- 1Rapiscan Systems Ltd., Sydney, NSW, Australia
- 2Taronga Institute of Science and Learning, Taronga Conservation Society Australia, Sydney, NSW, Australia
- 3Department of Agriculture, Fisheries and Forestry, Canberra, ACT, Australia
Wildlife trafficking is a global problem involving the deliberate and illegal transport of wildlife across international borders. Animals are either removed directly from their natural environment or bred specifically to fuel demand driven by activities such as the illegal pet trade or for purported medicinal reasons. In Australia, wildlife trafficking poses a serious environmental and biosecurity risk through the removal of native species and the introduction of exotic invasive wildlife. This has the potential to impact the natural ecosystem and Australia’s multibillion-dollar agricultural industry. To help detect and restrict this activity, innovative technologies such as 3D X-ray CT technology using Real Time Tomography has been trialed to create wildlife detection algorithms for deployment across Australian mail/traveller luggage pathways. Known species of trafficked Australian wildlife and additional model species of exotics were scanned to create an image reference library for algorithm detection. A total of 294 scans from 13 species of lizards, birds and fish were used to develop initial wildlife algorithms with a detection rate of 82% with a false alarm rate at 1.6%. In combination with human and biosecurity dog detection, this innovative technology is a promising complementary platform for wildlife detection at Australian international borders, with potential worldwide applications.
Introduction
The illegal wildlife trade (IWT) is a growing international problem estimated to be worth US$8 billion to $21 billion annually (Scheffers et al., 2019; UNODC, 2020). The process of wildlife trafficking involves the illegal and deliberate movement of wildlife across international borders. Animals are often concealed in cruel and inhumane circumstances in order to avoid being detected via mail/air cargo pathway and the traveller pathway within luggage or carried on one’s person. Growing demand for wildlife on the black market can have detrimental impacts on individual animals and populations worldwide, threatening some species with extinction (Brown et al., 2020; Rosen and Smith, 2010; Scheffers et al., 2019; Sollund, 2016; Sollund 2019; Tella and Hiraldo, 2014).
Australia is home to some of the world’s most diverse and unique wildlife, which makes it an attractive market for the IWT. Wildlife trafficking pathways, out of and into Australia, create serious biosecurity risks to the natural environment and to the ensuing health and well-being of all life-forms which depend on functioning, resilient ecosystems. Known trafficked animals such as Australian reptiles (e.g. lizards) (Heinrich et al., 2021) and birds (e.g. parrots) are targeted for the illegal pet trade (primarily exports), which often involve the removal of species from the wild (Alacs and Georges, 2008; AUSTRAC, 2020; Toomes et al., 2020a). Illegal exotic animal imports (e.g. lizards, turtles, snakes and fish) which include their associated microbes have great potential to exacerbate biodiversity loss in Australia (Toomes et al., 2020b). The introduction of invasive alien species also poses significant risks to Australia’s multibillion-dollar agricultural industry (Garcia-Diaz et al. 2017; Brown et al., 2020).
Globally, the movement of wildlife is regulated by the Convention on International Trade in Endangered Species of Wild Fauna and Flora (CITES), which is an international agreement between governments that aims to ensure international trade in wildlife does not threaten wild populations of plants and animals (CITES, 2021). A total of 183 countries, including Australia, are signatories to this Convention. While CITES is in place to support the legal animal trade to ensure species do not go extinct, it does not account for the entirety of the international market for wildlife, such as the IWT (Rosen and Smith, 2010; UNODC, 2020). To help combat illegal wildlife crime, CITES, together with The International Criminal Police Organization (INTERPOL), the United Nations Office on Drugs and Crime (UNODC), the World Bank and the World Customs Organization, have come together to form the International Consortium on Combating Wildlife Crime (CITES, 2021). As part of this, all five inter-governmental organisations have signed a Letter of Understanding, which outlines the responsibilities, capabilities and priorities to aid with combating the IWT globally. One particular focus is on developing new capabilities and tools to enhance the combat of illegal wildlife crime (CITES, 2021).
Existing measures to detect wildlife in the IWT involves a combination of tools and strategies. Well known examples at international borders include X-ray scans of mail and luggage, physical detections via border security (Runhovde, 2015; Cooney et al., 2017) and use of biosecurity dogs (Braun, 2013). Detections have also evolved to target wildlife traffickers online via cyber-crime investigations (Sonricker et al., 2012; Xu et al., 2019, AUSTRAC, 2021). In addition, new technologies have led to the development of wildlife forensic science for detections (Alacs et al., 2010; Kumar et al., 2014; Brandis et al., 2018; Stringham et al., 2020; Smart et al., 2021) and the development of mobile phone reporting tools such as the ‘Wildlife Witness App’ (Taronga Conservation Society Australia, 2021). Despite these advances, the IWT continues to grow and so too the methods traffickers take to conceal wildlife e.g. within luggage, food containers or in appliances. As a result, there is growing demand for innovative and adaptive detection capabilities to help combat this international problem (Patel et al., 2015).
As part of Australia’s national and international commitment to protecting wildlife (as a member of CITIES), innovative technologies have been investigated to help combat the IWT. One promising detection route is the use of new X-ray scanning technology to advance existing detection capabilities in air cargo, mail and traveller pathways. In this short communication we report an experimental trial of newly developed 3D X-ray CT technology using Real Time Tomography (RTT) as a potential way of on-the-ground detection of illegally trafficked wildlife at Australia’s frontline. Use of volumetric 3D computed tomography has already proven a promising tool and level-up from existing 2D technology in the detection the detection of prohibited items such as hand guns in airport baggage security screening (Megherbi et al., 2012; Mouton and Breckon, 2015; Akcay and Breckon 2021; Mery et al., 2020; Wang et al., 2020; Yang et al., 2021). Here, we also describe findings on a trial utilising computer-based algorithms created from 3D X-ray images of wildlife as a potential tool to be used in conjunction with existing detection methods (e.g. visual inspection of articles via inspection/unpacking items by operators and the use of biosecurity detection dogs).
