- 1Department of Medical Physiology, Biomedical Engineering Program, Makerere University, Kampala, Uganda
- 2Habib Medical School, Islamic University in Uganda (IUIU), Kampala, Uganda
- 3Department of Human Anatomy, Makerere University, Kampala, Uganda
- 4Department of Mechanical Engineering, Makerere University, Kampala, Uganda
Background: Leukemia encompasses various subtypes, each with unique characteristics and treatment approaches. The challenge lies in developing targeted therapies that can effectively address the specific genetic mutations or abnormalities associated with each subtype. Some leukemia cases may become resistant to existing treatments over time making them less susceptible to chemotherapy or other standard therapies.
Objective: Developing new treatment strategies to overcome resistance is an ongoing challenge particularly in Low and Middle Income Countries (LMICs). Computational studies using COMSOL software could provide an economical, fast and resourceful approach to the treatment of complicated cancers like leukemia.
Methods: Using COMSOL Multiphysics software, a continuous flow microfluidic device capable of delivering anti-leukemia drugs to early-stage leukemia cells has been computationally modeled using dielectrophoresis (DEP).
Results: The cell size difference enabled the micro-particle drug attachment to the leukemia cells using hydrodynamic focusing from the dielectrophoretic force. This point of care application produced a low voltage from numerically calculated electrical field and flow speed simulations.
Conclusion: Therefore, such a dielectrophoretic low voltage application model can be used as a computational treatment reference for early-stage leukemia cells with an approximate size of 5 μm.
1. Introduction
Due to the prevailing limited data and resources, 80% of the 300,000 children diagnosed with cancer annually emerge from LMICs (1–3). Worse still, unlike liquid tumors like leukemia, much of the diagnosis and treatment best suits solid tumors which are easier to detect and diagnose. Liquid tumors, though also characterized by genetic abnormalities, may exhibit more complex molecular profiles, making targeted therapies more challenging to develop and implement. Additionally, evaluating treatment response in liquid tumors can be more complex, as circulating cancer cells may not be as easily quantifiable or measurable using traditional imaging methods (4). Leukemia encompasses various subtypes, each with unique characteristics and treatment approaches. The challenge lies in developing targeted therapies that can effectively address the specific genetic mutations or abnormalities associated with each subtype. Some leukemia cases may become resistant to existing treatments over time (5). This resistance can occur due to genetic mutations or changes within leukemia cells, making them less susceptible to chemotherapy or other standard therapies. Developing new treatment strategies or combination therapies to overcome resistance is an ongoing challenge.
Leukemia patients are treated using chemotherapy as the number one treatment method in LMICs. Such a treatment method renders poor selectivity, low treatment efficacy, hair loss, muscle weakening, general body weakness and high remission periods. These effects worsen as the tumor changes from solid to liquid nature which characterizes leukemia cancer cells (6–8). Additionally, this leukemia treatment is hampered more by the infrastructural challenges causing longer travel/wait times and assessment delays (9, 10–12). As compared to other treatment methods, many researchers have highlighted Modeling and Simulation Approaches (MSAs) as phenomenal approaches in targeting leukemia cells (13–16). These approaches have provided early detection techniques which are key factors in cancer treatment hence leading to long-term cancer survival for patients from the western world (17–21). Although many of these approaches involve in vitro and in vivo tools, computational tools have also emerged as preparatory tools capable of providing deeper insight than in vitro/in vivo tools (22, 23). Additionally, such computational models can easily provide a stepping platform for LMICs with limited data and therapeutic results (24).
In comparison to the proposed computational DEP approach, several leukemia treatment approaches that have been used include the following; Targeted Therapies (25)—These therapies focus on targeting specific molecules involved in leukemia growth and survival. Tyrosine kinase inhibitors (TKIs) have been used for certain types of leukemia, such as chronic myeloid leukemia (CML). Imatinib, dasatinib, and nilotinib are examples of TKIs that have shown promising results. Immunotherapy (26–28)—Chimeric Antigen Receptor (CAR) T-cell therapy has gained attention for the treatment of certain leukemias. CAR T-cell therapy involves modifying a patient's own immune cells to target and kill leukemia cells. Approved therapies like Kymriah and Yescarta have shown remarkable success in treating certain types of leukemia and lymphoma. Stem Cell Transplantation (29)—Allogeneic stem cell transplantation (bone marrow transplant) remains a crucial treatment option for many leukemia patients. Advances in this field include better matching techniques, reduced-intensity conditioning regimens, and improved post-transplant care. New Drug Approvals (30–31)—New drugs continue to be developed and approved for different types of leukemia. Venetoclax, for instance, has shown promise in treating certain cases of chronic lymphocytic leukemia (CLL) and acute myeloid leukemia (AML). Gene Editing (32)—Emerging technologies like CRISPR-Cas9 hold potential for treating leukemia by targeting and modifying specific genes in leukemia cells. Combination Therapies (33–35)—Researchers are exploring the use of combination therapies that involve different drugs or treatment approaches to improve outcomes and reduce resistance. Minimal Residual Disease (MRD) Monitoring (36, 37)—Advances in MRD detection allow for more sensitive monitoring of treatment response. This helps doctors assess the effectiveness of treatment and make informed decisions about further interventions. Precision Medicine (38–40)—As our understanding of the genetic and molecular basis of leukemia improves, personalized treatment plans based on a patient's specific genetic profile are becoming more common. Supportive Care (41, 42)—Improved supportive care measures, including management of side effects and infections, have led to better outcomes and quality of life for leukemia patients undergoing treatment.
Modeling and simulation using software like COMSOL can play a significant role in providing treatment reference models for leukemia. By using COMSOL software, researchers can create computational models that simulate the behavior of leukemia cells, the interaction with the immune system, and the impact of various treatment options. These models can help deepen our understanding of the disease, its progression, and the underlying mechanisms (43–46). COMSOL software allows for the creation of patient-specific models based on individual characteristics such as genetic information, medical history, and diagnostic test results. These models can be used to predict the response of a specific patient to different treatment strategies, helping doctors make informed decisions and design personalized treatment plans. Simulating the effects of different drug compounds on leukemia cells using COMSOL can aid in drug development. Researchers can test virtual compounds in silico, analyze their interactions with specific cellular targets, and predict their efficacy. This approach can help identify potential therapeutic agents and optimize drug dosages before moving to in vitro or clinical trials. Once a treatment plan is initiated, COMSOL can assist in monitoring the patient's response to therapy. By integrating real-time patient data with the computational model, healthcare providers can track the progression of leukemia, assess treatment effectiveness, and predict potential relapses. This information can guide treatment adjustments and optimize patient outcomes. COMSOL can facilitate the design and execution of virtual clinical trials. Instead of relying solely on costly and time-consuming traditional trials, researchers can simulate the effects of different treatment protocols on a large virtual patient population. This approach enables the evaluation of treatment strategies more efficiently, potentially accelerating the development and approval of new therapies. While using COMSOL, DEP where polarizable particles experience a force when exposed to a non-uniform electric field can be utilized to manipulate and separate cells or particles based on their electrical properties, such as their dielectric properties or surface charge (47, 48). In the context of leukemia treatment, microfluidic channels with integrated DEP systems could potentially offer several benefits. For example, DEP can be used to isolate and separate leukemia cells from blood samples, allowing for the detection and analysis of circulating tumor cells. This could aid in the diagnosis and monitoring of leukemia progression. Additionally, DEP-based microfluidic systems could enable the precise manipulation and positioning of cells, facilitating various treatment strategies. For instance, the targeted delivery of therapeutic agents to leukemia cells or the sorting of different cell populations based on their response to specific treatments. The systematic nature and cost-effectiveness of computational modeling and simulation has facilitated the understanding of several cancer-related therapies. Experimental designs are not only time-consuming, but they are also cumbersome and expensive as compared to computational models. Therefore, computational models might assist in analyzing the different leukemia cancer states to reduce the burden posed by the experimental approaches (49). Our group recently published a review showing a technological advancement in leukemia treatment concerning MSAs for HICs. This review characterized several computational models using various software platforms and revealed a gap in the usage of such models to enhance leukemia treatment in LMICs (50). Modeling and simulation using DEP is among the options that have revolutionized therapy. There is always a need to observe a specific voltage threshold while applying the DEP fields. This is because cell membrane permeabilization only occurs at a critical threshold that can prevent irreversible damage to the normal cells (51). Dielectrophoretic field parameters should therefore be adapted to each leukemic cell size to preserve the viability of the normal cells. Table 1 presents a summary of different dielectrophoretic models with their respective threshold voltages and therapeutic use.
