- 1Fisheries and Oceans Canada, Bedford Institute of Oceanography, Dartmouth, NS, Canada
- 2Rosenstiel School of Marine and Atmospheric Science, University of Miami, Miami, FL, United States
- 3Statistics in Ecology, Environment and Conservation, Department of Statistical Sciences, University of Cape Town, Rondebosch, South Africa
- 4Cape Research and Diver Development, Simon’s Town, South Africa
- 5Department of Ichthyology and Fisheries Science, Rhodes University, Grahamstown, South Africa
- 6Oceans Research Institute, Mossel Bay, South Africa
- 7South African Institute for Aquatic Biodiversity, Grahamstown, South Africa
It can be difficult to determine whether a prohibition to exploitation ensures effective conservation or recovery for species that remain exposed to fishing effort and other sources of mortality throughout their range. Here we used simulation modeling of four life history scenarios (different productivity and population size) to contextualize potential population response to multiple levels of mortality, using white sharks (Carcharodon carcharias) in South Africa as a case study. The species has been protected since 1991, yet substantial uncertainty about population dynamics persists and recent declines at two aggregation sites have renewed conservation concern. All scenarios indicated that annual removals in the 10s of individuals would substantially limit the potential for and magnitude of any abundance increase following prohibition. Because average known removals from the KwaZulu-Natal Sharks Board’s Bather Protection Program have typically remained higher than these thresholds, they likely eliminated much of the conservation benefit derived from prohibition. The only life history scenario to achieve appreciable increase when simulated removals were similar to published averages assumed maturation occurred at a much younger age than currently understood. Our results demonstrate why general application of life history-based simulations can provide a useful mechanism to evaluate the biological plausibility of life history information and abundance trends, and to explore the scope for population response to recovery actions. For South Africa, our results suggest that even known levels of white shark removals, which likely underestimate total removals within their range, may be sufficient to drive abundance decline and new mitigation measures may be required to ensure population recovery.
1 Introduction
There is widespread acceptance that conservation decision-making should not be delayed because of scientific uncertainty. However, for individual populations of conservation concern, identifying the appropriate threats for mitigation often relies on having abundance information or changes in abundance over time coupled with an understanding of the magnitude of mortality arising from specific sources (e.g., Lawler et al., 2002). For pelagic sharks in particular, there are few ways to monitor population abundance, evaluate mortality or predict status, especially once a population is at risk and becomes protected from exploitation (Jorgensen et al., 2022). Given that overfishing is considered a universal threat, science-based limits to exploitation are urgently needed at a global scale to minimize mortality for at-risk species (Dulvy et al., 2014; Dulvy et al., 2021). Yet in situations where strong landings prohibitions already exist, it becomes difficult to assess whether current conservation measures are effective, or additional protections are warranted from sparse and often contradictory data.
Effective management relies on a good understanding of the relative risks and potential benefits of different regulatory strategies, or in the absence of clear data, a precautionary approach can be applied. Developing concrete recommendations relies on having science advice that is presented in tangible and easily understood metrics to facilitate decision-making (e.g., Fausch et al., 2002). In an ideal scenario, abundance of an at-risk population would be known and could be directly compared to the magnitude of total mortality arising from different sources to develop targeted mitigation strategies. However, current complications in achieving this for at-risk sharks typically include: (1) poorly quantified incidental mortalities from suspected bycatch in commercial, artisanal and recreational fisheries, owing to a general lack of reported interactions (Oliver et al., 2015), (2) uncertainty in regional or population-level abundance, with little opportunity for fishery-independent monitoring (Kuhnert et al., 2011), (3) a potential mis-match between a population’s distribution and the management unit, which can influence the representativeness of monitoring (Cadrin, 2020), and (4) uncertainty in the population’s capacity for increase, owing to data limitations or characteristics affecting our understanding of life history and productivity (Jorgensen et al., 2022).
Ideally, targeted non-lethal research will address each of these deficiencies for at-risk sharks (e.g., Hammerschlag and Sulikowski, 2011) such that the underlying dynamics of individual populations can be appropriately characterized. This would enable future quantitative predictions of status and response to threats for individual populations within a Population Viability Analysis (PVA; e.g., Morris and Doak, 2002; Dulvy et al., 2004). In the interim, the expected outcomes of different scenarios can be assessed through more general simulation modeling, which contextualizes the scope for population response under varying assumptions. This is more similar to sensitivity analyses undertaken during stock assessment (e.g., Hilborn, 2003) or to the development of multiple operating models during Management Strategy Evaluation (e.g., Punt et al., 2016). Both explore the implications of different data inputs and/or alternate population dynamics on predicted status of a particular stock or population to determine the robustness of advice. While it does not necessarily describe the ‘true’ state of the system, it allows a better understanding of risk for precautionary management and provides guidance on appropriate actions once empirical data become available.
Our approach used information on life history and available abundance estimates to explore the scope for population increase following protection from exploitation and to demonstrate potential future implications of various levels of removals. To put the work in a real-world context, we parameterized our models using data from white sharks (Carcharodon carcharias) in South Africa. We simulated four life history scenarios, giving insight into the conditions associated with recovery potential. We interpreted our simulation results using published mortality values for white sharks occurring in the KwaZulu-Natal Sharks Board’s (KZNSB) Bather Protection Program to determine the potential for and likely magnitude of any abundance increase following prohibition, and to assess how abundance may change in the future. Our results demonstrate the specific challenges associated with white shark conservation in South Africa, and suggest that mitigation measures in addition to prohibition may be required to ensure functional protection. If applied more broadly, similar analyses may be useful to contextualize risk and facilitate recovery planning for other at-risk shark species affected by ongoing mortality from multiple sources.
2 Methods
2.1 Case study
The dramatic decline of white shark (Carcharodon carcharias) at two aggregation sites in southern African waters where they were historically abundant has prompted substantial recent conservation concern (e.g., Hammerschlag et al., 2019, 2022; Towner et al., 2022). White sharks were encountered frequently in Gansbaai and False Bay, supporting valuable ecotourism operations based on sightings (Johnson and Kock, 2006). A change point occurred in 2015 at Seal Island in False Bay, with strong declines in predation rates of Cape fur seals (Arctocephalus pusillus pusillus) as well as in white shark sightings per hour (2022; Hammerschlag et al., 2019) in the years that followed. Similarly, the decline in white shark sightings has substantially accelerated since 2017 in Gansbaai (Micarelli et al., 2021; Towner et al., 2022).
It is not immediately apparent if these recent declines at local aggregation sites represent regional changes in abundance, or if they are primarily related to changes in distribution for white shark in southern Africa. Increased conservation concern would only be warranted in the former scenario. There is no data to suggest that mortality has substantially increased either regionally or locally, beyond five documented predation events by transient killer whales (Orcinus orca) in Gansbaai (Towner et al., 2022). Substantial ecological changes in community structure were coincident with white shark declines: broadnose sevengill sharks (Notorynchus cepedianus) increased in abundance and shifted distribution in False Bay (2022; Hammerschlag et al., 2019), while bronze whaler sharks (Carcharhinus brachyurus) replaced white shark as the predominant predator in Gansbaai (Towner et al., 2022). However, these changes may simply reflect large-scale redistribution of white sharks, given that their presence is putatively increasing within the Eastern Cape in the last 5 years, particularly within Algoa Bay (DFFE, 2020).
White sharks were preemptively protected from exploitation in South Africa in 1991 even though their population size and status at that time were largely unknown (Compagno, 1991; Cliff et al., 1996). Since then, ongoing mortality have been occurring from the beach protection programs along the KwaZulu-Natal coastline (Cliff and Dudley, 2011), in recreational and subsistence fisheries along southern Africa’s coastlines, as well as in commercial fisheries (Kock et al., 2022). To our knowledge, an annual time series of catches and mortalities of white shark from the KZNSB program has yet to be published. Kock et al., 2022 cites a total of 1317 white shark captures between 1978 and 2018 where 209 (16%) were released alive, which would suggest average mortalities of 28 animals per year. Cliff and Dudley (2011) excluded the early part of the time series (when white sharks were killed when captured) and calculated average values of 33 animals (SD = 12.3) in the 1990s, and 25 animals (SD = 7.8) in the 2000s with 15% and 10%, respectively, released alive. This suggests that mortality was 28 animals per year (SD = 18-38) in the 1990s and 23 animals per year (15-30) in the 2000s from the netting component. Beginning in 2007, nets were partially replaced with drumlines (2018; Cliff and Dudley, 2011; Dicken et al., 2016) and removals were estimated at 6 animals per year (SD = 5-8) from 2007-2010 (Cliff and Dudley, 2011). Collectively, these analyses suggest that total mortality from the KZNSB program would have been in the range of 20-40 animals per year from 1991 onwards, and that removals have remained relatively constant through time. Similar consistency was suggested by the catch-per-unit-effort (CPUE) index derived from the program, where there was no systematic trend between 1978 and 2003 (Dudley and Simpfendorfer, 2006). Other sources of removals remain poorly quantified. Reported white shark mortality from bycatch in demersal longline fisheries targeting sharks has been negligible, yet unreported mortality is certainly possible given that white shark in the Southern Indian Ocean inhabit areas with a high risk of fisheries interactions (da Silva et al., 2015; Queiroz et al., 2019).