Methods
Data collection
Data collection took place between October 2020 and July 2021 at the Australia Post Sydney Gateway Facility, New South Wales, Australia. Wildlife specimens were limited to dead animals only and collected opportunistically from the Taronga Wildlife Hospital, Sydney, Australia under an institutional approval for opportunistic sampling (No. R20D308) and NSW Scientific Licence SL100104. Animals were acquired either from seized trafficking cases or from sick/injured wildlife brought into the hospital. This included a variety of known trafficked Australian species such as reptiles (e.g. lizards, turtles, snakes) and birds (Supplementary Table 1). Exotic (non-native) species (e.g. turtles) were also sampled to capture a diversity of species documented across the import and export pathways of illegal wildlife trafficking in Australia. Due to the nature of opportunistic wildlife collection, animals were obtained in a variety of states including fresh, chilled and frozen. In addition, a variety of fish species were purchased from a local fish market and sampled for the purposes of expanding algorithm capabilities across different species. For consistency, scanning data were collected from all animal carcasses after warming to ambient temperature, 19-21°C.
3D X-ray scanning
All wildlife specimens were scanned using the Rapiscan Systems Ltd. Real Time Tomography 110 (RTT®110) system (Figure 1). X-ray computed tomography is a technique which involves the use of X-rays to produce a series of image cross sections through an item. Unlike existing 2D technology, the RTT110 enables the production of images in 3D volumes, providing information about item contents with good spatial detail. The RTT110 uses a stationary gantry with multiple X-ray sources to produce high resolution 3D X-ray CT images in real time (Thompson et al., 2012), which can be manipulated to provide a 360-degree view of an animal. This system is currently in use at Australian international borders and around the world in various airports and mail cargo facilities for explosive detection screening.
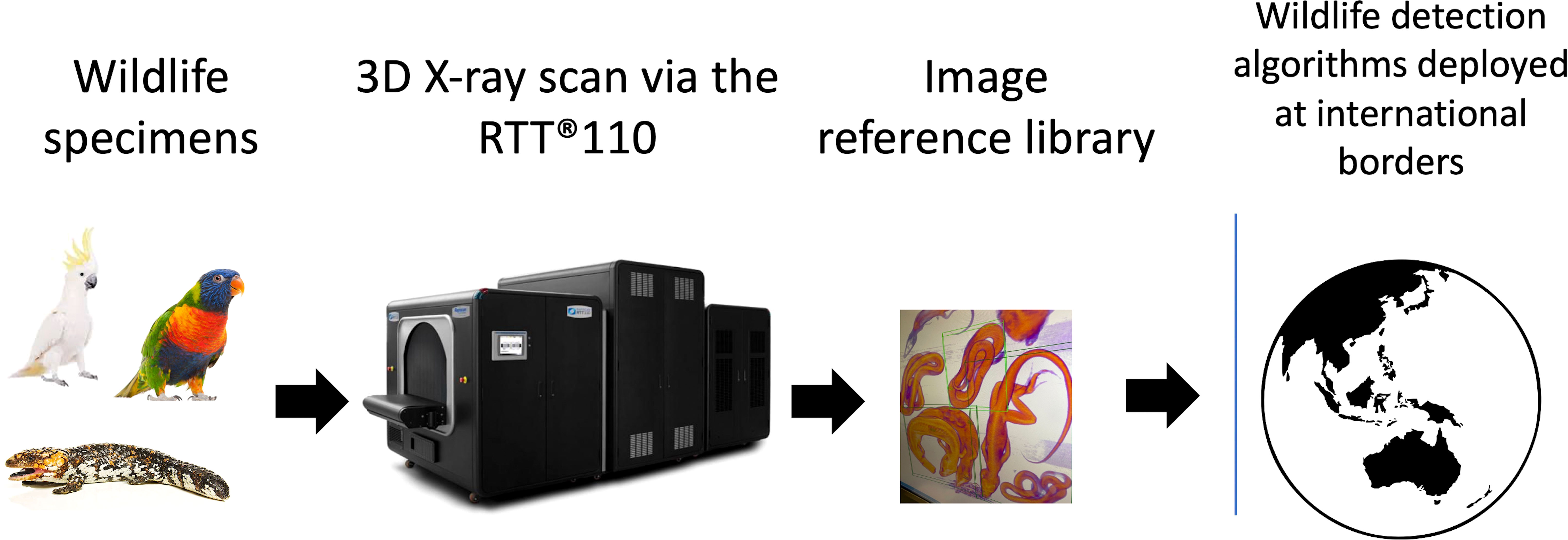
Figure 1 Data collection process used to scan Australian wildlife via the use of Real Time Tomography 3D X-ray imaging. All images contribute to a growing image reference library and used to create algorithms for wildlife detection. Algorithms are intended for deployment at Australia’s international borders as a complimentary means of wildlife trafficking detection. This is in addition to the Australian Border Force and biosecurity sniffer dogs.
