- 1 Department of Psychiatry, Harvard Medical School, Boston, MA, USA
- 2 Department of Physical Medicine and Rehabilitation, Spaulding Rehabilitation Hospital, Boston, MA, USA
- 3 Department of Speech-Language Pathology and Audiology, Northeastern University, Boston, MA, USA
- 4 Department of Neurology, Boston University School of Medicine, Boston, MA, USA
- 5 Braintree Rehabilitation Hospital, Braintree, MA, USA
- 6 Department of Physical Medicine and Rehabilitation, Harvard Medical School, Boston, MA, USA
Cognitive deficits following traumatic brain injury (TBI) commonly include difficulties with memory, attention, and executive dysfunction. These deficits are amenable to cognitive rehabilitation, but optimally selecting rehabilitation programs for individual patients remains a challenge. Recent methods for quantifying regional brain morphometry allow for automated quantification of tissue volumes in numerous distinct brain structures. We hypothesized that such quantitative structural information could help identify individuals more or less likely to benefit from memory rehabilitation. Fifty individuals with TBI of all severities who reported having memory difficulties first underwent structural MRI scanning. They then participated in a 12 session memory rehabilitation program emphasizing internal memory strategies (I-MEMS). Primary outcome measures (HVLT, RBMT) were collected at the time of the MRI scan, immediately following therapy, and again at 1-month post-therapy. Regional brain volumes were used to predict outcome, adjusting for standard predictors (e.g., injury severity, age, education, pretest scores). We identified several brain regions that provided significant predictions of rehabilitation outcome, including the volume of the hippocampus, the lateral prefrontal cortex, the thalamus, and several subregions of the cingulate cortex. The prediction range of regional brain volumes were in some cases nearly equal in magnitude to prediction ranges provided by pretest scores on the outcome variable. We conclude that specific cerebral networks including these regions may contribute to learning during I-MEMS rehabilitation, and suggest that morphometric measures may provide substantial predictive value for rehabilitation outcome in other cognitive interventions as well.
Introduction
Traumatic brain injury (TBI) is a heterogeneous phenomenon, with a variety of causes, severities, and anatomical injury distributions. Despite this heterogeneity, TBI has been associated with a constellation of typical anatomical injuries as well as a prototypical set of functional deficits. Anatomical injuries typically include diffuse axonal injury (DAI), traumatic axonal injury (TAI), focal contusions in frontotemporal areas, and regions particularly vulnerable to excitotoxicity (Povlishock and Katz, 2005). Damage may be concentrated in acetylcholine dense pathways (Salmond et al., 2005), but has the potential for a wide spatial distribution (Merkley et al., 2008). In terms of long-term functional consequences of TBI, deficits are commonly observed in memory, attention, and in tasks relying heavily on executive functioning (Cicerone et al., 2000, 2006; McAllister et al., 2006). It is presumed that the consistency, albeit modest, of anatomical alterations partially accounts for the commonly observed functional deficits.
One approach to investigate the contribution of different anatomical regions to functional deficits is to utilize quantitative brain morphological measures (Bigler, 2001b). Such measures are made by dividing, or parcellating, brain tissue into separate regions using either manual processes (Kennedy et al., 1989) or computational ones (Fischl et al., 2002, 2004; Chen et al., 2004; Mega et al., 2005). Computational processes have the advantages of reducing subjectivity, analysis time, and cost, making them feasible to apply even on large cohorts of subjects. Importantly, computational approaches have exhibited accuracy similar to manual parcellation for many brain structures (Fischl et al., 2002, 2004; Desikan et al., 2009). One approach for parcellating brain tissue on magnetic resonance imaging (MRI) scans is to fit a mesh of points to the pial surface of the brain and a second mesh to the gray–white matter border. These two computed surfaces are then parcellated into regions (precentral gyrus, central sulcus, and so forth) based on regional curvature plus structural priors (Fischl et al., 2002; Han and Fischl, 2007). Regional measurements can then be made on the resulting parcellations, including regional cortical thickness, regional gray matter volume, and local surface curvature (Fjell et al., 2009; Jovicich et al., 2009).
Such morphometric measures have been used in studies of aging (Salat et al., 2004), finding regionally specific cortical thinning with age, and in pediatric TBI, finding relatively widespread cortical thinning post-injury (Merkley et al., 2008; Bigler et al., 2010). Semi-automatic parcellation techniques have demonstrated widespread gray matter volume loss in TBI (Levine et al., 2008). In adults, such volume loss has been further associated with injury severity, using both parcellation techniques (Levine et al., 2008) and voxel-based morphometry (Gale et al., 2005). Recent clinical studies have demonstrated an association between prospective memory and regional cortical thickness in children (McCauley et al., 2010), and an association between regional cortical thickness and behavioral performance in Alzheimer’s disease (Dickerson et al., 2009). These results naturally lead to the hypothesis of a link between regional cortical gray matter volumes and TBI outcome.
Indeed, a number of studies have demonstrated a positive association between the extent of anatomical injury and general TBI outcome (Azouvi, 2000; Wilde et al., 2005; Ariza et al., 2006; Jorge et al., 2007; Kraus et al., 2007). In these, more extensive or severe injury to specific brain regions is associated with poorer performance on neuropsychological and cognitive tests. Less well understood, however, is the relationship between anatomical injury and outcome from any specific rehabilitation program, particularly in chronic TBI. While TBI rehabilitation programs can help improve memory and executive function (Cicerone et al., 2000), attention (Kim et al., 2009), community integration (Cicerone et al., 2004), and functional outcomes (Giles, 2001), little work has been done to predict outcomes from specific rehabilitation programs prior to their initiation. In a previous study of chronic TBI, we showed that functional brain activity may help predict memory rehabilitation outcome in this way (Strangman et al., 2008b). However, no studies have yet examined the ability of brain morphological measures to predict outcome from a TBI rehabilitation program, despite the widespread availability of structural MRI in clinical settings as compared to fMRI. We therefore sought to test whether regional volumetric measures derived from structural MRI scans could help predict outcomes from a TBI memory rehabilitation program.
The rehabilitation program we employed was developed as part of a recent study and was designed to improve participants’ memory by training individuals to use internally based semantic association and related strategies (O’Neil-Pirozzi et al., 2010). Prior neuroimaging research has demonstrated that such internal semantic and strategic cognitive manipulations typically involve dorsolateral prefrontal cortex (DLPFC), ventrolateral prefrontal cortex (VLPFC), posterior parietal cortex (PPC), and the hippocampal region (McAllister et al., 2001; Logan et al., 2002; Strangman et al., 2008a). We therefore hypothesized that decreased gray matter volumes in these regions would predict less favorable outcomes from our rehabilitation program. Given the complex nature of TBI, however, we also planned to examine the remaining brain regions from the parcellation process as an exploratory analysis.
Materials and Methods
Participants
Participants with TBI were recruited via mailings to clients of The Commonwealth of Massachusetts Brain Injury and Statewide Specialized Community Services Department, members of the Brain Injury Association of Massachusetts, local support groups, and to patients of study-affiliated physicians. Fifty-eight individuals were initially enrolled, following a procedure approved by the Human Research Committee at the Massachusetts General Hospital. All participants (1) were at least 18 years of age at the time of injury, (2) had sustained a TBI of any severity at least 12 months prior to the study, (3) were right-handed (Oldfield, 1971) and fluent in English, and (4) self-reported as having difficulty with memory following their injury. Exclusion criteria included a score of less than 4 on either the expression or comprehension items of the FIM, non-traumatic etiology of cerebral dysfunction in addition to TBI, active major illnesses, pre-injury history of psychiatric disease, inability to read single words at an eighth-grade reading level, and current drug or alcohol dependence (criteria from the Diagnostic and Statistical Manual of Mental Disorders, 4th Edition).
From this group, data was excluded from five individuals who failed to complete at least eight of 12 memory intervention sessions and/or return for posttesting. In addition, technical difficulties for two participants’ structural scans led to insufficient image quality to attempt parcellation. And in one participant, the cortical parcellation process could not run to completion, likely due to two particularly large focal regions of cortical tissue loss (approximately 8.95 and 3.98 cm3, respectively). We proceeded with analysis on the remaining 50 participants with TBI (14 female, 36 male). Demographic characteristics for these participants appear in Table 1.