Current complications for on-going management of white sharks in South Africa include: (1) a lack of reported interactions leading to poorly quantified incidental mortalities from suspected bycatch in commercial, artisanal and recreational fisheries along the southern African coastline (da Silva et al., 2015), (2) the relatively unknown impact of ongoing mortality from the early 1990s to present from the KZNSB Bather Protection Program (Cliff and Dudley, 2011; Kock et al., 2022), (3) uncertainty in regional abundance, with location-specific estimates ranging from ~350 to > 2000 animals (Cliff et al., 1996; Ryklief, 2012; Towner et al., 2013; Hewitt, 2014; Andreotti et al., 2016), and (4) apparently divergent trends in abundance suggested by recent monitoring from different locations (c.f. 2022; Hammerschlag et al., 2019; and DFFE, 2020). Depending on the information source, white sharks could be considered extremely rare in southern Africa and to be rapidly decreasing in abundance, or could be considered relatively abundant and stable. Unreported bycatch may be high or low, leading to cumulative removals or mortality having little effect on productivity and conservation status or having potentially large impacts. Precautionary management would be facilitated by the answers to several questions, including: (1) is it likely that abundance of white sharks in southern Africa has substantially increased following prohibition in 1991? (2) is it likely that ongoing removals have negligible effects on productivity? And (3) what level of removals might be too high and thus concerning if observed in South Africa?
2.2 Simulation approach
We adapted the simulation model described in Bowlby and Gibson (2020) to characterize productivity and predict how varying levels of incidental mortality may influence abundance trends. The first component of this model is a life table analysis describing population productivity, which was used to determine the intrinsic rate of population growth (r) from demographic information (Mollet and Cailliet, 2002; Cortés, 2016). The second component of the model used population projections relative to different levels of removals to demonstrate the population’s capacity for increase and to assess extinction risk.
2.2.1 Population productivity
We derived the intrinsic rate of population growth (r) from the Euler-Lotka equation (McAllister et al., 2001), which is a density-independent model of the maximum rate a population can increase from a severely depleted population size. This is equivalent to the maximum rate of population growth (rmax ) from a density-dependent model for a depleted population (McAllister et al., 2001; Gedamke et al., 2007). It is calculated from age-specific survival rates (lx ) and reproductive output of females (mx ) over their lifespan:
Survival to each age (i) is calculated from instantaneous natural (M) and fishing (F) mortality:
Here, we consider F to represent removals due to incidental human-caused mortality rather than directed fishing mortality. This would include any mortality from bycatch in fisheries off the southern African coast (da Silva et al., 2015; Kock et al., 2022) and mortalities from the KZNSB program (Cliff and Dudley, 2011).
Differences in selectivity over ontogeny are expected for white shark, with juveniles being more susceptible to incidental capture by fisheries (Dewar et al., 2013; Queiroz et al., 2019). From the beach protection program in KZN, no mature females and extremely few adult males were caught from 1978 to 2003 (Dudley and Simpfendorfer, 2006). Captures of adult females from Australia and New Zealand as well as along the southeastern coast of Africa have been documented infrequently from various fisheries (Francis, 1998; Cliff et al., 2000). Other human-caused mortality on adults may also come from long-term exposure and bioaccumulation of pollutants, although impacts on fecundity and longevity have yet to be determined (Huveneers et al., 2018). While the available data are not sufficient to estimate selectivity by age and thus F by age, we allowed for stage-specific differences over ontogeny by assuming that animals age 1 and older were vulnerable to capture, that F was constant for all juvenile ages (e.g. age 1 to age at maturity), and that F on adults (age at maturity to maximum age) dropped to 25% of the rate affecting juveniles. This makes the biological assumption that human-caused mortality on adults is not zero, but is much lower than the instantaneous rate experienced by juveniles. Exploration of alternate assumptions for adults (with F ranging from half (50%) of the rate experienced by juveniles to zero) led to minor differences in the predicted trajectories but did not change overall conclusions. Concentrating fishing pressure on juveniles leads to lower survival at age and thus reduced total reproductive output in the simulations. Given that the white shark population in the southwestern Indian Ocean is concentrated off South Africa (Bonfil et al., 2005; Kock et al., 2022), we consider the population to be closed and do not attempt to estimate immigration or emigration rates.
We originally used information on longevity of white sharks in the southwestern Indian Ocean (Christiansen et al., 2016) when calculating productivity. However, the estimated maximum age (38-40) and age at maturity for females (30) gave a negative value for r (-0.04). If females mature so late, they must live longer to ensure productivity is high enough that the population will not go deterministically extinct. Christiansen et al. (2016) identified two limitations that could lead to underestimated longevity for females: the scarcity of animals > 400 cm fork length and the use of combined data from males and females in the growth model. Comparatively, a mature female of very similar size in the growth model for the Northwest Atlantic led to a similar age at maturity estimate (33 years; Natanson and Skomal, 2015). Even though bomb radiocarbon was used for validation in both studies, the population in the Northwest Atlantic was predicted to have much greater longevity (~70 years), yet attain a smaller theoretical maximum size (c.f. 466.82 and 496.77 cm). We consider it likely that the maximum validated age for white sharks in the southwestern Indian Ocean underestimated longevity, so we used the value from the Northwest Atlantic in the simulations.
Similar to F, survival rates are expected to change over ontogeny, with juveniles experiencing higher mortality than adults (Benson et al., 2018; Dureuil et al., 2021). Therefore, we approximated natural mortality rates (M) from the longevity data based on two new estimators derived specifically for elasmobranchs: ‘Estimator Tmax’ and ‘Estimator Lta’ (Dureuil et al., 2021). This allowed for stage-specific rates of M for juveniles and adults when calculating survival at age. Estimator Tmax gives an estimate of adult mortality based on maximum age (A):
Juveniles represented all animals below female age at maturity and we derived mortality using the associated Estimator Lta:
where the reference age (ta ) was half of maximum age (longevity) and the length corresponding to half maximum age (Lta ) came from a growth function (Dureuil et al., 2021). Interestingly, the inflection point after which growth was asymptotic in a Schnute general model for white sharks was ~35 years at a size of ~450 cm, where maximum age was suggested to be ~70 and length at birth ~150 cm (Natanson and Skomal, 2015). Given the previously identified uncertainty in length at age for white shark in southern Africa, we used average juvenile length (300 cm) to represent L and 450 cm to represent Lta when deriving the juvenile mortality rate from Equation 4. For reproduction, litter size and the sex ratio at birth gave the expected number of female pups per reproductive event, and female age at maturity and gestation period determined the number of reproductive events (Table 1). Recognizing that life history parameters are uncertain for white sharks (Bruce, 2008; Huveneers et al., 2018), we allowed for variability in all vital rates by Monte Carlo sampling within minimum and maximum bounds for each parameter (Caswell et al., 1998; Cortés, 2002; Dans et al., 2003; Bowlby and Gibson, 2020) to generate a distribution for r. Rates of adult and juvenile M were calculated directly from each randomly-drawn value for longevity to ensure compatibility.

Table 1 Population vital rates used in the life table analyses to calculate the intrinsic rate of population growth (r) for South African white shark.
From the life table analyses described above, we calculated a limit reference point called Fcrit , which was estimated by finding the value of F (Equation 2) such that the net reproductive rate equals 1:
This metric represents the level of removals or incidental mortality that would equal the population’s annual potential for growth, such that abundance cannot change and population size remains constant (Campana et al., 2008). Incidental mortality in excess of Fcrit would cause the population to go deterministically extinct. The size of the population required to produce a level of recruitment that equals the number of removals at Fcrit is defined as Ncrit:
The second term corrects for the proportion of the population subject to incidental mortality, which could occur from age 1 onwards. This represents the total number of individuals that would be required to support removals at Fcrit (Campana et al., 2008).
2.2.2 Abundance trends and extinction risk
The simulation used a simple exponential model to project backwards from the terminal year to calculate abundance in the previous year (Nt−1 ) as a function of r, abundance in the current year (Nt ), and removals:
Note that when Nt−1 Nt abundance is declining, which means that a given level of removals is greater than annual population growth via recruitment. We considered the terminal year to be 2017, to avoid the inclusion of years characterized by substantial changes in the monitoring time series (Hammerschlag et al., 2019; Hammerschlag et al., 2022; Towner et al., 2022). We evaluated 11 hypothetical levels of removals using the backwards projections, ranging from 1 to 100 animals per year. While known removals seem to be in the vicinity of 20-40 individuals per year, we evaluated higher levels to explore the potential implications of different magnitudes of unreported bycatch. The lower levels could give goals for management to aspire to, depending on how they influence the abundance trajectory. For reference, we also incorporated a zero removals scenario (F = 0) to determine the maximum potential rate of increase for the population following conservation protection in 1991. While not realistic because of known ongoing mortality, the zero removals scenario gives the upper limit to abundance increase based on life history characteristics alone. If historical and current removals become known with high certainty (i.e. the annual time series of white shark mortalities from the bather protection program can be added with annual estimates of bycatch), there would be the potential to account for annual variability in removals to increase biological plausibility of the backward projections. This would allow greater certainty in how white shark abundance changed following prohibition in 1991, and how much historical mortality would be expected to influence future capacity for population growth.