For each specimen, five treatments were used to gather baseline data for the creation of an image reference library for algorithm development (Figure 2; Supplementary Table 2). These ranged from a series of simple scanning scenarios (e.g. animal scanned on its own) to more complicated realistic trafficking-based scenarios (e.g. animals under items with different densities (under fry pan) or in complicated real-world bag scenarios). This also included the replication of known concealment cases e.g. animals hidden in luggage and food containers. Additional objects scanned with specimens were selected based on several variables for complexities in algorithm construction. This included: density, size, material type and their potential to create artefacts (shadows) during the scanning progress. For biosecurity, health and safety reasons, all specimens were scanned double bagged in plastic. Use of plastic bagging did not affect scanning as the X-rays easily penetrated the thin layers to produce images of animal items. Due to the high-speed throughput of specimens in the RTT110, batches of wildlife were scanned during import mail scanning at the Sydney Gateway Facility. After each scanning session, data were stored directly onto the RTT110 machine and transferred onto cloud remote storage. All images were labelled appropriately in an image library for algorithm development.
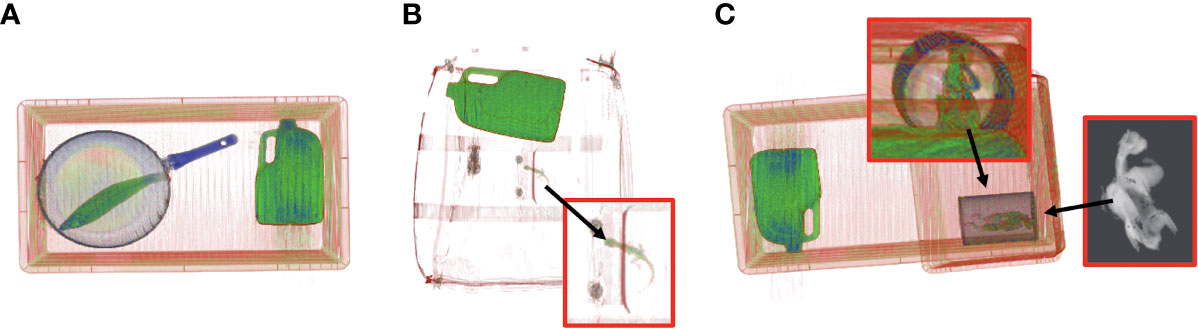
Figure 2 Scanned wildlife examples using Real Time Tomography 3D X-ray imaging (Rapiscan Systems RTT110). All wildlife in the study were initially scanned alone to train computer-based algorithms to learn the shapes of various species. Individual animals were later scanned in more complicated scenarios with other items varying in shape and density to train algorithms for wildlife detection. Examples of wildlife scans with varying degrees of complexity include; (A) Eastern school whiting fish (Sillago flindersi) under fry pan and next to three litre water bottle (B) Australian water dragon (Intellagama lesueurii) in complicated luggage type scenario with metal toy car, sock and water bottle and (C) Rainbow lorikeet parrot (Trichoglossus moluccanus) placed in chocolate milk tin. This is a replication of previously seized trafficking example in Australia.
Algorithm development and image segmentation
Projection data for scanned objects were reconstructed using the RTT110 system’s standard reconstruction software, generating volumetric images of radiodensity in offset Hounsfield Units (HU). The reconstructed CT images (Figure 3) were first segmented by thresholding on the HU values to extract the volumes within the range of organic materials, followed by a morphological opening operation. The extracted volumes were labelled by a connected component analysis. For each labelled component, features characterizing the intensity distribution (e.g. mean, standard deviation, skewness, and kurtosis), size and shape of the component (e.g. number of voxels, length of the main axis, etc.) are computed. These features were then used in the machine learning process to develop the detection model, and based on the machine learning methods we used, no dimension reduction was performed.
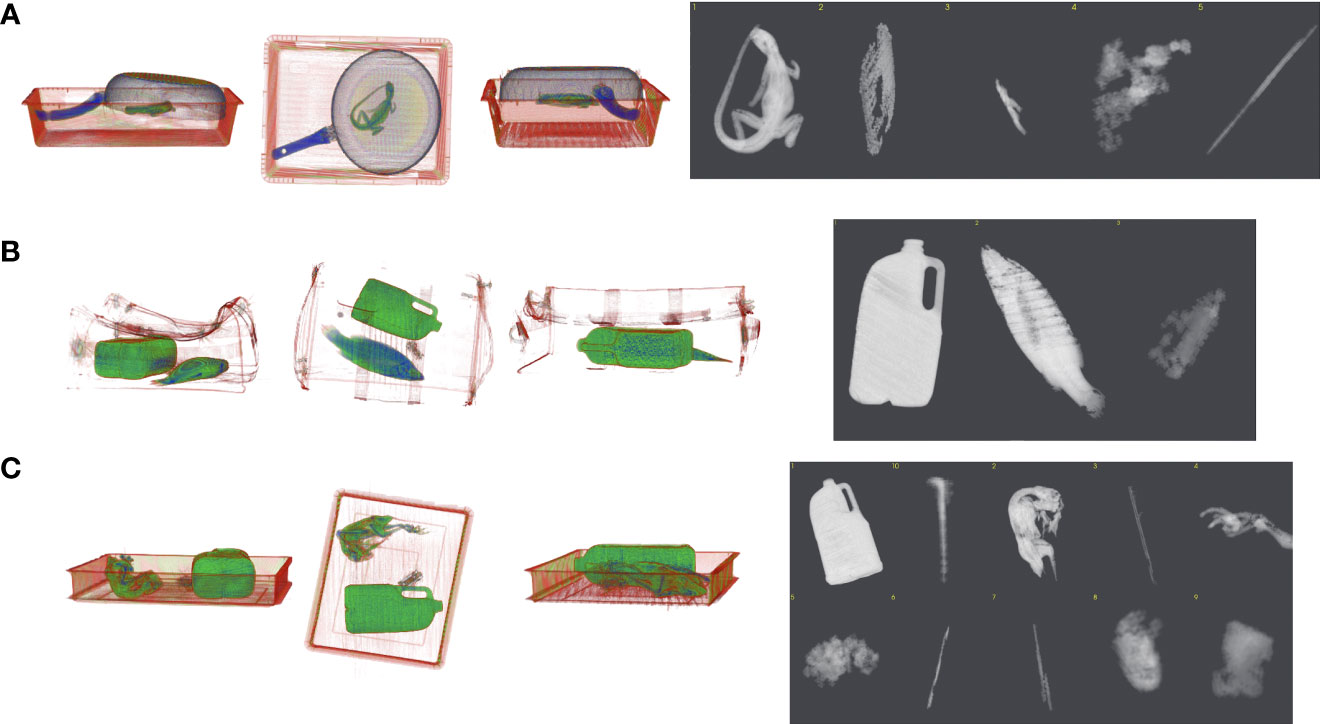
Figure 3 Example of segmentation using scanned CT images of wildlife for algorithm development to produce greyscale images. The colored 3D images are used for visualization only; image segmentations are calculated directly from the reconstructed radiodensity values in grayscale. (A) Australian water dragon (Intellagama lesueurii) under metal fry pan, (B) Barramundi fish (Lates calcarifer) in mock testing bag scenario with metal toy car, sock and water bottle, (C) Rainbow lorikeet parrot (Trichoglossus moluccanus) next to three litre water bottle.
