similar site-level metrics were not found to influence habitat use (Lombardi et al., 2020b). To measure site-level factors, we obtained light detection and ranging (LiDAR) point cloud 0.6 m data flown by the US Geological Survey (Texas Natural Resource Information System, Austin, TX, USA) in 2018. We classified and processed LiDAR point cloud data in Program LP360 (GeoCue, Madison, AL, USA) into six categories: vegetation, bare ground, water, paved roadways, bridges, and buildings at different height stratum (0-1 m, 1-2 m, overall; Sergeyev et al. In Press; Barnes et al., 2016). We used a digital elevation model and a digital surface model and calculated the difference to derive an estimate of canopy height (Sergeyev et al. In Press; Barnes et al., 2016). We derived an estimate of canopy cover at different height stratum by first calculating the vegetation point density of the point-cloud data to quantify the horizontal vegetation structure. Next, we used the vertical structure of the vegetation to estimate the percent canopy cover at each height strata (Barnes et al., 2016). We resampled the data to 30 m and generated a 10 m buffered sampling unit around each camera station and estimated the mean canopy height, and canopy cover at 1 m, 2m, and overall (Table 1).
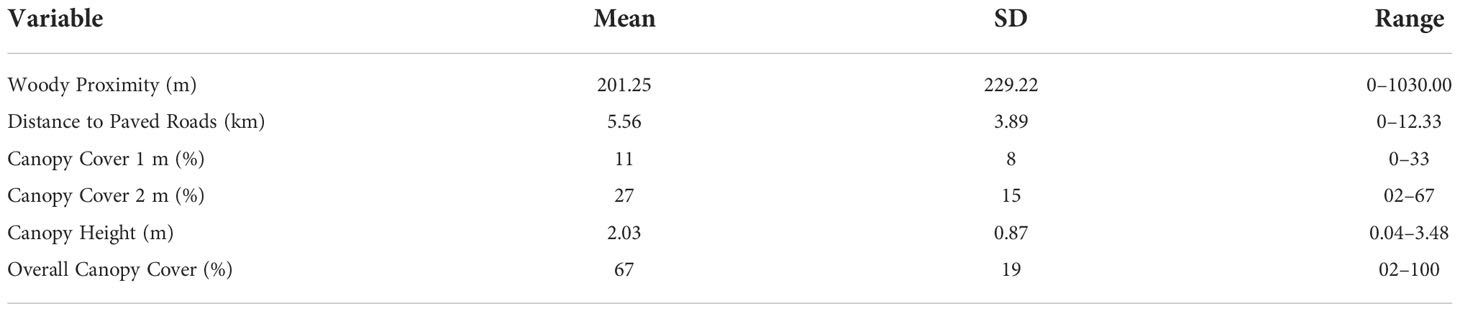
Table 1 Summary of the mean (SD) and range of site-level metrics used to model ocelot (Leopardus pardalis pardalis) encounter probabilities (g0, σ) on the East Foundation’s El Sauz Ranch in southern Texas, USA from September 2020 to May 2021.
We included two additional linear distance metrics to determine if proximity to woody patches and paved roads also affected ocelot encounter rates based on previous ocelot research (Wang et al., 2019; Lombardi et al., 2020b; Veals et al., 2022). We followed the methodology and classification schemes described by Lombardi et al. (2020b) to classify remotely sensed 30 m landsat imagery from the U.S. Geological Survey Global Visualization Viewer (USGS, Location, Access). We used an unsupervised classification in ERDAS Imagine 2021 (Hexagon Geospatial, Norcross, GA, USA) based on six land cover types: woody cover, herbaceous cover, agriculture, urban, water, and bare soil (inland dune, caliche roads, and earthen soil). We assessed the classified image accuracy of our images using a confusion matrix and 200 random points that resulted in a 91.9% accuracy (Lombardi et al., 2020b). In ArcGIS 10.8 (ESRI, Redlands, CA), we measured the linear distance from each camera station to the nearest patch of woody cover (m, Woody Proximity) and the linear distance to the state highway (km, Paved Dist.; Table 1).
2.5. Spatial explicit capture-recapture analysis
We used a single-season spatial capture-recapture model to estimate the population density and size of ocelots. We assumed a closed population during this study period and addressed the potential recruitment through birth by including only adult ocelots in this analysis. We did not include kittens (photographed with their mother) in the analysis. Ocelots have 1 (usual) to 2 kittens, which spend 12-18 months with their mother (Laack et al., 2005; Hunter, 2015). This extended period with their mothers was far beyond the length of our study period. During our study, we knew of only two kittens, both born before the start of the study and stayed with their mothers the entire study duration. We were not aware of any mortalities during the study by known camera-trap or GPS-collared individuals but survival in this local population is potentially high with several known individuals living over 10 years of age (J. Lombardi, unpub. data). We believed that because both recruitment of new individuals into the adult population and mortality of adults are unlikely events it was reasonable to assume population closure over this 9-month study period.
Before analysis, we built an ocelot-photographic capture history and camera trap-covariate matrix. Using our individual identified ocelots, we created a capture history for each individual, dates, and camera station identification of each detected. We defined each occasion based on a 24-hour daily period (00:00-23:59 hr), where we considered the first detection of an individual ocelot at each camera location. Repeat detections of the same individual within 24 hours at the same camera station were removed from the analysis (Lombardi et al., 2022). For each camera station in the camera station-covariate file, we provided a unique station identifier, spatial coordinates (x, y) of each station with the operational status for each 24-hour daily period (1: active; 0: non-active), and the value of each covariate for that station.