From the terminal year, we also projected the population forwards using an exponential model to evaluate extinction risk under the same levels of removals. In the forward projections, the assumed level of historical removals reduced the population’s capacity for increase (r) by reducing survival at age (as in Equation 2). Future removals affected the abundance trajectory (Nt , as in Equation 5). A low level of environmental variability effecting annual population growth was incorporated through autocorrelated deviates on r, as in Bowlby and Gibson (2020). This increased the biological realism of the forwards projections by allowing the population growth rate to vary slightly from year to year.
2.2.3 Scenarios
In the parameterization of the life history simulations, we generated four life history scenarios to capture the main sources of uncertainty relating to white shark longevity and age at maturity, as well as abundance in the terminal year. These four simulated scenarios included: (1) Longer Lifespan, (2) Shorter Lifespan, (3) Smaller Population, and (4) Larger Population, described below. With respect to white shark longevity and age at maturity, age determination for sharks is uncertain even when validated with modern techniques like bomb radiocarbon (Cailliet, 2015). While we argued that the estimated age at maturity from Christiansen et al. (2016) was likely more representative than the associated longevity estimate for female white sharks, we considered two different scenarios for lifespan. We set longevity at 70 years and age at maturity at 30 years in the Longer Lifespan scenario and compared this to a Shorter Lifespan scenario with a longevity of 40 years and age at maturity of 10 years (Bowlby and Gibson, 2020).
Abundance in the terminal year (2017) must be initialized in the projections of the simulation model to calculate population trends relative to different levels of removals (Bowlby and Gibson, 2020; Bowlby and Cortés, 2020). While there are several local abundance estimates for white sharks in South Africa derived from mark-recapture data (e.g., Cliff et al., 1996; Ryklief, 2012; Towner et al., 2013; Hewitt, 2014; Andreotti et al., 2016), predicted population sizes range widely, even from adjacent years (e.g. Cliff et al., 1996; c.f. Towner et al., 2013 and Andreotti et al., 2016). A limitation of these analyses when estimating abundance is the assumption of homogeneous survival and capture probabilities among individuals, even though heterogeneity arising from resident behavior and sex or size-based population structuring is known for white sharks in the southwestern Indian Ocean (Hewitt, 2014; Irion et al., 2017; Hewitt et al., 2018). Because the magnitude of estimation bias for abundance is unknown, we initialized population size in the Longer Lifespan and Shorter Lifespan scenarios to range between 700 and 1300 individuals, with a median of 1000. These values are moderately larger than the most recent abundance estimates focused on the Gansbaai aggregation site of 438 (Andreotti et al., 2016) and 908 (Towner et al., 2013) individuals. Both of these mark-recapture studies used data up to 2011.
Due to the debate on the extent to which abundance estimates using data from localized aggregations represent white shark abundance throughout southern Africa (e.g. Irion et al., 2017), we consider 1000 animals to be reasonable on the basis of previous research. However, we also evaluated two scenarios that allowed for a wider range. These incorporated the life history parameters from the Longer Lifespan scenario, given that they are likely more representative. In the Smaller Population scenario, we initialized abundance at a median of 500 animals, which is similar to the estimate of Andreotti et al. (2016). In the Larger Population scenario, we initialized abundance at a median of 2000 animals, which is closer to estimates from the 1990s in South Africa (Cliff et al., 1996) as well as to those derived for white sharks in other populations (Burgess et al., 2014; Hillary et al., 2018).
To describe population trends in the backwards and forwards projections for each scenario, we summarized abundance trajectories using lognormal regression, where the slope estimate gives the annual population growth rate (Bowlby and Gibson, 2020). To characterize relative risk to the population from various levels of removals, we evaluated the proportion of the future projections that were declining as well as assessed extinction risk as the proportion of population trajectories that went below a quasi-extinction threshold of 10 animals after one generation (53 years; Rigby et al., 2019). While we recognize that there is considerable debate on how true extinction is measured (e.g. Dulvy et al., 2004) and/or how minimum viable population sizes are characterized (e.g., Traill et al., 2007), such a small population would be unlikely to maintain reproductive output and could be considered functionally extinct.
3 Results
Based on the life table analyses, the potential for white sharks in southern African waters to increase in abundance is naturally low. The intrinsic rate of population growth (r) was 0.026 (80th percentiles = 0.010, 0.042) in the Longer Lifespan scenario and 0.076 (0.038, 0.129) in the Shorter Lifespan Scenario. Even with the large difference in r, the expected lifetime reproductive output from a single female was only marginally higher in the Shorter Lifespan scenario (c.f. median values of 3.96 and 3.14). This meant that higher survival to maturity (Shorter Lifespan) outweighed the additional 10 years over which reproduction occurred (Longer Lifespan) when characterizing lifetime reproductive output.
The total number of animals required to balance removals at Fcrit (i.e. to ensure that gains from reproduction were equal to removals) increased dramatically as removals increased (Table 2). In the Shorter Lifespan scenario, removals of one animal per year could be balanced by the annual reproductive output from a median of 11 animals (80th percentiles = 7, 20), yet if removals were 30 animals per year, this jumped to 320 animals, and so on up to 1066 animals if removals were 100 animals per year (Table 2). In the Longer Lifespan scenario, 33 animals (80th percentiles = 20, 84) would balance out the removal of one white shark per year, while 982 animals would be required if removals were 30 per year, increasing up to 3272 animals if removals were 100 per year (Table 2). It is noteworthy that the number of animals contributing to reproductive output needed to increase by more than two orders of magnitude over the different levels of removals, just to keep overall abundance constant. Because the intrinsic rate of increase (r) is the same for the Longer Lifespan, Larger Population and Smaller Population scenarios, it meant that the rate of abundance increase in the absence of removals as well as predictions of Ncrit were the same (Table 2).
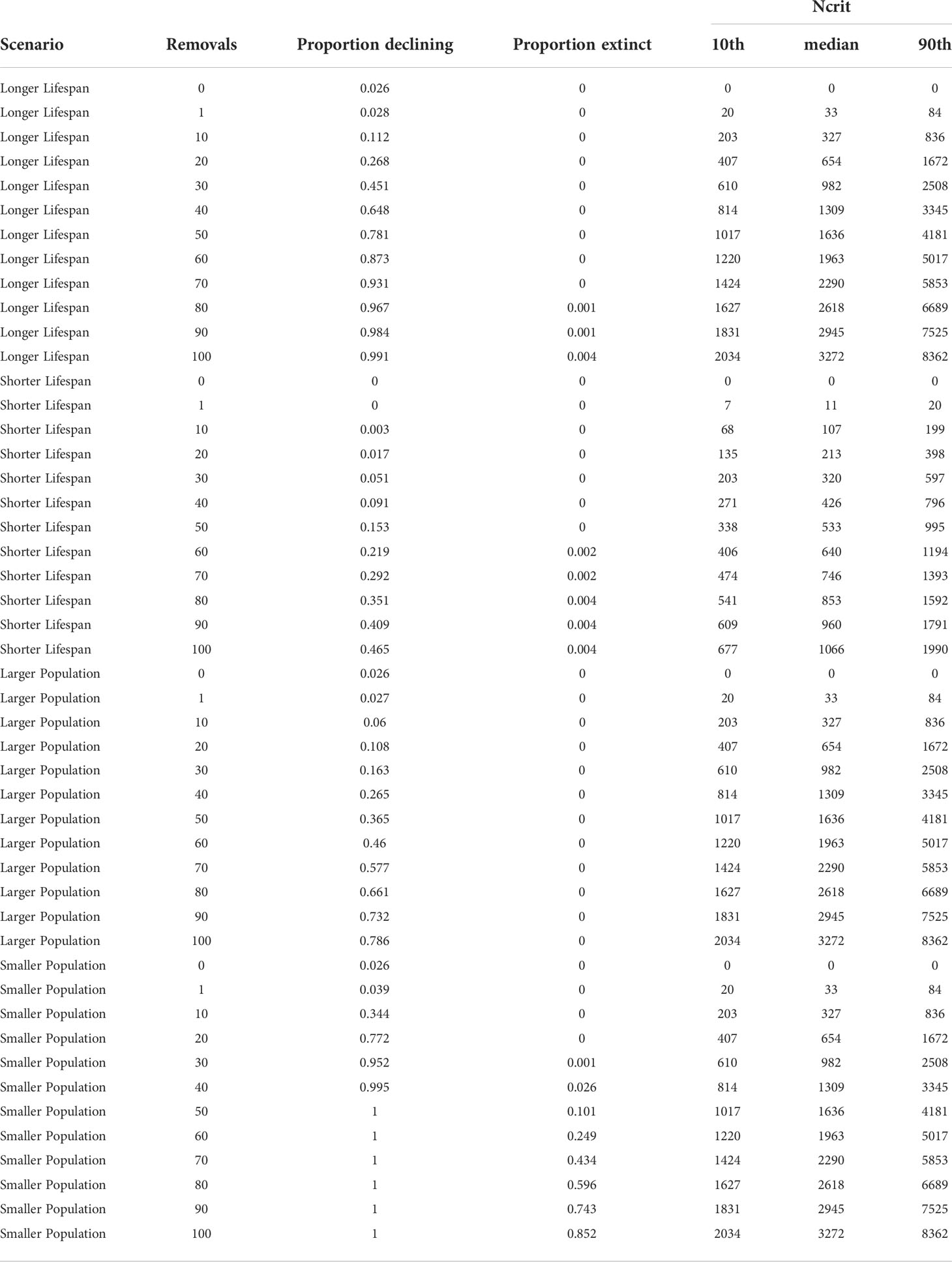
Table 2 The proportion of simulations that were declining and the proportion that reached the extinction threshold in the future projections (2018-2070; one generation). The median (and 80th percentile) number of females that would be required to balance removals so that abundance remained constant (Ncrit) is also shown for each life history scenario and removal level.