Experimental Procedures
Session 1 consisted of a series of standard neuropsychological tests of memory, executive function, and language. Outcome variables included the Hopkins Verbal Learning Test-Revised (HVLT-R) and the Rivermead Behavioral Memory Test II (RBMT-II). In the HVLT, a person is verbally presented a list of 12 words (four words from each of three semantically related groups), in a semantically unrelated order. The delayed correct recall score measures the ability to remember three presentations of these words after a 20-min delay. HVLT was a primary outcome because it is widely used, it emphasizes semantic association memory (a focus of the I-MEMS intervention), and it has multiple parallel versions to help minimize practice effects. RBMT test items include asking a patient to remember a hidden personal belonging, to remember to ask an appointment-related question, and to deliver a message. The RBMT is an ecologically valid, broad measure of impairment in everyday memory functioning. It has four parallel versions with good alternate form reliability. Other tests included the Boston Naming Test (BNT) 2nd Edition Short Form, the Boston Diagnostic Aphasia Examination (BDAE) 3rd Edition Animal Naming, Trail Making Test Part A and B, and Wechsler Memory Scale-Revised Digit Span (Forward and Backward). In Session 2, approximately 3 days later, each study participant underwent structural MRI scanning as described below.
Sessions 3 through 14 were memory intervention sessions led by one of the investigators that emphasized internal memory strategy training and were designed around evidence-based approaches; details are described in (O’Neil-Pirozzi et al., 2010). In brief, we utilized a group intervention with 3–6 members per group cycle and 2–3 group facilitators. Each group cycle ran twice weekly for 6 weeks, 90 min per session, for a total of 12 sessions. Sessions emphasized semantic organization and other internally based strategies (e.g., elaboration and imagery) from encoding, storage, and retrieval perspectives.
Session 15 (posttest 1) was conducted within 3 days after the final memory intervention session and repeated all of the tests from session 1, except for the BNT and BDAE. Session 16 (posttest 2) was conducted 1 month following session 15 and again repeated the same tests. No MRI scanning was performed at either posttest session. Forms of the various tests were counterbalanced across participants and sessions. Pre- and posttest evaluations were conducted by different individuals, with regular cross-validation of test administrators.
MRI Data Acquisition
MRI was performed with a Siemens Avanto 1.5 Tesla scanner with eight-channel Tim head-coil. Two MPRAGE sequences were collected for high-resolution anatomy: relaxation time (TR) = 1.91 s, echo time (TE) = 4.13 ms, inversion time (TI) = 1.1 s, flip angle = 15°, 120 slices, matrix 128 × 128, 1 × 1 × 1 mm resolution), which was designed to provide optimal differentiation of gray and white matter tissue for Freesurfer analysis. The following scans were also performed for neuroradiological evaluation: T2-weighted spin-echo (TR = 4.54 s, TE = 110 ms, 21 slices, 0.8 × 0.4 × 5.0 mm), FLAIR (TR = 9.0 s, TE = 89 ms, TI = 2500 ms, 21 slices, 1.0 × 0.8 × 5.0 mm), T2-weighted hemosiderin scan (TR = 800 ms, TE = 26 ms, flip angle = 30°, 21 slices, 1.1 × 0.9 × 5.0 mm) and one diffusion-weighted echo planar scan (TR = 5.0 s, TE = 84 ms, 23 slices, 1.7 × 1.7 × 5.0 mm, 30 directions, six B0 images).
Data Analysis
Neuropsychological tests were scored per respective standards. The two rehabilitation outcome measures we considered were (1) the HVLT delayed correct recall score (range: 0–12), and (2) the RBMT total standardized profile score (range: 0–24). Injury severity was categorized as mild, moderate or severe based on a method used in our previous studies (Strangman et al., 2008b). In brief, 85% of cases were based on duration of loss of consciousness (LOC), (Ruff et al., 1993; Katz and Alexander, 1994; Dikmen et al., 2003) where mild was 0–30 min LOC (unless post traumatic amnesia exceeded 24 h); moderate was 30 min < LOC ≤ 24 h, and >24 h LOC was designated severe. In the absence of LOC data, the determination was made based on Glasgow coma scale scores (<9 = severe, 9–12 = moderate, 13–15 = mild) (Katz and Alexander, 1994; Temkin et al., 1995).
Structural MRI data was analyzed using Freesurfer v4.5 (Fischl et al., 2004), with the associated recon-all processing stream applied to each participant’s pair of MPRAGE scans. In brief, the preprocessing stream consists of co-registering the two scans, non-uniform intensity normalization, Talairach transformation, skull stripping, volumetric labeling, tissue type segmentation, fitting of the two cortical surfaces (pial and white matter), and cortical parcellation. From this, data summaries for each cortical region are computed, including regional volume, surface area, curvature, and thickness; for details, including information on the 2009 version of the Destrieux atlas used for parcellation (see Fischl et al., 1999; Fischl et al., 2004; Destrieux et al., 2010; Schmansky, 2010). The Destrieux atlas was selected, as opposed to the Desikan atlas (Desikan et al., 2006), because it differentiates gyral and sulcal tissue. We deemed this difference potentially important in TBI where many gyri (as opposed to sulci) may contact the skull upon impact. In all, we collected – for each subject – gray matter volumes for 75 labeled cortical regions per hemisphere (see Figure 1) plus gray matter volume in 23 distinct subcortical regions. Of these latter 23 regions, we retained nine – specifically excluding total hemisphere measures, ventricles, vessels, image hypo-intensities, and the optic chiasm (Fischl et al., 2002). Upon completion of the automated processing, results were manually examined for clear failures to fit cortical surfaces (e.g., crossover of pial and white matter surfaces, errors related to inadequate skull stripping), major topological defects (holes or handles) in these surfaces, and failures in subcortical segmentation (e.g., distinct “islands” for contiguous regions). We sought to identify only gross errors for two reasons: (1) manual editing can cause bias, and (2) such editing is impractical in many clinical settings. Beyond the one failure to reach process completion (indicated earlier), no defects or failures were identified as requiring correction.
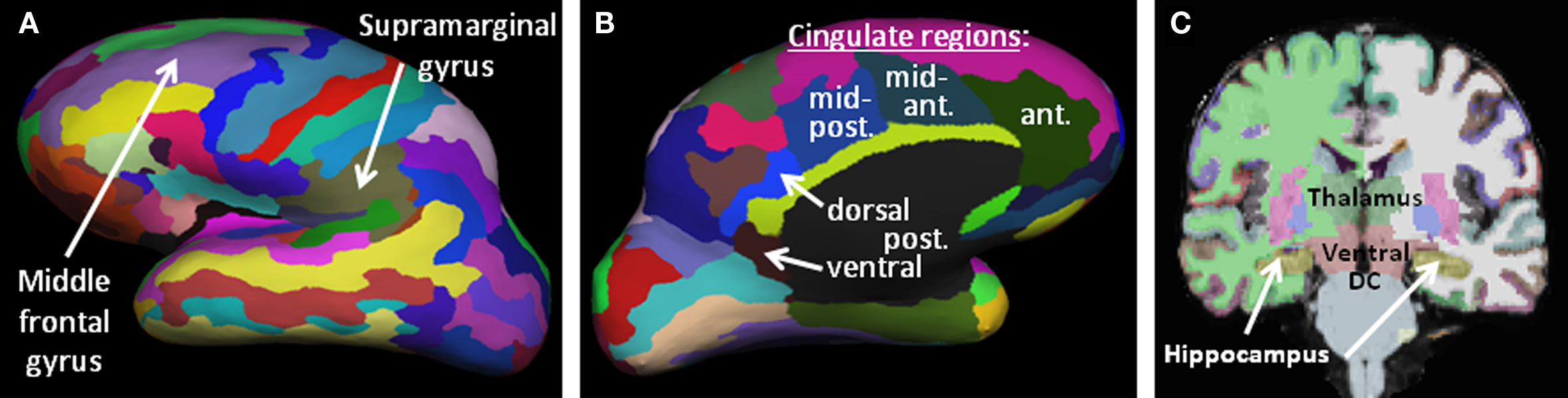
Figure 1. Brain morphometric parcellation results for an example subject, identifying key regions from this study. (A,B) Lateral and medial representations of the inflated cortical surface showing the 75 parcellated cortical regions per hemisphere. Only the left hemisphere is shown, laterally (A) and medially (B). (C) Coronal slice highlighting several subcortical structures automatically identified by the Freesurfer algorithms (thalamus, ventral diencephalon, hippocampus, basal ganglia, etc).