We estimated the population density (individual ocelots per 100 km2), population size, and drivers of encounter probabilities using R package secr 4.5.1 (Efford, 2021). For our modeling process, we used a half-normal detection function, and a Bernoulli independent encounter model, which accounts for each individual being detected at most once at a given trap on a given occasion (Efford, 2021). We used the suggest.buffer function in package secr to ensure the state space area encompassed the activity centers of all individuals that have a probability of being detected in the camera trap array and defined a 3500 m buffer for the state space area for ocelots (Efford, 2021). We then created a habitat mask for the state space area using a spatial polygon of the study area which excluded areas of non-habitat (i.e., inland parabolic dunes, agriculture, and a paved roadway) (Efford, 2021). The state space (206.25 km2) extended beyond the boundaries of the ranch. These areas beyond the ranch are characterized by high woody landscape suitability (Lombardi et al., 2021), high connectivity (Veals, 2021), and high probability of use based on GPS collars (Veals et al., 2022) and should thus support similar ocelot densities. We also considered cordgrass areas east of our study area, because ocelots have been observed moving through herbaceous areas to access woody cover in Texas (Veals et al., 2022; Sergeyev et al. In Press) and other parts of their range (Grasiela et al., 2018; Paolino et al., 2018). Before constructing our density models, we used Pearson’s correlation analysis to examine correlation(s) among distance-based and LiDAR-derived metrics. We considered and grouped non-correlated variables (|r| < 0.70) to be used in our SECR modeling process based on ocelot ecology (Lombardi et al., 2020b; Lombardi et al., 2022, Supplemental Material Table 1). To ensure model convergence and comparability across models, we scaled covariates in our camera station matrix.
For the modeling process, we wanted to identify the drivers of ocelot encounter probability (g0) and movement parameter (σ). In this context g0 is defined as the probability of detecting an ocelot with a home range center located at the camera station, σ is defined as the decay rate of the encounter probability over distance (m) from the home range center. Because we were not interested in variations in density (D), we assumed a fixed density parameter (D~1). We followed a stepwise modeling approach for detection parameters (g0 and σ), to discern whether detection varied based on sex-specific variation, behavior, and environmental variation. Before building any environmental variation models, we first assessed whether there were sex-specific variations in g0 and/or σ. Initial modeling revealed detection did not vary by sex and we then focused the modeling approach to examine other drivers of g0 (Table 2). We next built four grouped candidate models to examine whether g0 varied by site-level non-correlated vegetation and distance-based variables. In each of these models, we included overall canopy cover and canopy height. However, due to high correlation, we separated 0-1 m and 1-2 m canopy cover and each distance-based variable. As a result, we defined the following models: 1) overall and 0-1 m canopy cover, canopy height and woody proximity, 2) overall and 0-1 m canopy cover, canopy height and paved distance, 3) overall and 1-2 m canopy cover, canopy height, and paved distance, and 4) overall and 1-2 m canopy cover, canopy height, and woody proximity. Based on recent studies (Satter et al., 2019; Lombardi et al., 2022) that indicate that a behavioral response to camera traps may influence ocelot encounter probability, we also incorporated behavior in each