After white shark were protected in South African waters, annual removals needed to remain low in order to allow abundance increase. If removals were zero and white shark could increase at their theoretical maximum level, median predicted abundance would be 2.03 times higher in 2017 as compared with 1991 in the Longer Lifespan, Larger Population and Smaller Population scenarios, and 7.77 times higher in the Shorter Lifespan scenario. However, the potential for increase dropped dramatically as removals increased under all life history scenarios. If a single white shark was removed each year, annual population growth estimates ranged from 2.4-7.3% among scenarios. If 10 animals were removed each year, this range dropped to 0.1-5.3% (Figure 1; left panels). Once removals were 50 animals, median slopes were negative in two scenarios (Longer Lifespan, Smaller Population) and were consistently negative when removals were 100 animals per year (Figure 1; left panels). To achieve any appreciable amount of abundance increase from 1991-2017, removals needed to remain below 10 animals per year, except in the Shorter Lifespan scenario where they could be as high as 30 per year and still allow abundance to double. In addition, to have > 50% of simulated trajectories increase in abundance from 1991 to 2017, removals needed to be less than 30 animals per year in the Longer Lifespan scenario, less than 80 animals per year in the Shorter Lifespan scenario, approximately 50 animals per year or less in the Larger Population scenario and approximately 10 animals or less in the Smaller Population scenario (Figure 2). To achieve either a high probability of abundance increase and/or for abundance increase to have been substantial, annual removals needed to have been very low from 1991 onwards.
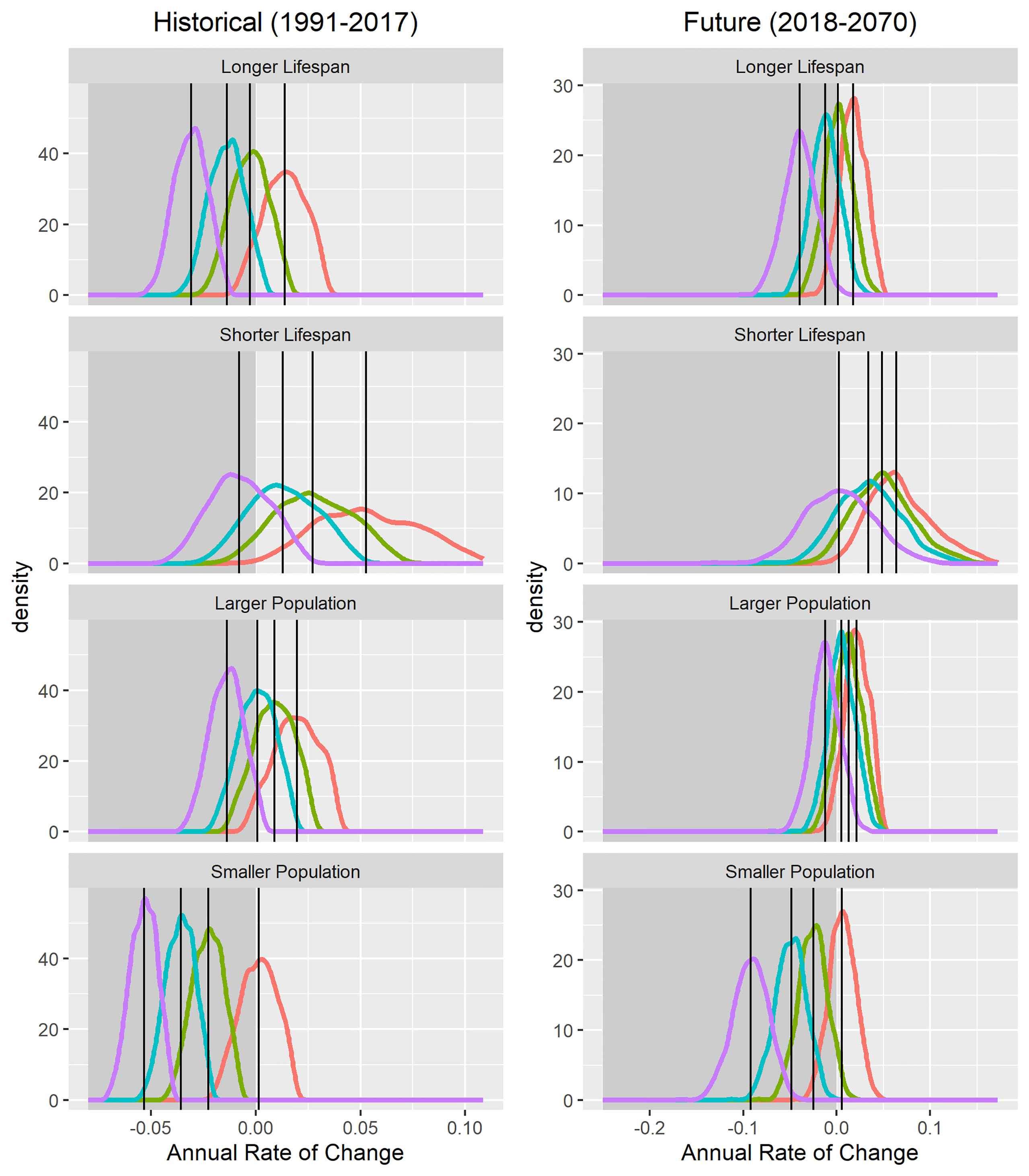
Figure 1 A comparison of the distribution of annual rates of abundance change from lognormal regression under four levels of removals: 10 (red lines), 30 (green lines), 50 (blue lines) and 100 (purple lines) animals per year in the backwards projections (left panels) and forwards projections (right panels) of the simulation model for four life history scenarios. The median of each distribution is identified by a vertical line and the dark grey shading identifies values < 0 (indicative of abundance decline).
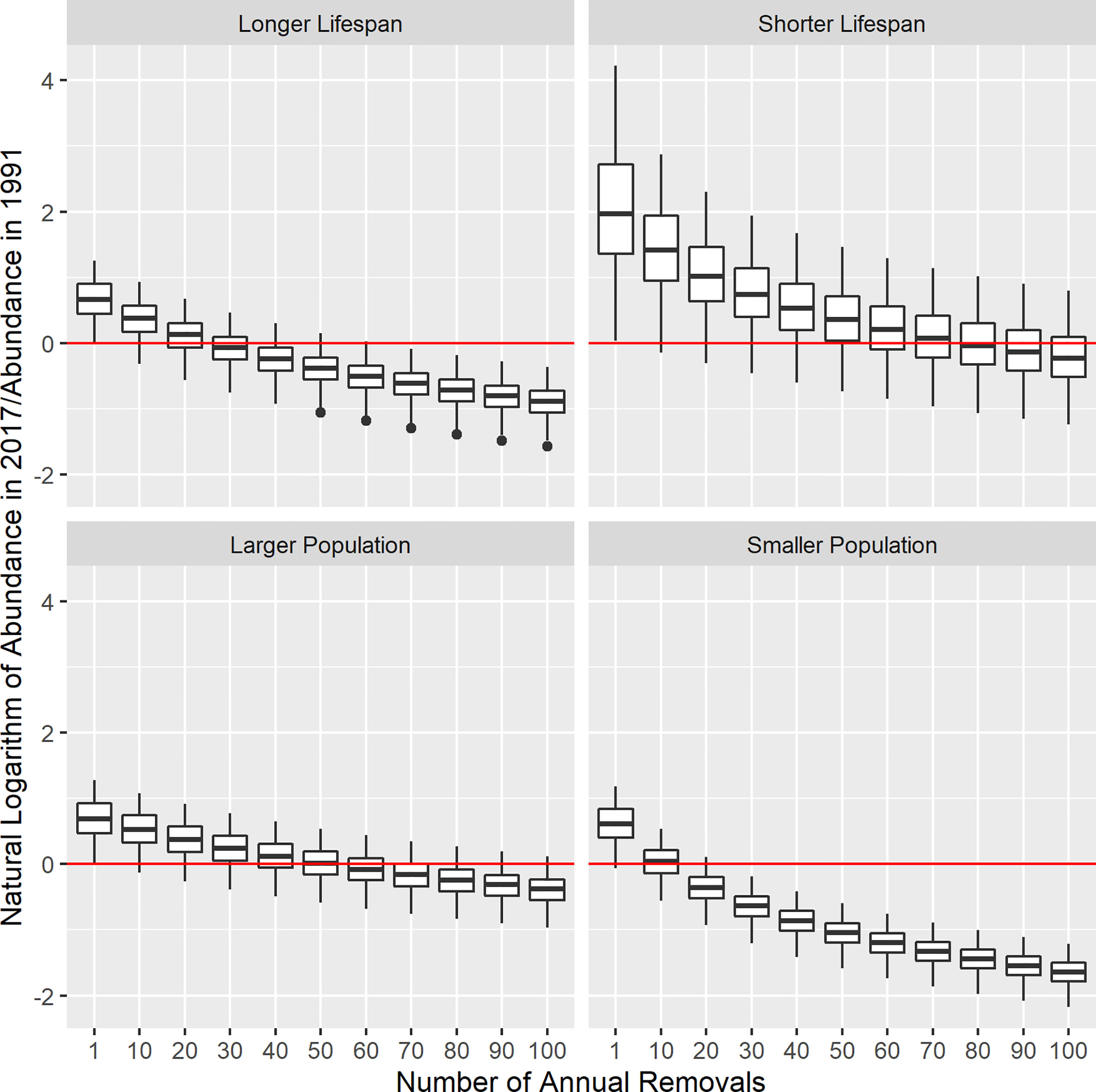
Figure 2 Boxplots representing the ratio of abundance in the terminal year (2017) as compared to predicted abundance in 1991, plotted on a log scale for each life history scenario and level of removals. The horizontal line represents the median, the box is drawn around the first and third quartile and the whiskers approximate a 95% confidence interval. Positive values indicate abundance increase, negative values indicate decline, with the red horizontal line at zero (no change). The median value must be > 0 for 50% of simulations to be increasing from 1991 to 2017.