Regions of Interest
As discussed, we hypothesized that the volume of the dorsolateral and ventrolateral prefrontal cortex (DLPFC, VLPFC), the PPC and the hippocampus were likely to provide significant predictive value for rehabilitation outcome. The Freesurfer region most coincident with the DLPFC region previously identified as involved in strategic memory processing (Strangman et al., 2008b) was the middle frontal gyrus (mFG). Our VLPFC region, also derived from (Strangman et al., 2008b), spanned two cortical labels, so we examined both the triangular and opercular parts of the inferior frontal gyrus. The predicted region of the PPC also straddled two cortical labels, so we examined both the angular and supramarginal portions of the inferior parietal gyrus. Each subject contributed two volume measurements per region, one from each hemisphere, as we hypothesized that the integrity of any particular region could be important bilaterally and in fact one hemisphere may “compensate” for damage in the opposite hemisphere (Cramer, 2004).
In addition to analysis of our a priori regions of interest, the remaining 70 cortical regions as well as eight of the subcortical brain regions (excluding ventricles, and other non-gray matter tissue types) were retained for further analysis, for a total of 84 regions analyzed.
Constructing the Prediction Model
Selecting a prediction model to use for all brain regions was achieved via a two-pass procedure. In the first pass, we began with a linear mixed effects regression model to predict posttest outcome from the following predictors: pretest outcome, age, gender, time since injury, education, moderateTBI, severeTBI, regional volume, TIV, and regional volume*TIV, where posttest outcome was either the HVLT delayed correct recall score or RBMT total standardized profile score at posttest 1 or 2 (i.e., immediately or 1 month after completion of therapy), pretest outcome was the same outcome measure at pretest, age was the participant’s age in decades at the time of the MRI, gender was a binary variable, education and time since injury were in years, regional volume was either the left or right hemisphere volume of the brain region being tested, total intracranial volume (TIV) was the reciprocal of the volume of the total brain mask (gray matter plus white matter plus ventricles and cerebrospinal fluid, as generated by Freesurfer), and finally the interaction of regional volume with TIV. These last three terms were needed to compensate for the relationship between regional volumes and TIV, given that regional volume tends to be positively associated with TIV (Kronmal, 1993). ModerateTBI and severeTBI were binary variables indicating whether a participant had sustained a moderate or severe injury, as determined from Glasgow Coma Scale and length of loss of consciousness data (Strangman et al., 2008b). This model was fit for each region and outcome, and we then counted the number of models where each term survived a simple p < 0.05 significance threshold. Using this process, education, time since injury, and gender terms never reached significance in any of the models and were discarded from further consideration.
In the second pass, we computed the following multiple linear regression model for each brain region, which retained the remaining terms from our initial fits:
posttest_outcome = pretest_outcome + age + moderateTBI + severeTBI + regional_volume + TIV + regional_volume*TIV
The separate regional volume measurements are largely independent measures, but not entirely so due to part–whole relationships, so the optimal adjustment for multiple comparisons is not known. Due to the exploratory component of the study, we report all models where the final volume coefficient exhibited p < 0.05 uncorrected, discussing in detail those that that meet a conservative Bonferroni correction: for our primary hypotheses this is 0.5/6 = 0.0083, and for the whole-brain search this is p < 0.05/84 regions = 0.0006.
Supplementary Analyses
Two additional analyses were also conducted. First, we sought to directly test for laterality effects to identify any potential hemispheric asymmetries. To do so, we utilized the same final regression model as described in the previous section, but added a binary variable to indicate whether the regional volume data came from the participant’s left or right hemisphere.
Second, all candidate final models were checked for non-additivity, non-normality of residuals, quadratic components of regional volume and age, and effects of leverage. Leverage was identified by computing Cook’s distance for every data point in the regression and determining whether the largest and fourth largest distance values were separated by a factor greater than 10 (Draper and Smith, 1998). All regression analyses were performed using R v2.8.1.
Results
Participant Characterization and Behavioral Results
Demographics for study participants appear in Table 1. Injury evaluations appear in Table 2, revealing that many of the brain injuries were remote (>5 years), and nearly half were severe. The outcome measures pre- and post-therapy for TBI participants appear in Table 3, along with the results of simple paired t-tests of posttest versus pretest scores. As a whole, participants with TBI demonstrated significant improvements for both semantic memory (HVLT; up to 2.6 points) and everyday memory function (RBMT; 2.1 points), and at both posttest 1 and 2 (i.e., immediate and delayed time points; see also O’Neil-Pirozzi et al. (2010).
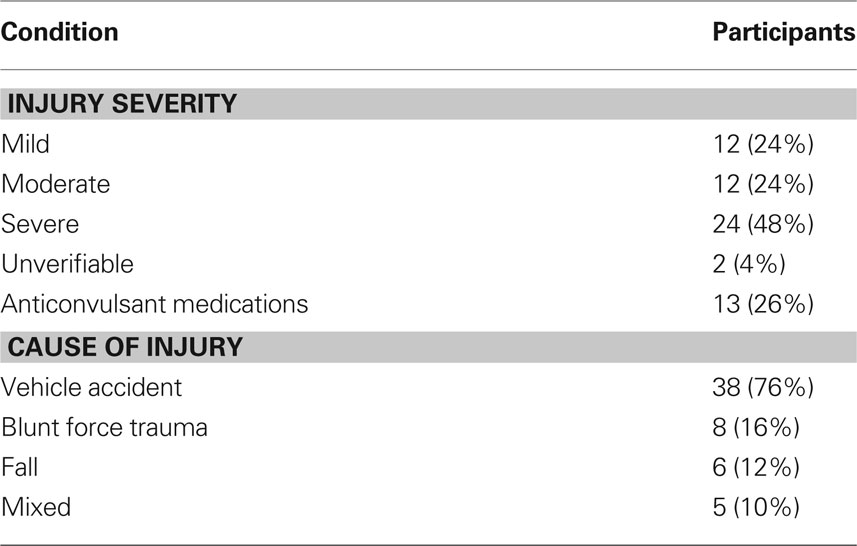
Table 2. Injury severity, patients taking anticonvulsant medications, and cause of injury information for participants with TBI.
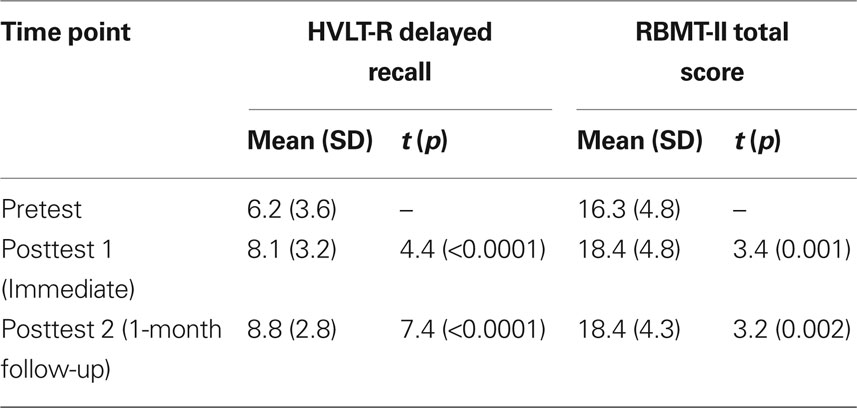
Table 3. Outcome scores for TBI participants (n = 50) measured at three time points, with statistical comparisons against pretest scores.
Morphometric Parcellation
The fully automated Freesurfer cortical reconstruction and parcellation process completed successfully for 50 participants. Even in cases of significant tissue degeneration (n = 7), the reconstruction process was able to identify all sulci and gyri, and provide reasonable parcellations and regional volumes. The results from a participant with a particularly large region of right temporal lobe encephalomalacia appear in Figure 2.