Detection algorithm
A machine learning approach was adopted to develop a detection model that classifies the labelled components described by the extracted features into wildlife subcategories (this included ‘Lizard’, ‘Fish’ and ‘Birds’ due to high sample sizes of each, Figure 4) and non-wildlife components. This detection model is established through a training process on a collected dataset usually referred to as the training set by optimizing the model parameters to minimize the detection error on the given training set. The ability of generalization of the detection model thus trained on the unseen data is usually estimated by model performance on a ‘testing set’ sampled from the same population distribution as the training set. Specifically, we used a Random Forest (Breimann, 2001) model which aggregates the classification results from a large number of decision trees classifying each labelled component, built from random samples of the training data to produce the detection results. In experiments, we built a random forest consisting of 160 decision trees, each with maximum depth 9, classifying the labelled components described by 27 features calculated using the process described in section 2.3. To train the random forest, we used the implementation of RandomForestClassifier from the sklearn package (Pedregosa et al., 2011) version 0.23.2. The model was evaluated using a 5-fold cross-validation.
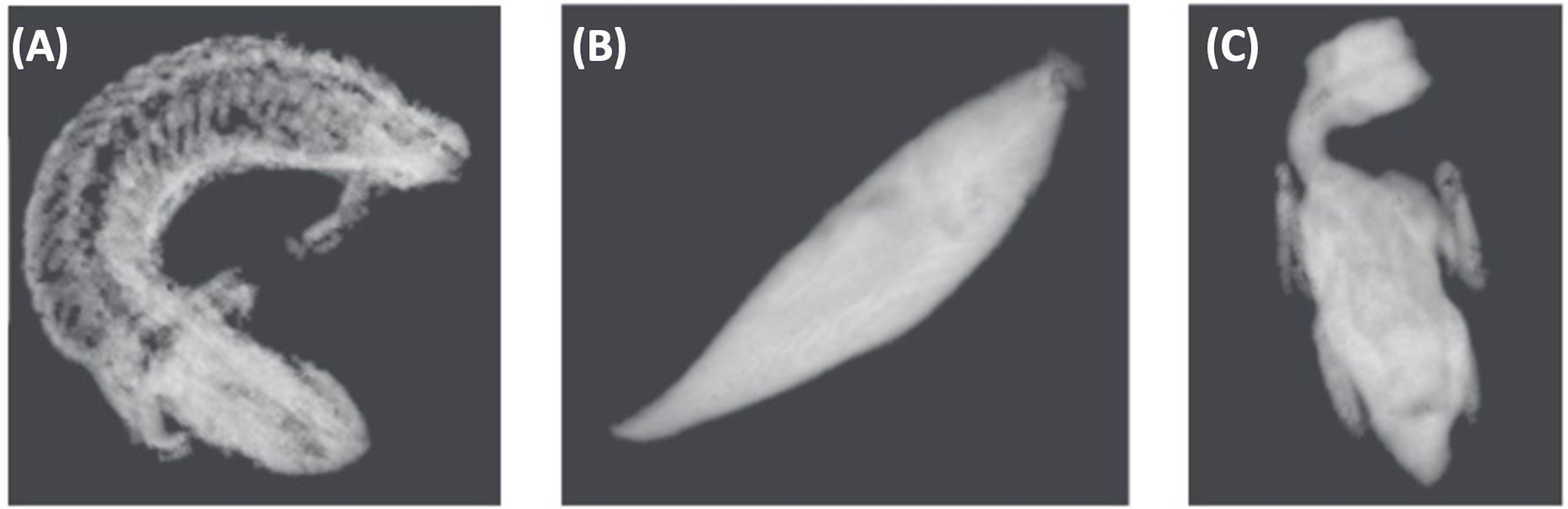
Figure 4 Grayscale image examples of three different species used in algorithm development. This includes (A) Shingleback lizard (Tiliqua rugosa), (B) Eastern school whiting (Sillago flindersi) and (C) Rainbow lorikeet parrot (Trichoglossus moluccanus). These images are a result of algorithm training using Real Time Tomography technology to produce 3D volume data.
