Application of multiple testing procedures for identifying relevant comorbidities, from a large set, in traumatic brain injury for research applications utilizing big health-administrative data
- 1Department of Mathematics, Indian Institute of Technology, Hyderabad, India
- 2Toronto Western Hospital, Toronto, ON, Canada
- 3Dalla Lana School of Public Health, University of Toronto, Toronto, ON, Canada
- 4KITE Research Institute Toronto Rehabilitation Institute, University Health Network, Toronto, ON, Canada
- 5Rehabilitation Sciences Institute, Temerty Faculty of Medicine, University of Toronto, Toronto, ON, Canada
- 6Global Brain Health Institute, Institute of Neuroscience, Trinity College Dublin, Dublin, Ireland
- 7Acquired Brain Injury Research Lab, University of Toronto, Toronto, ON, Canada
- 8Institute of Health Policy, Management and Evaluation, University of Toronto, Toronto, ON, Canada
- 9Faculty of Health Sciences, Ontario Tech University, Oshawa, ON, Canada
- 10ICES (fomerly Institute for Clinical Evaluative Sciences), Toronto, ON, Canada
Background: Multiple testing procedures (MTP) are gaining increasing popularity in various fields of biostatistics, especially in statistical genetics. However, in injury surveillance research utilizing the growing amount and complexity of health-administrative data encoded in the International Statistical Classification of Diseases and Related Health Problems 10th Revision (ICD-10), few studies involve MTP and discuss their applications and challenges.
Objective: We aimed to apply MTP in the population-wide context of comorbidity preceding traumatic brain injury (TBI), one of the most disabling injuries, to find a subset of comorbidity that can be targeted in primary injury prevention.
Methods: In total, 2,600 ICD-10 codes were used to assess the associations between TBI and comorbidity, with 235,003 TBI patients, on a matched data set of patients without TBI. McNemar tests were conducted on each 2,600 ICD-10 code, and appropriate multiple testing adjustments were applied using the Benjamini-Yekutieli procedure. To study the magnitude and direction of associations, odds ratios with 95% confidence intervals were constructed.
Results: Benjamini-Yekutieli procedure captured 684 ICD-10 codes, out of 2,600, as codes positively associated with a TBI event, reducing the effective number of codes for subsequent analysis and comprehension.
Conclusion: Our results illustrate the utility of MTP for data mining and dimension reduction in TBI research utilizing big health-administrative data to support injury surveillance research and generate ideas for injury prevention.
Introduction
Biological functions are interrelated since they co-occur in the human body. This gives rise to complex relationships between different body functions and the effects of multiple diseases on the human body; therefore, it is essential to consider multiple coexisting medical disorders referred to as comorbidities (Feinstein, 1970) in this article and their implications in injury surveillance. Of all injuries known to date, traumatic brain injury (TBI) is among the most disabling injuries affecting many individuals in the prime of their life (Feigin et al., 2021). Concern about TBI related to the expansion of industrialization and armed conflict has led to increased interest in the epidemiology of TBI in civilians and among service members. Published estimates of TBI vary worldwide; although when estimates from studies with comprehensive data collection methods are extrapolated internationally, reports suggest that 50–60 million people are affected annually, and the pooled international incidence rate of TBI (excluding TBI with no overt pathologic features) is reported to be a staggering 349 (95% confidence interval (CI) 96–1,266) per 100,000 person-years. To develop prevention initiatives and guide injury surveillance research, it is necessary to consider the multiple comorbidities occurring in the time preceding injury in the population of interest.
Population-based health-administrative data housing information across multiple diagnostic conditions from millions of patients have become a popular data source for evaluating relationships between comorbidities with a specific condition of interest. Due to rapid advancement in technology and the evolution of computation and storage facilities over the last few decades, recording and accessing information across millions of study units in large electronic databases have become feasible, giving rise to “high-dimensional” or “big” data. There is a need to develop appropriate data mining and dimension reduction methods for managing big data efficiently. This change in thinking mirrors the change in the analyses of genetic data.
Initially, researchers encountered only a small group of genes in laboratory studies. However, it is now common for genetic studies to simultaneously analyse millions or trillions of genes (Thomas et al., 2005) in multiple testing (MT) procedure (MTP) that involves the simultaneous testing of more than one hypothesis. One strategy used by genetic researchers has been to look at the false discovery rate (FDR) (Tsai et al., 2003), which looks at a set of variables that have a high probability of having a “signal”. Instead of seeing if one gene is, say, statistically significant, the goal is to find a set of genes where there is a high probability that most of the genes are significant. This promising approach has yet to be widely used in analyzing healthcare data from large administrative databases of injury data of patients with TBI.