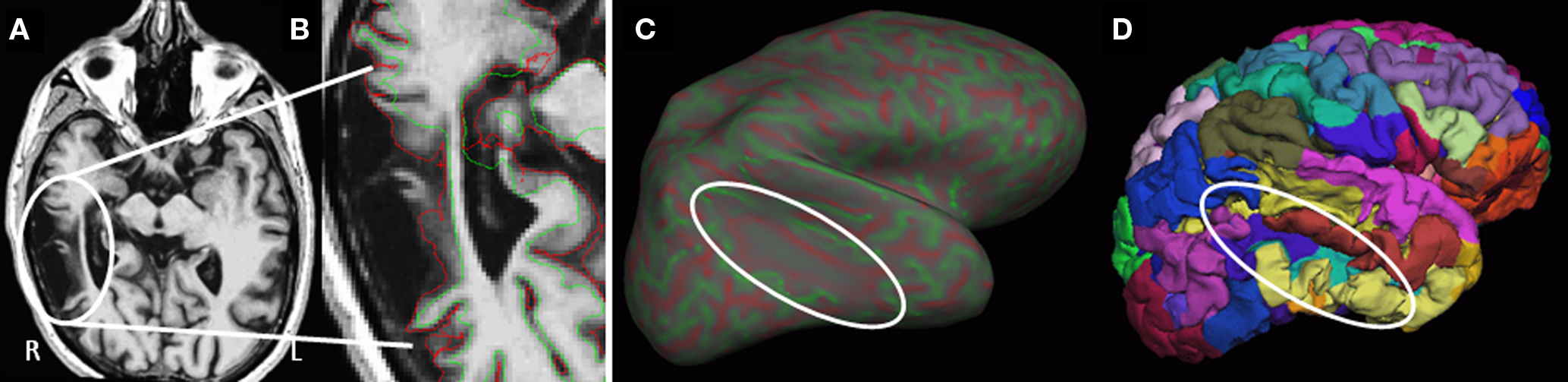
Figure 2. Example surface reconstruction in a case of substantial right temporal lobe encephalomalacia. (A) T1-weighted axial MRI image through the region of degeneration (oval). (B) Close up of T1 with overlay of the surfaces generated at the white-/gray-matter interface (green) and the pial surface (red). (C) Inflated right hemisphere showing curvature (reds = depth of sulci, greens = peak of gyri), with minimal curvature in the region of degeneration. (D) Re-folded surface with individual regional cortical labels (colors).
Prediction Results
From our a priori regions of interest, we found a significant positive relationship between hippocampal volume (pooling across left and right hemispheres) and HVLT outcome at posttest 2 (p = 0.007; cf. Table 4 and Figure 3A). The regression coefficient for hippocampal volume was 1.056 per 1 cm3 (1,000 mm3) of tissue, whereas that for the HVLT pretest score was 0.53; thus a 1-unit change in pretest was roughly equivalent to a 0.5 cm3 change in hippocampal volume in terms of predicting HVLT at posttest 2. Over the full range of hippocampal volumes (1.335–5.182 cm3), the hippocampal volume term predicted a range of 4.1 points on the HVLT, or 34% of the full-scale (12 point) range. For comparison, the HVLT pretest score predicted a range of 6.38 points, or 53% of the full-scale HVLT range. The hippocampal prediction model also included a significant effect of age, whereby older individuals had better rehabilitation outcomes (1.6 points on HVLT across the span of four decades), and marginal effects of injury severity (severities greater than mild tended towards an average 0.85 point decrease in HVLT at outcome). Other results from our six regions of interest with volume terms exhibiting p < 0.05 appear in Table 5. This included the mFG which exhibited modest predictive value for HVLT at posttest 2 (coefficient = 0.27 on a 7.633 cm3 range) as well as at posttest 1, and the supramarginal gyrus, which predicted both HVLT and RBMT outcomes, but only at posttest 1.
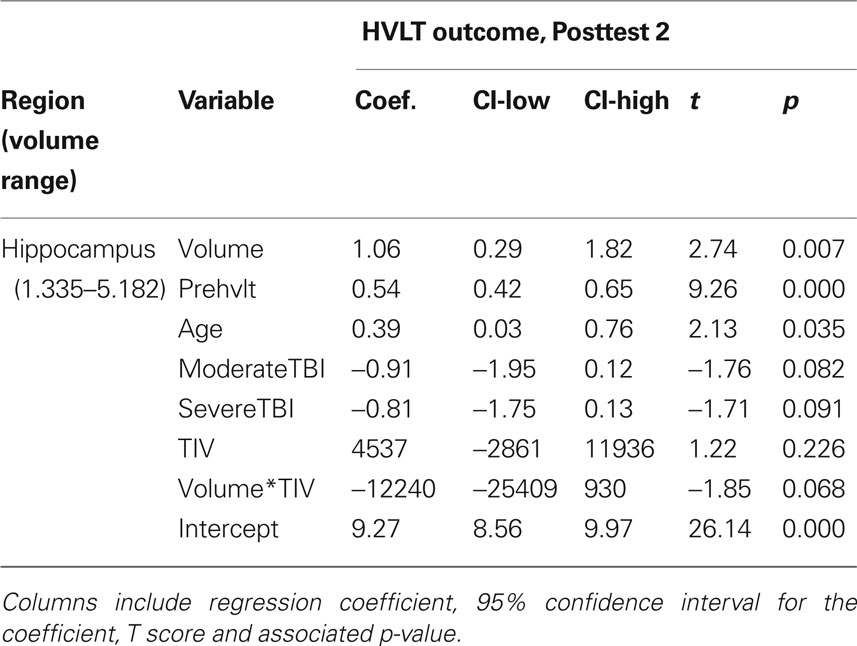
Table 4. All model terms for the primary prediction analysis, pooling across left and right hippocampal gray matter volume.
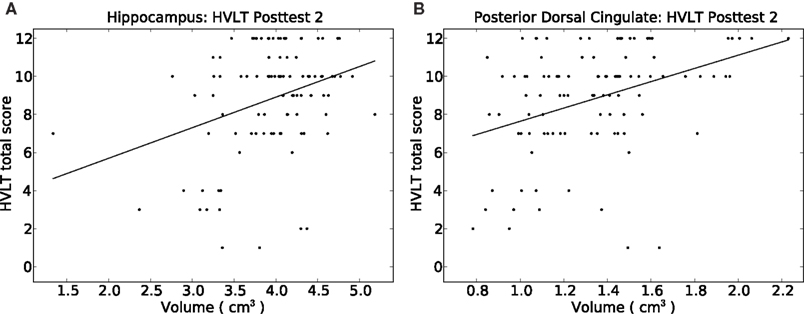
Figure 3. Depiction of the relationship between HVLT delayed correct recall score at posttest 2 versus the volume of (A) the hippocampus, and (B) the posterior dorsal cingulate gyrus. Simple least-squares lines are plotted; detailed regression results appear in Tables 4 and 5.
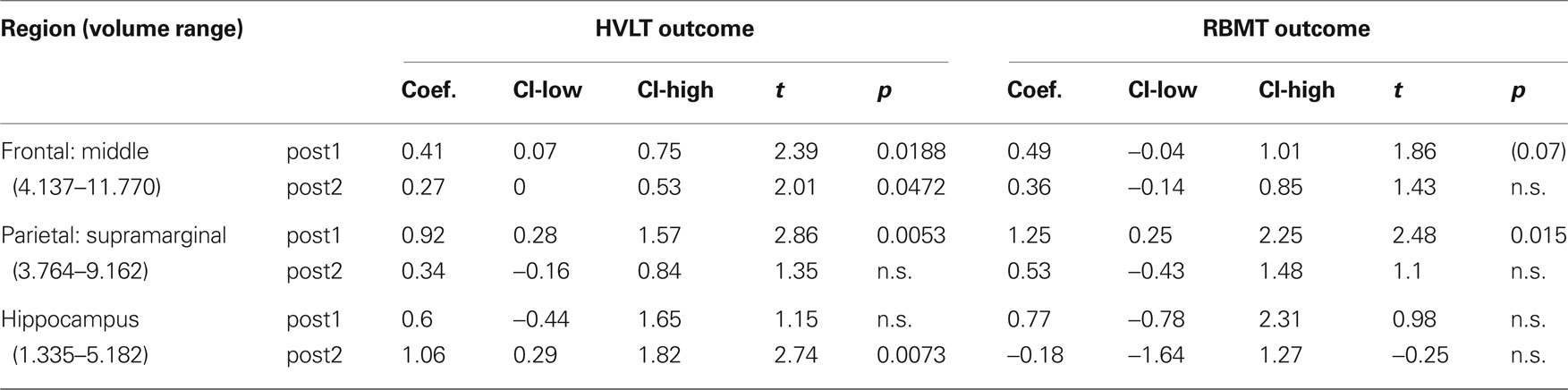
Table 5. Positive prediction regression results for the regional gray matter volume term in a priori regions of interest for all outcomes.