This result was the same for the future projections of the next generation of white shark in southern Africa (Figure 1; right panels). In particular, the Longer Lifespan and Smaller Population scenarios were associated with substantial probabilities of abundance decline at relatively low levels of annual removals (Figure 3). Removals of< 40 animals per year in the former and< 20 animals per year in the latter were associated with > 50% of forward projections declining in abundance (Table 2). At the highest level of removals considered, abundance decline occurred in 99.1 and 100% of simulations, respectively. Even when the majority of trajectories were declining, the probability of extinction remained relatively low in the Longer Lifespan scenario. This was in stark contrast to the Smaller Population scenario, where abundance quickly declined to the extinction threshold (10 animals) in 85.2% of simulations at the highest level of removals (Table 2). In the Shorter Lifespan scenario, the projections that underwent abundance decline did so relatively rapidly, resulting in similar extinction probabilities as in the Longer Lifespan scenario even though a lower proportion of simulations were in decline (Table 2). The projections were slightly more optimistic if the population was characterized by a higher intrinsic rate of increase (Shorter Lifespan) or if current abundance was more than double any recent estimate (Larger Population): if removals remained below 100 and 70 animals per year, respectively, the majority of future projections increased over the next generation (Table 2), yet the magnitude of this increase would be extremely small (Figure 3).
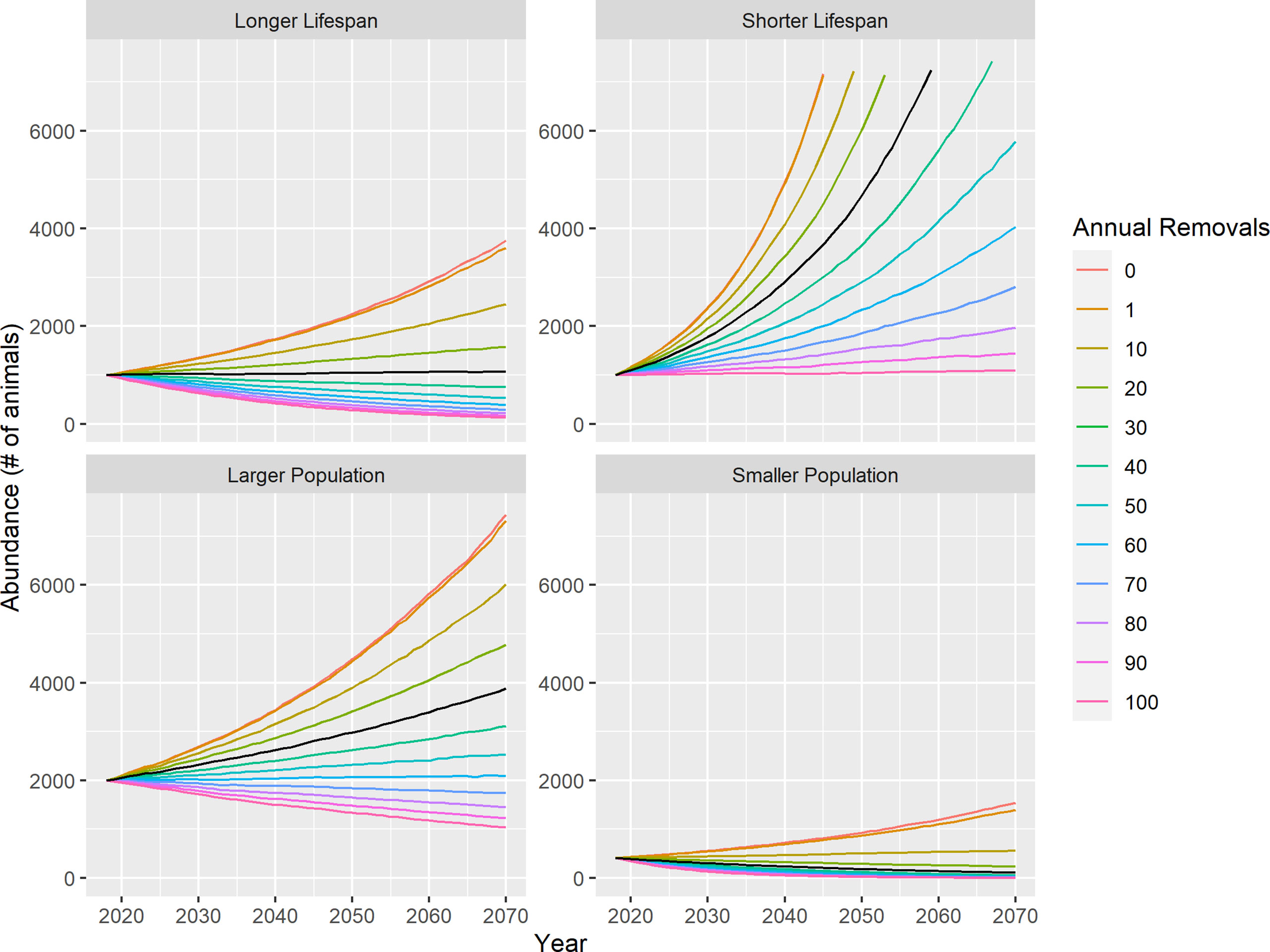
Figure 3 Predicted median abundance for the four life history scenarios at each level of removals, when abundance was projected one generation (53 years) into the future for white shark in southern Africa (2018-2070). The black line identifies removals of 30 animals per year, which is approximately the long-term average for mortalities from the KZNSB bather protection program.
4 Discussion
Several general considerations for the precautionary management of at-risk sharks can be derived from this case study. First, it is necessary to consider longevity and underlying population productivity when trying to interpret trends in monitoring indices or to evaluate the plausibility of life history information. Through a simple calculation of r, it became obvious that there was an inconsistency in current understanding of age at maturity relative to longevity for white sharks in the southwestern Indian Ocean (Christiansen et al., 2016). Similarly, annual gains that exceed the reproductive capacity of a population demonstrate that monitoring is not indexing abundance, but is influenced by factors such as observation error, spatial redistribution or movement, and/or changes in catchability or observability (e.g., Maunder et al., 2006). In our case study, the maximum rate of annual increase was only 2.6% if removals were zero when female white sharks mature in their 30s (Christiansen et al., 2016) and are as long-lived as currently thought (Natanson and Skomal, 2015). This annual rate of increase would be lower if productivity in white shark was density dependent (Walker, 1998). This limited capacity for increase from recruitment alone argues strongly that extreme gains in monitoring indices over a short time periods (DFFE, 2020) arose primarily from other factors for white sharks in southern Africa. Recovery for such long-lived species would take decades, rather than being evident after relatively few years.
Second, the impression that low levels of ongoing mortality have negligible impact on recovery potential should be explicitly evaluated. Our case study demonstrates that the level of mortality experienced by white sharks immediately upon protection in South Africa could still have caused regional abundance decline, particularly in specific years. Removals in the range of 20 - 40 animals per year represented a tipping point between abundance increase and decline in 2 of 4 scenarios. For example, more than 50% of trajectories were predicted to increase when removals were 20 animals per year in the Longer Lifespan and Smaller Population scenarios, switching to more than 50% of trajectories predicted to decline when removals were 40 animals per year. Only the Larger Population scenario and Shorter Lifespan scenarios could support removals in excess of 40 animals per year without experiencing decline. It is concerning that levels of ongoing mortality from the KZNSB program are associated with no significant change in CPUE of white sharks (Dudley and Simpfendorfer, 2006; https://www.shark.co.za/Pages/ReducingCatches) because it implies that ongoing mortality from the program is sufficient to prevent population growth. However, this conclusion only holds if the KZNSB data indexes regional abundance rather than local patterns (Cliff et al., 1989; Dudley and Simpfendorfer, 2006). Given the dramatic changes in ecological structure at previous aggregation sites, as well as the divergent trends in other available monitoring indices from False Bay and Gansbaai, the development of a regional abundance index should be prioritized to better track changes in status.
