- 1 Wellcome Trust Centre for Neuroimaging, University College London, London, UK
- 2 Laboratory for Cognitive and Computational Neuroscience, Centre for Biomedical Technology, Technical University of Madrid and Complutense University of Madrid, Madrid, Spain
- 3 Trinity Centre for BioEngineering, School of Engineering and School of Medicine, Trinity College, College Green, Dublin, Ireland
Theta oscillations in the local field potential of neural ensembles are considered key mediators of human working memory. Theoretical accounts arising from animal hippocampal recordings propose that the phase of theta oscillations serves to instantiate sequential neuronal firing to form discrete representations of items held online. Human evidence of phase relationships in visual working memory has enhanced this theory, implicating long theta cycles in supporting greater memory capacity. Here we use human magnetoencephalographic recordings to examine a novel, alternative principle of theta functionality. The principle we hypothesize is derived from information theory and predicts that rather than long (low frequency) theta cycles, short (high frequency) theta cycles are best suited to support high information capacity. From oscillatory activity recorded during the maintenance period of a visual working memory task we show that a network of brain regions displays an increase in peak 4–12 Hz frequency with increasing memory load. Source localization techniques reveal that this network comprises bilateral prefrontal and right parietal cortices. Further, the peak of oscillation along this theta–alpha frequency axis is significantly higher in high capacity individuals compared to low capacity individuals. Importantly while we observe the adherence of cortical neuronal oscillations to our novel principle of theta functioning, we also observe the traditional inverse effect of low frequency theta maintaining high loads, where critically this was located in medial temporal regions suggesting parallel, dissociable hippocampal-centric, and prefrontal-centric theta mechanisms.
Introduction
Working memory (WM) is considered an essential cognitive resource, enabling the encoding, maintenance, and manipulation of information during short periods of time, thus facilitating action of current goals. It is widely assumed that WM is a supporting process for high level cognitive abilities. Despite its crucial role across a range of processes, studies of visual WM (VWM) in humans demonstrate a surprisingly restricted capacity to retain information from a visual scene, with people able to store only around three items of information (Luck and Vogel, 1997; Cowan, 2001). Neuroimaging studies of VWM have elaborated the neuroanatomical correlates of this capacity bottleneck. Functional magnetic resonance imaging (fMRI) studies demonstrate activity during VWM maintenance that saturates once capacity is reached in posterior parietal (Linden et al., 2003; Todd and Marois, 2004) and prefrontal cortices (Barch et al., 1997; Callicott et al., 1999), while inter-individual differences in memory capacity have been shown to correlate with signal changes in frontal regions and the basal ganglia (McNab and Klingberg, 2008). Electrophysiological studies of evoked brain responses similarly demonstrate modulation by memory load that covaries with inter-individual differences in capacity, over posterior parietal and occipital sites (Vogel and Machizawa, 2004; Vogel et al., 2005; Ikkai et al., 2010).
New evidence suggests that the oscillatory nature of electrophysiological signals in some of these regions is a key factor in determining WM performance. While invasive and non-invasive recordings of electrical and magnetic signals in humans have shown changes across theta (4–8 Hz), alpha (8–16 Hz), beta (16–30 Hz), and gamma (30–60 Hz) frequencies during the retention period of WM tasks (Tallon-Baudry et al., 1998; Raghavachari et al., 2001; Onton et al., 2005; Deiber et al., 2007; Jokisch and Jensen, 2007; Palva and Palva, 2007; Meltzer et al., 2008; Grimault et al., 2009), the actual role of each frequency in representing the contents of WM remains unclear. The theta band in particular has been identified as a crucial component of WM related activity. In this band, both power and duration have been related to increases in the amount of information to be retained, with theta modulations localized to frontal (Gevins et al., 1997; Jensen and Tesche, 2002), hippocampal (Tesche and Karhu, 2000), and parietal (Sarnthein et al., 1998) regions. More recently mechanistic accounts of the theta signal have been tested. These studies are derived from a theoretical model of hippocampal function where theta oscillations entrain gamma bursts at a particular phase in its cycle (Lisman and Idiart, 1995; Jensen and Lisman, 1996, 1998; Lisman, 2005), with each gamma burst representing a sequential item held online. In humans, heightened theta–gamma phase synchronization in parietal cortex is associated with successful maintenance of information (Sauseng et al., 2009), while physiological recordings in behaving animals have shown that prefrontal firing entrainment by hippocampal theta is associated with correct WM responses (Hyman et al., 2010). Importantly, this model (Lisman and Idiart, 1995) makes specific predictions about the frequency of theta that likely enhances performance, namely that low frequency theta oscillations subserve greater WM capacity. Providing empirical support for this model, human intracranial hippocampal data demonstrate that a greater memory load results in an increased average theta period, leading to lower frequency oscillations (Axmacher et al., 2010).
Here, we test an alternative oscillatory coding scheme in the brain, and examine in particular the inter-individual differences in this coding scheme and how this relates to performance. Appealing to the fundaments of information theory, we propose, in contrast to the above theory (Lisman and Idiart, 1995) that higher frequency theta oscillations mediate retention during periods of high memory load. The theoretical underpinnings are derived from communication theory (Shannon, 1948) where bandwidth, the range (band) of frequencies over which information can be encoded, serves as the resource most valuable for the transmission of information. In the case of the theta rhythm, considered to occupy the 4–8 Hz range, information theory predicts that the capacity of information transfer could be higher at a bandwidth where the peak frequency is 8 than say at 6 Hz. An initial examination of the task-related frequency structure of our data revealed both theta and alpha activity. We therefore test specifically for individual differences in peak high theta, low alpha frequencies, and associated bandwidth, to determine whether these parameters operate as a limiting factor engendering capacity differences among subjects. While we will consider localized oscillatory patterns rather than transmission per se, we draw an analogy with the reverberant circuit dynamics observed in animals during memory maintenance (Goldman-Rakic, 1995) and examine 4–12 Hz responses within these networks. Theta rhythms in such circuits could operate with low frequency theta in a “narrowband” regime where, for example, frequencies from 4 to 7 Hz support item retention, or, alternatively, they could form “wideband” limited signals with notably higher frequency spectral components, recruiting higher frequency bands.