of these four models, for a total of eight additional grouped models that considered additive effects of behavior. The two trap-level behavior variables considered included a transient trap response (Bk; different encounter probability only in the occasion immediately following a detection event) and permanent trap response (bk; different encounter probability on all occasions following the first capture). We evaluated candidate models using an AICc model selection framework and considered competing models with an ∆AICc ≤2.00 (Burnham and Anderson, 2002). For each parameter (D, g0, σ), we present parameter estimates with 95% confidence intervals.
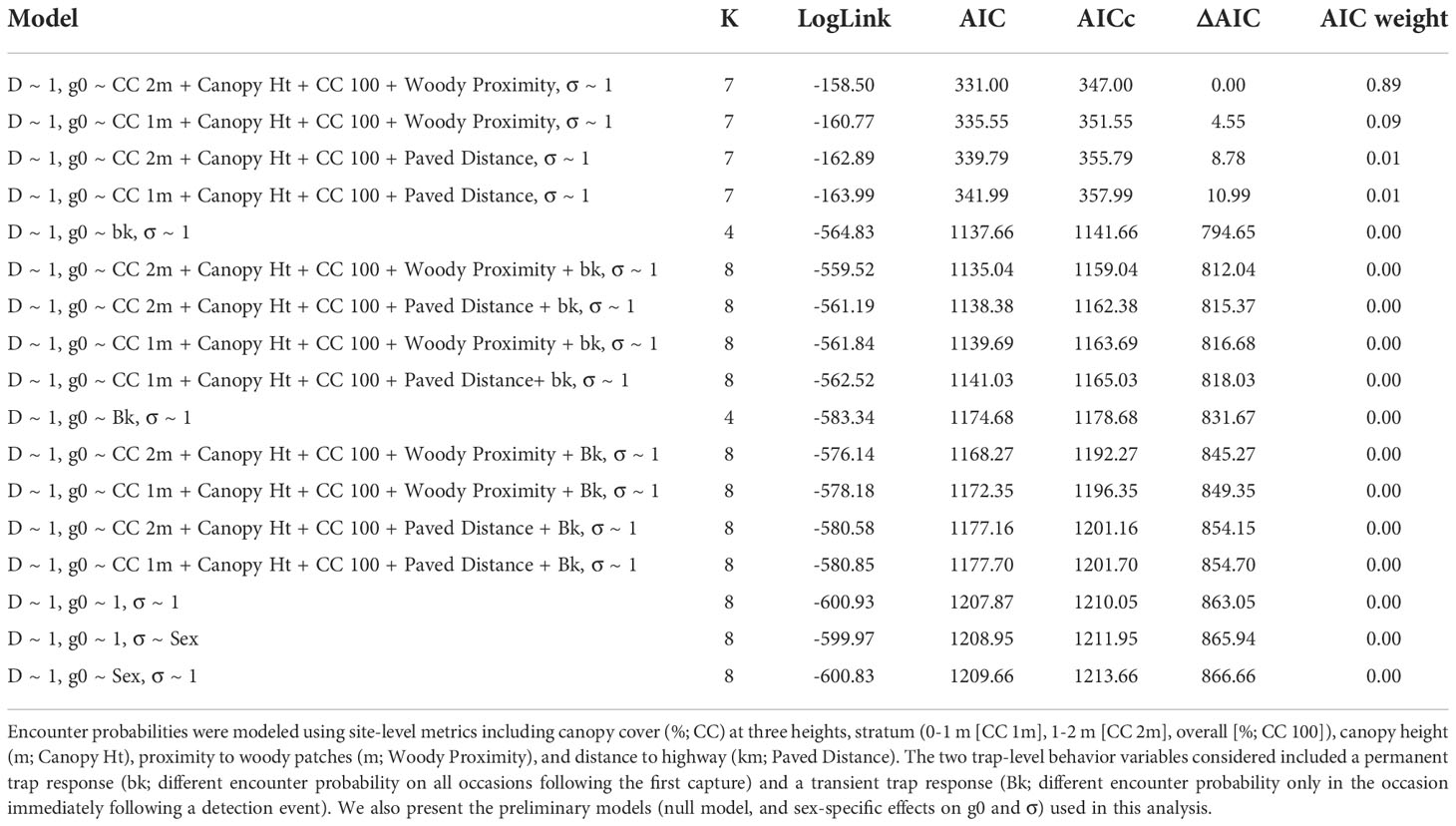
Table 2 Model selection results for the estimation of ocelot (Leopardus pardalis pardalis) density and encounter probabilities (g0, σ) on the East Foundation’s El Sauz Ranch in Willacy and Kenedy counties, Texas, USA from September 2020 to May 2021. .
We next estimated population size within the state space area using the top supported model and spatial habitat mask. We used the pop.regN function in package secr (Efford and Fewster, 2013; Efford, 2021) which derives a realized ocelot population size estimate (# of ocelots) for the 206.25 km2 of suitable habitat within the state space and present 95% confidence intervals.
3. Results
From September 2020 to May 2021, we documented 15 ocelots (6 males, 9 females). Model selection indicated support for one top model that included site-level vegetation metrics influencing encounter rates but did not have a behavioral effect (Table 2). The population density was estimated at 17.60 ocelots/100 km2 (95% CI: 10.32–29.18). Realized population size for the state space area (206.25 km2) was estimated to be 36.33 ocelots (95% CI: 26.10–58.60). Ocelot encounter probabilities were 0.02 (95% CI: 0.01-0.04) and the decay distance from the activity centers (σ) was 621.87 m (95% CI: 531.20–728.02 m). Encounter probability of ocelots increased with increasing canopy cover at 2 m (β = 3.23; 95% CI 1.53–6.23) and decreasing proximity to woody cover (β = -2.11; 95% CI -3.44– -0.78); the other two variables in this model, overall canopy cover (β = -0.57; 95% CI -1. 61– 0.47) and canopy height (β = -3.05; 95% CI -0.69– 0.08) had 95% CI that overlapped zero.
4. Discussion
Estimates of population density and size are vital in wildlife conservation, as they can play key roles in determining conservation status and management. For a species that has been endangered in the United States since 1982, the absence of a true analytical-based estimate of population size, even in one portion of their range has hampered recovery efforts. Subjective estimates based on other data sources may paint an incomplete picture and affect abilities to make science-based recovery decisions. Further, our knowledge of ocelots has been reliant on broad-scale landscape assessments or field measurements of vegetation structure to inform where ocelots are detected or the types of habitats they may occur in or select (Shindle and Tewes, 1998; Jackson et al., 2005; Haines et al., 2006b; Horne et al., 2009; Lombardi et al., 2020b). By incorporating vegetation metrics derived from LiDAR and distance-based metrics, we gained a stronger understanding of factors likely contributing to where we are likely to detect ocelots, in turn, aiding future population monitoring efforts. To date, this is the first application of LiDAR in a SECR framework, and the second application of LiDAR to investigate an aspect of ocelot ecology in Texas. The estimates of population size and density presented here reflect the first SECR-derived and most recent population estimates for ocelots in a portion of their northernmost geographic range in Texas.