Third, while prohibition is a useful first step, it does not replace the need to quantify other sources of mortality affecting at-risk shark populations. How abundance may change in future years critically depends on current population size as well as future removals. In southern Africa, there remains a diversity of commercial, subsistence and recreational fisheries in addition to other threats that may be compounding annual mortality of white sharks. For commercial fisheries, spatial risk of incidental capture from offshore and demersal longliners has been evaluated (e.g., Queiroz et al., 2019; Kock et al., 2022), yet temporal overlap and bycatch magnitude has not been. In addition, the spatiotemporal overlap of other large-scale, small-scale and/or coastal operations remains largely unknown. For instance, there are numerous types of shark fisheries within South Africa’s EEZ (DAFF, 2012; da Silva et al., 2015) as well as off neighbouring countries along the African coastline (Roux and Shannon, 2004). Compounding any direct mortality from captures would be indirect mortality arising from displacement to less optimal areas, prey limitation, and/or environmental stressors such as pollution (da Silva et al., 2015; Hammerschlag et al., 2019; Merely et al., 2019). Finally, the near-disappearance of immature white sharks from Gansbaai since 2017 has been linked to predation risk from transient killer whales (Orcinus orca) (Towner et al., 2022), which raises the possibility that natural mortality rates may also be increasing. This could easily lead to a scenario where unmonitored mortality from any one source is relatively low (e.g. a few animals each year), but collectively high enough to constitute an ongoing threat. Improved monitoring is sorely needed for white shark in South Africa, particularly in light of other ecological changes that may constitute emerging threats. In such situations, implementing successful regional monitoring would be logistically complex as well as dependent on government initiative, and would need to be backed by strong stakeholder and industry support (Pfaff et al., 2019).
Lastly, using a simulation model as a mechanism for hypothesis testing is a useful way to demonstrate the logical outcome of different states of nature that can then be compared to existing information. The population trajectories, growth rates, and probabilities of decline or extinction presented here are not intended as absolute predictions of the future or past dynamics of white shark in southern Africa. However, one interesting result is that the Smaller Population and Shorter Lifespan scenarios seemed to be largely incompatible with known removals as well as the trends in the available monitoring data. Even without accounting for how environmental and demographic stochasticity puts small populations at greater risk of extinction (Caughley, 1994; Dulvy et al., 2004), the Smaller Population scenario produced fewer total recruits in a given year than a larger population, simply because there were fewer females. Consequently, fewer removals resulted in greater rates of abundance decline than in other scenarios. Annual population growth rates became progressively more negative when removals exceeded 10 individuals per year (Figure 1), suggesting that abundance would have progressively declined from 1991 onwards to reach ~500 animals by 2017, and would be expected to continue to decline over the next generation with high probability. Even though known removals likely ranged from 20-40 animals per year throughout the 1990s and 2000s (Cliff and Dudley, 2011; Kock et al., 2022), there was no evidence of long-term abundance decline from the CPUE index derived from the beach protection program (Dudley and Simpfendorfer, 2006) or from a comparison of mean catches pre- and post-2007 (https://www.shark.co.za/Pages/ReducingCatches). Until very recently, there was little evidence of precipitous decline in the available monitoring data from Gansbaai and False Bay (Hammerschlag et al., 2019; Hammerschlag et al., 2022; Johnson and Kock, 2006; Towner et al., 2016). The simplest explanation for these discrepancies is that modelled population size was unrealistically low (Irion et al., 2017) and that white shark are currently more abundant in South African waters than suggested by Andreotti et al. (2016). Similarly, the lack of progressive and substantial increase in the monitoring data when removals are low suggests that the Shorter Lifespan scenario is unlikely to characterize population dynamics. For removals in the vicinity of 20-40 animals per year, a population characterized by the Shorter Lifespan should have doubled in abundance from 1991 to 2017, unless unmonitored mortality was very high. The abundance trajectory was stable in the Shorter Lifespan scenario only when removals ranged between 70-80 animals per year. These results highlight the need to refine absolute estimates of population size, potentially by implementing alternative techniques such as close-kin mark-recapture (Bravington et al., 2016; Ruzzante et al., 2019).
Specifically for white sharks in southern Africa, continued conservation concern is likely warranted, given the sensitivity of the species to low levels of removals. All scenarios indicated that removals in the 10s of individuals substantially limited the potential for and magnitude of any abundance increase following prohibition in 1991. When results were compared with known average removals from the KZNSB, it seems likely that the landings prohibition essentially maintained the status quo rather than provided substantial conservation benefit to white shark in South Africa. Since the 1990s, the KZNSB program reduced pelagic shark mortality through a substantial (~50%) reduction in net length and partial replacement of nets with drumlines (Dicken et al., 2016; Dicken et al., 2018) while maintaining the societal and socioeconomic benefits associated with the program (Cliff and Dudley, 1992; Cliff and Dudley, 2011). However, there has been no significant difference in the mean annual catch or CPUE of white shark pre-2007 and post-2007 (https://www.shark.co.za/Pages/ReducingCatches), suggesting that removals of white shark will continue at similar levels as long as the program is in operation. Under the most probable life history scenario (Longer Lifespan), ongoing mortality of 20 animals per year was associated with 27% of future trajectories declining, which increased to 45% if removals were 30 animals per year and to 65% if removals were 40 per year.
To develop more targeted advice for future management of white sharks in South Africa, it would be necessary to address remaining sources of uncertainty. Although we were able to demonstrate that abundance is likely greater than estimated by Andreotti et al. (2016), future recovery potential depends on whether current abundance remains larger, and on how much larger. The Longer Lifespan scenario already assumed that abundance was greater than the Towner et al. (2013) estimate, and thus has the potential to overestimate recovery potential from 2017 onwards. Additionally, a better understanding of removals has become critical in light of recent ecological changes and increased Orca predation. Even if average known removals kept abundance relatively constant from 1991-2017, these new and potentially substantial threats are associated with precipitous declines at established aggregation sites from 2017 onward. Tracking changes in status will depend on improved monitoring at a regional level. In particular, monitoring that expands coverage into the Eastern Cape given the potential for redistribution. However, unless we make the assumption that exclusion from previous habitat plus additional predation does not lead to additional mortality, these threats will compound with known removals. Even if all known removals were halted immediately, appreciable abundance increase would still take decades to manifest given the most probable life history characteristics of white sharks in southern Africa. To be precautionary until abundance and population trajectory are known with greater certainty, managers should consider that: (1) substantial increases in abundance following prohibition were very unlikely, (2) on-going removals limit productivity and are likely high enough to be causing abundance decline, particularly if recent estimates of population size are accurate, and (3) this makes current levels of removals concerning when considered within the context of other ecological changes in South Africa.
Globally, extinction risk for sharks and rays remains high (Dulvy et al., 2021) and there is a pressing need to develop new methods that can be applied to existing data to promote conservation for numerous populations (Jorgensen et al., 2022). Relatively simple simulation models can provide a mechanism to explore the biological implications of different life history assumptions, assess the consistency of different types of data (e.g. longevity and trends), determine the potential for population increase following protection, and evaluate relative risks associated with various levels of threats. Ultimately this case study demonstrates that effective conservation remains a challenge for white sharks in South Africa, one that may require additional measures to ensure functional protection.
Data availability statement
The original contributions presented in the study are included in the article/supplementary material. Further inquiries can be directed to the corresponding author.
Author contributions
EG conceived the work; HB developed and undertook analyses and wrote MS; NH, EG and DI contributed to analyses and edited MS. All authors contributed to the article and approved the submitted version. Publishing was supported by the South African Institute for Aquatic Biodiversity.
Funding
This research was supported by the South African Institute for Aquatic Biodiversity.
Acknowledgments
We thank Dr. Jamie Gibson who developed the original simulation code that was adapted for this work, as well as Matt Dicken and Allison Towner for their perspectives and engaging discussion on white shark in South Africa.
Conflict of interest
The authors declare that the research was conducted in the absence of any commercial or financial relationships that could be construed as a potential conflict of interest.
Publisher’s Note
All claims expressed in this article are solely those of the authors and do not necessarily represent those of their affiliated organizations, or those of the publisher, the editors and the reviewers. Any product that may be evaluated in this article, or claim that may be made by its manufacturer, is not guaranteed or endorsed by the publisher.
References
Andreotti S., Rutzen M., van der Walt S., Von der Heyden S., Henriques R., Meÿer M., et al. (2016). An integrated mark-recapture and genetic approach to estimate the population size of white sharks in south Africa. Mar. Ecol. Prog. Ser. 552, 241–253. doi: 10.3354/meps11744
Benson J. F., Jorgensen S. J., O'Sullivan J. B., Winkler C., White C. F., Garcia-Rodriguez E., et al. (2018). Juvenile survival, competing risks, and spatial variation in mortality risk of a marine apex predator. J. Appl. Ecol. 55 (6), 2888–2897. doi: 10.1111/1365-2664.13158
Bonfil R., Meÿer M., Scholl M. C., Johnson R., O'Brien S., Oosthuizen H., et al (2005). Transoceanic migration, spatial dynamics, and population linkages of white sharks. Science 310 (5745), 100–103. doi: 10.1126/science.1114898
Bowlby H. D., Cortés E. (2020). An incidental catch model for porbeagle assessment and status evaluation. Collect. Vol. Sci. Pap. ICCAT. 77 (6), 132–147.
Bowlby H. D., Gibson A. J. F. (2020). Implications of life history uncertainty when evaluating status in the Northwest Atlantic population of white shark (Carcharodon carcharias). Ecol. Evol. 10, 4990–5000. doi: 10.1002/ece3.6252
Bravington M. V., Skaug H. J., Anderson E. C. (2016). Close-kin mark-recapture. Stat. Sci. 31 (2), 259–274. doi: 10.1214/16-STS552
Bruce B. D. (2008). “The biology and ecology of white shark, carcharodon carcharias,” in Sharks of the open ocean; biology, fisheries and conservation. Eds. Camhi M. D., Pikitch E. K., Babcock E. A. (Oxford, UK: Blackwell Publishing), 69–81.