We applied magnetoencephalography (MEG) to test whether the properties of human theta/alpha frequency during a VWM retention period conform to these principles. We recorded MEG signals while subjects performed a VWM task (Vogel and Machizawa, 2004) with a parametric load manipulation. This change-detection paradigm has been previously used to investigate event-related potentials (ERPs) during item retention (Vogel and Machizawa, 2004). In particular, this task has been used to probe hemisphere specific responses to bilateral stimulus arrays of which one is attended and the other ignored. Contralateral delay activity (CDA) is an evoked response contralateral to the attended hemifield which has been shown to increase in amplitude with increasing item load (Fukuda et al., 2010). Similarly, at contralateral sites, theta frequency measures such as theta–gamma coupling have been shown to increase with load (Sauseng et al., 2009), while alpha frequencies thought to represent unattended stimulus suppression have been shown to increase over ipsilateral regions (Jokisch and Jensen, 2007; Sauseng et al., 2009). We tested whether humans efficiently employ a circuit mediating both theta and alpha oscillations, recruiting low frequency signals (∼4 Hz) when retaining few items in memory, and higher frequency signals (∼10 Hz) for higher retention requirements. Moreover, this approach enabled us to examine whether inter-individual differences in capacity can be explained by different operant theta/alpha frequencies at high VWM load.
Materials and Methods
Subjects
Fourteen healthy, right-handed, native Spanish speaking subjects took part in our study (age 24–32; mean age 26.9, SD = 3.3). All subjects were free from neurological and psychiatric history. Ethical approval was obtained from the local ethics committee.
Task
During each trial, subjects were presented with a fixation cross (350 ± 50 ms) followed by an arrow (250 ± 50 ms) indicating the hemifield (left/right) to be attended. A target stimulus of colored square arrays was then presented within two rectangular regions that were centered to the left and right on a black background. These memory arrays consisted of 2, 3, 4, or 6 colored squares (2.5° × 2.5°) with randomized position (within a rectangle) and were randomly colored (red, blue, green, yellow, gray, cyan, and violet). The target stimulus appeared for 100 ms duration and was followed by a retention period of 1200 ms during which subjects had to retain the target memory array. This was followed by the presentation of a probe image. Subjects had 2000 ms before the onset of the next trial to make a push-button response to indicate whether or not the probe was identical to the target. This hemifield (left/right) × array size (2, 3, 4, 6) × probe match (yes/no) design had 130 trials per condition, where match and no-match trials occurred randomly, with equal probability (Figure 1A). For each subject, a measure of the number of items actually retained for the four load conditions, known as the K value (Cowan, 2001), was computed as K = S*(H − F), where S is the array size, H is the observed hit rate and F the false alarm rate over all trials.
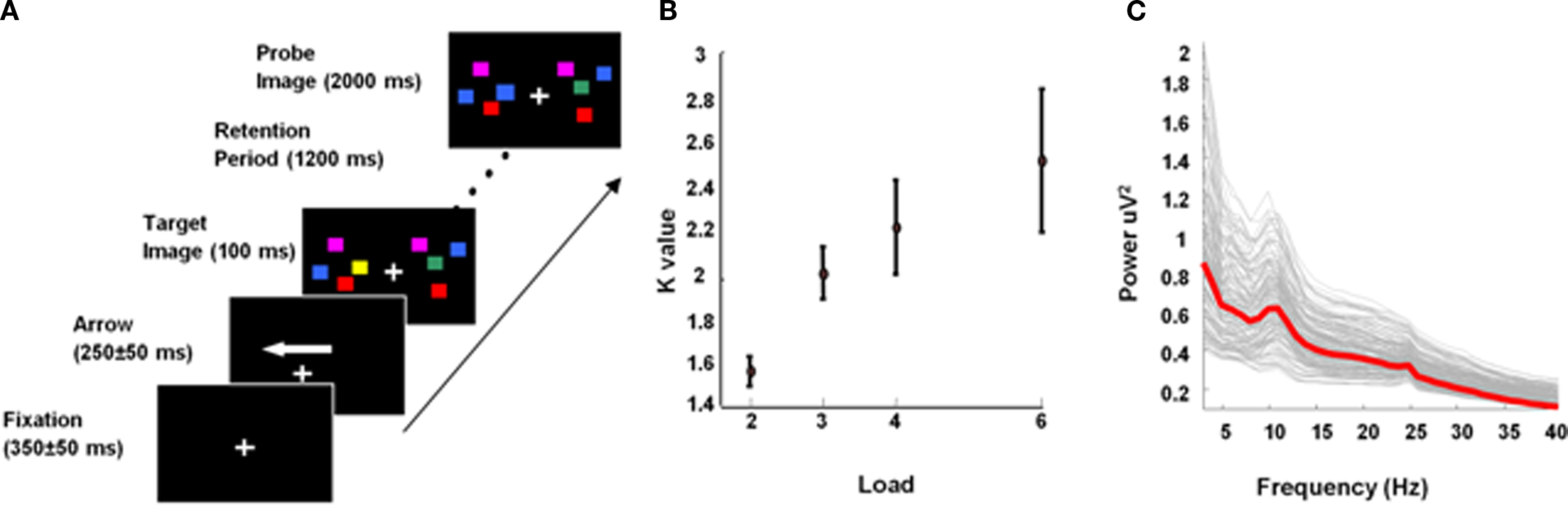
Figure 1. Experimental design. (A) Experimental design. (B) Load-specific K, averaged across subjects. Error bars, here and in subsequent figures, depict SEM. (C) MEG sensor data: frequency spectra for maintenance period at each sensor, averaged over trial type and subjects; average is illustrated with the red line.