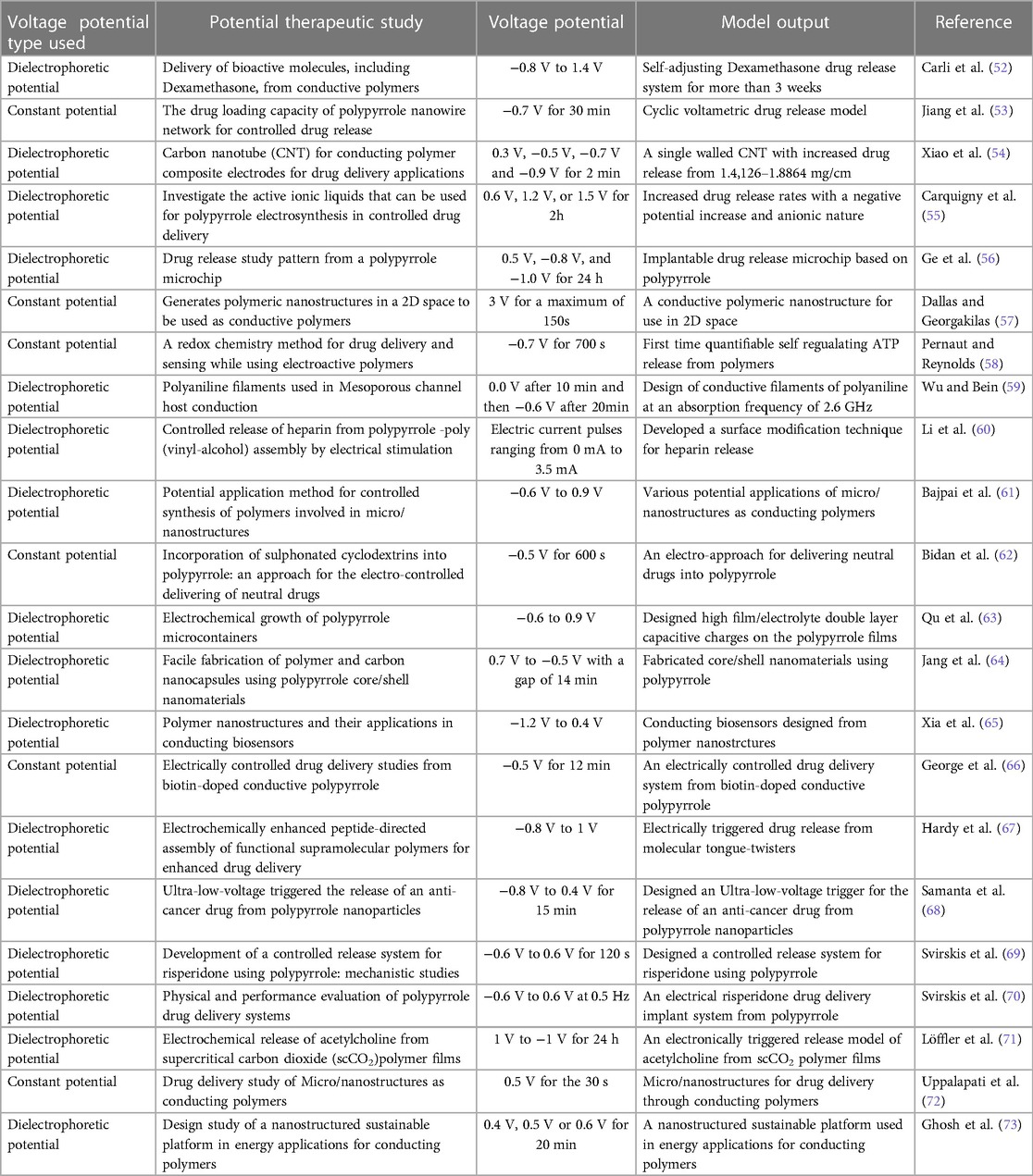
Table 1. Micro-fluidic models using either dielectrophoretic or constant voltage potentials and their corresponding therapeutic uses.
Unfortunately, most of these models are in-vitro using polymers that would require dedicated resources, specialized skills, and more time to implement. Such challenges have motivated our work to come up with a novel computational model that can provide useful insights and formulations for leukemia treatment in LMICs.
2. Methods
By exploiting the fact that the smallest leukemia cell can be around 5 micrometers in size (74) and can easily be seen by light microscopes (75) commonly used in LMICs, it is possible to provide a treatment reference for such early-stage leukemia cells. This can be through simulating and studying size-based attachment of anti-cancer microdrugs to the tiniest leukemia cells using a DEP force. When the electric field is computed in the frequency domain, the dielectrophoretic force feature adds contribution (Equation 1) to the total force exerted on the particles. Both leukemia and the iron-oxide particles onto which the drug is conjugated are assumed to be spherical. The following parameter values are additionally assumed while simulating the drug attachment as shown in Table 2 (48),
where
• rp = radius of a spherical particle in the field,
• = 8.854187817·10−12 F/m is the vacuum permittivity,
• = complex relative permittivity of the buffer,
• = complex relative permittivity of the particle,
• Erms = root mean square electric field.
The shell sub-node is used to model the drugs that are conjugated onto the micro iron-oxide particles. This sub-node is added to the dielectrophoretic force node to model the dielectrophoretic force on particles with thin dielectric shells. The electrical conductivity of the drug is different from the electrical conductivity of the iron-oxide particle to easily simulate the drug attachment application. When computing the dielectrophoretic force, the complex permittivity of the particle is replaced by the equivalent complex relative permittivity of a homogeneous particle comprising both the shell (drug) and the interior of the particle. Therefore, the equivalent relative permittivity in Equation 2 substitutes for in Equation 1 to compute the DEP force (48).
where
and = outer and inner radii of the shell, respectively,
= complex relative permittivity of the particle,
= complex relative permittivity of the outer shell.