Ocelots have been associated with densely vegetated communities and large areas of forest cover (Haines et al., 2006b; Hunter, 2015; Wang et al., 2019; Veals et al., 2022). Previous regional studies postulated ocelots were strongly tied to extremely dense (>95% canopy cover; >85% vertical obstruction) up to 2 m in height (Tewes, 1986; Shindle and Tewes, 1998; Harveson et al., 2004; Horne et al., 2009). These studies, however, were based on ocular field measurements of woody vegetation, not fine-scale LiDAR-derived metrics of both woody and herbaceous cover. Consequently, we focused our modeling of encounter probabilities to examine canopy height and canopy cover at 0-2 m and overall. We found that ocelots were most likely to be detected in areas with increasing canopy cover at 2 m (Horne et al., 2009). Upon closer inspection, we found that these canopies were not as extremely dense as previous studies have observed. Across our camera sites, the maximum vegetative canopy cover at 2 meters was 67%, and the average was 27%, far lower than the 85% at 2 m suggested by Shindle and Tewes (1998) as ideal habitat. Further, herbaceous cover (i.e., guinea grass and cordgrass) in our study area tend to grow in high plant densities and can reach over 2 m in height. It has been suggested that these areas may facilitate ocelot movement forays (Veals et al., 2022; Sergeyev et al. In Press) and den-site selection (Laack et al., 2005). These results reflect ocelots being detected in areas with more heterogeneity in vegetative (i.e., woody and herbaceous) canopy cover at 1-2 meters, which corroborates recent studies that suggested ocelots may use (Lombardi et al., 2020b) and select (Sergeyev et al. In Press) for more heterogeneous vegetation patches.
On a more macro-scale, the probability of ocelot encounters increased near and within forested patches. Higher encounter rates within or near woody cover suggest ocelots likely rely on woody cover for their activity patterns (Pérez-Irineo and Santos-Moreno, 2014; Wang et al., 2019; Lombardi et al., 2020b; Lombardi et al., 2022). Use of herbaceous cover located within a mosaic of proximate woody cover may provide ocelots with additional food resources (Paolino et al., 2018) or travel corridors between patches, rather than use of extensive grasslands. The importance of forested and woody interior areas cannot be understated though, as it has been linked to access to potential mates, avoidance of interspecific competitors, and increased prey availability (Horne et al., 2009; Booth-Binczik et al., 2013; Wang et al., 2019).
Identification of the vegetation structure where ocelots are likely to be encountered is an important aspect of camera trap placement for concurrent and future camera trapping efforts. Understanding ocelot use of particular vegetation types will help maximize encounter rates for future population estimates and regional monitoring efforts. Our results suggest ocelots are not solely tied to extremely dense vegetation, but that they are likely to be detected across more heterogenous vegetative landscapes. Future camera trap studies and monitoring efforts should focus or expand to areas in proximity to woody cover and place cameras within vegetative cover with 2 m canopies to increase ocelot detections.
Our results indicate the presence of an abundant local ocelot population and may reflect the quality of the habitat to support such high population densities. Notably, these estimates are reflective of densities more commonly observed in more remote areas of their geographic range in Central and South America (Ayala et al., 2010; Martínez-Hernández et al., 2015; Satter et al., 2019; Lombardi et al., 2022). Ocelot densities are likely to be greatest towards the core of their range (i.e., Amazon Forest; Penido et al., 2016). and not on the geographic peripheries (Di Bitetti et al., 2006; Bolze et al., 2021, this study). In the Texas-Northeastern Mexico biogeographic region, ocelots are considered endangered in each country, but populations in northeastern Mexico are thought to be more secure than in Texas (Martínez-Hernández et al., 2015; Gomez-Ramirez et al., 2017; Lombardi et al., 2022). However, we report densities 33.5% greater than reported in the more secure and remote foothills of the Sierra of Tamaulipas Biosphere Reserve (Lombardi et al., 2022) located < 350 km south of our study site. Further, our reported density of 17.6 ocelots/100 km2 is similar to the maximum density (18 ocelots/100 km2) reported further south in the Sierra Abra-Tanchipa Biosphere Reserve in eastern San Luis Potosi, Mexico (Martínez-Hernandez et al., 2015). Higher densities of ocelots were reported in Belize (Satter et al., 2019) an area of high primary productivity closer to the core of the ocelot’s geographic range. At the austral (southern) geographic periphery in the Rio Grande du Sul region of Brasil, equally high densities of ocelots (15.5 ocelots/100 km2) have been reported using the same study design (i.e., 1 x 1 km arrays) as this study (Bolze et al., 2021).
Population density estimates reported in this study represent a more accurate estimate of the density of ocelots at the northernmost distribution of their geographic range. Previous estimates in Texas used traditional CR methods and a non-structured camera array which may have contributed to an inflated density estimate of 30 ocelots/100 km2 (Haines et al., 2006a). We considered a closed population during our study and controlled for births by not including any kittens in the analysis; however, death was harder to control. We were not aware of any deaths during our study based on known GPS-collared individuals (from a concurrent study; Sergeyev et al. In Press) and long-term camera trap monitoring. However, because we cannot control for unaccounted-for deaths if a death did occur it would positively bias our estimates.
Our results indicate there are at least 36 ocelots (95% CI: 29-56) within the state space area, which included the camera trap array and surrounding suitable habitat. These local estimates may reflect a potentially much larger overall Ranch Ocelot Population throughout the neighboring adjacent extensive ranchlands than previously believed. Based on known individuals, population estimates for the entire hypothesized Ranch Population were 35-50, but this included known individuals from the Lower Rio Grande Valley National Wildlife Refuge Conservation Easements on the Yturria Ranch (8 km west of our study site). Localized density estimates reported here coupled with a wider distribution of suitable woody patches (Lombardi et al., 2021), and a more flexible selection of woody and herbaceous vegetation in these ranchlands (Veals et al., 2022; Sergeyev et al. In Press) may help support the notion of a much larger population than previously postulated. Although further examination of population densities across this property and other ranches with known ocelot populations is warranted to better understand the potential size of the Ranch Population. Future monitoring should also place more emphasis on monitoring areas of herbaceous cover that lie within proximity of woody cover or occur amongst mottes/islands of woody cover, to help maximize potential detections/movements in these areas. This study represents a critical snapshot in time reflecting current population size and density estimates in a portion of their northernmost range which will inform ongoing population viability analyses. Additionally, our estimates demonstrate that large working ranchlands with strong habitat management practices can provide suitable habitats to support large ocelot densities.