Data Acquisition and Preprocessing
Magnetoencephalography data were recorded continuously (sample rate: 254.3 Hz, band pass online filter: 0.1–50 Hz) using a 148-channel whole head system (MAGNES 2500 WH, 4D Neuroimage Inc., San Diego, CA, USA). EOG and ECG were acquired with a Synamps amplifier (NeuroScan™, El Paso, TX, USA) using Ag/AgCl electrodes (same sample rate and online filters as above). We next epoched delay period activity (1200 ms) for each trial, for each subject. Data were filtered from 1 to 48 Hz. Artifact rejections were performed using a thresholoding algorithm implemented in SPM8 (http://www.fil.ion.ucl.ac.uk/spm/). Sensor averaged root mean square (RMS) power was computed and the threshold set to 10 times this level. To check for confounding incidental signal changes, we correlated the proportion of rejected trials with the individual’s memory capacity but found no correlation (R = 0.15, p = 0.61). The key analysis routines used for the present paper are freely available in the M/EEGTools toolboxes distributed with SPM8; i.e., the SPM8 installation is sufficient to reproduce our analysis.
Sensor Space Analysis
Frequency domain representations were constructed from the time series using a vector auto regression (VAR) model of order p = 16. Specifically, data from the each sensor was modeled as an AR process (Roberts and Penny, 2002).

The autoregressive coefficients were estimated using each sensor’s auto time-series components. These provided a direct estimate of the spectral density at each sensor for frequencies from 2 to 40 Hz. These were then averaged across all trials and subjects to provide a summary of the oscillatory components present during maintenance. The estimation of the auto-regression coefficients uses the spectral toolbox in SPM8.
For the statistical analysis of sensor level data, sixteen time-frequency representations comprising responses at 4–5, 6–7, 8–9, and 10–12 Hz at each load condition (2, 3, 4, and 6) were extracted. This was done separately for each subject at each sensor, using a seven-cycle Morlet wavelet transform as implemented in SPM8. These data were then scaled logarithmically and averaged within their respective frequency sub-bands to obtain a sensor × time representation for each frequency window. These data were then converted to two-dimensional images (Kilner et al., 2005). Hence, for each load condition we obtained a representation of “low theta” (4–5 Hz), “mid theta” (6–7 Hz) “high theta” (8–9 Hz), and “low alpha” (10–12 Hz) at each sensor; giving a total of sixteen images per subject. We denote these images as Im1, corresponding to 4–5 Hz power at a load of 2, Im2, corresponding to 4–5 Hz power at load 3, up to Im16 corresponding to 10–12 Hz power at load 6.
Statistical Analysis
Sensor level images were analyzed employing a two-level random effects design using SPM8. Specifically, in order to test for regions in sensor space which display the principle of increasing 4–12 Hz frequency for increasing item retention, we applied a subject specific K × Band contrast to all the sensors (first level, i.e., individual subject level analysis). Thus, we tested whether sensor level data exhibit enhanced low frequency theta power at low K values and high frequency alpha power at high K values. Increasing peak frequency was modeled as the midpoint of the four frequency bands, i.e., [4.5 6.5 8.5 11], which after mean correction is [−3.125 −1.125 0.875 3.375]. For example, if a subject had K values of 2, 3, 4, and 6 at loads 2, 3, 4, and 6 respectively (i.e., they performed with 100% accuracy) then the mean corrected K values were [−1.75, −0.75, 0.25, and 2.25]. The K × Band contrast can be expressed as [−1.75*Im1 + −0.75*Im2 + 0.25*Im3 + 2.25*Im4]*−3.125 + [−1.75*Im5 + −0.75*Im6 + 0.25*Im7 + 2.25*Im8]*−1.125 + [−1.75*Im9 + −0.75*Im10 + 0.25*Im11 + 2.25*Im12]*0.875 + [−1.75*Im13 + −0.75*Im14 + 0.25*Im15 + 2.25*Im16]*3.375. In other words, the 16 images per subject are multiplied by the above weights in order to create a final “contrast” image for each subject representing the K × Band interaction.
The ensuing subject-specific contrast images were entered into a one-sample t-test (second level analysis) and the resulting statistical parametric map (SPM) examined for regional-specific effects at a threshold of p < 0.005 uncorrected. After finding regions of sensor space demonstrating a significant K × Band interaction, we then selected the single sensor over the maximum t-value for further illustration. The frequency spectrum from this sensor was computed for each subject using the AR method above. In order to illustrate the principle of increasing frequency peak for increasing memory retention, we plotted these spectra after a median split of the subjects based on performance. We also calculated the average bandwidth for each group. The maximum of the band was calculated as the average frequency at which the group peaked, and the minimum was computed as the frequency at which the average spectra reaches a value 50% higher than baseline, i.e., the 3 dB point (Oppenheim and Schafer, 1974), where we define baseline as mean spectral power between 2 and 4 Hz.
Source Modeling
SPM8’s multiple sparse prior routine was used to estimate the cortical origin of the maintenance period neuronal response (Friston et al., 2008). A tessellated cortical mesh template surface in canonical Montreal Neurological Institute (MNI) anatomical space (http://www.bic.mni.mcgill.ca/brainweb) and implemented in SPM8, served as a brain model to estimate the current source distribution (Mattout et al., 2007). This dipole mesh was used to calculate the forward solution using a spherical head model. The inverse solution was calculated using a time window from 200 to 1200 ms during the maintenance epoch. The inverse was constructed using the multiple sparse priors (default) option in SPM8. Here several hundred patches of activation are iteratively reduced until an optimal number and location of active patches are found using an iterative Bayesian search method (Friston et al., 2008). This was performed for each frequency bin as before (i.e., 4–5 Hz; 6–7, 8–9, 10–12 Hz) and for each load condition. Dipole measures were then interpolated into MNI voxel space, as implemented in SPM8.
We tested for three specific effects of interest in source space, employing a two level random effects analysis as above, computing first a contrast image per subject and then entering these images into a one-sample t-test across subjects. We first examined the effects of memory retention independent of particular frequency. For this we computed subject-specific contrast images, scaling each load image by the appropriate K value for that subject at that load. To extend our findings at the sensor level to source space, we next examined the K × Band interaction, having generated contrast images in source space in an analogous manner as applied to the sensor data. The positive tail of this one-sample t-test tests for an increase in peak theta/alpha frequency with increase memory retention. The opposite comparison (the negative contrast) tests for a negative K × Band interaction, i.e., regions that show a lower frequency theta response for increasing item retention.