We next performed our exploratory analysis investigating the predictive value of all remaining brain regions generated by the Freesurfer parcellation (results in Table 6). The dorsal posterior cingulate cortex passed a strict Bonferroni correction (p < 0.0006) for predicting HVLT at posttest 2 (Figure 3B). The remaining entries in Table 6 are for those regions passing a p < 0.05 cutoff for at least one of the outcome measures. These identified regions, rather than being randomly distributed around the brain, fell into one of four geographic clusters. The first cluster was on the medial wall surrounding the corpus callosum, including four of five cingulate subregions and the subcallosal gyrus (immediately inferior to the anterior cingulate). The second cluster included the intraparietal sulcus and postcentral sulcus, which are immediately adjacent to our supramarginal and angular gyrus regions of interest. The third cluster included the occipital–temporal and medial lingual gyrus and sulcus, plus the inferior temporal sulcus, all on the inferior surface of the temporal lobe. The fourth cluster was in the diencephalon (thalamus and ventral diencephalon). A final region, the inferior frontal sulcus, was immediately adjacent to our two a priori VLPFC regions of interest. In essentially all regions, the regression coefficients were similar in magnitude to those discussed above, resulting in ranges of predicted outcome scores similar to those for outcome pretest scores. Only one region, the subcallosal gyrus, exhibited negative regression coefficients for RBMT – that is, increasing volume tended to predict poorer RBMT outcome scores.
The strongest findings in Table 6 appeared in the cingulate gyrus, including the anterior cingulate, anterior mid-cingulate, and dorsal posterior cingulate for HVLT, and the posterior mid-cingulate cortex (pMCC) for both HVLT and RBMT (see Table 6). The pMCC produced the strongest effect of all regions at posttest 2 for RBMT, the most functionally relevant outcome (Figure 4). With a regression coefficient estimate of 2.378 and a volume range of 2.568 cm3, this region had a prediction range of 6.1 points on the RBMT 24 point scale, compared to a prediction range of 6.7 points over the full observed range for RBMT pretest scores. As with all other models, the positive regression coefficient implies that larger volumes were associated with better outcome scores.
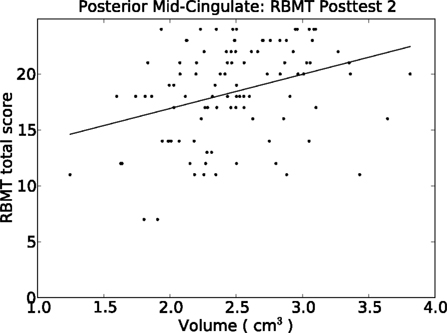
Figure 4. Relationship between our functionally relevant outcome (RBMT) 1-month post-therapy and the volume of the pMCC. A simple least-squares regression line is plotted. Results from the multiple regression model appears in Table 6.
Our test for laterality differences only revealed one significant effect, in the anterior cingulate region. In particular, for the HVLT outcome at posttest 1, the left anterior cingulate predicted an outcome score 1.3 points higher than the right (t = 2.73, p = 0.02).
Finally, our Cook’s distance check for outliers proved negative in all cases where significant relationships with volume were identified, indicating that no individual data points had substantial influence on the regression results. Each regression with a significant regional volume term was also checked for quadratic effects of age and regional volume. Two volume-squared terms were found significant: there were negative quadratic effects of volume in the thalamus and ventral diencephalon when predicting HVLT at posttest 2.
Discussion
Using a fully automated morphometric brain parcellation technique, we measured regional gray matter volumes in individuals with chronic TBI prior to their participation in a standardized memory rehabilitation program. We then identified brain regions for which the regional brain volume predicted post-therapy outcome. Substantial predictive value was found both for an outcome closely related to the rehabilitation training (i.e., HVLT), as well as for a more functionally relevant outcome (i.e., RBMT). Predictive regions included the hippocampus, the DLPFC and the PPC, which had been previously demonstrated as important to task performance and exhibited decreased activity in individuals with TBI versus controls (Strangman et al., 2009). In exploratory analysis, we also observed substantial predictive value from various subregions of the cingulate gyrus, areas adjacent to the predicted VLPFC and PPC regions, and the thalamus. Importantly, the magnitude of the regression coefficients for regional volumes rivaled those for pretest scores on the outcome variable – that is, knowledge of regional brain volumes tended to account for similar amounts of variance on the posttest score as did the pretest score. Finally, we found very little evidence for hemispheric specialization. Only in the anterior cingulate did we find modest support for the left hemisphere predicting significantly higher rehabilitation outcome scores than the right hemisphere.
Predicting Outcome from Memory Rehabilitation
Given the hippocampus’ well known role in learning and memory (Squire, 1992; Burgess et al., 2002), it is not surprising that the volume of the hippocampus exhibited a positive relationship with memory improvement following memory rehabilitation. This is also consistent with previous findings of degeneration in the medial temporal lobe following TBI (Ng et al., 2008), as well as the association between hippocampal damage and TBI outcome (Umile et al., 2002).
The findings in the mFG and supramarginal gyrus, as well as the regions adjacent to the supramarginal and angular gyri and in the VLPFC, are all regions previously identified as functionally important in HVLT-like tasks (Strangman et al., 2008b, 2009). This adds support to the notion that the volume of regions functionally relevant to task performance is important to rehabilitation outcomes. The same argument also extends to the cingulate regions identified in this study. While in previous work the cingulate did not exhibit a main effect difference in activation between controls and individuals with TBI, functional activation in this region held a key role in various interactions between population and task performance (Strangman et al., 2009).
While the location of identified regions was consistent with previous work, the large magnitude of the regression coefficients for regional volume was less expected. Prediction ranges afforded by regional volume often rivaled those of pretest scores, even after accounting for variables such as pretest, age, and injury severity. This strongly supports the notion that regional volumes can provide substantial added value for predicting rehabilitation outcome, at least in individuals one or more years post-injury. The generalizability of these findings to individuals less than a year post-injury remains to be investigated. However, we do note that structural MRI scans are commonly obtained on patients with TBI, such scans are the only data required for the Freesurfer parcellation programs, and our results involved no manual editing of the parcellation process, all of which support the clinical feasibility of investigating individuals closer to their date of injury.
The reason for the extent of the added value remains to be understood. The regional specificity and lack of significant predictive value for global measures such as all cerebral gray matter, argues strongly against a mass effect. It is likely, however, that the anatomical specificity provided by regional morphometric measurements, and/or the sensitivity of such measurements as compared to radiological reports (Bigler, 2001b), provides key information about the disruptions to functional brain networks. Such information is presumably lost when simply considering, for example, overall injury severity or total lesion load. Other imaging modalities that provide detailed anatomical information about individual injuries, such as diffusion tensor imaging (Wilde et al., 2006), may prove equally or more useful in predicting outcomes from cognitive rehabilitation programs, or may complement morphological measures such as those used here. Regardless of imaging modality, we speculate that optimizations can still be made in terms of the level of anatomical detail considered. Our morphological measures lie in a middle ground between gross pathology and voxel-wise assessments. It is not yet clear which level of analysis may be optimally effective at predicting TBI rehabilitation outcomes.