To control for family-wise error rate (FWER) in MTPs, the most common method was Bonferroni correction (Dunn, 1961). This is an adjustment so that the possibility of falsely rejecting the null hypothesis for each of the multiple tests is held at α. For example, for the usual α of 0.05, the chance of falsely rejecting the null hypothesis for all tests combined is 0.05, not for each individual test. For Bonferroni, one would require the probability of falsely rejecting each individual test to be fixed at α/m, where m is the total number of tests. However, in most genetic experiments and biomedical and epidemiological studies, scientists are generally interested in detecting true signals rather than just guarding against a large number of false positives by controlling FWER. In their seminal paper in 1995, Benjamini and Hochberg suggested an alternative approach for dealing with multiple tests, which has increased power, and is less conservative (Reiner et al., 2003; Tsai et al., 2003; Benjamini et al., 2006; Narum, 2006; Sun et al., 2006; Jones et al., 2008; Verhoeven et al., 2017). The authors (Benajmini and Hochberg, 1995) suggested controlling FDR, which is the expected proportion of false discoveries, a discovery being a rejected hypothesis or in other words a “signal”.
In this study, we provided an explanation and an illustration of how to utilize these FDR control methods to support injury surveillance research in TBI. Determining the significant association of multiple comorbidities with the TBI event requires us to test multiple hypotheses, which increases the chances of inferring false-positive results, and this rate accelerates with the number of hypotheses. This brings us to the domain of MT theory, which provides a mechanism to protect against false positive conclusions by controlling for error rate (Bender and Lange, 2001; Reiner et al., 2003). Although MTPs are gaining popularity in genetics literature (Tsai et al., 2003; Sun et al., 2006) and clinical trials (Marshall et al., 2004; Mehrotra and Heyse, 2004; Burkom et al., 2005; Mehrotra and Adewale, 2012), it is, however, underutilized in biomedical and epidemiologic research until recently by a few (Jones et al., 2008; Anderson et al., 2016; Sollmann et al., 2018, 2019), even though it is a common problem in this field (Bender and Lange, 2001). Before further discussion, it is essential to point out to readers that we will not be looking at complex interactions between comorbidities; we will simply be looking at distinct associations of comorbidities with the condition of interest, TBI.
The objective of this study is to demonstrate the use of contemporary methodologies of MTPs for accurate statistical analysis in big health-administrative data to capture distinct comorbidities associated with TBI. This article outlines the procedures, using a case study, so that researchers could potentially use while working with multiple comorbidities in health-administrative data of other complex injuries and conditions. In other words, this article demonstrates the use of modern data mining methods for handling big data from healthcare settings. The intent of this article is pedagogical, and we used knowledge translations and draw inspirations from other fields of big data such as statistical genetics (Tsai et al., 2003; Sun et al., 2006). Table 1 presents the applications of different MTPs across various domains.
Methods
The methods mentioned below are guided by the scientific question to perform an MTP on a matched sample of TBI and non-TBI patients, enriched with complementary information of previous TBI research. We first discussed the development of required steps that can be easily translated for any similar big administrative healthcare data and then discussed the results in the light of previous TBI research. We would like to emphasize again that our objective in this article is simply pedagogical. Similar approaches already exist in statistical genetics literature, and we intended to translate that knowledge to the healthcare field. Details of the underlying MTPs have been provided in Appendix.
Identification of data analytics methods and steps
In large health-administrative data sets, one way of classifying health conditions and their circumstances is to use the International Statistical Classification of Diseases and Related Health Problems, Tenth Revision (ICD-10) codes (Walker et al., 2012). Before using MTPs, we found that the complete set of ICD-10 codes was too granular, so we first grouped the codes by the first three characters – the first character being a letter and the next two being digits. Individual codes are nested into these groups, reducing the problem to only looking at a possible 2,600 codes.
Therefore, with techniques to fully exploit the potential wealth of information in health-administrative data and data reduction tools, we considered 2,600 ICD-10 codes for an aggregative association study of different comorbidities on a particular condition of interest, thus leading to 2,600 multiple tests. We elaborated on the application of MT theory for health-administrative data in the context of associations between 2,600 ICD-10 codes and TBI. Below are the basic steps of the analysis (Figure 1). The objective is to identify a subset of variables with a high probability of being related to the outcome of interest.
Step 1: Define the set of variables one wishes to test. In the example below, the purpose is to look at which ICD-10 codes appear to be related to a future TBI event.
Step 2: Decide the basic analytical model for each of the 2,600 variables being tested. The investigator can use almost any model in this study and obtain a p-value as a measure of the significance of the variable in the model. In this study, a matched case-control study was used; hence, McNemar test statistics were calculated.
Step 3: Repeat the test for each variable using the basic analytical model from step 2. In each analysis, the p-value is obtained. MTP is then used to identify a small set of p-values, controlling for FDR. In the example that follows, Benjamini and Yekutieli (2001) approach is used to account for possible dependence among the 2,600 tests to determine significant comorbidities.
Proof-of-concept and internal validation
We used health-administrative data on emergency departments extracted from National Ambulatory Care Reporting System (NACRS) database and acute care data extracted from Discharge Abstract Database (DAD). Both data sets were obtained from ICES, which collects and stores health administrative data on publicly funded services provided to residents of Ontario, Canada. Information on the study subjects' income quintile was extracted from the Registered Persons Database.