3. Results
Figure 1A shows a proposed Y- configuration model design that can be used to deliver the anti-cancer drugs to the leukemia cells using the dielectrophoretic force. The proposed simulation model partly resembles a physical model previously fabricated for functionalizing nanoparticles (78). By convention, the left and right sides of the channel are taken in the direction the particles see while flowing. The model assumes a planar liquid electrode pattern at the bottom with dead-end chambers positioned perpendicularly to the main channel, as defined by Mernier et al. (79). Such chambers help in providing homogeneous electrical fields over the total channel height while keeping a simple process flow with a single planar metal layer. DEP signals are applied on liquid electrodes placed on both sides of the channel as partly described by Tornay et al. (80). The dielectrophoretic force attracts the leukemic cells to the micro drug particles during the movement process. The proposed model assumes a buffer flow to focus the cell towards the right side of the channel using the dielectrophoretic force. As the cells considered here have sizes larger than 500 nm, their diffusion in the attachment section can be considered to be negligible (80).
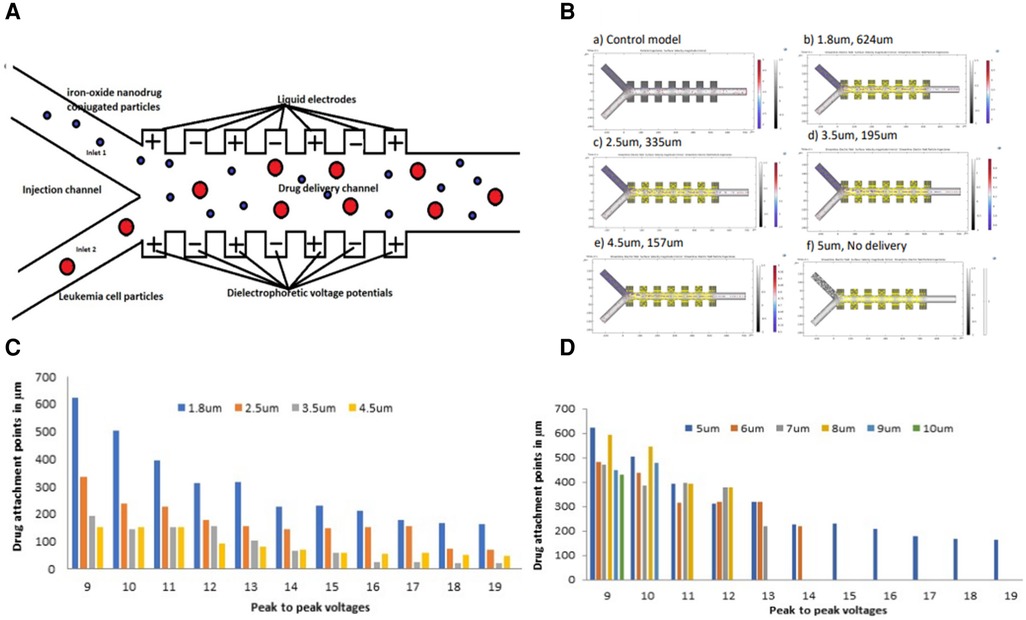
Figure 1. (A) Schematic geometry of the dielectrophoretic micro model used for drug attachment to the leukemia cells (B) simulations showing drug attachment points for 1.8 μm, 2.5 μm, 3.5 μm, and 4.5 μm iron-oxide particle sizes conjugated with a drug at a 9 V peak-to-peak (C) a histogram showing drug attachment points from the drug conjugated onto various iron-oxide particle sizes to a 5 μm leukemia cell along the micro-channel at different peak-to-peak voltages (D) a histogram showing drug attachment points onto various leukemia cell sizes along the micro-channel at different peak-to-peak voltages.
The force vectors used to calculate the trajectory of the particles are obtained by data post-processing using COMSOL software version 6.0 and calibrating the particle position with the steady-state velocity. The model uses the following physics interfaces to carry out the numerical simulations. (1) Electric Currents to model the electric field in the microchannel, (2) Creeping flow to model the fluid flow and (3) Particle tracing for fluid flow to compute the trajectories of the leukemia cells and the micro-drug under the influence of hydrodynamic focusing and dielectrophoretic forces. Our group simulated three parameters in studying the drug attachment to the leukemia cells using this model and these included; (1) Dielectrophoretic peak-to-peak voltage, (2) Leukemia cell size and (3) Iron-oxide particle size conjugated with the drug. Starting with the 5 μm leukemia cell size as our reference early-stage size of leukemia cell and 1.8 μm iron-oxide particle size conjugated with the drug, 9 Vpp was applied and the simulation ran for approximately 3 min. More voltages were applied ranging from 9 Vpp to 19 Vpp beyond which no attachment could be registered. Various leukemia cell sizes were also simulated ranging from 5 μm to 10 μm while keeping a constant 1.8 μm iron-oxide particle size and constant peak-to-peak voltage. Iron-oxide particle sizes were also simulated ranging from 1.8 μm to 5 μm while keeping a constant 5 μm leukemia cell size and constant peak-to-peak voltage. All these simulation values were performed up to a point when no drug attachment could be attained.
Attraction by positive DEP is used here in combination with hydrodynamic focusing to enable particle attachment (80).
Figure 1B shows the drug attachment simulations from 1.8 μm, 2.5 μm, 3.5 μm, to 4.5 μm iron-oxide particle sizes conjugated with a drug at 9 V peak-to-peak. The simulations were also taken at a constant leukemia cell size of 5 μm. A control model clearly shows no attachment of the drug to the leukemia cells without DEP force. The simulations also indicate a general decrease in the drug attachment points from 624 μm to 157 μm with an increase in the iron-oxide particle size from 1.8 μm to 4.5 μm. Finally, the model reaches a final point with a 5 μm iron-oxide particle size from which no drug was attached to the leukemia cells.
Figure 1C shows a cross-section of drug attachment points onto a 5 μm leukemia cell using various iron-oxide particle sizes onto which a drug is conjugated. Although there was a steady decrease in the attachment points as the peak-to-peak voltages increased, all the various iron-oxide particle sizes were able to deliver the drug to the 5 μm leukemia cell.
Figure 1D shows a cross-section of drug attachment points onto various leukemia cell sizes using different peak-to-peak voltages. These results show a tremendous decrease (improved attachment efficiency) in the attachment points with an increase in the voltages. The results additionally show a drug attachment failure as the cells increased in size with an increase in the voltage potential.