Establishing relationships with conservation-minded private landowners who own large tracts of private land is an effective strategy for researchers studying endangered carnivores (Haines et al., 2006a; Veals et al., 2022). Since the rediscovery of ocelots on our study site in 2011, the East Foundation has been an active research partner with on-the-ground ocelot conservation efforts. For biologists and researchers, working with private landowners requires open communication about research objectives and findings, and may start under a veil of confidentiality, but eventually, the benefits outweigh the costs. Private land holdings can provide immense opportunities to monitor populations, restore connectivity between populations, and preserve habitat (Hilty and Merenlender, 2003; Bennett et al., 2018; Veals et al., 2022). Cooperative conservation efforts with private landowners will continue to be a key conservation strategy for studying and preserving threatened and endangered species (Bennett et al., 2018; Kobilinsky, 2022).
4.1. Conservation implications
Accurate population estimates of endangered species are a critical component for informing species recovery and advancing conservation efforts. Texas contains 83% privately owned large working lands, forests, and farms (Samson et al., 2021) and southern Texas, in particular, is a region with some of the largest ranchlands in the state coupled with a growing metropolitan area in the Lower Rio Grande Valley along the US-Mexico border. Unlike in other areas of the United States, conservation-minded private landowners and academic institutions are leading the effort to recover and restore ocelots. These private lands in Texas, hold large patches of suitable landscape structure (Lombardi et al., 2021) and heterogeneous vegetative cover are the key to ocelot recovery (Haines et al., 2006a; Lombardi et al., 2020a; Veals et al., 2022). In the past, several organizations worked separately to recover ocelot populations with little change in the listing status or population size (Tewes, 2019). We have found, however, that a private landowner-led effort with cooperation from multiple state, federal, academic, and zoological institutions can pave the way for ocelot recovery in Texas. Studies like ours are critical to providing the best available science to inform concurrent reintroduction and habitat restoration efforts to support ocelot recovery in the United States.
Data availability statement
The datasets presented in this article are not readily available because Camera trap data used in this analysis contains possible identifying and sensitive location information and ocelot detection locations on private ranchlands are to remain confidential as per the United States Fish and Wildlife Service federal regulations for ocelot research (permit number #PRT-67681, Federal Register). Parties with a legitimate interest in obtaining these data for replication purposes may contact Dr. David G. Hewitt, Director of the Caesar Kleberg Wildlife Research Institute, at RGF2aWQuSGV3aXR0QHRhbXVrLmVkdQ==. Requests to access the datasets should be directed to ZGF2aWQuaGV3aXR0QHRhbXVrLmVkdQ==.
Ethics statement
Ethical review and approval was not required for the animal study because We used non-invasive camera traps (survey methodology) for the study. No animals were captured or handled for this study.
Author contributions
All authors listed have made a substantial, direct, and intellectual contribution to the work and approved it for publication.
Funding
Thank you to the Caesar Kleberg Wildlife Research Institute and Brown Foundation for providing funding for batteries and fuel. We thank the Texas Department of Transportation-Division of Environmental Affairs for an in-kind donation of the camera traps.
Acknowledgments
We thank the East Foundation for access to the property and on-site lodging and logistical support in accessing remote areas of the ranch. Thank you to AL Martinez, N Kappel, TJ Yamashita, AB Branney, ZM Wardle, DG Scognamillo, and J Edlin for assistance with camera monitoring in the field. Thank you to MJ Cherry for consultation on the modeling approach in the initial stages of this analysis. We thank AM Foley and TA Campbell, the guest editor, and the two referees for providing critical feedback on earlier versions of this manuscript. This is East Foundation manuscript number 088 and manuscript number #22-125 of the Caesar Kleberg Wildlife Research Institute.
Conflict of interest
The authors declare that the research was conducted in the absence of any commercial or financial relationships that could be construed as a potential conflict of interest.
Publisher’s note
All claims expressed in this article are solely those of the authors and do not necessarily represent those of their affiliated organizations, or those of the publisher, the editors and the reviewers. Any product that may be evaluated in this article, or claim that may be made by its manufacturer, is not guaranteed or endorsed by the publisher.
Supplementary material
The Supplementary Material for this article can be found online at: https://www.frontiersin.org/articles/10.3389/fcosc.2022.1003044/full#supplementary-material
References
Ayala G., Viscarra M. E., Wallace R. (2010). Density and activity patterns of ocelots (Leopardus pardalis) in Rio Hondo, madidi national park and integrated management natural area, la paz, Bolivia. Rev. Bol. Ecol. Cons. Amb. 28, 119–128.