Burgess G. H., Bruce B. D., Cailliet G. M., Goldman K. J., Grubbs R. D., Lowe C. G., et al. (2014). A re-evaluation of the size of the white shark (Carcharodon carcharias) population off California, USA. PloS One 9, e98078. doi: 10.1371/journal.pone.0098078
Cadrin S. X. (2020). Defining spatial structure for fishery stock assessment. Fish. Res. 221, 105397. doi: 10.1016/j.fishres2019.105397
Cailliet G. M. (2015). Perspectives on elasmobranch life-history studies: a focus on age validation and relevance to fishery management. J. Fish Biol. 87, 1271–1292. doi: 10.1111/jfb.12829
Campana S. E., Gibson J., Brazner J., Marks L., Joyce W. (2008). Status of basking sharks in Atlantic Canada. DFO Can. Sci. Advis. Sec. Sci. Sci. Advis. 2008/004, vi + 61.
Caswell H., Brault S., Read A. J., Smith T. D. (1998). Harbor porpoise and fisheries: uncertainty in analysis of incidental mortality. Ecol. Appl. 8, 1226–1238. doi: 10.1890/1051-0761(1998)008[1226:HPAFAU]2.0.CO;2
Caughley G. (1994). Directions in conservation biology. J. Anim. Ecol. 63, 215–244. doi: 10.2307/5542
Christiansen H. M., Campana S. E., Fisk A. T., Cliff G., Wintner S. P., Dudley S. F., et al. (2016). Using bomb radiocarbon to estimate age and growth of the white shark, Carcharodon carcharias, from the southwestern Indian ocean. Mar. Biol. 163 (6), 1–13. doi: 10.1007/s00227-016-2916-9
Christiansen H. M., Lin V., Tanaka S., Velikanov A., Mollet H. F., Wintner S. P., et al. (2014). The last frontier: catch records of white sharks (Carcharodon carcharias) in the Northwest pacific ocean. PloS One 9, e94407. doi: 10.1371/journal.pone.0094407
Cliff G., Dudley S. F. (1992). Protection against shark attack in south Africa 1952-90. Mar. Freshw. Res. 43 (1), 263–272. doi: 10.1071/MF9920263
Cliff G., Dudley S. F. (2011). Reducing the environmental impact of shark-control programs: a case study from KwaZulu-natal, south Africa. Mar. Freshw. Res. 62 (6), 700–709. doi: 10.1071/MF10182
Cliff G., Dudley S. F. J., Davis B. (1989). Sharks caught in the protective gill nets off natal, south africa. 2. the great white shark Carcharodon carcharias (Linnaeus). S. Afr. J. Mar. Sci. 8 (1), 131–144. doi: 10.2989/02577618909504556
Cliff G., Dudley S. F. J., Jury M. R. (1996). “Catches of white sharks in KwaZulu-natal, south Africa and environmental influences,” in Great white sharks: the biology of carcharodon carcharias. Eds. Klimley A. P., Ainley D. G. (San Diego, CA: Academic Press), 351–362.
Cliff G., Compagno L. J.V., Smale M. J., van der Elst R. P., Wintner S. P. (2000) First records of white sharks, Carcharodon carcharias, from Mauritius, Zanzibar, Madagascar and Kenya. S. Afr J. Mar. Sci. 96, 365–367. doi: 10.10520/AJA00382353_8942
Compagno L. J. V. (1991). Government protection for the great white shark (Carcharodon carcharias) in South Africa. South African Journal of Science 87(7), 284–285. doi: 10.10520/AJA00382353_6665
Cortés E. (2002). Incorporating uncertainty into demographic modeling: application to shark populations and their conservation. Conserv. Biol. 16, 1048–1062. doi: 10.1046/j.1523-1739.2002.00423.x
Cortés E. (2016). Perspectives on the intrinsic rate of population growth. Methods Ecol. Evol. 7, 1136–1145. doi: 10.1111/2041-210X.12592
DAFF (2012) Status of south African marine fishery resources 2012. department of agriculture, forestry and fisheries. south Africa. Available at: https://www.nda.agric.za/docs/media/STATUS%20REPORT%202012FINAL%20DRAFT.pdf (Accessed December 2021).
Dans S. L., Koen-Alonso M., Pedraza S. N., Crespo E. A. (2003). Incidental catch of dolphins in trawling fisheries off Patagonia, Argentina: can populations persist? Ecol. Appl. 13, 754–762. doi: 10.1890/1051-0761(2003)013[0754:ICODIT]2.0.CO;2
da Silva C., Booth A. J., Dudley S. F. J., Kerwath S. E., Lamberth S. J., Leslie R. W., et al. (2015). The current status and management of south africa's chondrichthyan fisheries. Mar. Sci. 37 (2), 233–248. doi: 10.2989/1814232X.2015.1044471
Dewar H., Eguchi T., Hyde J., Kinzey D. H., Kohin S., Moore J., et al. (2013)Status review of the northeastern pacific population of white sharks (Carcharodon carcharias) under the endangered species act. In: NOAA. Available at: https://repository.library.noaa.gov/view/noaa/17705 (Accessed January 2022).
DFFE (2020)Review of the south African national plan of action for the conservation and management of sharks. In: Department of environment, forestry and fisheries. south Africa. Available at: https://www.dffe.gov.za/sites/default/files/reports/sharksconservationmanagement_nationaplanofaction_review2020october.pdf (Accessed November 2020).
Dicken M. L., Cliff G., Winker H. (2016). Sharks caught in the KwaZuluNatal bather protection programme, south africa. 13. the tiger shark Galeocerdo cuvier. Afr. J. Mar. Sci. 38, 285–301. doi: 10.2989/1814232X.2016.1198276
Dicken M. L., Winker H., Smale M. J., Cliff G. (2018). Sharks caught in the KwaZulu-natal bather protection programme, south africa. 14. the smooth hammerhead shark Sphyrnazygaena (Linnaeus). Afr. J. Mar. Sci. 40 (2), 157–174. doi: 10.2989/1814232X.2018.1470031
Domeier M. L., Nasby-Lucas N. (2013). Two-year migration of adult female white sharks (Carcharodon carcharias) reveals widely separated nursery areas and conservation concerns. Anim. Biotelemetry. 1 (1), 1–10. doi: 10.1186/2050-3385-1-2
Dudley S., Simpfendorfer C. (2006). Population status of 14 shark species caught in the protective gillnets off KwaZulu-natal beaches, south africa-2003. Mar. Freshw. Res. 57 (2), 225–240. doi: 10.1071/MF05156
Dulvy N. K., Ellis J. R., Goodwin N. B., Grant A., Reynolds J. D., Jennings S. (2004). Methods of assessing extinction risk in marine fishes. Fish Fish. 5, 255–276. doi: 10.1111/j.1467-2679.2004.00158.x
Dulvy N. K., Fowler S. L., Musick J. A., Cavanagh R. D., Kyne P. M., Harrison L. R. (2014). Extinction risk and conservation of the world’s sharks and rays. Elife 3, e00590. doi: 10.7554/eLife.00590
Dulvy N. K., Pacoureau N., Rigby C. L., Pollom R. A., Jabado R. W., Ebert D. A., et al. (2021). Overfishing drives over one-third of all sharks and rays toward a global extinction crisis. Curr. Biol. 31 (21), 4773–4787. doi: 10.1016/j.cub.2021.08.062
Dureuil M., Aeberhard W. H., Burnett K. A., Hueter R. E., Tyminski J. P., Worm B. (2021). Unified natural mortality estimation for teleosts and elasmobranchs. Mar. Ecol. Prog. Ser. 667, 113–129. doi: 10.3354/meps13704
Fausch K. D., Torgersen C. E., Baxter C. V., Li H. W. (2002). Landscapes to riverscapes: bridging the gap between research and conservation of stream fishes: a continuous view of the river is needed to understand how processes interacting among scales set the context for stream fishes and their habitat. BioScience 52 (6), 483–498. doi: 10.1641/0006-3568(2002)052[0483:LTRBTG]2.0.CO;2
Francis M. P. (1998). “Observations on a pregnant white shark with a review of reproductive biology,” in Great white sharks: the biology of carcharodon carcharias. Eds. Klimley A. P., Ainley D. G. (San Diego: Academic Press), 157–172.
Gedamke T., Hoenig J. M., Musick J. A., DuPaul W. D., Gruber S. H. (2007). Using demographic models to determine intrinsic rate of increase and sustainable fishing for elasmobranchs: pitfalls, advances, and applications. N. Am. J. Fish. Manage. 27 (2), 605–618. doi: 10.1577/M05-157.1
Hammerschlag N., Fallows C., Meÿer M., Seakamela S. M., Orndorff S., Kirkman S., et al. (2022). Loss of an apex predator in the wild induces physiological and behavioural changes in prey. Biol. Lett. 18, 20210476. doi: 10.1098/rsbl.2021.0476
Hammerschlag N., Sulikowski J. (2011). Killing for conservation: the need for alternatives to lethal sampling of apex predatory sharks. Endanger. Species Res. 14, 135–140. doi: 10.3354/esr00354
Hammerschlag N., Williams L., Fallows M., Fallows C. (2019). Disappearance of white sharks leads to the novel emergence of an allopatric apex predator, the sevengill shark. Sci. Rep. 9, 1908. doi: 10.1038/s41598-018-37576-6
Hewitt A. M. (2014). Demographics of a seasonal aggregation of white sharks at seal island, false bay, south Africa (Cape Town, SA: University of Cape Town).