Results
Behavioral Results
The K value (Cowan, 2001), an estimate of the actual number of items retained by the individual for the given load array, was calculated for each subject for all load conditions (Figure 1B). We refer to capacity as the maximum number of items retained (Kmax), which can occur at any load. Consistent with previous findings (see Awh and Vogel, 2008), Kmax varied among subjects from around 1.5 to 5 items (mean 2.5 ± 1.2). Since the maximum items retained by any individual was five, all subjects received a stimulus set size that comprised both sub- and supracapacity stimuli sets, i.e., no subject could retain six items in WM.
Sensor Space Analysis
To illustrate the oscillatory components present during memory maintenance, averaged frequency responses were computed for each sensor using all trials and all subjects for frequencies from 2 to 40 Hz. Figure 1C illustrates that theta and low alpha activity was most prominent during memory maintenance. Our analysis was hence constrained to the frequencies from 4 to 12 Hz. This comprised the traditional theta (Jensen and Tesche, 2002) and low alpha (Jensen et al., 2002) and also allows our findings to be related to theta studies of rodent LFPs which treat “theta” as those oscillatory components in a higher, e.g., 4–10 Hz, range (Vertes, 2005; Raghavachari et al., 2006; Shirvalkara et al., 2010).
To first test our hypothesis that increased memory capacity is reflected in increasing frequency into the alpha band, we performed a statistical analysis on the sensor level data. We tested for regions in sensor space demonstrating an item retention × band interaction, where item retention is again the subject-specific K value for that load and band is modeled as an increase in 4–12 Hz frequencies employing four levels at 4–5, 6–7, 8–9, and 10–12 Hz. Thus, for each subject we created a two-dimensional sensor space “image” of the item retention × band interaction, collapsing across attended hemifield conditions. This image is simply an interpolated representation of all sensors, smoothed with a Gaussian kernel of 12 mm full width half maximum (FWHM). Each subject’s image was then entered into a one-sample t-test to yield a SPM in 2D sensor space testing for an item retention × band interaction across subjects. This initial analysis, which collapsed over attended-hemifield, revealed a significant interaction in channels over bilateral frontal and right parietal regions (Figure 2A). These sensor data thus express greater activity in the low frequency theta band, 4–5 Hz, when load is low and fewer items need to be held in VWM, in contrast to greater activity at higher alpha frequencies, 10–12 Hz, when item retention is at capacity. Thus, as predicted, we demonstrate a principle of higher frequency oscillatory responses during conditions that necessitate greater information maintenance.
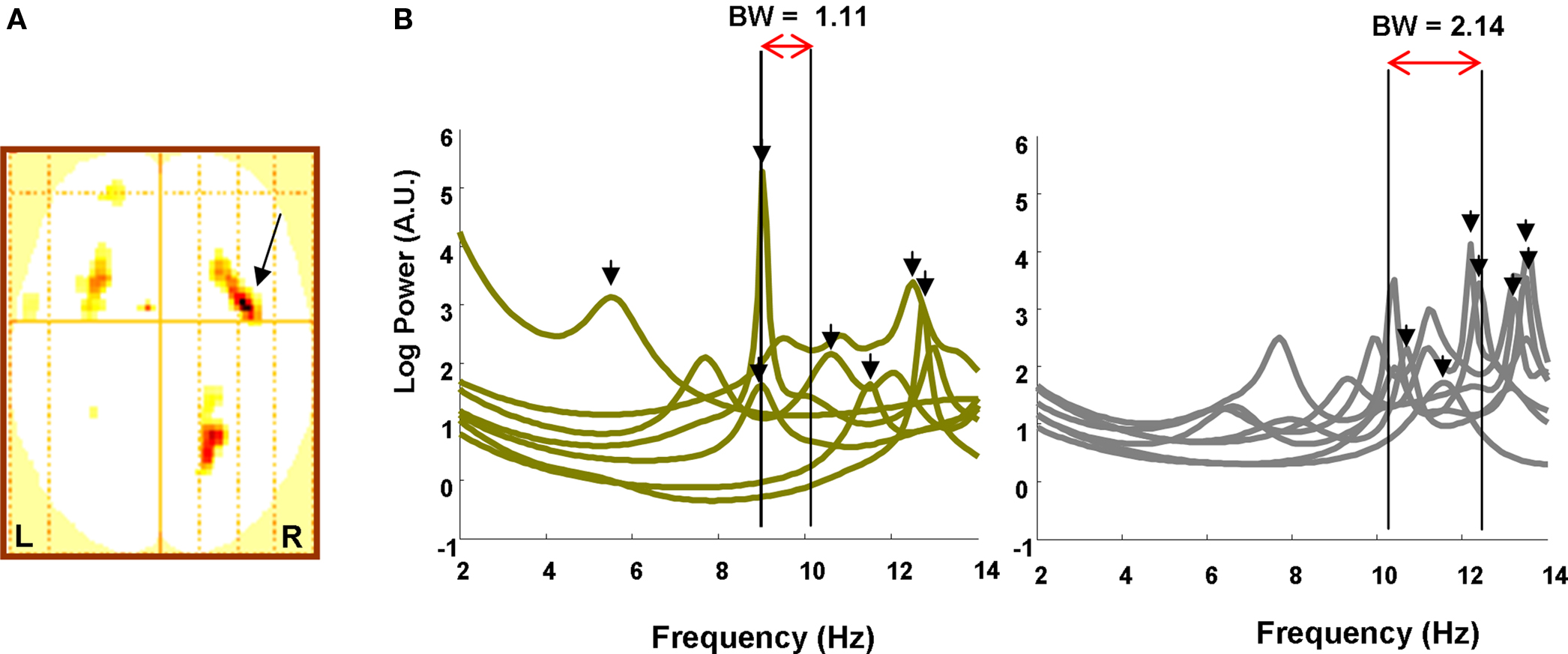
Figure 2. Sensor data. (A) SPM (threshold p < 0.005) in two dimensional channel space (up is anterior, left is left) showing a significant K × Band interaction over frontal and parietal sites. Arrow points to global maximum in right frontal site (z = 4.00; p = 0.000). (B) The power spectrum from the right frontal sensor overlaying the global maximum in (A) is plotted from 2 to 14 Hz for Left, low WM capacity and Right, high capacity individuals (high and low capacity defined by median split). Whereas low capacity individuals express 4–12 Hz peaks at (mean, SEM) 10.11 Hz (0.96), high capacity individuals achieve peaks at 12.44 Hz (0.40). Bandwidth measures from 4 to 12 Hz onset, defined as a rise in power greater than 50% of baseline (2–4 Hz) activity (i.e., the 3 dB point), to the peaks are illustrated for each group. Low capacity subjects have a narrowband occupancy (1.11 Hz) while high capacity subjects use a comparatively wideband signal (2.14 Hz).