We constructed a histogram for the days from the index date of hospital visits for all the TBI patients included in the study. We observed a peak around the index date, with the frequency dropping to a stationary point at 30 days before, and after, the index date. Therefore, we used this 60-day window as a TBI-related window, where we considered, all ED and acute care visits within 5 years, between the fiscal years 2007/08 and 2015/16, up to 30 days prior to a TBI event as the pre-injury phase. For further details on these and the histogram, we refer the readers to the follow-up study (Mollayeva et al., 2019).
We split the data into three groups, namely, training sample, validation sample, and test sample when doing this analysis. The advice on percentages of the data to put into each group varies. In this study, the master data were split into 50–25–25% of the data for train/validate/test data sets (Friedman et al., 2008). The analysis was first done on the training sample to obtain relevant codes, then retested using the validation data set. Relevant codes were reconfirmed using the validation data set. The testing data set was used for reporting the final output. This approach provided independent data sets to replicate the findings and to further guard against overfitting.
A 1:1 match was performed among the two groups being compared in this study, namely, TBI patients and non-TBI patients, or in other words, patients who were also discharged from ED or acute care during the same time period for a reason other than TBI. They were matched based on four demographic variables, namely, age, sex, income, and rural or urban neighborhood, using exact matching for sex, income quintile, neighborhood, and caliper matching for age with a caliper of 2 years. For additional details, the readers are referred to Mollayeva et al. (2019).
Results
In total, 2,600 McNemar tests were conducted on the training data set. The conservative Bonferroni procedure captured 630 out of 2,600 as significant codes while controlling FWER at 5%, whereas the BY procedure captured 775 significant ICD-10 codes when controlling FDR. Note that, all 630 codes captured by the Bonferroni procedure were also captured by BY procedure. The p-values for the BY procedure, with FDR controlled at 5%, were plotted (Figure 2). We can get a visual impression of the proportion of significant ICD-10 codes. The solid black line represents the cut-off value, indicating that all p-values below the cut-off value are significant. Odds ratios (ORs) and their respective 95% confidence intervals (CIs) were calculated for the 775 codes, of which 684 had OR>1.
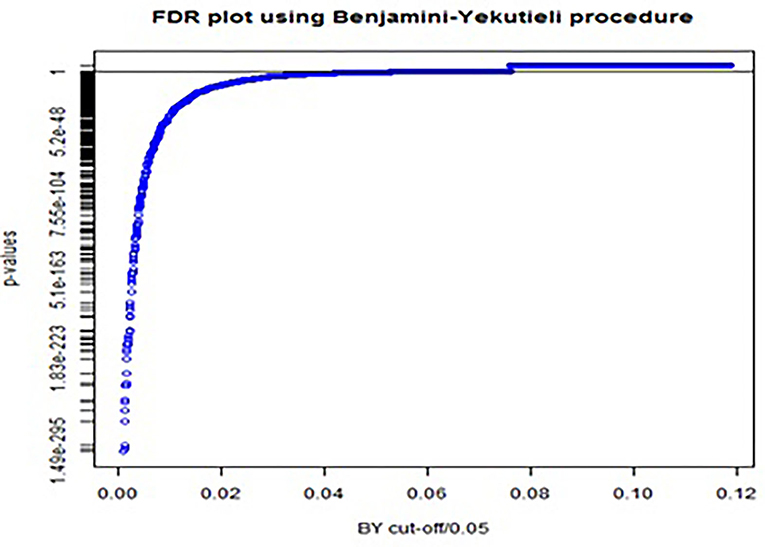
Figure 2. FDR plot using Benjamini-Yekutieli procedure for adjusting p-values in multiple testing with FDR controlled at 5%.
The 684 codes identified from the training data set were retested using the validation data set, and the BY procedure captured 584 of them as significant. Finally, ORs for these 584 codes were calculated using the testing data set. Top six significant ICD-10 codes with the highest ORs are listed in Table 2. These codes were found to be associated with TBI in the existing epidemiologic literature. The remaining ICD-10 codes relevant to TBI are reported in a follow-up work (Mollayeva et al., 2019).
As this is a pedagogical article, we have provided a sample data set (Supplementary material) and a sample R code (Supplementary material), which readers may use to become more acquainted with the steps employed in this study. The data set and R code that have been provided, however, are solely for illustration purposes. The sample dataset contains 1,239 ICD-10 codes, corresponding McNemar test statistic values, unadjusted p-values, ORs, and 95% CIs of the ORs. Once the R code is run using this data set, one will obtain 781 significant ICD-10 codes out of 1,239 codes provided in the data set and 686 relevant codes out of 781. This sample code illustrates the use of the BY method, which is the prime focus of this study. The dataset can be regarded as a training data set, and the same steps can be repeated on these 686 codes using the validation data set. Finally, the testing data set can be used for the final reporting of ORs and 95% CIs. Please note that this is only a sample data and not the complete data set used to obtain the results in this or the follow-up works (Mollayeva et al., 2019, 2022).