Barnes K. W., Islam K., Auer S. A. (2016). Integrating LiDAR-derived canopy structure into cerulean warbler habitat models. J. Wildl. Manage. 80, 101–116. doi: 10.1002/jwmg.995
Bennett D. E., Pejchar L., Romero B., Knight R., Berger J. (2018). Using practitioner knowledge to expand the toolbox for private lands conservation. Biol. Conserv. 227, 152–159. doi: 10.1016/j.biocon.2018.09.003
Blackburn A., Anderson C. J., Veals A. M., Tewes M. E., Wester D. B., Young J. H., et al. (2021). Landscape patterns of ocelot–vehicle collision sites. Lands. Ecol. 36, 497–511. doi: 10.1007/s10980-020-01153-y
Bolze G. J., Tirelli F. P., Queirolo D., Ramos Pereira M. J. (2021). Living on the edge: density and activity patterns of the ocelot, leopardus pardalis, in the austral limit of the Atlantic forest. Stud. Neotrop. Fauna. Envir., 1–14. doi: 10.1080/01650521.2021.2008146
Booth-Binczik S. D., Bradley S. D., Thompson C. W., Bender L. C., Huntley J. W., Harvey J. A., et al. (2013). Food habits of ocelots and potential for competition with bobcats in southern Texas. Southwest. Nat. 58, 403–410. doi: 10.1894/0038-4909-58.4.403
Brodie J., Giordano A. J. (2012). Density of the vulnerable sunda clouded leopard neofelis diardi in a protected area in sabah, Malaysian Borneo. Oryx 46, 427–430. doi: 10.1017/S0030605312000087
Burger L. W. Jr., Evans K. O., McConnell M. D., Burger L. M. (2019). Private lands conservation: A vision for the future. Wildl. Soc Bull. 2043, 398–407. doi: 10.1002/wsb.1001
Burnham K. P., Anderson D. R. (2002). Model selection and multimodal inference: a practical information theoretic. 2nd edition (New York: Springer-Verlag).
Cortez J. J.G. (2021). “Accelerating maturation of thornscrub habitat for ocelots,” in Thesis (Kingsville: Texas A&M University-Kingsville).
Crall J. P., Stewart C. V., Berger-Wolf T. Y., Rubenstein D. I., Sundaresan S. R. (2013). “HotSpotter–patterned species instance recognition,” in 2013 IEEE workshop on applications of computer vision, 230–237. doi: 10.1109/WACV.2013.6475023
Di Bitetti M. S., Paviolo A., De Angelo C. (2006). Density, habitat use and activity patterns of ocelots (Leopardus pardalis) in the Atlantic forest of misiones, Argentina. J. Zool. 270, 153–163. doi: 10.1111/j.1469-7998.2006.00102.x
Donnelly J. P., Naugle D. E., Hagen C. A., Maestas J. D. (2016). Public lands and private waters: scarce mesic resources structure land tenure and sage-grouse distributions. Ecosphere 7, e01208. doi: 10.1002/ecs2.1208
Efford M. G., Fewster R. M. (2013). Estimating population size by spatially explicit capture-recapture. Oikos 122, 918–928. doi: 10.1111/j.1600-0706.2012.20440.x
Frye E. O., Lay D. W. (1942). “Fur resources and fur animals of Texas,” in Bulletin no 25 (Austin: Texas Game, Fish, and Oyster Commission).
Gogoi K., Ujjwal K., Kausik B., Yadvendradev V. J. (2020). Spatially explicit density and its determinants for Asiatic lions in the gir forests. PloS One 15, e0228374. doi: 10.1371/journal.pone.0228374
Gomez-Ramirez M. A., Gutierrez-Gonzelaez C. E., López-González C. A. (2017). Ocelots thrive in a typical of northwestern Mexico. End. Sp. Res. 32, 471–478. doi: 10.3354/esr00828
Grasiela P., Pedro S., Carlos F. (2018). Camera traps as a tool for carnivore conservation in a mosaic of protected areas in the pantanal wetlands, Brazil. Nat. Conserv. Res. 3, 57–67. doi: 10.24189/ncr.2018.035
Greenspan E., Anile S., Nielsen C. K. (2020). Density of wild felids in Sonora, Mexico: a comparison of spatially explicit capture-recapture methods. Euro. J. Wildl. Res. 66, 1–12. doi: 10.1007/s10344-020-01401-1
Haines A. M., Janečka J. E., Tewes M. E., Grassman L. I. Jr., Morton P. (2006a). The importance of private lands for ocelot leopardus pardalis conservation in the united states. Oryx 40, 1–5. doi: 10.1017/S0030605306000044
Haines A. M., Tewes M. E., Laack L. L., Horne J. S., Young J. H. (2006b). A habitat-based population viability analysis for ocelots (Leopardus pardalis) in the united states. Biol. Conserv. 132, 424–436. doi: 10.1016/j.biocon.2006.04.035
Harveson P. M., Tewes M. E., Anderson G. L., Laack L. L. (2004). Habitat use by ocelots in south Texas: implications for restoration. Wildl. Soc Bull. 32, 948–954. doi: 10.2193/0091-7648(2004)032[0948:HUBOIS]2.0.CO;2
Hilty J., Merenlender A. M. (2003). Studying biodiversity on private lands. Conserv. Biol. 17, 132–137. doi: 10.1046/j.1523-1739.2003.01361.x
Hooker M. J., Clark J. D., Bond B. T., Chamberlain M. J. (2021). Evaluation of connectivity among American black bear populations in Georgia. J. Wildl. Manage. 85, 979–988. doi: 10.1002/jwmg.22041
Horne J. S., Haines A. M., Tewes M. E., Laack L. L. (2009). Habitat partitioning by sympatric ocelots and bobcats: implications for recovery of ocelots in southern Texas. Southwest Nat. 54, 119–126. doi: 10.1894/PS-49.1
International Union for the Conservation of Nature (2022) The IUCN red list of threatened species. Available at: http://www.iucnredlist.org (Accessed 29 January 2022).