4. Discussion
The electrical conductivity of iron-oxide particles is due to the de-localization of π-electrons along the π-conjugated backbone. Such delocalized electrons get stabilized by dopant ions to the oxidized leukemia cells and then create a continuous conduction flow. During such a flow, the drug conjugated onto the micro iron-oxide particle undergoes a redox reaction with the leukemia cells leading to a drug attachment onto this cell (81). Such drugs are mainly released by electrostatic repulsion caused by an applied potential that reduces the iron-oxide particles to attach the drug to the leukemia cells. The drug attachment rate depends on the morphology of the iron-oxide particle (including its size and density) and its electromechanical properties. The parameters used during this simulation protocol (especially the peak-to-peak voltages and the size variations) directly affect the electrical properties of the iron-oxide particles and the preceding drug attachment rate. Although other parameters like the pH and the surrounding temperatures can also affect the drug attachment patterns, the peak-to-peak voltages and the particle sizes can additionally be customized in various drug attachment studies (82). This simulation protocol similarly affects the drug attachment rate as it does the response of the particles to the electric field. The drug conjugated onto the iron-oxide particle was designed with a tiny thickness to become non-electroactive at applied voltage potentials during the drug attachment onsets (83). This tiny thickness was aimed at providing a neutrally charged drug that easily attaches to the iron-oxide particles without being limited by either the positive or the negative charges (84). Dielectrophoretic fields may have different effects on cellular structures related to pulse durations and strengths. Such fields cause the cell membrane effects to decrease while increasing the intracellular effects to enhance the attachment process (85). Furthermore, increasing the iron-oxide particle diameter with the dielectrophoretic voltage enhanced the attachment efficiency as shown in Figures 1C,D. However, in Figure 1D, due to a further increase in leukemia cell particles from 6 μm to 10 μm at a constant iron oxide micro particle size of 1.8 μm, there was no drug attachment.
The surface area to volume (SAV) ratio plays a crucial role in the attachment of iron-oxide micro particles to micro-sized leukemia cells during targeted drug simulations. In the context of drug delivery, it determines the available surface area for interactions between the particles and the cells (86, 87). A higher SAV ratio means a larger surface area relative to the volume of the particles. This increased surface area provides more sites for attachment and enhances the probability of interaction between the iron-oxide micro particles and the leukemia cells. Consequently, a higher SAV ratio can promote more effective and efficient attachment of the particles to the cells, leading to improved targeted drug delivery.
The attachment process relies on various factors, including surface chemistry, charge, and ligand functionalization of the particles, as well as the characteristics of the target cells (88, 89–91). However, assuming all other factors remain constant, a higher SAV ratio generally facilitates greater contact between the particles and the leukemia cells, increasing the likelihood of successful attachment. This phenomenon explains the reason as to why no attachment occurred between 1.8 μm iron oxide particles and leukemia cell particles greater than 6 μm. Therefore, by maximizing the SAV ratio, researchers can enhance the binding efficiency of iron-oxide micro particles to leukemia cells during targeted drug simulations, ultimately improving the efficacy of drug delivery systems designed for treating leukemia or other diseases.
Although our proposed model is computationally microfluidic, it rhymes well with physically designed models that have used DEP with whole blood samples. DEP has been studied as a method to isolate Circulating Tumor Cells (CTCs) from whole blood samples based on their different electrical properties compared to normal blood cells (92). Once isolated, these CTCs can be analyzed for genetic mutations, drug susceptibility, and other factors that can guide treatment decisions. A separation test was conducted with live K562 cells, achieving a high separation efficiency of 94.74% using the DEP force at 7 Vp-p with 10 kHz. Separation efficiencies were evaluated for treated samples at 12 and 24-h durations, achieving high efficiencies of 94.71% and 93.25%, respectively. Other researchers have employed DEP analysis to delve into the underlying mechanisms governing separation and apoptosis principles at both gene and cellular levels using different leukemia cells (93, 94). The discernible variations in cell membrane capacitance and cytoplasmic conductivity, as identified by DEP analysis, offer the potential for the early detection of apoptosis. This technology holds the promise of aiding physicians in promptly detecting apoptosis, facilitating more timely and tailored patient treatments. As the field continues to evolve, the advancement of this technology could pave the way for increasingly individualized and personalized therapeutic approaches for patients.
The cost of leukemia treatment can vary significantly depending on several factors, including the type of leukemia, the stage of the disease, the specific treatment regimen prescribed, the location of treatment, the duration of treatment, and the healthcare system of the country. Leukemia treatment costs can encompass a wide range of expenses, such as consultations, diagnostic tests, medications, hospital stays, procedures, supportive care, and follow-up appointments. It's important to note that leukemia treatment costs can be substantial, and they can create financial burdens for patients and their families. Although different attempts have been implored to reduce the leukemia treatment costs (25, 95–98), the overall prices may still be unaffordable to the LMICs, hence necessitating cheaper and affordable options. While our proposed model may not directly lower the costs of medications or medical procedures, it can help make the treatment process more efficient and targeted, potentially leading to cost savings in the long run. The setup involves acquisition of a one-time strong computer that could cost approximately $1,500, COMSOL yearly software license and modules that could cost $3,500. Such a setup could support as many patients as possible and could provide pre-treatment guidance to the Physicians.
5. Conclusion
We have computationally developed a simulation model able to deliver micro-drugs to the early-stage leukemia cells. The device uses a combination of flow focusing and DEP to deliver these drugs to the cells depending on their size. The electrical field and flow speed have been calculated by numerical simulations. Furthermore, the use of relatively low voltages makes it suitable for point-of-care applications. In a broader perspective, the proposed model can be used to deliver micro-drugs to other cancer cell types featuring similar differences in size. Such a model provides a favorable discussion about the efficacy, safety and the affordability plans of the therapy before implementing it to the patient.
Data availability statement
The original contributions presented in the study are included in the article/Supplementary Material, further inquiries can be directed to the corresponding author.
Ethics statement
Ethical review and approval was not required for this study in accordance with the national legislation and the institutional requirements.
Author contributions
HK performed the repeated rounds of modeling and simulation, RS and JK designed, tailored and supervised the study, HM, ML and CI analyzed the data. All authors contributed to the article and approved the submitted version.
Funding
We are grateful to the African Centre of Excellence in Materials, Product Development and Nanotechnology (MAPRONANO ACE) under Makerere University for funding this work. We are additionally grateful to the Ugandan Government through Makerere Research and Innovation Fund (MakRIF) for their kind contribution towards this work.
Acknowledgments
We are grateful to COMSOL Multiphysics company for all the support rendering in modeling and simulating this drug attachment design. COMSOL was not involved in the study design, analysis, interpretation of data, the writing of this article or the decision to submit it for publication.
Conflict of interest
The authors declare that the research was conducted in the absence of any commercial or financial relationships that could be construed as a potential conflict of interest.
Publisher's note
All claims expressed in this article are solely those of the authors and do not necessarily represent those of their affiliated organizations, or those of the publisher, the editors and the reviewers. Any product that may be evaluated in this article, or claim that may be made by its manufacturer, is not guaranteed or endorsed by the publisher.