Statistical analyses in this article were done using the statistical software R 3.3.0 (R Foundation for Statistical Computing; www.r-project.org) and SAS 9.3 (SAS software: version 9.3, SAS Inc., Cary, NC).
Discussion
We implemented two MTPs to assess associations between 2,600 ICD-10 codes and TBI in this research. A total of 684 relevant codes captured in this study have been reported and used for further analysis in the follow-up studies (Mollayeva et al., 2019, 2022). We have successfully shown that the developed proof-of-concept worked with data and that supportive evidence on the association of TBI with the top six codes was found in contemporary literature. Although the proof-of-concept implementation only shows one case example, TBI, the methods can be reused for different population data.
The BY procedure implemented in this study initially captured 775 ICD-10 codes as significant codes. However, we would like to highlight that, of these 775 codes, we considered only codes with OR>1 as codes relevant to TBI, which led to only 684 relevant codes. This is because our reference group, unlike any control group, does not consist of healthy individuals. Therefore, comorbid conditions, such as female infertility, were observed to be highly correlated with TBI with a p-value of 3.3 × 10−16 and an OR of 0.072, appearing to be protective of a TBI event. This interpretation is, however, misleading. Such a strong negative correlation between female infertility and TBI is because there were many patients in our data set diagnosed with female infertility who did not have TBI, and this has no link with the association between female infertility and TBI. TBI severity or concussions were unspecified in the data set. The observed association might be owing to care-seeking behaviors in concussive injury, few females sought care after mild TBI/concussion as compared to females without TBI.
This phenomenon of strong negative correlation was observed for a few other ICD-10 codes as well. This is also termed as collider effect or sampling bias and has been illustrated in detail in Carvajal-Rodríguez (2018). Hence, we removed significant codes with OR < 1. As is evident, the top 2 codes with the highest ORs captured in this study are alcohol involvement and neurological disorders. These comorbidities were observed to be present in TBI and acquired brain injury (ABI) patients in other studies as well (Colantonio et al., 2011; Thompson et al., 2012). Interesting findings about contrasting profiles of TBI vs. non-TBI patients have been presented in this study (Colantonio et al., 2011).
Some limitations are related to the use of health-administrative data in our research. Typically, acute care data provided by ICES are reported to be accurate and undergo quality assurance on a regular basis1 (Cole et al., 2010). While we used validated algorithms to define TBI, each comorbidity captured within the emergency department and acute care visits is also characterized by certain sensitivity and specificity, resulting in possible misclassification, although the misclassification, if present, would refer to both TBI cohort and the reference cohort. Methods for adjusting for misclassification exist; however, it was not within the scope of this study to perform the adjustments. Further studies are required to address this limitation.
What this study adds
The purpose of using MTPs in a study such as this is dimension reduction. Big health-administrative data sets contain information on multiple correlated comorbidities. To study associations of these comorbidities with the condition of interest, thousands of tests need to be performed for each comorbidity simultaneously, which would lead to a multiplicity problem, leading to a huge number of false rejections. However, a screening approach using MT adjustments to capture relevant codes for correlated tests considerably reduces the dimension of the data set to a smaller space, consequently reducing the multiplicity. This is an essential step before any subsequent analysis is done, as it improves the predictive power of the analysis.
Conclusion and future directions
In this study, using knowledge translations from other fields of high-dimensional data, such as statistical genetics, we developed a statistical approach and applied it for analyzing decade-long health-administrative data of patients with TBI and individually matched on sex, age, socio-economic status and neighborhood, with non-TBI patients. We illustrated the utility of classical and modern statistical tools for assessing comorbidity in big health-administrative data sets, which can be applied to any extensive health-administrative data set to study associations between comorbidities represented by ICD-10 codes and any condition of interest.
Future directions
Assuming a general dependence structure among comorbidities, we used the Benjamini-Yekutieli method to adjust multiplicity in correlated tests. Other more powerful methods for FDR control based on resampling can be used as well; however, implementing such methods on high-dimensional data sets will require huge computational time, system memory allocation and state-of-the-art computational facilities.
We would like to highlight that, in this study, we have done the analysis only on 2,600 ICD-10 codes, due to time and system memory constraints; however, the analysis can be easily extended to more specific 26,000 ICD-10 codes. One can be as granular as one wishes for ICD-10 codes, subject to system and time constraints.
Future work can also consider assimilating data from different populations/strata and implementing more modern FDR controlling procedures such as stratified FDR to give us an overall picture. Please note that the regular FDR is a weighted average of the stratified FDR, and hence, the latter is computationally intensive (Sun et al., 2006).
Considerable work is required for the automation of the process outlined in this article using autonomic computing, which is the future of the next-generation computing (Gill et al., 2022). The benefit of having such AI-based automated computing system is the low cost incurred in implementing and maintaining them. Anomaly detection, record-keeping, data organization, and cleaning can be made up to date with real-time inputs and computation (Gill et al., 2022). Such methods can be easily implemented across several domains of applications including mining of health-administrative data and would be an interesting future research that can benefit the field of public health.