A possible, alternative basis for this observation, however, is that it represents an ongoing heightened cortical alpha rather than alpha responses being a function of task. To address this possibility, we examined a baseline period during fixation (350 ms). We extracted the RMS power for 10–12 Hz and averaged over sensors and correlated this measure with memory capacity. Critically, there was no significant correlation between these measures (R = −0.27; p = 0.35). We therefore surmise that these higher frequency responses are produced by task demands.
In Figure 2B we further illustrate the adherence of our data to a principle of higher frequency responses when greater information needs to be maintained by plotting the spectrum from the right frontal sensor overlaying the global maximum of Figure 2A. We performed a median split of the subjects based on their individual memory capacity. Low capacity subjects had an average maximum item retention of 1.90 ± 0.11 (SEM) while high capacity subjects could retain 3.53 ± 0.32 items in working memory. We highlight the frequency at which the spectrum peaks between 2 and 14 Hz and observe that, for low capacity subjects, spectral peaks occur, on average, at 10.11 ± 0.96 Hz while for high capacity subjects, peaks are found at 12.44 ± 0.40 Hz. The peak frequencies were significantly greater in the high capacity group (one tailed t-test, t(12) = 2.25; p = 0.022), whereas the amplitude of the peak was not significantly different (one tailed t-test, t(12) = −0.36); p = 0.36), showing that the effect is associated with the frequency of 4–12 Hz oscillations and not maximum power. We then constructed a bandwidth measure for each group where the band minimum was defined as the frequency at which the average spectrum for that group rose to 50% above the baseline and the maximum was set at the average peak frequency. As illustrated in Figure 2B, we observe a wider band for the high capacity subjects (BW = 2.14 Hz) compared to the low capacity subjects (BW = 1.11 Hz). Thus, high capacity subjects, in addition to displaying higher frequency responses, employ a wideband signal for maintenance.
Previous studies employing the current task demonstrate that increasing memory load increases theta power primarily over contralateral sites, whereas alpha power is increased ipsilaterally and decreased contralaterally (Jokisch and Jensen, 2007; Sauseng et al., 2009). We therefore next examined laterality effects by testing item retention × band interactions for right and left hemifield attended conditions separately. This followed an identical procedure as that described above, except that here 2D images representing an item retention × band interaction were created separately for left- and right-hemifield attended trials and entered into separate one-sample t-tests across subjects. Hence data from contralateral and ipsilateral sensors were examined separately, in different regions of the image (except at the midline where there will be some effect of smoothing). For arrays attended on the right, we observe a significant interaction bilaterally with a maximum over left frontal sensors (Figure 3A). By contrast, for arrays attended to on the left we observe bilateral activity but with maximum over right frontal sensors (Figure 3B). This observation is critical to the interpretation of our data because the initial analysis, collapsing across hemifield (Figure 2A), potentially confounded task relevant processes over contralateral sites and task irrelevant/competing processes over ipsilateral