The model performance was evaluated on a data set consisting of 4,382 bags, among which 145 contain lizards, 70 contain fish, 79 contain birds, and 4,088 negative bags contain no wildlife items. The detection rate (probability of detection, PD) for lizards, fish, and birds is the proportion of the detected scans among all scans containing these items in each category respectively, and the overall false alarm rate (probability of false alarm, PFA) is the proportion of any false detection among scans containing no wildlife items. False alarm rate on the negative sample raised by detection of each wildlife category was also calculated.
Results
A total of 85 different animals were scanned using the Rapiscan RTT110 to generate 3D X-ray CT images (Figure 2). This resulted in 459 images of which 294 scans from species with the largest sample size (lizards, birds and fish) were used for initial algorithm development (Figure 5 and Table 1). The performance of the algorithm detection for the three animal classes varied (Table 2). The false alarm rate was similar for both fish and bird animal classes (Table 2).
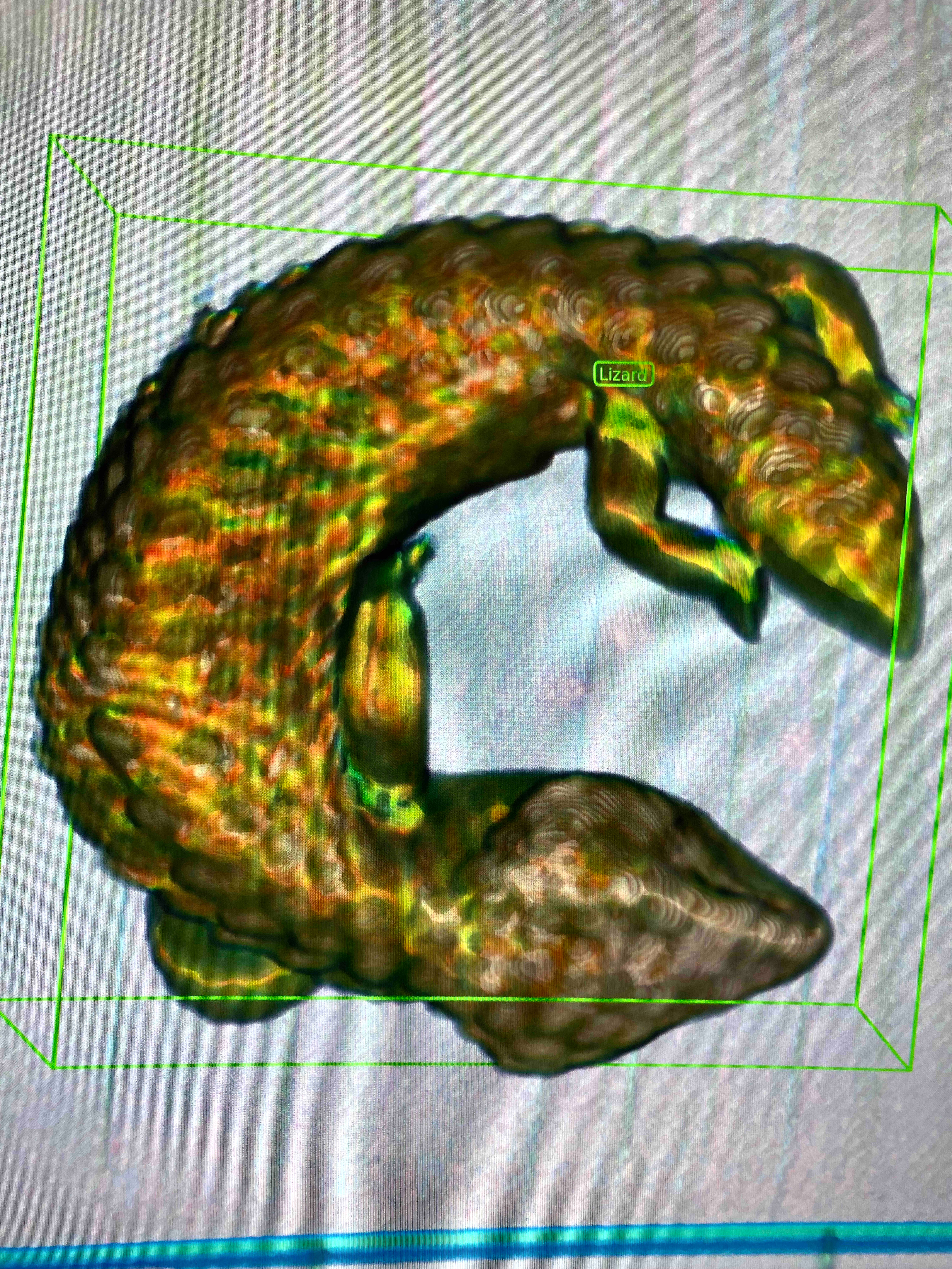
Figure 5 Example of successful lizard detection via the wildlife algorithm. This is a screenshot from the user interface alerting the operator of a detected shingleback lizard (Tiliqua rugosa) via the green bounding box which has labelled this a lizard.
Discussion
In Australia, wildlife trafficking poses tremendous threats to biodiversity and environmental health. To our knowledge, this is the first-time 3D X-ray CT technology using the Rapiscan RTT110 has been used to scan wildlife for the automated detection of illegal wildlife trafficking. Findings from this work of moderately high detectability (≥78%) and negligible false detection rates (1.5%) highlight this technology as a promising new tool for identifying illegally trafficked wildlife in mail, air cargo, and traveller pathways. This technology has been successfully trialled in other recent Australian biosecurity efforts, which has resulted in the world first automatic detection of risk items such as meat, fruit, vegetables and seeds via algorithms.