References
1. Ramirez O, Aristizabal P, Zaidi A, Ribeiro RC, Bravo LE. Implementing a childhood cancer outcomes surveillance system within a population-based cancer registry. J Glob Oncol. (2018) 4:1–11. doi: 10.1200/jgo.17.00193
2. Sharma H, Mishra PK, Talegaonkar S, Vaidya B. Metal nanoparticles: a theranostic nanotool against cancer. Drug Discov Today. (2015) 20(9):1143–51. doi: 10.1016/j.drudis.2015.05.009
3. Slone JS, Slone AK, Wally O, Semetsa P, Raletshegwana M, Alisanski S, et al. Establishing a pediatric hematology-oncology program in Botswana. J Glob Oncol. (2018) 4:1–9. doi: 10.1200/jgo.17.00095
4. Jiang Y, Lin W, Zhu L. Targeted drug delivery for the treatment of blood cancers. Molecules. (2022) 27(4):1–16. doi: 10.3390/molecules27041310
5. Kampa-Schittenhelm KM, Haverkamp T, Bonin M, Tsintari V, Bühring HJ, Haeusser L, et al. Epigenetic activation of O-linked β-N-acetylglucosamine transferase overrides the differentiation blockage in acute leukemia. EBioMedicine. (2020) 54. doi: 10.1016/j.ebiom.2020.102678
6. Cuellar S, Vozniak M, Rhodes J, Forcello N, Olszta D. BCR-ABL1 tyrosine kinase inhibitors for the treatment of chronic myeloid leukemia. J Oncol Pharm Pract. (2018) 24(6):433–52. doi: 10.1177/1078155217710553
7. Deepa KV, Gadgil A, Löfgren J, Mehare S, Bhandarkar P, Roy N. Is quality of life after mastectomy comparable to that after breast conservation surgery? A 5-year follow up study from Mumbai, India. Qual Life Res. (2020) 29(3):683–92. doi: 10.1007/s11136-019-02351-1
8. Zimmermann M, Oehler C, Mey U, Ghadjar P, Zwahlen DR. Radiotherapy for non-Hodgkin’s lymphoma: still standard practice and not an outdated treatment option. Radiat Oncol. (2016) 11(1):110. doi: 10.1186/s13014-016-0690-y
9. Abdelmabood S, Fouda AE, Boujettif F, Mansour A. Treatment outcomes of children with acute lymphoblastic leukemia in a middle-income developing country: high mortalities, early relapses, and poor survival. J Pediatr (Rio J). (2020) 96(1):108–16. doi: 10.1016/j.jped.2018.07.013
10. Gavidia R, Fuentes SL, Vasquez R, Bonilla M, Ethier MC, Diorio C, et al. Low socioeconomic status is associated with prolonged times to assessment and treatment, sepsis and infectious death in pediatric fever in El Salvador. PLoS One. (2012) 7:8. doi: 10.1371/journal.pone.0043639
11. Nwagbara UI, Ginindza TG, Hlongwana KW. Health systems influence on the pathways of care for lung cancer in low- and middle-income countries: a scoping review. Global Health. (2020) 16:1. doi: 10.1186/s12992-020-00553-8
12. Sullivan M, Bouffet E, Rodriguez-Galindo C, Luna-Fineman S, Khan MS, Kearns P, et al. The COVID-19 pandemic: a rapid global response for children with cancer from SIOP, COG, SIOP-E, SIOP-PODC, IPSO, PROS, CCI, and st jude global. Pediatr Blood Cancer. (2020) 67:7. doi: 10.1002/pbc.28409
13. Burgess A, Ayala-Grosso CA, Ganguly M, Jordão JF, Aubert I, Hynynen K. Targeted delivery of neural stem cells to the brain using MRI-guided focused ultrasound to disrupt the blood-brain barrier. PLoS One. (2011) 6(11):e27877. doi: 10.1371/journal.pone.0027877
14. Salimi M, Sarkar S, Fathi S, Alizadeh AM, Saber R, Moradi F, et al. Biodistribution, pharmacokinetics, and toxicity of dendrimer-coated iron oxide nanoparticles in BALB/c mice. Int J Nanomed. (2018) 13:1483–93. doi: 10.2147/IJN.S157293
15. Sriraman SK, Aryasomayajula B, Torchilin VP. Barriers to drug delivery in solid tumors. Tissue Barriers. (2014) 2(3):e29528. doi: 10.4161/tisb.29528
16. Tefft BJ, Uthamaraj S, Harburn JJ, Klabusay M, Dragomir-Daescu D, Sandhu GS. Cell labeling and targeting with superparamagnetic iron oxide nanoparticles. J Visualized Exp. (2015) 2015:104. doi: 10.3791/53099
17. Beksisa J, Getinet T, Tanie S, Diribi J, Hassen Y. Survival and prognostic determinants of prostate cancer patients in tikur anbessa specialized hospital, Addis Ababa, Ethiopia: a retrospective cohort study. PLoS One. (2020) 15:3. doi: 10.1371/journal.pone.0229854
18. de Oliveira CM, Musselwhite LW, de Paula Pantano N, Vazquez FL, Smith JS, Schweizer J, et al. Detection of HPV E6 oncoprotein from urine via a novel immunochromatographic assay. PLoS One. (2020) 15:4. doi: 10.1371/journal.pone.0232105
19. Halalsheh H, Abuirmeileh N, Rihani R, Bazzeh F, Zaru L, Madanat F. Outcome of childhood acute lymphoblastic leukemia in Jordan. Pediatr Blood Cancer. (2011) 57(3):385–91. doi: 10.1002/pbc.23065
20. Hoekstra HJ, Wobbes T, Heineman E, Haryono S, Aryandono T, Balch CM. Fighting global disparities in cancer care: a surgical oncology view. Ann Surg Oncol. (2016) 23(7):2131–6. doi: 10.1245/s10434-016-5194-3
21. Vasudevan L, Schroeder K, Raveendran Y, Goel K, Makarushka C, Masalu N, et al. Using digital health to facilitate compliance with standardized pediatric cancer treatment guidelines in Tanzania: protocol for an early-stage effectiveness-implementation hybrid study. BMC Cancer. (2020) 20:1. doi: 10.1186/s12885-020-6611-3
22. Dawidczyk CM, Russell LM, Searson PC. Nanomedicines for cancer therapy: state-of-the-art and limitations to pre-clinical studies that hinder future developments. Front Chem. (2014) 2(Aug). doi: 10.3389/fchem.2014.00069
23. Stéphanou A, McDougall SR, Anderson ARA, Chaplain MAJ. Mathematical modelling of flow in 2D and 3D vascular networks: applications to anti-angiogenic and chemotherapeutic drug strategies. Math Comput Model. (2005) 41(10):1137–56. doi: 10.1016/j.mcm.2005.05.008
24. Jaime-Pérez JC, López-Razo ON, García-Arellano G, Pinzón-Uresti MA, Jiménez-Castillo RA, González-Llano O, et al. Results of treating childhood acute lymphoblastic leukemia in a low-middle income country: 10 year experience in northeast Mexico. Arch Med Res. (2016) 47(8):668–76. doi: 10.1016/j.arcmed.2017.01.004