Jackson V. L., Laack L. L., Zimmerman E. G. (2005). Landscape metrics associated with habitat use by ocelots in south Texas. J. Wildl. Manage. 69, 733–738. doi: 10.2193/0022-541X(2005)069[0733:LMAWHU]2.0.CO;2
Janecka J. E., Tewes M. E., Davis I. A., Haines A. M., Caso A., Blankenship T. L. (2016). Genetic differences in the response to landscape fragmentation by a habitat generalist, the bobcat, and a habitat specialist, the ocelot. Conserv. Genet. 17, 1093–1108. doi: 10.1007/s10592-016-0846-1
Karanth K. U., Nichols J. D. (1998). Estimation of tiger densities in India using photographic captures and recaptures. Ecol 79, 2852–2862. doi: 10.1890/0012-9658(1998)079[2852:EOTDII]2.0.CO;2
Kittle A. M., Watson A. C., Cushman S. A., Macdonald D. W. (2018). Forest cover and level of protection influence the island-wide distribution of an apex carnivore and umbrella species, the Sri Lankan leopard (Panthera pardus kotiya). Biodivers. Conserv. 27, 235–263. doi: 10.1007/s10531-017-1431-8
Kobilinsky D. (2022). Saving a place for nature: conservation easements are aiding wildlife on private lands (Bethesda, MD: The Wildlife Professional), 16–24.
Laack L. L., Tewes M. E., Haines A. M., Rappole J. H. (2005). Reproductive life history of ocelots leopardus pardalis in southern Texas. Acta Their. 50, 505–514. doi: 10.1007/BF03192643
Laliberte A. S., Ripple W. J. (2004). Range contractions of north American carnivores and ungulates. BioSci 54, 123–138. doi: 10.1641/0006-3568(2004)054[0123:RCONAC]2.0.CO;2
Leslie D. M. Jr. (2016). An international borderland of concern: Conservation of biodiversity in the lower Rio grande valley (Stillwater: US Geological Survey). doi: 10.3133/sir20165078
Lewis J. S., Logan K. A., Alldredge M. W., Bailey L. L., VandeWoude S., Crooks K. R. (2015). The effects of urbanization on population density, occupancy, and detection probability of wild felids. Ecol. Appl. 25, 1880–1895. doi: 10.1890/14-1664.1
Lombardi J. V., Perotto-Baldivieso H. L., Sergeyev M., Veals A. M., Schofield L., Young J. H., et al. (2021). Landscape structure of woody cover patches for endangered ocelots in southern Texas. Rem. Sens. 13, 4001. doi: 10.3390/rs13194001
Lombardi J. V., Perotto-Baldivieso H. L., Tewes M. E. (2020a). Land cover trends in south Texas: potential implications for wild felids. Rem. Sens. 12, 659. doi: 10.3390/rs12040659
Lombardi J. V., Stasey W. C., Caso A., Carvajal-Villarreal S., Tewes M. E. (2022). Ocelot density and habitat use in tamaulipan thornshrub and tropical deciduous forests in northeastern méxico. J. Mamm. 103 (1), 57–67. doi: 10.1093/jmammal/gyab134
Lombardi J. V., Tewes M. E., Perotto-Baldivieso H. L., Mata J. M., Campbell T. A. (2020b). Spatial structure of woody cover affects habitat use patterns of ocelots in Texas. Mamm. Res. 65, 555–563. doi: 10.1007/s13364-020-00501-2
López-Bao J. V., Godinho R., Pacheco C., Lema F. J., García E., Llaneza L., et al. (2018). Toward reliable population estimates of wolves by combining spatial capture-recapture models and non-invasive DNA monitoring. Sci. Rep. 8, 1–8. doi: 10.1038/s41598-018-20675-9
Martínez-Hernández A., Rosas-Rosas O. C., Clemente-Sánchez F., Tarango-Arámbula L. A., Palacio-Núñez J., Bender L. C., et al. (2015). Density of threatened ocelot leopardus pardalis in the Sierra abra-tanchipa biosphere reserve, San Luis potosí, Mexico. Oryx 49, 619–625. doi: 10.1017/S0030605313001452
McGowan C. P., Allan N., Servoss J., Hedwall S., Wooldridge B. (2017). Incorporating population viability models into species status assessment and listing decisions under the US endangered species act. Glob. Ecol. Conserv. 12, 119–130. doi: 10.1016/j.gecco.2017.09.004
Moreno-Sosa A. M., Yacelga M., Craighead K. A., Kramer-Schadt S., Abrams J. F. (2022). Can prey occupancy act as a surrogate for mesopredator occupancy? a case study of ocelot (Leopardus pardalis). Mamm. Biol. 102, 163–175. doi: 10.1007/s42991-022-00232-8
Nipko R. B., Holcombe B. E., Kelly M. J. (2020). Identifying individual jaguars and ocelots via pattern-recognition software: comparing HotSpotter and wild-ID. Wildl. Soc Bull. 44, 424–433. doi: 10.1002/wsb.1086
Norwine J., Kuruvilla J. (2007). The changing climate of south texas 1900–2100: problems and prospects, impacts, and implications (Kingsville: CREST-RESSACA, Texas A&M University-Kingsville).
Otis D. L., Burnham K. P., White G. C., Anderson D. R. (1978). Statistical inference from capture data on closed animal populations. Wildl. Monographs. 62, 3–135.