In this study, we further developed automatic detection of wildlife using 3D CT, incorporating state-of-the-art development from the statistical machine learning and artificial intelligence fields, as well as 3D imaging processing and analysis techniques. Our results demonstrate a successful application of an automatic detection algorithm for illegal wildlife trafficking using 3D X-ray CT data (Figure 5). This further adds to the growing body of literature using 3D X-rays for object detection (e.g. Mery et al., 2020; Yang et al., 2021; Akcay and Breckon, 2021). Collective findings are in support of applying this technology to a wider range of animals such as snakes and other species potentially implicated in trafficking cases in Australia. Crucially, this technology is adaptable and able to keep up with changing concealment environments traffickers may use in the future. This is timely given the increased demand for mail driven pathways during times of restricted international travel as seen during the COVID-19 pandemic and as countries continue to open up borders.
Whilst the application of this innovative technology is an additional tool to help dismantle IWT networks, there are several limitations to consider. Firstly, the use of 3D X-ray technology will not be a replacement for existing manual detection methods such as those undertaken by border enforcement personnel and biosecurity detection dogs. These existing detection methodologies are crucial elements of Australia’s biosecurity protection platform and would be strengthened by the automated detection capabilities described here. Integration of the wildlife algorithms we describe would serve as an efficient means of triggering further inspection of potential trafficking cases by border personnel (e.g. in mail and luggage items) and would provide flow-on effects that help to identify trafficking pathways.
Secondly, whilst initial algorithm developments have been made in this study, more data are needed to broaden the reference database of algorithms for other wildlife species and to increase overall wildlife detectability. Dataset limitations and the need for larger sample sizes is also a limitation on the design of our deep learning algorithms. This has also been noted as a limitation when working in the area of automatic threat detection (Akcay and Breckon, 2021). Additionally, this work is similar to Mouton et al. (2014) regarding the machine learning classifier choice and to that of Megherbi et al. (2012) regarding feature representation. Both showed high performances of using x-ray CT to detect security threats, usually of explosive and metal materials, for security screening with larger datasets and less advanced quality CT scans than those of the modern RTT. Our results of detecting wildlife animals suggest great potential of using x-ray CT imaging modality for the detection and distinguishing objects of organic materials. This is beyond the usual application CT imaging in the security screening and is a key difference from that of weapon detection as described by Mouton et al. (2014) and Akcay and Breckon (2021).
Furthermore, the sample size in this study is largely focused on species specific to Australia e.g. native lizards and birds. This highlights the need for additional research to develop algorithms with a focus on a variety of species, both native and exotic. It also presents an opportunity to collaborate with international partners using existing RTT technology to implement algorithms in airports and mail centers around the world targeting high profile species e.g. rhinos and rhino horn products (Haas and Ferreira, 2015; Haas and Ferreira, 2016).
Adaptable and innovative technologies such as Real Time Tomography 3D X-ray imaging heralds a new era in efforts to curb illegal wildlife trafficking. This study successfully demonstrates the application of computer-based detection algorithms at Australia’s frontline as a complementary means of protecting native species and the environment from exotic pests. Future development and incorporation of a wider variety of species implicated in the IWT will enable this technology to be deployed not only at Australia’s frontline but also around the world in the global fight against wildlife crime.
Data availability statement
The datasets presented in this article are not readily available because some restrictions may apply to the sharing of images. All requests will be considered and subject to approval by the Department of Agriculture, Fisheries and Forestry. Requests to access the datasets should be directed to VP, vpirotta@rapiscansystems.com.
Ethics statement
Ethical review and approval was not required for the animal study because wildlife specimens were limited to dead animals only and collected opportunistically from the Taronga Wildlife Hospital, Sydney, Australia under an institutional approval for opportunistic sampling (No. R20D308) and NSW Scientific Licence SL100104.
Author contributions
Paper conception: VP, JO’B, PM, JM, and EM. Experiment design: VP, KS, JO’B, PM, and EM. Field work: VP. Analysis and interpretation of data: VP, KS, SL, HP, JO’B, PM, and EM. Wrote paper: VP, KS, JO’B, PM, JW, and EM. All authors contributed to the article and approved the submitted version.
Funding
This project was funded by the Department of Agriculture, Fisheries and Forestry.
Acknowledgments
This research was supported by the Australian Government (Department of Agriculture, Fisheries and Forestry and the Department of Climate Change, Energy, the Environment and Water (previously the Department of Agriculture, Water and the Environment)), Rapiscan Systems Ltd, and the Taronga Conservation Society Australia. We would like to acknowledge Craig Thornhill, Luke Cascarino and Jayden Mosley from the Department of Agriculture, Fisheries and Forestry for their assistance with data collection at the Sydney Gateway Facility and Melbourne Jet base Facility. Thank you to Greig Turner and Kira O’Grady for their assistance with wildlife scanning at the Melbourne Gateway Facility. Nadine Samy and Drs Larry Vogelnest and Lydia Tong from the Taronga Wildlife Hospital are also thanked for their help in providing carcasses for this research. We thank Will Thompson for looking over our draft manuscript.
Conflict of interest
Authors VP, KS, and HP are employed by Rapiscan Systems. Authors SL and EM were employed by Rapiscan Systems.
The remaining authors declare that the research was conducted in the absence of any commercial or financial relationships that could be construed as a potential conflict of interest.
Publisher’s note
All claims expressed in this article are solely those of the authors and do not necessarily represent those of their affiliated organizations, or those of the publisher, the editors and the reviewers. Any product that may be evaluated in this article, or claim that may be made by its manufacturer, is not guaranteed or endorsed by the publisher.