Data availability statement
The data analyzed in this study is subject to the following licenses/restrictions: The datasets generated during and/or analyzed during the current study are available in the ICES repository, under accession DAS 2016-257(2018 0970 084 000). Data sharing agreements prohibit ICES from making the datasets publicly available, however access may be granted to those who meet pre-specified criteria for confidential access. This study made use of de-identified data from the ICES Data Repository, which is managed by the Institute for Clinical Evaluative Sciences with support from its funders and partners: Canada's Strategy for Patient-Oriented Research (SPOR), the Ontario SPOR Support Unit, the Canadian Institutes of Health Research and the Government of Ontario. The opinions, results and conclusions reported are those of the authors. No endorsement by ICES or any of its funders or partners is intended or should be inferred. Parts of this material are based on data and information compiled and provided by the Canadian Institute for Health Information (CIHI). However, the analyses, conclusions, opinions and statements expressed herein are those of the author, and not necessarily those of CIHI. The full dataset creation plan and underlying analytic code are available from the authors upon request, understanding that the computer programs may rely upon coding templates or macros that are unique to ICES and are therefore either inaccessible or may require modification. A sample dataset (Supplementary material) with the ICD-10 codes and p-values from the McNemar test statistics on which the MTPs were used are attached as Supplementary material to this manuscript. A sample R code (Supplementary material) is also provided for the readers to run the code and obtain outputs from the data. Requests to access these datasets should be directed to www.ices.on.ca/DAS, http://www.ices.on.ca/DAS.
Ethics statement
The studies involving human participants were reviewed and approved by the Ethics Committees at the Clinical (Toronto Rehabilitation Institute-University Health Network) and Academic (University of Toronto) Institutions. Written informed consent from the patient/participants or patients/participants' legal guardian/next of kin was not required to participate in this study in accordance with the national legislation and the institutional requirements.
Author contributions
SJ carried out the analysis with support from ME, MS, and VC and drafted the manuscript. MS cleaned and prepared the data set for analysis, and developed and implemented codes for some supplementary analysis with support from ME. AC, VC, and TM conceived the original concept and initiated the work. ME designed and optimized statistical analyses for this work. He provided major feedback on the manuscript, and supervised and mentored during the analysis and manuscript development stage. SJ is the first author and ME is the senior author. All authors provided their feedback on the manuscript and the analysis.
Funding
This study was supported by the Eunice Kennedy Shriver National Institute of Child Health & Human Development of the National Institutes of Health (NIH) [Award No. R21HD089106] and the National Institute of Neurological Disorders and Stroke of the National Institutes of Health under Award Number R01NS117921. AC was funded by the Canadian Institutes of Health Research (CIHR) Chair in Gender, Work and Health [Grant No. CGW-126580], and TM was supported by Canada Research Chair in Neurological Disorders and Brain Health and the Alzheimer's Association Grant [AARF-16-442937]. Please note that the content of the article is solely the responsibility of the authors and does not necessarily represent the official views of the NIH. This work was funded in part by the Canada Research Chairs Programme. The funders had no role in the study design, data collection, decision to publish or preparation of the manuscript.
Acknowledgments
We acknowledge ICES and its staff for providing us with the data, technical support for its analysis and the matching algorithm. This study received approval from the Research Ethics Board at the Toronto Rehabilitation Institute-University Health Network (TRI-UHN).
Conflict of interest
The authors declare that the research was conducted in the absence of any commercial or financial relationships that could be construed as a potential conflict of interest.
Publisher's note
All claims expressed in this article are solely those of the authors and do not necessarily represent those of their affiliated organizations, or those of the publisher, the editors and the reviewers. Any product that may be evaluated in this article, or claim that may be made by its manufacturer, is not guaranteed or endorsed by the publisher.
Supplementary material
The Supplementary Material for this article can be found online at: https://www.frontiersin.org/articles/10.3389/fdata.2022.793606/full#supplementary-material
Abbreviations
MTP, multiple testing procedure; MT, multiple testing; FDR, false discovery rate; FWER, family-wise error rate; ICD-10, International Statistical Classification of Diseases and Related Health Problems (10th revision); TBI, traumatic brain injury; ICES, Institute for Clinical Evaluative Sciences.
Footnotes
1. ^Available online at: https://www.cihi.ca/en/topics/emergency-and-ambulatory-care#_Databases_and_Data.