One particularly interesting finding from the exploratory analysis was in the pMCC, which exhibited substantial predictive capability for the more functionally relevant RBMT outcome at 1-month post-therapy. The RBMT is comprised of a number of quite disparate memory tasks, each of which has considerable ecological validity (e.g., remembering where a personal item is, remembering to ask an appointment-related question, and remembering to deliver a message). While the prefrontal cortex in general is known to be involved in working memory (Hillary et al., 2006), long-term episodic memory (Ranganath et al., 2003), and executive control (Messinger et al., 2009; Rossi et al., 2009), it is still unexpected that any one brain area might be particularly important for successful performance across the broad range of RBMT tasks. While predicting RBMT at 1-month post-therapy is of high clinical importance, we nevertheless anticipated only modest success in predicting functionally relevant outcomes such as RBMT, particularly a month post-intervention. Identification the pMCC as having substantial predictive range for this outcome was thus quite promising. It provides evidence in favor of predicting outcome not just for laboratory tasks like the HVLT, but also for more ecologically valid outcomes. It further provides guidance on what brain regions and networks to focus on.
Unlike more anterior portions of the cingulate cortex, relatively little is known about the pMCC and its involvement in cognitive processing. However, the pMCC is known to interact with the PPC (Vogt, 2005), a key area for multimodal integration. It was also recently found to be functionally linked with various medial temporal lobe regions as well as the insular cortex (Taylor et al., 2009). One hypothesized role is orienting the individual to sensory events (Vogt, 2005). Collectively, the evidence suggests that pMCC interacts with areas involved in multimodal integration and declarative and autobiographical memory processing. It is therefore plausible that the pMCC is a key component of a functional network involving stimulus orientation, integration, and transfer to memory. If sufficiently general purpose, such a network would be expected to have predictive value for specific outcomes (e.g., HVLT) as well as more general outcomes (e.g., RBMT), as we observed. It remains to be determined whether this posited integrative role of the pMCC is specific to memory rehabilitation or extends in some way to the rehabilitation of attentional or executive functioning following TBI.
Finally, previous TBI studies have demonstrated negative relationships between age and rehabilitation outcome. In addition, a number of researchers have suggested that TBI may have a particularly negative effect on older brains (Rapoport and Feinstein, 2000; Bigler, 2001a; Marquez de la Plata et al., 2008), implying that age at time of injury may be an important variable in rehabilitation outcome. In this study, we found age to be positively related to rehabilitation outcome when predicting HVLT from the volume of the hippocampus. This positive relationship with age held when controlling for age at time of injury, and continued to hold in supplementary analyses where we excluded all volume terms, and even when predicting HVLT outcome solely from age. Thus, we had no evidence of a negative relationship between age and outcome. While these findings contradict previous results, we note that (1) our therapy was atypical relative to other studies in that it focused exclusively on internal strategies for memory improvement, and (2) the coefficient on age was quite modest at approximately 0.4 points on HVLT per decade, meaning our oldest participant was predicted to score 1.6 points higher than our youngest, across our 40 year participant age range. Thus, while significant and positive, the effect of age was substantially less than half the effect contributed by volume, and in a range below what would typically be considered a clinically meaningful change.
Limitations
Our study included individuals with a wide range of injury severities. Thus, there may have been differential responses from mild or severe cases that did not correspond to the “average” prediction coefficients we observed, or the group as a whole may have been skewed by one particular subpopulation. However, we consider our severity range to be a strength of the study: among individuals post-TBI who present with memory difficulties, the ability to predict memory rehabilitation outcome remained strong in spite of such heterogeneity, and hence the findings may apply to a relatively broad clinical population. Similarly, although the MRI scans were all chronic and were collected no sooner than 1-year post-injury, the time since injury and rehabilitation histories differed widely across participants. This additional variability almost certainly contributed to reduced (rather than inflated) prediction sensitivity. In addition, our approach may be less sensitive if applied exclusively in mild TBI where neurostructural changes may be less evident, or exclusively in severe TBI where MRI may be contraindicated. It remains to be determined whether our approach would be suitable for individuals who sustained a TBI less than 1 year prior, particularly given the variability of injury evolution in the first few months immediately post-injury.
We recognize that automated approaches may contain segmentation or parcellation errors, may be fooled by hemorrhagic lesions, and may miss subtle variations or complexities that can be more appropriately handled by an expert neuroanatomist. For a relatively large study such as this, we believe the benefits of automation actually make not only the study, but potential clinical translation of the approach, realistically feasible. Related, it is difficult to judge volumetric accuracy in face of encephalomalacia or other gross structural injury. Scar tissue may lead to over-estimation of gray matter volume, white matter may degenerate sufficiently to be classified as gray matter, or a major loss of tissue may result in unrecoverable segmentation failures. For this study, we assumed that such effects contribute to the noise in our data and prediction models. Finally, there are various alternatives to compensate for TIV, a common approach being to divide all regional volumes in an individual by their TIV. While the best approach is debated, the divisor approach is has certain statistical shortcomings (Kronmal, 1993), and hence we utilized an interaction approach to help control for differing brain volumes.
Conclusions
We identified a number of specific regions that provided substantial predictive value for memory rehabilitation outcomes. While this represents only an early stage in predicting outcomes from specific cognitive rehabilitation programs, and is specific to just one such program, our work suggests that the approach is viable, and provides a benchmark with which to compare other neuroimaging prediction approaches. Since our study was conducted at the chronic stage (all participants being more than 1-year post-injury), further work needs to be done to determine if more acute imaging provides equivalent, better, or worse predictive value. If the prediction of cognitive rehabilitation outcomes can be similarly achieved with other interventions, structural neuroimaging could eventually become a tool to help individuals with TBI and their caregivers more objectively select among candidate rehabilitation programs based on post injury structural scans. Based on our prior work, we further anticipate that combining results from multiple imaging modalities – for example, T1-, T2-, hemosiderin-, and diffusion-weighted MRI, spectroscopy, resting functional MRI and task-related functional MRI – may help achieve even greater sensitivity, specificity and predictive value. Such an approach must always balance the costs and benefits of scanning and analysis relative to the therapy. However, for expensive and human-intensive efforts such as TBI rehabilitation, neuroimaging may provide a reliable method for better targeting and customizing TBI rehabilitation therapies.
Conflict of Interest Statement
The authors declare that the research was conducted in the absence of any commercial or financial relationships that could be construed as a potential conflict of interest.
Acknowledgments
We would like to thank Dana Christina, Kalika Kelkar, the Statewide Head Injury Program, and Community Rehabilitation Care for their help and support. The contents of this publication were developed under a grant from the National Institute on Disability and Rehabilitation Research, Department of Education (H133A020513). However, those contents do not necessarily represent the policy of the Department of Education, and you should not assume endorsement by the Federal Government. Funding for this project also came from the NIH (NINDS: K25-NS046554). Supported by National Institute on Disability and Rehabilitation Research (grant no. H133A020513), and the National Institute of Neurological Disorders and Stroke (grant no. K25-NS046554).
References
Ariza, M., Serra-Grabulosa, J. M., Junque, C., Ramirez, B., Mataro, M., Poca, A., Bargallo, N., and Sahuquillo, J. (2006). Hippocampal head atrophy after traumatic brain injury. Neuropsychologia 44, 1956–1961.
Azouvi, P. (2000). Neuroimaging correlates of cognitive and functional outcome after traumatic brain injury. Curr. Opin. Neurol. 13, 665–669.
Bigler, E. D. (2001a). Distinguished Neuropsychologist Award Lecture 1999. The lesion(s) in traumatic brain injury: implications for clinical neuropsychology. Arch. Clin. Neuropsychol. 16, 95–131.
Bigler, E. D. (2001b). Quantitative magnetic resonance imaging in traumatic brain injury. J. Head Trauma Rehabil. 16, 117–134.
Bigler, E. D., Abildskov, T. J., Wilde, E. A., McCauley, S. R., Li, X., Merkley, T. L., Fearing, M. A., Newsome, M. R., Scheibel, R. S., Hunter, J. V., Chu, Z., and Levin, H. S. (2010). Diffuse damage in pediatric traumatic brain injury: a comparison of automated versus operator-controlled quantification methods. Neuroimage 50, 1017–1026.
Burgess, N., Maguire, E. A., and O’Keefe, J. (2002). The human hippocampus and spatial and episodic memory. Neuron 35, 625–641.
Chen, K., Reiman, E. M., Alexander, G. E., Bandy, D., Renaut, R., Crum, W. R., Fox, N. C., and Rossor, M. N. (2004). An automated algorithm for the computation of brain volume change from sequential MRIs using an iterative principal component analysis and its evaluation for the assessment of whole-brain atrophy rates in patients with probable Alzheimer’s disease. Neuroimage 22, 134–143.