Supplementary material
The Supplementary Material for this article can be found online at: https://www.frontiersin.org/articles/10.3389/fcosc.2022.757950/full#supplementary-material
References
Akcay S., Breckon T. (2021). Towards automatic threat detection: A survey of advances of deep learning within X-ray security imaging (Ithaca: Cornell University Library).
Alacs E., Georges A. (2008). Wildlife across our borders: A review of the illegal trade in Australia. Aust. J. Forensic Sci. 40, 147–160. doi: 10.1080/00450610802491382
Alacs E. A., Georges A., FitzSimmons N. N., Robertson J. (2010). DNA Detective: a review of molecular approaches to wildlife forensics. Forensic Sci. Med. Pathol. 6 (3), 180–194. doi: 10.1007/s12024-009-9131-7
AUSTRAC. (2020). Stopping the illegal trafficking of Australian wildlife, Financial Crime Guide, October 2020. Available at: https://www.austrac.gov.au/sites/default/files/2020-10/AUSTRAC_IWT%20Guide_October%202020.pdf
Brandis K. J., Meagher P. J., Tong L. J., Shaw M., Mazumder D., Gadd P., et al. (2018). Novel detection of provenance in the illegal wildlife trade using elemental data. Sci. Rep. 8 (1), 1–8. doi: 10.1038/s41598-018-33786-0
Braun B. (2013). Wildlife Detector Dogs—a Guideline on the Training of Dogs to Detect Wildlife in Trade. WWF Germany, Berlin, 1–16
Brown A., De Costa C., Guo F. (2020). Our food future: trends and opportunities, ABARES, research report 20.1 (Canberra: Australian Bureau of Agricultural and Resource Economics and Sciences (ABARES). doi: 10.25814/5d9165cf4241d
Brown A. O., Frankham G. J., Bond L., Stuart B. H., Johnson R. N., Ueland M. (2021). An overview of risk investment in the transnational illegal wildlife trade from stakeholder perspectives. WIREs Forensic Sci. 3, e1397. doi: 10.1002/wfs2.1397
Brown A. O., Frankham G. J., Stuart B. H., Ueland M. (2021). Reptile volatilome profiling optimisation: A pathway towards forensic applications. Forensic. Sci. Int.: Animals and Environments 1, 100024. doi: 10.1016/j.fsiae.2021.100024
CITES (2021) The international consortium on combating wildlife crime. Available at: https://www.cites.org/eng/prog/iccwc_new.php (Accessed June 1, 2021).
Cooney R., Roe D., Dublin H., Phelps J., Wilkie D., Keane A., et al. (2017). From poachers to protectors: engaging local communities in solutions to illegal wildlife trade. Conserv. Lett. 10 (3), 367–374. doi: 10.1111/conl.12294
Ege G., Schloenhardt A., Schwarzenegger C. (Eds.) (2020). Wildlife trafficking: the illicit trade in wildlife, animal parts, and derivatives (Berlin, Germany: Sui generis). doi: 10.24921/2020.94115945
García-Díaz P., Ross J. V., Woolnough A. P., Cassey P. (2017). The illegal wildlife trade is a likely source of alien species. Conserv. Lett. 10, 690–698. doi: 10.1111/conl.12301
Haas T. C., Ferreira S. M. (2015). Federated databases and actionable intelligence: using social network analysis to disrupt transnational wildlife trafficking criminal networks. Secur Inform. 4, 2. doi: 10.1186/s13388-015-0018-8
Haas T. C., Ferreira S. M. (2016). Combating rhino horn trafficking: The need to disrupt criminal networks. PLoS One 11 (11), e0167040. doi: 10.1371/journal.pone.0167040
Heinrich S., Toomes A., Shepherd C. R., Stringham O. C., Swan M., Cassey P. (2022). Strengthening protection of endemic wildlife threatened by the international pet trade: the case of the Australian shingleback lizard. Animal Conservation 25(1), 91–100. doi: 10.1111/acv.12721
Kumar V. P., Kumar D., Goyal S. P. (2014). wildlife DNA forensic in curbing illegal wildlife trade: specie identification from seizures. Int. J. Forensic Sci. Pathol. 2 (5), 38–42. doi: 10.19070/2332-287X-1400012
Megherbi N., Han J., Breckon T. P., Flitton G. T. (2012). “A comparison of classification approaches for threat detection in CT based baggage screening,” in 2012 19th IEEE International Conference on Image Processing. (Orlando, FL, USA) 2012 3109–3112. doi: 10.1109/ICIP.2012.6467558
Mery D., Saavedra D., Prasad M. (2020). X-Ray baggage inspection with computer vision: A survey in IEEE Access. 8, 145620–145633. doi: 10.1109/ACCESS.2020.3015014
Mouton A., Breckon T. P. (2015). A review of automated image understanding within 3D baggage computed tomography security screening. J. Xray Sci. Technol. 23 (5), 531–555. doi: 10.3233/XST-150508
Mouton A., Breckon T. P., Flitton G. T., Megherbi N. (2014). 3D object classification in baggage computed tomography imagery using randomised clustering forests, IEEE International Conference on Image Processing (ICIP), pp. 5202–5206. doi: 10.1109/ICIP.2014.7026053.
Patel N. G., Rorres C., Joly D. O., Brownstein J. S., Boston R., Levy M. Z., et al. (2015). Quantitative methods of identifying the key nodes in the illegal wildlife trade network. PNAS 112, 7948–7953. doi: 10.1073/pnas.1500862112
Pedregosa F., Varoquaux G., Gramfort A., Michel V., Thirion B., Grisel O., et al. (2011). Scikit-learn: Machine learning in Python. J. Mach. Learn. Res. 12, 2825–2830.