sites. We show that at frontal sites, the effect of maintenance on band is bilateral, with a stronger effect contralateral to the attended hemifield. Thus these observations confirm that the effect of item retention on frequency band illustrated in Figure 2A is not driven by an ipsilateral increase in alpha power (see Supplementary Material for further discussion). To further demonstrate the contralateral locus of the effect we describe, we performed a paired t-test comparing the K × band interaction between the two attended hemifield conditions. This confirms that higher frequency for higher maintenance levels are expressed over contralateral hemispheres (Figures 3C,D). We demonstrate these effects using averaged frontal sensor spectra from ipsi and contralateral sites for the two attended hemifields at high and low load conditions in Figure 3E.
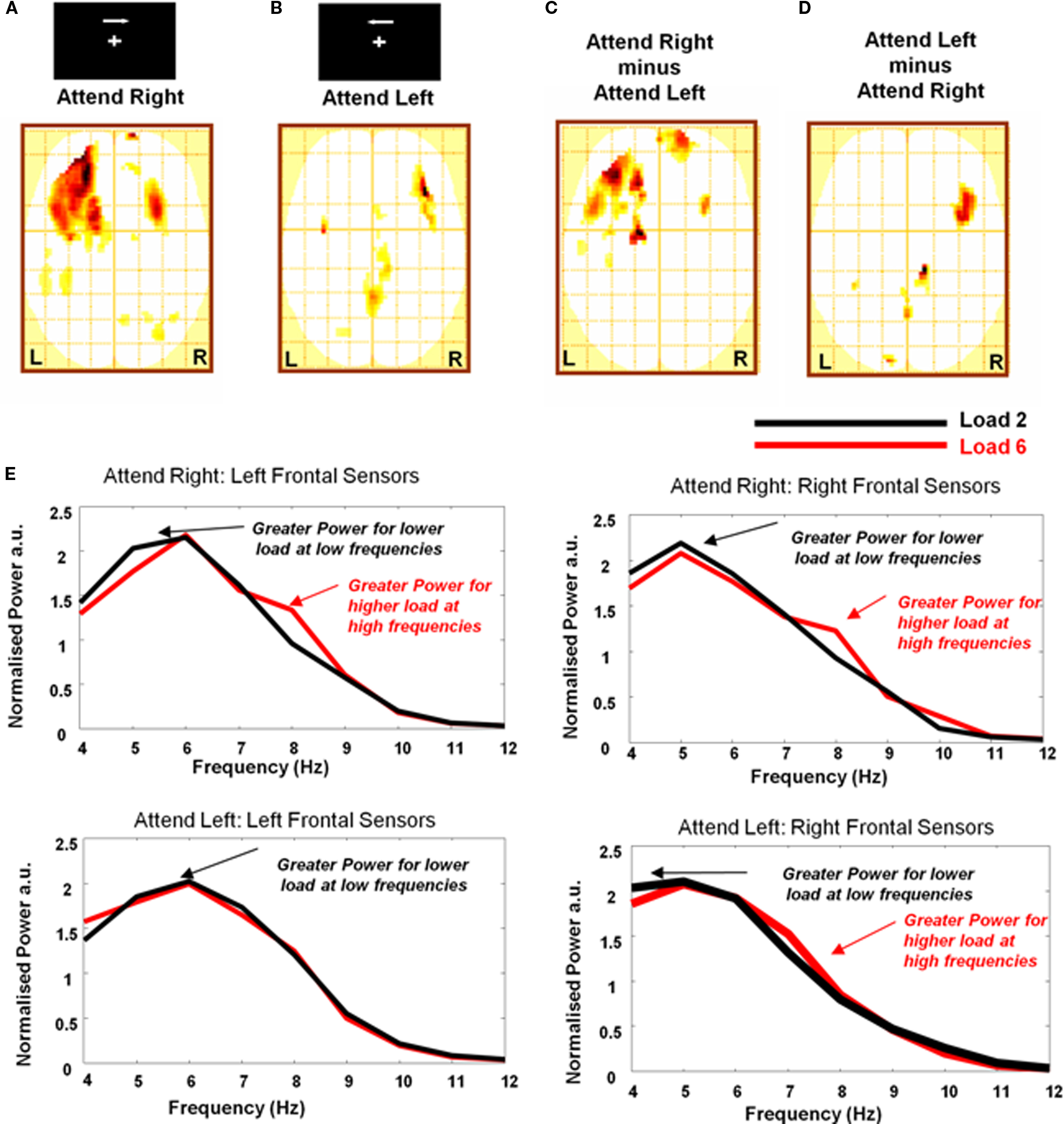
Figure 3. Contralateral and ipsilateral sensor analysis. (A) SPM (threshold p < 0.01, one-sample t-test) showing K × Band interaction for stimulus arrays attended to in the right hemifield with significant effects observed bilaterally over frontal and occipito-parietal sensors and a maximum over left frontal cortex. (B) SPM (threshold p < 0.01, one-sample t-test) showing interaction for stimulus arrays attended to in the left hemifield with again significant effects observed bilaterally over frontal and occipito-parietal sensors but here the maximum is over contralateral right frontal cortex. (C,D) Significant differences (threshold p < 0.01, paired t-test) between the K × Band interaction are observed with peaks over contralateral sensors. (E) Average normalized spectra for loads of 2 (black line) and 6 (red line) demonstrating this effect at 1 Hz resolution for the theta–alpha frequencies included above (4–12 Hz). Spectra are averaged over frontal sensors from ipsilateral and contralateral sites for both attend right and attend left hemifield trials. Greater power at lower frequencies are observed for low (2) load trials while for high (6) load trials, power increases at higher theta/alpha frequencies. Individual subject spectra are included in Figure S1 in Supplementary material.
Source Space Analysis
Having established a significant K × Band interaction at the level of MEG sensors, we were interested in determining the underlying generative sources. We first localized the maintenance period activity for each subject and each condition, for four different frequency regions of interest (4–5, 6–7, 8–9, and 10–12 Hz). We next tested for cortical responses displaying increased 4–12 Hz power for increasing item retention. This analysis, which averaged over the four frequency measures, revealed a significant effect of item retention in left and right parieto-occipital and left temporo-occipital regions (Figure 4). This pattern of activity is consistent with previous reports of correlations between WM capacity and parieto-occipital activity, indexed by ERPs (Vogel and Machizawa, 2004; Vogel et al., 2005) and fMRI activations (Todd and Marois, 2004; Xu and Chun, 2005).
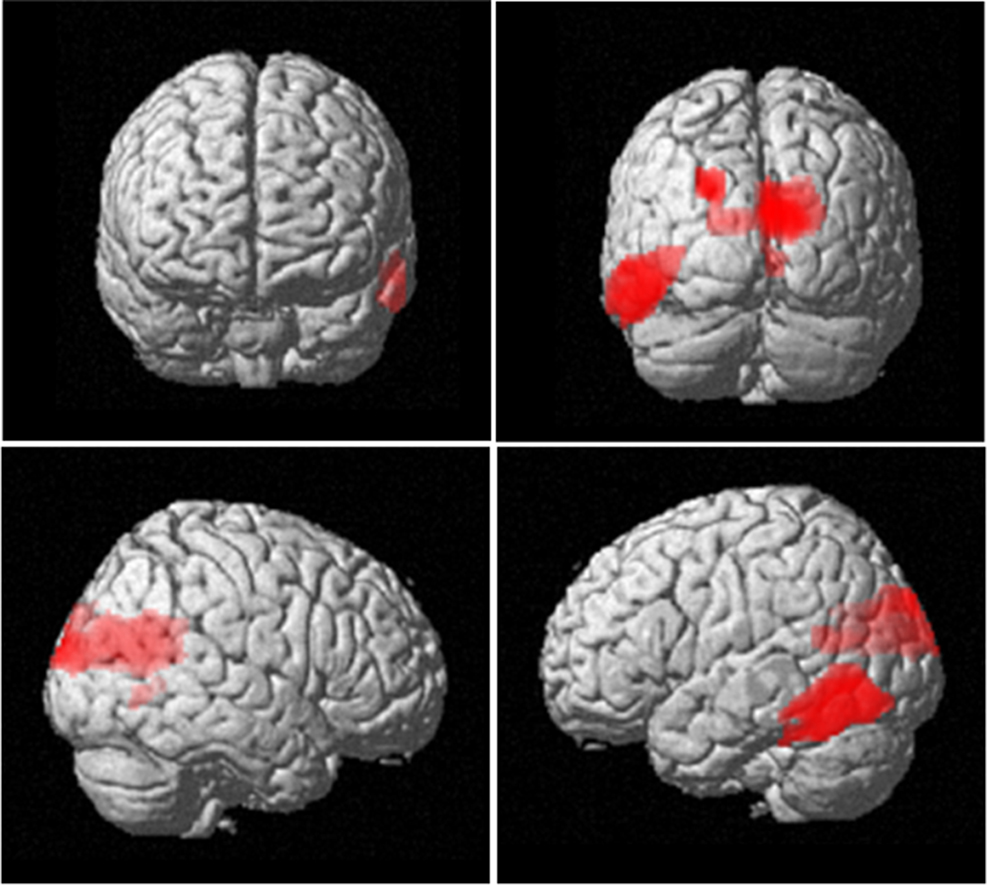
Figure 4. Neuroanatomical network showing increased 4–12 Hz power with increasing item retention. The SPM has been rendered onto a canonical T1 structural image (height threshold p < 0.015 uncorrected; extent threshold 15 contiguous voxels) and demonstrates activation bilaterally in occipital areas (x, y, z co-ordinates 2, −62, 14; z = 3.19; p = 0.001), extending into inferior temporal cortex (−58, −68, −4; z = 2.63; p = 0.004).