Paolino R. M., Royle J. A., Versiani N. F., Rodrigues T. F., Pasqualotto N., Krepschi V. G., et al. (2018). Importance of riparian forest corridors for the ocelot in agricultural landscapes. J. Mamm. 99, 874–884. doi: 10.1093/jmammal/gyy075
Pasa J. B., Arrais R. C., Massara R. L., Pereira G., de Azevedo F. C. C. (2021). Factors influencing the habitat use by ocelots in one of the last large Atlantic Forest remnants in southeastern Brazil. Ecology and Evolution 11 (9), 4631–4643.
Penido G., Astete S., Furtado M. M., Jácomo A. T. D. A., Sollmann R., Torres N., et al. (2016). Density of ocelots in a semiarid environment in northeastern Brazil. Biota Neotrop. 16. doi: 10.1590/1676-0611-BN-2016-0168
Pérez-Irineo G., Santos-Moreno A. (2014). Density, distribution, and activity of the ocelot leopardus pardalis (Carnivora: Felidae) in southeast Mexican rainforests. Rev. Biol. Trop. 62, 1421–1432. doi: 10.15517/rbt.v62i4.12941
Qi J., Shi Q., Wang G., Li Z., Sun Q., Hua Y., et al. (2015). Spatial distribution drivers of amur leopard density in northeast China. Biol. Conserv. 191, 258–265. doi: 10.1016/j.biocon.2015.06.034
Rather T. A., Kumar S., Khan J. A. (2021). Density estimation of tiger and leopard using spatially explicit capture-recapture framework. PeerJ 9, e10634. doi: 10.7717/peerj.10634
Samson A., McDonald A., Howe B., McCann B., Langford D. K., Yeates D., et al. (2021). Creating resilient landscapes: the critical role of working lands in a changing climate (San Antonio, TX: Texas Agricultural Land Trust).
Satter C. B., Augustine B. C., Harmsen B. J., Foster R. J., Kelly M. J. (2019). Sex-specific population dynamics of ocelots in Belize using open population spatial capture-recapture. Ecosph 10, e02792. doi: 10.1002/ecs2.2792
Sergeyev M., Holbrook J. D., Lombardi J. V., Tewes M. E., Campbell T. A. (In Press). “Behaviorally mediated coexistence of ocelots, bobcats, and coyotes using hidden Markov models,” in Oikos.
Sharma R. K., Jhala Y., Qureshi Q., Vattakaven J., Gopal R., Nayak K. (2010). Evaluating capture-recapture population and density estimation of tigers in a population with known parameters. Anim. Conserv. 13, 94–103. doi: 10.1111/j.1469-1795.2009.00305.x
Shindle D. B., Tewes M. E. (1998). Woody species composition of habitats used by ocelots (Leopardus pardalis) in the tamaulipan biotic province. Southwest. Nat. 43, 273–279.
Sollmann R., Gardner B., Chandler R. B., Shindle D. B., Onorato D. P., Royle J. A., et al. (2013). Using multiple data sources provides density estimates for endangered Florida panther. J. Appl. Ecol. 50, 961–968. doi: 10.1111/1365-2664.12098
Tewes M. E. (1986). “Ecological and behavioral correlates of ocelot spatial patterns,” in Dissertation (Moscow: University of Idaho).
Tewes M. E. (2019). “Conservation status of the endangered ocelot in the united states – a 35-year perspective,” in 37th annual faculty lecture (Kingsville: Texas A&M University-Kingsville).
U. S. Fish and Wildlife Service (2016). Recovery plan for the ocelot (Leopardus pardalis) first revision (Albuquerque: Southwest Region U. S. Fish and Wildlife Service).
Veals A. M. (2021). “Resource selection and landscape connectivity of the ocelot (Leopardus pardalis) in southern Texas,” in Dissertation (Kingsville: Texas A&M University-Kingsville).
Veals A. M., Holbrook J. D., Blackburn A., Anderson C. J., DeYoung R. W., Campbell T. A., et al. (2022). Multiscale habitat relationships of a habitat specialist over time: the case of ocelots (Leopardus pardalis) in south Texas from 1982-2017. Ecosphere. 13 (8), e4204. doi: 10.1002/ecs2.4204
Keywords: carnivore conservation, endangered species, Leopardus pardalis, private lands, species recovery, vegetation structure, light image detection and ranging (LiDAR)
Citation: Lombardi JV, Sergeyev M, Tewes ME, Schofield LR and Wilkins RN (2022) Spatial capture-recapture and LiDAR-derived vegetation metrics reveal high densities of ocelots on Texas ranchlands. Front. Conserv. Sci. 3:1003044. doi: 10.3389/fcosc.2022.1003044
Received: 25 July 2022; Accepted: 29 November 2022;
Published: 14 December 2022.
Edited by:
Aaron Haines, Millersville University of Pennsylvania, United StatesReviewed by:
Bo Luo, China West Normal University, ChinaRahel Sollmann, Leibniz Institute for Zoo and Wildlife Research (LG), Germany
Copyright © 2022 Lombardi, Sergeyev, Tewes, Schofield and Wilkins. This is an open-access article distributed under the terms of the Creative Commons Attribution License (CC BY). The use, distribution or reproduction in other forums is permitted, provided the original author(s) and the copyright owner(s) are credited and that the original publication in this journal is cited, in accordance with accepted academic practice. No use, distribution or reproduction is permitted which does not comply with these terms.
*Correspondence: Jason V. Lombardi, bG9tYmFyZGlqdkBnbWFpbC5jb20=