References
Alberton, B. A. V., Thomas, E. N., Humberto, R. G., and Anderson, M. W. (2020). Multiple testing correction over contrasts for brain imaging. NeuroImage. 216, 116760. doi: 10.1016/j.neuroimage.2020.116760
Anderson, A. E., Kerr, W. T., Thames, A., Li, T., Xiao, J., Cohen, M. S., et al. (2016). Electronic health record phenotyping improves detection and screening of type 2 diabetes in the general United States population: A cross-sectional, unselected, retrospective study. J. Biomed. Inform. 60, 162–168. doi: 10.1016/j.jbi.2015.12.006
Bartenschlager, C. C., and Brunner, J. O. (2021). new user specific multiple testing method for business applications: The SiMaFlex procedure. J. Stat. Plann. Infer. 214, 25–40. doi: 10.1016/j.jspi.2021.01.004
Benajmini, Y., and Hochberg, Y. (1995). Controlling the false discovery rate: a practical and powerful approach to multiple testing. J. R Stat. Soc. Ser. B. 57, 289–300. doi: 10.1111/j.2517-6161.1995.tb02031.x
Bender, R., and Lange, S. (2001). Adjusting for multiple testing - When and how? J. Clin. Epidemiol. 54, 343–349. doi: 10.1016/S0895-4356(00)00314-0
Benjamini, Y., Krieger, A. M., Yekutieli, D., and Krieger, A. M. (2006). Adaptive linear step-up procedures that control the false discovery rate. Biometrika. 93, 491–507. doi: 10.1093/biomet/93.3.491
Benjamini, Y., and Yekutieli, D. (2001). The control of the false discovery rate in multiple testing under dependency. Ann. Stat. 29, 1165–1188. doi: 10.1214/aos/1013699998
Boake, C., McCauley, S. R., Levin, H. S., Pedroza, C., Contant, C. F., Song, J. X., et al. (2005). Diagnostic criteria for postconcussional syndrome after mild to moderate traumatic brain injury. J. Neuropsych. Clin. Neurosci. 17, 350–356. doi: 10.1176/jnp.17.3.350
Burkom, H. S., Murphy, S., Coberly, J., and Hurt-Mullen, K. (2005). Analytic methods public health monitoring tools for multiple data streams. Morb. Mortal Wkly. Rep. Surveill. Summ. 54, 55–62.
Carvajal-Rodríguez, A. (2018). Myriads: P-value-based multiple testing correction. Bioinformatics. 34, 1043–1045. doi: 10.1093/bioinformatics/btx746
Cheng, P., Yin, P., Ning, P., Wang, L., Cheng, X., Liu, Y., et al. (2017). Trends in traumatic brain injury mortality in China, 2006–2013: A population-based longitudinal study. PLoS Med. 14, 2006–2013. doi: 10.1371/journal.pmed.1002332
Colantonio, A., Gerber, G., Bayley, M., Deber, R., Yin, J., Kim, H., et al. (2011). Differential profiles for patients with traumatic and non- traumatic brain injury. J. Rehab. Med. 43, 311–315. doi: 10.2340/16501977-0783
Colantonio, A., Saverino, C., Zagorski, B., Swaine, B., Lewko, J., Jaglal, S., et al. (2010). Hospitalizations and emergency department visits for TBI in Ontario. Can. J. Neurol. Sci. 37, 783–790. doi: 10.1017/S0317167100051441
Cole, S. R., Platt, R. W., Schisterman, E. F., Chu, H., Westreich, D., Richardson, D., et al. (2010). Illustrating bias due to conditioning on a collider. Int. J. Epidemiol. 39, 417–420. doi: 10.1093/ije/dyp334
Courtney, A. C., and Courtney, M. W. A. (2009). thoracic mechanism of mild traumatic brain injury due to blast pressure waves. Med. Hypotheses. 72, 76–83. doi: 10.1016/j.mehy.2008.08.015
Courtney, M. W., and Courtney, A. C. (2011). Working toward exposure thresholds for blast-induced traumatic brain injury: Thoracic and acceleration mechanisms. Neuroimage. 54, S55–61. doi: 10.1016/j.neuroimage.2010.05.025
Cunningham, R. M., Maio, R. F., Hill, E. M., and Zink, B. J. (2002). The effects of alcohol on head injury in the motor vehicle crash victim. Alcohol. Alcohol. 37, 236–240. doi: 10.1093/alcalc/37.3.236
Dunn, O. J. (1961). Multiple comparisons among means. J. Am. Stat. Assoc. 56, 52–64. doi: 10.1080/01621459.1961.10482090
Feigin, V. L., Vos, T., Alahdab, F., Amit, A. M. L., Bärnighausen, T. W., Beghi, E., et al. (2021). Burden of neurological disorders across the US from 1990-2017: a global burden of disease study. JAMA Neurol. 78, 165–176. doi: 10.1001/jamaneurol.2020.4152
Feinstein, A. R. (1970). The pre-therapeutic classification of comorbidity in chronic disease. J. Chronic. Dis. 23, 455–468. doi: 10.1016/0021-9681(70)90054-8
Fernandes, R. N. R., and Silva, M. (2013). Epidemiology of Traumatic Brain Injury in Brazil. Arq. Bras. Neurocir. Brazilian Neurosurg. 32, 136–142. doi: 10.1055/s-0038-1626005
Friedman, J., Hastie, T., and Tibshirani, R. (2008). The Elements of Statistical Learning. New York: Springer Series in Statistics. Vol. 1. p. 1–745.