25. Lachaine J, Guinan K, Aw A, Banerji V, Fleury I, Owen C. Impact of fixed-duration oral targeted therapies on the economic burden of chronic lymphocytic leukemia in Canada. Curr Oncol. (2023) 30(5):4483–98. doi: 10.3390/curroncol30050339
26. Chen KTJ, Gilabert-Oriol R, Bally MB, Leung AWY. Recent treatment advances and the role of nanotechnology, combination products, and immunotherapy in changing the therapeutic landscape of acute myeloid leukemia. Pharm Res. (2019) 36(9):125. doi: 10.1007/s11095-019-2654-z
27. Fu Q, Li ZZ, Ye J, Li ZZ, Fu F, Lin SL, et al. Magnetic targeted near-infrared II PA/MR imaging guided photothermal therapy to trigger cancer immunotherapy. Theranostics. (2020) 10(11):4997–5010. doi: 10.7150/thno.43604
28. Gao S, Yang X, Xu J, Qiu N, Zhai G. Nanotechnology for boosting cancer immunotherapy and remodeling tumor microenvironment: the horizons in cancer treatment. ACS Nano. (2021) 15(8):12567–603. doi: 10.1021/acsnano.1c02103
29. Bluhmki T, Schmoor C, Finke J, Schumacher M, Socié G, Beyersmann J. Relapse- and immunosuppression-free survival after hematopoietic stem cell transplantation: how can we assess treatment success for complex time-to-event endpoints? Biol Blood Marrow Transplant. (2020) 26:992–7. doi: 10.1016/j.bbmt.2020.01.001
30. Hemmati S, Zamenian T, Delsooz N, Zangeneh A, Mahdi Zangeneh M. Preparation and synthesis a new chemotherapeutic drug of silver nanoparticle-chitosan composite; chemical characterization and analysis of their antioxidant, cytotoxicity, and anti-acute myeloid leukemia effects in comparison to daunorubicin in a leukemic. Appl Organomet Chem. (2020) 34(2):1–12. doi: 10.1002/aoc.5274
31. Newham G, Mathew RK, Wurdak H, Evans SD, Ong ZY. Polyelectrolyte complex templated synthesis of monodisperse, sub-100 nm porous silica nanoparticles for cancer targeted and stimuli-responsive drug delivery. J Colloid Interface Sci. (2021) 584:669–83. doi: 10.1016/j.jcis.2020.10.133
32. Ashmore-Harris C, Iafrate M, Saleem A, Therapy GF-M, 2020, undefined. (n.d.). Non-invasive reporter gene imaging of cell therapies, including T-cells and stem cells. Elsevier. Available at: https://www.sciencedirect.com/science/article/pii/S1525001620301507 (Retrieved May 21, 2020).
33. Bloom M, Virostko J, Sorace A, Yankeelov T. Abstract P1-18-25: quantifying the effects of combination trastuzumab and radiation therapy in HER2 positive breast cancer under normoxic and hypoxic conditions. (2020). Available at: https://cancerres.aacrjournals.org/content/80/4_Supplement/P1-18-25.short
34. Wang BY, Lin YC, Lai YT, Ou JY, Chang WW, Chu CC. Targeted photoresponsive carbazole–coumarin and drug conjugates for efficient combination therapy in leukemia cancer cells. Bioorg Chem. (2020) 100. doi: 10.1016/j.bioorg.2020.103904
35. Zhan W. Convection enhanced delivery of anti-angiogenic and cytotoxic agents in combination therapy against brain tumor. Eur J Pharm Sci. (2020) 141. doi: 10.1016/j.ejps.2019.105094
36. Loosveld M, Nivaggioni V, Arnoux I, Bernot D, Fossat C, Michel G, et al. Comparative value of the assessment of minimal residual disease in peripheral blood at days 8 and 15 by flow cytometry in childhood acute lymphoblastic leukemia. Blood. (2016) 128(22):5270. doi: 10.1182/BLOOD.V128.22.5270.5270
37. Nikitaev V, Pronichev A, Polyakov E, Chernysheva O, Serebryakova I, Tupitsyn N. Bone marrow cells recognition methods in the diagnosis of minimal residual disease. Procedia Comput Sci. (2020) 169:353–8. doi: 10.1016/j.procs.2020.02.229
38. Chen Y, Wu T, Zhu Z, Huang H, Zhang L, Goel A, et al. An integrated workflow for biomarker development using microRNAs in extracellular vesicles for cancer precision medicine. Semin Cancer Biol. (2021) 74:134–55. doi: 10.1016/J.SEMCANCER.2021.03.011
39. Cheng L, Majumdar A, Stover D, Wu S, Lu Y, Li L. Computational cancer cell models to guide precision breast cancer medicine. Genes (Basel). (2020) 11(3):263. doi: 10.3390/genes11030263
40. Winterhoff B, Kommoss S, Heitz F, Konecny GE, Dowdy SC, Mullany SA, et al. Developing a clinico-molecular test for individualized treatment of ovarian cancer: the interplay of precision medicine informatics with clinical and health economics dimensions. AMIA Annu Symp Proc. (2018) 2018:1093–102.30815151
41. Distelhorst SR, Cleary JF, Ganz PA, Bese N, Camacho-Rodriguez R, Cardoso F, et al., Optimisation of the continuum of supportive and palliative care for patients with breast cancer in low-income and middle-income countries: executive summary of the breast health global initiative, 2014. Lancet Oncol. (2015) 16(3):e137–47. doi: 10.1016/S1470-2045(14)70457-7 (Lancet Publishing Group).25752564
42. Soanes L, Gibson F. Protecting an adult identity: a grounded theory of supportive care for young adults recently diagnosed with cancer. Int J Nurs Stud. (2018) 81:40–8. doi: 10.1016/j.ijnurstu.2018.01.010
43. Al Faruque H, Choi ES, Lee HR, Kim JH, Park S, Kim E. Targeted removal of leukemia cells from the circulating system by whole-body magnetic hyperthermia in mice. Nanoscale. (2020) 12(4):2773–86. doi: 10.1039/c9nr06730b
44. Nakielski P, Kowalczyk T, Kowalewski TA. Modeling drug release from materials based on electrospun nanofibers. (n.d.).
45. Raveendran S, University T, Sanal Sukuvihar J, Technologies S, Kumar S, Maekawa T. (n.d.). Computer simulation of drug release kinetics of Mauran-Chitosan nanoparticle in COMSOL.
46. Si XA, Gaide R. The computational simulation of electrophoretic focusing and navigation for intranasal target drug delivery with comsol. (2013). 1–5.