Cicerone, K., Levin, H., Malec, J., Stuss, D., and Whyte, J. (2006). Cognitive rehabilitation interventions for executive function: moving from bench to bedside in patients with traumatic brain injury. J. Cogn. Neurosci. 18, 1212–1222.
Cicerone, K. D., Dahlberg, C., Kalmar, K., Langenbahn, D. M., Malec, J. F., Bergquist, T. F., Felicetti, T., Giacino, J. T., Harley, J. P., Harrington, D. E., Herzog, J., Kneipp, S., Laatsch, L., and Morse, P. A. (2000). Evidence-based cognitive rehabilitation: recommendations for clinical practice. Arch. Phys. Med. Rehabil. 81, 1596–1615.
Cicerone, K. D., Mott, T., Azulay, J., and Friel, J. C. (2004). Community integration and satisfaction with functioning after intensive cognitive rehabilitation for traumatic brain injury. Arch. Phys. Med. Rehabil. 85, 943–950.
Desikan, R. S., Cabral, H. J., Hess, C. P., Dillon, W. P., Glastonbury, C. M., Weiner, M. W., Schmansky, N. J., Greve, D. N., Salat, D. H., Buckner, R. L., and Fischl, B. (2009). Automated MRI measures identify individuals with mild cognitive impairment and Alzheimer’s disease. Brain 132, 2048–2057.
Desikan, R. S., Segonne, F., Fischl, B., Quinn, B. T., Dickerson, B. C., Blacker, D., Buckner, R. L., Dale, A. M., Maguire, R. P., Hyman, B. T., Albert, M. S., and Killiany, R. J. (2006). An automated labeling system for subdividing the human cerebral cortex on MRI scans into gyral based regions of interest. Neuroimage 31, 968–980.
Destrieux, C., Fischl, B., Dale, A., and Halgren, E. (2010). Automatic parcellation of human cortical gyri and sulci using standard anatomical nomenclature. Neuroimage 53, 1–15.
Dickerson, B. C., Bakkour, A., Salat, D. H., Feczko, E., Pacheco, J., Greve, D. N., Grodstein, F., Wright, C. I., Blacker, D., Rosas, H. D., Sperling, R. A., Atri, A., Growdon, J. H., Hyman, B. T., Morris, J. C., Fischl, B., and Buckner, R. L. (2009). The cortical signature of Alzheimer’s disease: regionally specific cortical thinning relates to symptom severity in very mild to mild AD dementia and is detectable in asymptomatic amyloid-positive individuals. Cereb. Cortex 19, 497–510.
Dikmen, S. S., Machamer, J. E., Powell, J. M., and Temkin, N. R. (2003). Outcome 3 to 5 years after moderate to severe traumatic brain injury. Arch. Phys. Med. Rehabil. 84, 1449–1457.
Draper, N. R., and Smith, H. (1998). Applied Regression Analysis. New York, NY: John Wiley & Sons, Inc.
Fischl, B., Salat, D. H., Busa, E., Albert, M., Dieterich, M., Haselgrove, C., van der Kouwe, A., Killiany, R., Kennedy, D., Klaveness, S., Montillo, A., Makris, N., Rosen, B., and Dale, A. M. (2002). Whole brain segmentation: automated labeling of neuroanatomical structures in the human brain. Neuron 33, 341–355.
Fischl, B., Sereno, M. I., and Dale, A. M. (1999). Cortical surface-based analysis. II: Inflation, flattening, and a surface-based coordinate system. Neuroimage 9, 195–207.
Fischl, B., van der Kouwe, A., Destrieux, C., Halgren, E., Segonne, F., Salat, D. H., Busa, E., Seidman, L. J., Goldstein, J., Kennedy, D., Caviness, V., Makris, N., Rosen, B., and Dale, A. M. (2004). Automatically parcellating the human cerebral cortex. Cereb. Cortex 14, 11–22.
Fjell, A. M., Westlye, L. T., Amlien, I., Espeseth, T., Reinvang, I., Raz, N., Agartz, I., Salat, D. H., Greve, D. N., Fischl, B., Dale, A. M., and Walhovd, K. B. (2009). High consistency of regional cortical thinning in aging across multiple samples. Cereb. Cortex 19, 2001–2012.
Gale, S. D., Baxter, L., Roundy, N., and Johnson, S. C. (2005). Traumatic brain injury and grey matter concentration: a preliminary voxel based morphometry study. J. Neurol. Neurosurg. Psychiatr. 76, 984–988.
Giles, G. M. (2001). “The effectiveness of neurorehabilitation,” in Neurobehavioral Disability and Social Handicap Following Traumatic Brain Injury, eds R. L. Wood and T. M. McMillan (Hove, England: Psychology Press), 231–255.
Han, X., and Fischl, B. (2007). Atlas renormalization for improved brain MR image segmentation across scanner platforms. IEEE Trans. Med. Imaging 26, 479–486.
Hillary, F. G., Genova, H. M., Chiaravalloti, N. D., Rypma, B., and DeLuca, J. (2006). Prefrontal modulation of working memory performance in brain injury and disease. Hum. Brain Mapp. 27, 837–847.
Jorge, R. E., Acion, L., Starkstein, S. E., and Magnotta, V. (2007). Hippocampal volume and mood disorders after traumatic brain injury. Biol. Psychiatry 62, 332–338.
Jovicich, J., Czanner, S., Han, X., Salat, D., van der Kouwe, A., Quinn, B., Pacheco, J., Albert, M., Killiany, R., Blacker, D., Maguire, P., Rosas, D., Makris, N., Gollub, R., Dale, A., Dickerson, B. C., and Fischl, B. (2009). MRI-derived measurements of human subcortical, ventricular and intracranial brain volumes: reliability effects of scan sessions, acquisition sequences, data analyses, scanner upgrade, scanner vendors and field strengths. Neuroimage 46, 177–192.
Katz, D. I., and Alexander, M. P. (1994). Traumatic brain injury. Predicting course of recovery and outcome for patients admitted to rehabilitation. Arch. Neurol. 51, 661–670.
Kennedy, D. N., Filipek, P. A., and Caviness, V. R. (1989). Anatomic segmentation and volumetric calculations in nuclear magnetic resonance imaging. IEEE Trans. Med. Imaging 8, 1–7.
Kim, Y. H., Yoo, W. K., Ko, M. H., Park, C. H., Kim, S. T., and Na, D. L. (2009). Plasticity of the attentional network after brain injury and cognitive rehabilitation. Neurorehabil. Neural Repair 23, 468–477.
Kraus, M. F., Susmaras, T., Caughlin, B. P., Walker, C. J., Sweeney, J. A., and Little, D. M. (2007). White matter integrity and cognition in chronic traumatic brain injury: a diffusion tensor imaging study. Brain 130, 2508–2519.
Kronmal, R. A. (1993). Spurious correlation and the fallacy of the ration standard revisited. J. R. Stat. Soc Ser. A 156, 379–392.
Levine, B., Kovacevic, N., Nica, E. I., Cheung, G., Gao, F., Schwartz, M. L., and Black, S. E. (2008). The Toronto traumatic brain injury study: injury severity and quantified MRI. Neurology 70, 771–778.
Logan, J. M., Sanders, A. L., Snyder, A. Z., Morris, J. C., and Buckner, R. L. (2002). Under-recruitment and nonselective recruitment: dissociable neural mechanisms associated with aging. Neuron 33, 827–840.
Marquez de la Plata, C. D., Hart, T., Hammond, F. M., Frol, A. B., Hudak, A., Harper, C. R., O’Neil-Pirozzi, T. M., Whyte, J., Carlile, M., and Diaz-Arrastia, R. (2008). Impact of age on long-term recovery from traumatic brain injury. Arch. Phys. Med. Rehabil. 89, 896–903.
McAllister, T. W., Flashman, L. A., McDonald, B. C., and Saykin, A. J. (2006). Mechanisms of working memory dysfunction after mild and moderate TBI: evidence from functional MRI and neurogenetics. J. Neurotrauma 23, 1450–1467.
McAllister, T. W., Sparling, M. B., Flashman, L. A., and Saykin, A. J. (2001). Neuroimaging findings in mild traumatic brain injury. J. Clin. Exp. Neuropsychol. 23, 775–791.