Rosen G. E., Smith K. F. (2010). Summarizing the evidence on the international trade in illegal wildlife. EcoHealth 7 (1), 24–32. doi: 10.1007/s10393-010-0317-y
Runhovde S. R. (2015). Seizures of inconvenience? policy, discretion and accidental discoveries in policing the illegal wildlife trade at the Norwegian border. Crime Law Soc. Change 64 (2-3), 177–192. doi: 10.1007/s10611-015-9596-0
Scheffers B. R., Oliveira B. F., Lamb I., Edwards D. P. (2019). Global wildlife trade across the tree of life. Science 366 (6461), 71–76. doi: 10.1126/science.aav5327
Smart U., Cihlar J. C., Budowle B. (2021). International wildlife trafficking: a perspective on the challenges and potential forensic genetics solutions. Forensic Sci. International: Genet. 54, 102551.doi.org/10.1016/j.fsigen.2021.102551
Sollund R. (2016). “Wildlife trafficking in a globalized world: An example of motivations and modus operandi from a Norwegian case study,” in Problematic wildlife (Cham: Springer), 553–570.
Sollund R. A. (2019). The crimes of wildlife trafficking: Issues of justice, legality and morality (London: Routledge).
Sonricker Hansen A. L., Li A., Joly D., Mekaru S., Brownstein J. S. (2012). Digital surveillance: A novel approach to monitoring the illegal wildlife trade. PloS One 7 (12), e51156. doi: 10.1371/journal.pone.0051156
Stringham O., Toomes A., Kanishka A. M., Mitchell L., Heinrich S., Ross J. V., et al. (2020). A guide to using the Internet to monitor and quantify the wildlife trade. Conservation Biology 35 (4), pp. 1130–1139. doi: 10.32942/osf.io/5yzw9
Taronga Conservation Society Australia (2020) Wildlife witness application. Available at: https://taronga.org.au/conservation-and-science/act-for-the-wild/wildlife-witness (Accessed November 4, 2020).
Tella J. L., Hiraldo F. (2014). Illegal and legal parrot trade shows a long-term, cross-cultural preference for the most attractive species increasing their risk of extinction. PloS One 9 (9), e107546. doi: 10.1371/journal.pone.0107546
Thompson W. M., Lionheart W. R., Morton E. J. (2012). “High-speed dynamic imaging with a real time tomography system,” in Proceedings of the Second International Conference on Image Formation in X-Ray Computed Tomography. Manchester Institute for Mathematical Sciences, School of Mathematics, The University of Manchester, England. 99–102.
Toomes A., García-Díaz P., Wittmann T. A., Virtue J., Cassey P. (2020a). New aliens in Australia: 18 years of vertebrate interceptions. Wildlife Res. 47 (1), 55–67. doi: 10.1071/WR18185
Toomes A., Stringham O. C., Mitchell L., Ross J. V., Cassey P. (2020b). Australia’s wish list of exotic pets: biosecurity and conservation implications of desired alien and illegal pet species. NeoBiota 60, 43. doi: 10.3897/neobiota.60.51431
UNODC, World Wildlife Crime Report (2020) United nations office on drugs and crim. Available at: https://www.unodc.org/documents/data-andanalysis/wildlife/2020/World_Wildlife_Report_2020_9July.pdf (Accessed October 29, 2020).
Wang Q., Bhowmik N., Breckon T. P. (2020). “On the evaluation of prohibited item classification and detection in volumetric 3D computed tomography baggage security screening imagery,” in Proceedings of the 2020 International Joint Conference on Neural Networks (IJCNN), Glasgow, UK Piscataway, NJ. 1–8. doi: 10.1109/IJCNN48605.2020.9207389
Wyatt T. (2016). A comparative analysis of wildlife trafficking in Australia, new Zealand and the united kingdom. J. Trafficking Organized Crime Secur. 2 (1), 62–81.
Xu Q., Li J., Cai M., Mackey T. K. (2019). Use of machine learning to detect illegal wildlife product promotion and sales on twitter. Front. Big Data 2. doi: 10.3389/fdata.2019.00028
Keywords: wildlife trafficking, illegal wildlife trade, 3D X-ray, algorithm, real time tomography, biosecurity, wildlife crime, illegal pet trade
Citation: Pirotta V, Shen K, Liu S, Phan HTH, O’Brien JK, Meagher P, Mitchell J, Willis J and Morton E (2022) Detecting illegal wildlife trafficking via real time tomography 3D X-ray imaging and automated algorithms. Front. Conserv. Sci. 3:757950. doi: 10.3389/fcosc.2022.757950
Received: 13 August 2021; Accepted: 19 August 2022;
Published: 22 September 2022.
Edited by:
J. Sean Doody, University of South Florida, United StatesReviewed by:
Daniele Pelliccia, Instruments & Data Tools Pty Ltd., AustraliaToby Breckon, Durham University, United Kingdom
Copyright © 2022 Pirotta, Shen, Liu, Phan, O’Brien, Meagher, Mitchell, Willis and Morton. This is an open-access article distributed under the terms of the Creative Commons Attribution License (CC BY). The use, distribution or reproduction in other forums is permitted, provided the original author(s) and the copyright owner(s) are credited and that the original publication in this journal is cited, in accordance with accepted academic practice. No use, distribution or reproduction is permitted which does not comply with these terms.
*Correspondence: Vanessa Pirotta, dnBpcm90dGFAcmFwaXNjYW5zeXN0ZW1zLmNvbQ==