Hewitt A. M., Kock A. A., Booth A. J., Griffiths C. L. (2018). Trends in sightings and population structure of white sharks, Carcharodon carcharias, at seal island, false bay, south Africa, and the emigration of subadult female sharks approaching maturity. Environ. Biol. Fishes. 101 (1), 39–54. doi: 10.1007/s10641-017-0679-x
Hilborn R. (2003). The state of the art in stock assessment: where we are and where we are going. Scientia Marina 67 (S1), 15–20. doi: 10.3989/scimar.2003.67s115
Hillary R. M., Bravington M. V., Patterson T. A., Bradford R., Feutry P., Gunasekera R., et al. (2018). Genetic relatedness reveals total population size of white sharks in eastern Australia and new Zealand. Nature. 8, 2661. doi: 10.1038/s41598-018-20593-w
Huveneers C., Apps K., Becerril-Garcia E. E., Bruce B., Butcher P. A., Carlisle A. B., et al. (2018). Future research directions on the “elusive“ white shark. Front. Mar. Sci. 5. doi: 10.3389/fmars.2018.00455
Irion D. T., Noble L. R., Kock A. A., Gennari E., Dicken M. L., Hewitt A. M., et al. (2017). Pessimistic assessment of white shark population status in south Africa: comment on andreottiet al 2016. Mar. Ecol. Prog. Ser. 577, 251–255. doi: 10.3354/meps12283
Johnson R., Kock A. (2006). “South Africa’s white shark cage-diving industry-is there cause for concern,” in Finding a balance: White shark conservation and recreational safety in the inshore waters of Cape Town, South Africa. Eds. Nel D. C., Peschak T. P.. Stellenbosch, SA: WWF South Africa Report Series. 2006/Marine/001.
Jorgensen S. J., Micheli F., White T. D., Van Houtan K. S., Alfaro-Shigueto J., Andrzejaczek S., et al. (2022). Emergent research and priorities for shark and ray conservation. Endanger. Species Res. 47, 171–203. doi: 10.3354/esr01169
Kock A. A., Lombard A. T., Daly R., Goodall V., Meyer M., Johnson R., et al. (2022). Sex and size influence the spatiotemporal distribution of white sharks, with implications for interactions with fisheries and spatial management in the southwest Indian ocean. Front. Mar. Sci. 9. doi: 10.3389/fmars.2022.811985
Kuhnert P. M., Griffiths S., Brewer D. (2011). Assessing population changes in bycatch species using fishery-dependent catch rate data. Fish. Res. 108, 15–21. doi: 10.1016/j.fishres.2010.11.014
Lawler J. J., Campbell S. P., Guerry A. D., Kolozsvary M. B., O'Connor R. J., Seward L. C. (2002). The scope and treatment of threats in endangered species recovery plans. Ecol. Appl. 12, 663–667. doi: 10.1890/1051-0761(2002)012[0663:TSATOT]2.0.CO;2
Maunder M. N., Sibert J. R., Fonteneau A., Hampton J., Kleiber P., Harley S. J. (2006). Interpreting catch per unit effort data to assess the status of individual stocks and communities. ICES J. Mar. Sci. 63, 1373–1385. doi: 10.1016/j.icesjms.2006.05.008
McAllister M. K., Pikitch E. K., Babcock E. A. (2001). Using demographic methods to construct Bayesian priors for the intrinsic rate of increase in the Schaefer model and implications for stock rebuilding. Can. J. Fish. Aquat. Sci. 58, 1871–1890. doi: 10.1139/f01-114
Merely L., Lange L., Meÿer M., Hewitt A. M., Koen P., Fischer C., et al. (2019). Blood plasma levels of heavy metals and trace elements in white sharks (Carcharodon carcharias) and potential health consequences. Mar. pollut. Bull. 142, 85–92. doi: 10.1016/j.marpolbul.2019.03.018
Micarelli P., Bonsignori D., Compagno L. J. V., Pacifico A., Romano C., Reinero F. R. (2021). Analysis of sightings of white sharks in gansbaai (South Africa). Eur. Zool. J. 88 (1), 363–374. doi: 10.1080/24750263.2021.1892216
Mollet H. F., Cailliet G. M. (2002). Comparative population demography of elasmobranchs using life history tables, Leslie matrices and stage-based matrix models. Mar. Freshw. Res. 53, 503–516. doi: 10.1071/MF01083
Natanson L. J., Skomal G. B. (2015). Age and growth of the white shark, Carcharodon carcharias, in the western north Atlantic ocean. Mar. Freshw. Res. 66, 387–398. doi: 10.1071/MF14127
Oliver S., Braccini M., Newman S. J., Harvey E. S. (2015). Global patterns in the bycatch of sharks and rays. Mar. Policy. 54, 86–97. doi: 10.1016/j.marpol.2014.12.017
Pfaff M. C., Logston R. C., Raemaekers S. J. P. N., Hermes J. C., Blamey L. K., Cawthra H. C., et al. (2019). A synthesis of three decades of socio-ecological change in false bay south Africa: setting the scene for multidisciplinary research and management. Elem. Sci. Anth. 7, 32. doi: 10.1525/elementa.367
Punt A. E., Butterworth D. S., de Moor C. L., De Oliveira J. A., Haddon M. (2016). Management strategy evaluation: best practices. Fish Fisheries 17 (2), 303–334. doi: 10.1111/faf.12104
Queiroz N., Humphries N. E., Couto A., Vedor M., Da Costa I., Sequeira A. M., et al. (2019). Global spatial risk assessment of sharks under the footprint of fisheries. Nature. 572 (7770), 461–466. doi: 10.1038/s41586-019-1444-4
Rigby C. L., Barreto R., Carlson J., Fernando D., Fordham S., Francis M. P., et al. (2019)Carcharodon carcharias. In: The IUCN red list of threatened species. Available at: https://www.iucnredlist.org/species/3855/2878674 (Accessed May 04, 2022).
Roux J. P., Shannon L. J. (2004). Ecosystem approach to fisheries management in the northern benguela: the Namibian experience. Afr. J. Mar. Sci. 26, 79–93. doi: 10.2989/18142320409504051
Ruzzante D. E., McCracken G. R., Førland B., MacMillan J., Notte D., Buhariwalla C., et al. (2019). Validation of close-kin mark–recapture (CKMR) methods for estimating population abundance. Methods Ecol. Evol. 10 (9), 1445–1453. doi: 10.1111/2041-210X.13243
Ryklief R. (2012). Population dynamics of the white shark, carcharodon carcharias, at mossel bay south Africa (Port Elizabeth, SA: Nelson Mandela Metropolitan University).
Towner A. V., Leos-Barajas V., Langrock R., Schick R. S., Smale M., Kaschke T., et al. (2016). Sex-specific and individual preferences for hunting strategies in white sharks. Funct. Ecol. 30 (8), 1397–1407. doi: 10.1111/1365-2435.12613
Towner A. V., Watson R. G. A., Kock A. A., Papastamatiou Y., Sturup M., Gennari E., et al. (2022). Fear at the top: Killer whale predation drives white shark absence at south africa's largest aggregation site. Afr. J. Mar. Sci. 44 (1), 1–14. doi: 10.2989/1814232X.2022.2066723
Towner A. V., Wcisel M. A., Reisinger R. R., Edwards D., Jewell O. J. (2013). Gauging the threat: the first population estimate for white sharks in south Africa using photo identification and automated software. PloS One 8, e66035. doi: 10.1371/journal.pone.0066035
Traill L. W., Bradshaw C. J. A., Brook B. W. (2007). Minimum viable population size: a meta-analysis of 30 years of published estimates. Biol. Conserv. 139, 159–166. doi: 10.1016/j.biocon.2007.06.011
Keywords: life history, threats, shark conservation, recovery ability, white shark (Carcharodon carcharias)
Citation: Bowlby HD, Hammerschlag N, Irion DT and Gennari E (2022) How continuing mortality affects recovery potential for prohibited sharks: The case of white sharks in South Africa. Front. Conserv. Sci. 3:988693. doi: 10.3389/fcosc.2022.988693
Received: 07 July 2022; Accepted: 24 August 2022;
Published: 15 September 2022.
Edited by:
Oded Berger-Tal, Ben-Gurion University of the Negev, IsraelReviewed by:
Shawn Larson, Seattle Aquarium, United StatesColin Simpfendorfer, James Cook University, Australia
Copyright © 2022 Bowlby, Hammerschlag, Irion and Gennari. This is an open-access article distributed under the terms of the Creative Commons Attribution License (CC BY). The use, distribution or reproduction in other forums is permitted, provided the original author(s) and the copyright owner(s) are credited and that the original publication in this journal is cited, in accordance with accepted academic practice. No use, distribution or reproduction is permitted which does not comply with these terms.
*Correspondence: Heather D. Bowlby, aGVhdGhlci5ib3dsYnlAZGZvLW1wby5nYy5jYQ==