Gill, S. S., Xu, M., Ottaviani, C., Patros, P., Bahsoon, R., Shaghaghi, A., et al. (2022). AI for next generation computing: Emerging trends and future directions. Internet Things. 19, 100514. doi: 10.1016/j.iot.2022.100514
Halbauer, J. D., Ashford, J. W., Zeitzer, J. M., Adamson, M. M., Lew, H. L., Yesavage, J. A., et al. (2009). Neuropsychiatric diagnosis and management of chronic sequelae of war-related mild to moderate traumatic brain injury. J. Rehabil. Res. Dev. 46, 757–796. doi: 10.1682/JRRD.2008.08.0119
Hamill, V., Barry, S. J. E., McConnachie, A., McMillan, T. M., and Teasdale, G. M. (2015). Mortality from head injury over four decades in Scotland. J. Neurotrauma. 32, 689–703. doi: 10.1089/neu.2014.3670
Hyder, A. A., Wunderlich, C. A., Puvanachandra, P., Gururaj, G., and Kobusingye, O. C. (2007). The impact of traumatic brain injuries: A global perspective. NeuroRehabilitation. 22, 341–353. doi: 10.3233/NRE-2007-22502
Jamieson, L. M., Harrison, J. E., and Berry, J. G. (2008). Hospitalisation for head injury due to assault among indigenous and non-indigenous Australians, July 1999 - June 2005. Med. J. Aust. 188, 576–579. doi: 10.5694/j.1326-5377.2008.tb01793.x
Jones, H. E., Ohlssen, D. I., and Spiegelhalter, D. J. (2008). Use of the false discovery rate when comparing multiple health care providers. J. Clin. Epidemiol. 61, 232–240. doi: 10.1016/j.jclinepi.2007.04.017
Kashluba, S., Casey, J. E., and Paniak, C. (2006). Evaluating the utility of ICD-10 diagnostic criteria for postconcussion syndrome following mild traumatic brain injury. J. Int. Neuropsychol. Soc. 12, 111–118. doi: 10.1017/S1355617706060036
Kleiven, S., Peloso, P. M., and Holst, H. (2003). The epidemiology of head injuries in Sweden from 1987 to 2000. Inj. Control Saf. Promot. 10, 173–180. doi: 10.1076/icsp.10.3.173.14552
Kraus, J. F., Morgenstern, H., Fife, D., Conroy, C., and Nourjah, P. (1989). Blood alcohol tests, prevalence of involvement, and outcomes following brain injury. Am. J. Public Health. 79, 294–299. doi: 10.2105/AJPH.79.3.294
Langlois, J. A., Kegler, S. R., Butler, J. A., Gotsch, K. E., Johnson, R. L., Reichard, A. A., et al. (2003). Traumatic brain injury-related hospital discharges results from a 14-state surveillance system. Morb. Mortal. Wkly. Rep. Surveill. Summ. 52, 1–20.
Marshall, C., Best, N., Bottle, A., and Aylin, P. (2004). Statistical issues in the prospective monitoring of health outcomes across multiple units. J. R Stat. Soc. Ser. A. 167, 541–559. doi: 10.1111/j.1467-985X.2004.apm10.x
Mehrotra, D. V., and Adewale, A. J. (2012). Flagging clinical adverse experiences: Reducing false discoveries without materially compromising power for detecting true signals. Stat. Med. 31, 1918–1930. doi: 10.1002/sim.5310
Mehrotra, D. V., and Heyse, J. F. (2004). Use of the false discovery rate for evaluating clinical safety data. Stat. Methods Med. Res. 13, 227–238. doi: 10.1191/0962280204sm363ra
Mollayeva, T., Sutton, M., Chan, V., Colantonio, A., Jana, S., Escobar, M., et al. (2019). Data mining to understand health status preceding traumatic brain injury. Sci. Rep. 9, 1–10. doi: 10.1038/s41598-019-41916-5
Mollayeva, T., Tran, A., Chan, V., Colantonio, A., and Escobar, M. D. (2022). Sex-specific analysis of traumatic brain injury events: applying computational and data visualization techniques to inform prevention and management. BMC Med. Res. Methodol. 22, 30. doi: 10.1186/s12874-021-01493-6
Narum, S. R. (2006). Beyond Bonferroni: Less conservative analyses for conservation genetics. Conserv. Genet. 7, 783–787. doi: 10.1007/s10592-005-9056-y
Nell, V., and Brown, D. S. O. (1991). Epidemiology of traumatic brain injury in Johannesburg-II. Morbidity, mortality and etiology. Soc. Sci. Med. 33, 289–296. doi: 10.1016/0277-9536(91)90363-H
Parks, S. E., Kegler, S. R., Annest, J. L., and Mercy, J. A. (2012). Characteristics of fatal abusive head trauma among children in the USA: 2003-2007: An application of the CDC operational case definition to National Vital Statistics data. Inj. Prev. 18, 193–199. doi: 10.1136/injuryprev-2011-040128
Reiner, A., Yekutieli, D., and Benjamini, Y. (2003). Identifying differentially expressed genes using false discovery rate controlling procedures. Bioinformatics. 19, 368–375. doi: 10.1093/bioinformatics/btf877