47. Bai G, Li Y, Chu HK, Wang K, Tan Q, Xiong J, et al. Characterization of biomechanical properties of cells through dielectrophoresis-based cell stretching and actin cytoskeleton modeling. Biomed Eng Online. (2017) 16:1. doi: 10.1186/s12938-017-0329-8
48. Piacentini N, Mernier G, Tornay R, Renaud P. Separation of platelets from other blood cells in continuous-flow by dielectrophoresis field-flow-fractionation. Biomicrofluidics. (2011) 5(3):1–8. doi: 10.1063/1.3640045
49. Irfan SA, Shafie A, Yahya N, Zainuddin N. Mathematical modeling and simulation of nanoparticle-assisted enhanced oil recovery—a review. Energies. (2019) 12:8. doi: 10.3390/en12081575
50. Kiwumulo HF, Muwonge H, Ibingira C, Kirabira JB, Ssekitoleko RT. A systematic review of modeling and simulation approaches in designing targeted treatment technologies for leukemia cancer in low and middle income countries. Math Biosci Eng. (2021) 18(July):8149–73. doi: 10.3934/mbe.2021404
51. Wang XB, Huang Y, Wang X, Becker FF, Gascoyne PRC. Dielectrophoretic manipulation of cells with spiral electrodes. Biophys J. (1997) 72(4):1887–99. doi: 10.1016/S0006-3495(97)78834-9
52. Carli S, Trapella C, Armirotti A, Fantinati A, Ottonello G, Scarpellini A, et al. Biochemically controlled release of dexamethasone covalently bound to PEDOT. Chemistry. (2018) 24(41):10300–5. doi: 10.1002/chem.201801499
53. Jiang S, Sun Y, Cui X, Huang X, He Y, Ji S, et al. Enhanced drug loading capacity of polypyrrole nanowire network for controlled drug release. Synth Met. (2013) 163(1):19–23. doi: 10.1016/j.synthmet.2012.12.010
54. Xiao Y, Ye X, He L, Che J. New carbon nanotube-conducting polymer composite electrodes for drug delivery applications. Polym Int. (2012) 61(2):190–6. doi: 10.1002/pi.3168
55. Carquigny S, Lakard B, Lakard S, Moutarlier V, Hihn JY, Viau L. Investigation of pharmaceutically active ionic liquids as electrolyte for the electrosynthesis of polypyrrole and active component in controlled drug delivery. Electrochim Acta. (2016) 211:950–61. doi: 10.1016/j.electacta.2016.06.080
56. Ge D, Tian X, Qi R, Huang S, Mu J, Hong S, et al. A polypyrrole-based microchip for controlled drug release. Electrochim Acta. (2009) 55(1):271–5. doi: 10.1016/j.electacta.2009.08.049
57. Dallas P, Georgakilas V. Interfacial polymerization of conductive polymers: generation of polymeric nanostructures in a 2-D space. Adv Colloid Interface Sci. (2015) 224:46–61. doi: 10.1016/j.cis.2015.07.008
58. Pernaut JM, Reynolds JR. Use of conducting electroactive polymers for drug delivery and sensing of bioactive molecules. A redox chemistry approach. J Phys Chem B. (2000) 104(17):4080–90. doi: 10.1021/jp994274o
59. Wu CG, Bein T. Conducting polyaniline filaments in a mesoporous channel host. Science. (1994) 264(5166):1757–9. doi: 10.1126/science.264.5166.1757
60. Li Y, Neoh KG, Kang ET. Controlled release of heparin from polypyrrole-poly(vinyl alcohol) assembly by electrical stimulation. J Biomed Mater Res A. (2005) 73(2):171–81. doi: 10.1002/jbm.a.30286
61. Bajpai V, He P, Goettler L, Dong JH, Dai L. Controlled syntheses of conducting polymer micro- and nano-structures for potential applications. Synth Met. (2006) 156(5–6):466–9. doi: 10.1016/j.synthmet.2006.01.008
62. Bidan G, Lopez C, Mendes-Viegas F, Vieil E, Gadelle A. Incorporation of sulphonated cyclodextrins into polypyrrole: an approach for the electro-controlled delivering of neutral drugs. Biosens Bioelectron. (1995) 10(1–2):219–29. doi: 10.1016/0956-5663(95)96808-C
63. Qu L, Shi G, Chen F, Zhang J. Electrochemical growth of polypyrrole microcontainers. Macromolecules. (2003) 36(4):1063–7. doi: 10.1021/ma021177b
64. Jang J, Li X, Oh JH. Facile fabrication of polymer and carbon nanocapsules using polypyrrole core/shell nanomaterials. Chem Commun. (2004) 4(7):794–5. doi: 10.1039/b316881f
65. Xia L, Wei Z, Wan M. Conducting polymer nanostructures and their application in biosensors. J Colloid Interface Sci. (2010) 341(1):1–11. doi: 10.1016/j.jcis.2009.09.029
66. George PM, Lavan DA, Burdick JA, Chen CY, Liang E, Langer R. Electrically controlled drug delivery from biotin-doped.pdf. Adv Mater. (2022) 18(5):2022. doi: 10.1002/adma.200501242
67. Hardy JG, Amend MN, Geissler S, Lynch VM, Schmidt CE. Peptide-directed assembly of functional supramolecular polymers for biomedical applications: electroactive molecular tongue-twisters (oligoalanine-oligoaniline-oligoalanine) for electrochemically enhanced drug delivery. J Mater Chem B. (2015) 3(25):5005–9. doi: 10.1039/c5tb00106d
68. Samanta D, Hosseini-Nassab N, McCarty AD, Zare RN. Ultra-low voltage triggered release of an anti-cancer drug from polypyrrole nanoparticles. Nanoscale. (2018) 10(20):9773–9. doi: 10.1039/c8nr01259h
69. Svirskis D, Wright BE, Travas-Sejdic J, Rodgers A, Garg S. Development of a controlled release system for risperidone using polypyrrole: mechanistic studies. Electroanalysis. (2010) 22(4):439–44. doi: 10.1002/elan.200900401
70. Svirskis D, Wright BE, Travas-Sejdic J, Rodgers A, Garg S. Evaluation of physical properties and performance over time of an actuating polypyrrole based drug delivery system. Sens Actuators B. (2010) 151(1):97–102. doi: 10.1016/j.snb.2010.09.042
71. Löffler S, Seyock S, Nybom R, Jacobson GB, Richter-Dahlfors A. Electrochemically triggered release of acetylcholine from scCO2 impregnated conductive polymer films evokes intracellular Ca2+signaling in neurotypic SH-SY5Y cells. J Controlled Release. (2016) 243:283–90. doi: 10.1016/j.jconrel.2016.10.020
72. Uppalapati D, Boyd BJ, Garg S, Travas-Sejdic J, Svirskis D. Conducting polymers with defined micro- or nanostructures for drug delivery. Biomaterials. (2016) 111:149–62. doi: 10.1016/j.biomaterials.2016.09.021
73. Ghosh S, Maiyalagan T, Basu RN. Nanostructured conducting polymers for energy applications: towards a sustainable platform. Nanoscale. (2016) 8(13):6921–47. doi: 10.1039/c5nr08803h
74. Hao SJ, Wan Y, Xia YQ, Zou X, Zheng SY. Size-based separation methods of circulating tumor cells. Adv Drug Delivery Rev. (2018) 125:3–20. doi: 10.1016/j.addr.2018.01.002
75. Titles B, Links DG, Microscope D, Can I, Enhanced B, Are T, et al. Looking at the structure of cells in the microscope different components of the cell can be selectively stained specific molecules can be located in cells by fluorescence microscopy imaging of complex three-dimensional objects is possible with the optical. (n.d.).