Our critical comparison tested for the K × Band interaction where low frequency theta co-occurs with low retention demands and higher frequency alpha is expressed with high retention demands. We demonstrate a significant K × Band interaction in a network of bilateral dorsolateral prefrontal and right inferior parietal cortex (Figure 5A). To illustrate this effect, we extracted power estimates for each subject and for each load condition from the peak voxel in right dorsolateral prefrontal cortex. The difference in estimates of 4–12 Hz power between the load at which Kmax is attained versus the lowest load of two items (at which all subjects exhibited their minimum K value, Kmin) is plotted for each of the four sub-band ranges, averaged over subjects (Figure 5B). For example, if a subject reached capacity at an item load of six then the difference between 4 and 12 Hz activity on trials of load six versus load two was entered into this average. We demonstrate a capacity-dependent parametric increase across the range, where 4–5 Hz activity dominates in conditions of minimal item retention, frequencies from 6 to 9 Hz show similar profiles for low and high memory demands (i.e., power differences close to zero), and high frequency activity from 10 to 12 Hz is more active at Kmax compared to Kmin.
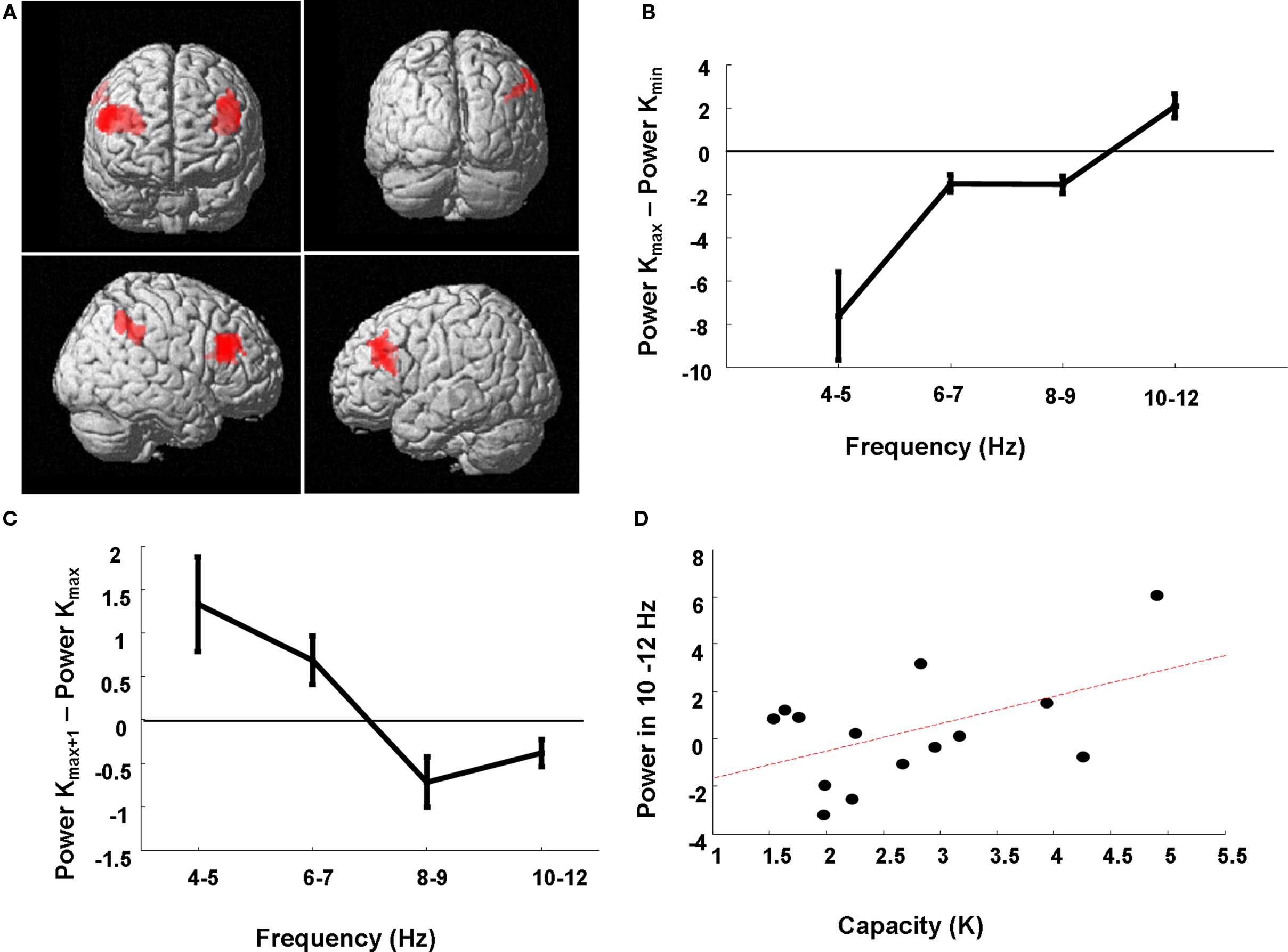
Figure 5. Neuroanatomical network subtending higher frequency responses for increasing item retention. (A) The SPM has been rendered onto a canonical T1 structural image (height threshold p < 0.015 uncorrected; extent threshold 15 contiguous voxels) and demonstrates a significant K × Band interaction in a prefrontal–parietal network in which low theta frequencies support low item retention and higher alpha frequencies support high item retention. Significant effects are observed in right (56, 28, 30; z = 2.92; p = 0.002) and left (−32, 22, 16; z = 2.78; p = 0.003) dorsolateral prefrontal cortex (dlPFC), and right inferior parietal cortex (60, −42, 44; z = 2.37; p = 0.009). (B) Power estimates from the peak voxel (right dlPFC) for each of the four sub-bands are plotted for the load at which subjects reached Kmax minus that for load 2, showing dominant low frequencies at low retention levels and increasingly higher frequencies employed for high retention at Kmax. (C) Power estimates from the peak voxel (right dlPFC) for frequency-specific theta power, for the six individuals with Kmax at loads less than 6, for the next higher load than the load at which they achieve Kmax (Kmax + 1) minus that at the load corresponding to Kmax. Three subjects achieved Kmax at load 4, and three subjects at load 3. These individuals failed to recruit higher alpha frequencies for higher loads. (D) Subject-specific capacity (Kmax) is plotted against the high frequency sub-band (R = 0.50). Power estimates are from the peak voxel (right dlPFC) for the 10–12 Hz frequency range at load 6 minus the average 10–12 Hz power across all loads.