McCauley, S. R., Wilde, E. A., Merkley, T. L., Schnelle, K. P., Bigler, E. D., Hunter, J. V., Chu, Z., Vasquez, A. C., and Levin, H. S. (2010). Patterns of cortical thinning in relation to event-based prospective memory performance three months after moderate to severe traumatic brain injury in children. Dev. Neuropsychol. 35, 318–332.
Mega, M. S., Dinov, I. D., Mazziotta, J. C., Manese, M., Thompson, P. M., Lindshield, C., Moussai, J., Tran, N., Olsen, K., Zoumalan, C. I., Woods, R. P., and Toga, A. W. (2005). Automated brain tissue assessment in the elderly and demented population: construction and validation of a sub-volume probabilistic brain atlas. Neuroimage 26, 1009–1018.
Merkley, T. L., Bigler, E. D., Wilde, E. A., McCauley, S. R., Hunter, J. V., and Levin, H. S. (2008). Diffuse changes in cortical thickness in pediatric moderate-to-severe traumatic brain injury. J. Neurotrauma 25, 1343–1345.
Messinger, A., Lebedev, M. A., Kralik, J. D., and Wise, S. P. (2009). Multitasking of attention and memory functions in the primate prefrontal cortex. J. Neurosci. 29, 5640–5653.
Ng, K., Mikulis, D. J., Glazer, J., Kabani, N., Till, C., Greenberg, G., Thompson, A., Lazinski, D., Agid, R., Colella, B., and Green, R. E. (2008). Magnetic resonance imaging evidence of progression of subacute brain atrophy in moderate to severe traumatic brain injury. Arch. Phys. Med. Rehabil. 89, S35–S44.
O’Neil-Pirozzi, T. M., Strangman, G. E., Goldstein, R., Katz, D. I., Savage, C. R., Kelkar, K., Supelana, C., Burke, D., Rauch, S. L., and Glenn, M. B. (2010). A controlled treatment study of internal memory strategies (I-MEMS) following traumatic brain injury. J. Head Trauma Rehabil. 25, 43–51.
Oldfield, R. (1971). The assessment and analysis of handedness. The Edinburgh inventory. Neuropsychologia 9, 97–113.
Povlishock, J. T., and Katz, D. I. (2005). Update of neuropathology and neurological recovery after traumatic brain injury. J. Head Trauma Rehabil. 20, 76–94.
Ranganath, C., Johnson, M. K., and D’Esposito, M. (2003). Prefrontal activity associated with working memory and episodic long-term memory. Neuropsychologia 41, 378–389.
Rapoport, M. J., and Feinstein, A. (2000). Outcome following traumatic brain injury in the elderly: a critical review. Brain Inj. 14, 749–761.
Rossi, A. F., Pessoa, L., Desimone, R., and Ungerleider, L. G. (2009). The prefrontal cortex and the executive control of attention. Exp. Brain Res. 192, 489–497.
Ruff, R. M., Marshall, L. F., Crouch, J., Klauber, M. R., Levin, H. S., Barth, J., Kreutzer, J., Blunt, B. A., Foulkes, M. A., Eisenberg, H. M., Janeh, J. A., and Marmaroufand, A. (1993). Predictors of outcome following severe head trauma: follow-up data from the traumatic coma data bank. Brain Inj. 7, 101–111.
Salat, D. H., Buckner, R. L., Snyder, A. Z., Greve, D. N., Desikan, R. S., Busa, E., Morris, J. C., Dale, A. M., and Fischl, B. (2004). Thinning of the cerebral cortex in aging. Cereb. Cortex 14, 721–730.
Salmond, C. H., Chatfield, D. A., Menon, D. K., Pickard, J. D., and Sahakian, B. J. (2005). Cognitive sequelae of head injury: involvement of basal forebrain and associated structures. Brain 128, 189–200.
Schmansky, N. (2010). Destrieux Atlas Changes. http://surfer.nmr.mgh.harvard.edu/fswiki/DestrieuxAtlasChanges.
Squire, L. R. (1992). Memory and the hippocampus: a synthesis from findings with rats, monkeys and humans. Psychol. Rev. 99, 195–231.
Strangman, G. E., Goldstein, R., O’Neil-Pirozzi, T. M., Kelkar, K., Supelana, C., Burke, D., Katz, D. I., Rauch, S. L., Savage, C. R., and Glenn, M. B. (2008a). Neurophysiological alterations during strategy-based verbal learning in traumatic brain injury. Neurorehabil. Neural Repair. 23, 226–236.
Strangman, G. E., O’Neil-Pirozzi, T. M., Goldstein, R., Kelkar, K., Katz, D. I., Burke, D., Rauch, S. L., Savage, C. R., and Glenn, M. B. (2008b). Prediction of memory rehabilitation outcomes in traumatic brain injury by using functional magnetic resonance imaging. Arch. Phys. Med. Rehabil. 89, 974–981.
Strangman, G. E., Goldstein, R., O’Neil-Pirozzi, T. M., Kelkar, K., Supelana, C., Burke, D., Katz, D. I., Rauch, S. L., Savage, C. R., and Glenn, M. B. (2009). Neurophysiological alterations during strategy-based verbal learning in traumatic brain injury. Neurorehabil. Neural Repair 23, 226–236.
Taylor, K. S., Seminowicz, D. A., and Davis, K. D. (2009). Two systems of resting state connectivity between the insula and cingulate cortex. Hum. Brain Mapp. 30, 2731–2745.
Temkin, N. R., Holubkov, R., Machamer, J. E., Winn, H. R., and Dikmen, S. S. (1995). Classification and regression trees (CART) for prediction of function at 1 year following head trauma. J. Neurosurg. 82, 764–771.
Umile, E. M., Sandel, M. E., Alavi, A., Terry, C. M., and Plotkin, R. C. (2002). Dynamic imaging in mild traumatic brain injury: support for the theory of medial temporal vulnerability. Arch. Phys. Med. Rehabil. 83, 1506–1513.
Vogt, B. A. (2005). Pain and emotion interactions in subregions of the cingulate gyrus. Nat. Rev. Neurosci. 6, 533–544.
Wilde, E. A., Chu, Z., Bigler, E. D., Hunter, J. V., Fearing, M. A., Hanten, G., Newsome, M. R., Scheibel, R. S., Li, X., and Levin, H. S. (2006). Diffusion tensor imaging in the corpus callosum in children after moderate to severe traumatic brain injury. J. Neurotrauma 23, 1412–1426.
Wilde, E. A., Hunter, J. V., Newsome, M. R., Scheibel, R. S., Bigler, E. D., Johnson, J. L., Fearing, M. A., Cleavinger, H. B., Li, X., Swank, P. R., Pedroza, C., Roberson, G. S., Bachevalier, J., and Levin, H. S. (2005). Frontal and temporal morphometric findings on MRI in children after moderate to severe traumatic brain injury. J. Neurotrauma 22, 333–344.
Keywords: cognitive rehabilitation, memory, structural neuroimaging, behavioral neurology, morphometrics, semantic clustering, brain trauma
Citation: Strangman GE, O’Neil-Pirozzi TM, Supelana C, Goldstein R, Katz DI and Glenn MB (2010) Regional brain morphometry predicts memory rehabilitation outcome after traumatic brain injury. Front. Hum. Neurosci. 4:182. doi:10.3389/fnhum.2010.00182
Received: 02 April 2010;
Accepted: 07 September 2010;
Published online: 14 October 2010.
Edited by:
Donald T. Stuss, Baycrest Centre for Geriatric Care, CanadaReviewed by:
Elisabeth Wilde, Baylor College of Medicine, USAErin Bigler, Brigham Young University, USA; University of Utah, USA
Copyright: © 2010 Strangman, O’Neil-Pirozzi, Supelana, Goldstein, Katz and Glenn. This is an open-access article subject to an exclusive license agreement between the authors and the Frontiers Research Foundation, which permits unrestricted use, distribution, and reproduction in any medium, provided the original authors and source are credited.
*Correspondence: Gary E. Strangman, Neural Systems Group, Department of Psychiatry, 149 13th St. Suite 2651, Charlestown, MA 02129, USA. e-mail:c3RyYW5nQG5tci5tZ2guaGFydmFyZC5lZHU=