Rivara, F. P., Jurkovich, G. J., Gurney, J. G., Seguin, D., Fligner, C. L., Ries, R., et al. (1993). The magnitude of acute and chronic alcohol abuse in trauma patients. Arch. Surg. 128, 907–913. doi: 10.1001/archsurg.1993.01420200081015
S Tate, P., David, M. F., Charles, H. B., Stephanie, L. H., and Brinkman, S. (1999). Traumatic brain injury: influence of blood alcohol level on post-acute cognitive function. Brain Inj. 13, 767–784. doi: 10.1080/026990599121160
Sollmann, N., Echlin, P. S., Schultz, V., Viher, P. V., Lyall, A. E., Tripodis, Y., et al. (2018). Sex differences in white matter alterations following repetitive subconcussive head impacts in collegiate ice hockey players. NeuroImage Clin. 17, 642–649. doi: 10.1016/j.nicl.2017.11.020
Sollmann, N., Weidlich, D., Cervantes, B., Klupp, E., Ganter, C., Kooijman, H., et al. (2019). High isotropic resolution T2 mapping of the lumbosacral plexus with T2-Prepared 3D turbo spin echo. Clin. Neuroradiol. 29, 223–230. doi: 10.1007/s00062-017-0658-9
Stiell, I. G., Wells, G. A., Vandemheen, K., Clement, C., Lesiuk, H., Laupacis, A., et al. (2001). The Canadian CT Head Rule for patients with minor head injury. Lancet. 357, 1391–1396. doi: 10.1016/S0140-6736(00)04561-X
Sun, L., Craiu, R. V., Paterson, A. D., and Bull, S. B. (2006). Stratified false discovery control for large-scale hypothesis testing with application to genome-wide association studies. Genet. Epidemiol. 30, 519–530. doi: 10.1002/gepi.20164
Thomas, D. C., Haile, R. W., and Duggan, D. (2005). Recent developments in genomewide association scans: a workshop summary and review. Am. J. Hum. Genet. 77, 337–345. doi: 10.1086/432962
Thompson, H. J., Dikmen, S., and Temkin, N. (2012). Prevalence of comorbidity and its association with traumatic brain injury and outcomes in older adults. Res. Gerontol. Nurs. 5, 17–24. doi: 10.3928/19404921-20111206-02
Tsai, A. C., Hsueh, H., and Chen, J. J. (2003). Estimation of false discovery rates in multiple testing : application to gene microarray data. Biometrics. 59, 1071–1081. doi: 10.1111/j.0006-341X.2003.00123.x
Verhoeven, K. J. F., Simonsen, K. L., Mcintyre, L. M., Verhoeven, K. J. F., Simonsen, K. L., Mcintyre, L. M., et al. (2017). Implementing false discovery rate control : increasing your power. Oikos. 108, 643–647. doi: 10.1111/j.0030-1299.2005.13727.x
Walker, R. L., Quan, H., Hennessy, D. A., Johansen, H., Sambell, C., Lix, L., et al. (2012). Implementation of ICD-10 in Canada: How has it impacted coded hospital discharge data'. BMC Health Serv. Res. 12, 1–9. doi: 10.1186/1472-6963-12-149
Williams, W. H., Potter, S., and Ryland, H. (2010). Mild traumatic brain injury and postconcussion syndrome: A neuropsychological perspective. J. Neurol. Neurosurg. Psychiatry. 81, 1116–1122. doi: 10.1136/jnnp.2008.171298
Keywords: Benjamini-Hochberg, Benjamini-Yekutieli, McNemar test, ICD-10 codes, health-administrative data
Citation: Jana S, Sutton M, Mollayeva T, Chan V, Colantonio A and Escobar MD (2022) Application of multiple testing procedures for identifying relevant comorbidities, from a large set, in traumatic brain injury for research applications utilizing big health-administrative data. Front. Big Data 5:793606. doi: 10.3389/fdata.2022.793606
Received: 11 May 2022; Accepted: 25 August 2022;
Published: 28 September 2022.
Edited by:
Enrico Capobianco, Jackson Laboratory, United StatesReviewed by:
Sukhpal Singh Gill, Queen Mary University of London, United KingdomLisa Lix, University of Manitoba, Canada
Copyright © 2022 Jana, Sutton, Mollayeva, Chan, Colantonio and Escobar. This is an open-access article distributed under the terms of the Creative Commons Attribution License (CC BY). The use, distribution or reproduction in other forums is permitted, provided the original author(s) and the copyright owner(s) are credited and that the original publication in this journal is cited, in accordance with accepted academic practice. No use, distribution or reproduction is permitted which does not comply with these terms.
*Correspondence: Sayantee Jana, sayantee.jana@math.iith.ac.in