76. Egger M, Donath E. Electrorotation measurements of diamide-induced platelet activation changes. Biophys J. (1995) 68(1):364–72. doi: 10.1016/S0006-3495(95)80197-9
77. Park S, Zhang Y, Wang TH, Yang S. Continuous dielectrophoretic bacterial separation and concentration from physiological media of high conductivity. Lab Chip. (2011) 11(17):2893–900. doi: 10.1039/c1lc20307j
78. Tornay R, Braschler T, Demierre N, Steitz B, Finka A, Hofmann H, et al. Dielectrophoresis-based particle exchanger for the manipulation and surface functionalization of particles. Lab Chip. (2008) 8(2):267–73. doi: 10.1039/B713776A
79. Mernier G, Piacentini N, Braschler T, Demierre N, Renaud P. Continuous-flow electrical lysis device with integrated control by dielectrophoretic cell sorting. Lab Chip. (2010) 10(16):2077–82. doi: 10.1039/c000977f
80. Lab on a chip dielectrophoresis-based particle exchanger for the manipulation and surface functionalization of particles. (2022). 207890.
81. Wang R, Peng Y, Zhou M, Shou D. Smart montmorillonite-polypyrrole scaffolds for electro-responsive drug release. Appl Clay Sci. (2016) 134:50–4. doi: 10.1016/j.clay.2016.05.004
82. Medeiros SF, Santos AM, Fessi H, Elaissari A. Stimuli-responsive magnetic particles for biomedical applications. Int J Pharm. (2011) 403(1–2):139–61. doi: 10.1016/j.ijpharm.2010.10.011 (Elsevier B.V.)20951779
83. Puiggalí-Jou A, del Valle LJ, Alemán C. Drug delivery systems based on intrinsically conducting polymers. J Controlled Release. (2019) 309(May):244–64. doi: 10.1016/j.jconrel.2019.07.035
84. Tang T, Hosokawa Y, Hayakawa T, Tanaka Y, Li W, Li M, et al. Rotation of biological cells: fundamentals and applications. Engineering. (2022) 10:110–26. doi: 10.1016/J.ENG.2020.07.031
85. Drasler B, Sayre P, Steinhäuser KG, Petri-Fink A, Rothen-Rutishauser B. In vitro approaches to assess the hazard of nanomaterials. NanoImpact. (2017) 8:99–116. doi: 10.1016/j.impact.2017.08.002 (Elsevier B.V.)
86. Ali A, Zafar H, Zia M, ul Haq I, Phull AR, Ali JS, et al. Synthesis, characterization, applications, and challenges of iron oxide nanoparticles. Nanotechnol Sci Appl. (2016) 9:49–67. doi: 10.2147/NSA.S99986
87. Thakor AS, Gambhir SS. Nanooncology: the future of cancer diagnosis and therapy. CA Cancer J Clin. (2013) 63(6):395–418. doi: 10.3322/caac.21199
88. Gupta AK, Gupta M. Synthesis and surface engineering of iron oxide nanoparticles for biomedical applications. Biomaterials. (2005) 26(18):3995–4021. doi: 10.1016/j.biomaterials.2004.10.012
89. Hanley MJ, Mould DR, Taylor TJ, Gupta N, Suryanarayan K, Neuwirth R, et al. Population pharmacokinetic analysis of bortezomib in pediatric leukemia patients: model-based support for body surface area-based dosing over the 2- to 16-year age range. J Clin Pharmacol. (2017) 57(9):1183–93. doi: 10.1002/jcph.906
90. Li Y, Lian Y, Zhang LT, Aldousari SM, Hedia HS, Asiri SA, et al. Cell and nanoparticle transport in tumor microvasculature: the role of size, shape and surface functionality of nanoparticles. Interface Focus. (2016) 6(1). doi: 10.1098/rsfs.2015.0086 (Royal Society of London)
91. Siafaka PI, Üstündağ Okur N, Karavas E, Bikiaris DN. Surface modified multifunctional and stimuli responsive nanoparticles for drug targeting: current status and uses. Int J Mol Sci. (2016) 17(9). doi: 10.3390/ijms17091440 (MDPI AG)
92. Lv Y, Zeng L, Zhang G, Xu Y, Lu Y, Mitchelson K, et al. Systematic dielectrophoretic analysis of the ara-C-induced NB4 cell apoptosis combined with gene expression profiling. Int J Nanomed. (2013) 8:2333–50. doi: 10.2147/IJN.S31678
93. Li Y, Li J, Huan Z, Hu Y. Quantitative characterization of mechano-biological interrelationships of single cells. Int J Adv Manuf Technol. (2019) 105(12):4967–72. doi: 10.1007/s00170-019-04591-4
94. Ringwelski B, Jayasooriya V, Nawarathna D. Dielectrophoretic high-purity isolation of primary T-cells in samples contaminated with leukemia cells, for biomanufacturing of therapeutic CAR T-cells. J Phys D Appl Phys. (2020) 54:6. doi: 10.1088/1361-6463/abc2f3
95. Anglin P, Elia-Pacitti J, Eberg M, Muratov S, Kukaswadia A, Sharma A, et al. Estimating the associated burden of illness and healthcare utilization of newly diagnosed patients aged ≥65 with mantle cell lymphoma (MCL) in Ontario Canada. Curr Oncol. (2023) 30(6):5529–45. doi: 10.3390/curroncol30060418
96. Chen M, Liu L, Zhang L, Lin Y, Lu X, Yang H, et al. Cost-effectiveness analysis of imatinib versus dasatinib in the treatment of pediatric Philadelphia chromosome-positive acute lymphoblastic leukemia when combined with conventional chemotherapy in China. BMC Health Serv Res. (2023) 23:1. doi: 10.1186/s12913-023-09600-7
97. Gallardo-Pérez MM, Gale RP, Reyes-Cisneros OA, Sánchez-Bonilla D, Fernández-Gutiérrez JA, Stock W, et al. Therapy of childhood acute lymphoblastic leukemia in resource-poor geospaces. Front Oncol. (2023) 13. doi: 10.3389/fonc.2023.1187268
Keywords: dielectrophoresis, leukemia, iron-oxide micro-particle, peak-to-peak voltage, modeling and simulation, COMSOL
Citation: Kiwumulo HF, Muwonge H, Ibingira C, Lubwama M, Kirabira JB and Ssekitoleko RT (2023) A di-electrophoretic simulation procedure of iron-oxide micro-particle drug attachment system for leukemia treatment using COMSOL software: a potential treatment reference for LMICs. Front. Med. Technol. 5:1250964. doi: 10.3389/fmedt.2023.1250964
Received: 30 June 2023; Accepted: 29 September 2023;
Published: 12 October 2023.
Edited by:
Maria Del Carmen Valdés Hernández, University of Edinburgh, United KingdomReviewed by:
Arunadevi Natarajan, Bharathiar University, IndiaMahboubeh Nabavinia, The Research Institute at Nationwide Children’s Hospital, United States
© 2023 Kiwumulo, Muwonge, Ibingira, Lubwama, Kirabira and Ssekitoleko. This is an open-access article distributed under the terms of the Creative Commons Attribution License (CC BY). The use, distribution or reproduction in other forums is permitted, provided the original author(s) and the copyright owner(s) are credited and that the original publication in this journal is cited, in accordance with accepted academic practice. No use, distribution or reproduction is permitted which does not comply with these terms.
*Correspondence: Robert Tamale Ssekitoleko cnNzZWtpQGdtYWlsLmNvbQ==