To further illustrate the capacity-dependence of the 4–12 Hz peak frequency effect we observe in right dorsolateral prefrontal cortex, we plot the power estimates from this region only for subjects who reached Kmax at loads lower than the maximum load of 6 (6 of the 14 subjects). Thus, in Figure 5C we plot the power estimate differences between activity at the next higher load than the load at which they achieve Kmax (i.e., Kmax + 1) minus that at the load corresponding to Kmax. We observe a negative difference at all frequencies above and including 8 Hz, highlighting how failure to recruit higher frequencies is associated with a failure to incorporate more items into working memory. Figure 5D presents a similar interpretation of these power estimates. Here we plot the average right prefrontal 10–12 Hz activity at Kmax minus the average 10–12 Hz power for all trial types measured in this region per subject against that subject’s memory capacity. This again serves to illustrate how high capacity subjects have greater 10–12 Hz resources to meet high memory demands (R = 0.50).
Discussion
Previous ERP (Vogel and Machizawa, 2004; Vogel et al., 2005) and fMRI (Callicott et al., 1999; Todd and Marois, 2004) studies of VWM capacity indicate that neuronal measures correlating with item retention asymptote once capacity is attained, i.e., no further increase in activity is observed with increase in load. We find, in sensors overlaying frontal and parietal sites, a circuit which operates at higher frequencies under high WM demands (Figure 2A). We show that once maximum item retention is attained, theta or alpha frequency asymptotes, showing no further increase despite an increase in visual input and VWM demands. Having found this effect in sensor space, we then localized this effect to reveal a distributed network of bilateral dorsolateral prefrontal cortex and inferior parietal cortex subtending these effects (Figure 5A). Of the subjects tested, six exhibited their maximum item retention (Kmax) at a load less than 6. Figure 5C illustrates that these subjects fail to recruit higher alpha bands at loads greater than that at which they achieve Kmax. Specifically, these subjects fail to show any significant increase in the higher frequency bands (8–12 Hz) and, in fact show a decrease in 8–12 Hz activity. Crucially, we show a prefrontal–parietal network of oscillations which follows this saturation at maximum capacity. While posterior cortical activity has been commonly reported to be related to VWM capacity (Vogel and Machizawa, 2004; Vogel et al., 2005; Ikkai et al., 2010), the prefrontal cortex has been more elusive in electrophysiological studies. Despite this failure to observe capacity limit effects in frontal sites, activation of frontal cortex is thought to be relevant in the oscillatory neural network associated with WM capacity (Sauseng et al., 2009). Accordingly, our current results converge with the conceptualization that prefrontal oscillations participate in controlled processing of the activity of posterior brain regions and suggest that a frequency modulated frontal-posterior network is responsible for memory capacity limits (Grimault et al., 2009).
Theoretical accounts of the role of theta frequency in WM are based on the observation of phase precession in hippocampal place cells, i.e., the gradual shifting of sequentially occurring spikes to earlier phases of the theta cycle as the rat approaches and passes through the cell’s place field (O’Keefe and Recce, 1993). Lisman and colleagues (Lisman and Idiart, 1995; Jensen and Lisman, 1996, 1998; Lisman, 2005) propose that oscillations in the medial temporal lobe could facilitate working memory “bundles” of 7 ± 2 items using a similar phase encoding scheme. For this, gamma bursts occurring at a particular theta phase could support a single item of memory maintained across time through auto-associative Hebbian plasticity (Hebb, 1949). It follows that longer theta cycles would accommodate more items in WM. Empirical support for this proposal comes from recent human intracranial hippocampal recordings (Axmacher et al. 2010), demonstrating that as memory load increases, theta oscillations in the hippocampus predominate at lower frequencies. By contrast, the model we advance predicts the opposite effect, i.e., that oscillations at alpha frequencies from 10 to 12 Hz predominate with increasing WM demands. We provide direct evidence that oscillations in a prefrontal–parietal network adhere to this model (Figure 5), which could be similarly maintained through auto-association among recurrent collaterals (Hebb, 1949). However, we note that the coding scheme elaborated by Lisman and colleagues, and the one proposed here, are not mutually exclusive and could indeed co-exist in the brain. To test for this possibility, we computed the negative K × Band interaction at the source level, which tests for areas expressing longer theta cycles (lower theta frequencies in our selected theta/alpha sub-bands) when subjects are at WM capacity. Figure 6 shows the result of this contrast, where strikingly we observe a significant effect in medial temporal regions including regions of the posterior hippocampus. Notably, however, the prefrontal cortex is absent from this analysis, suggesting dissociable WM circuits operating in the theta range.
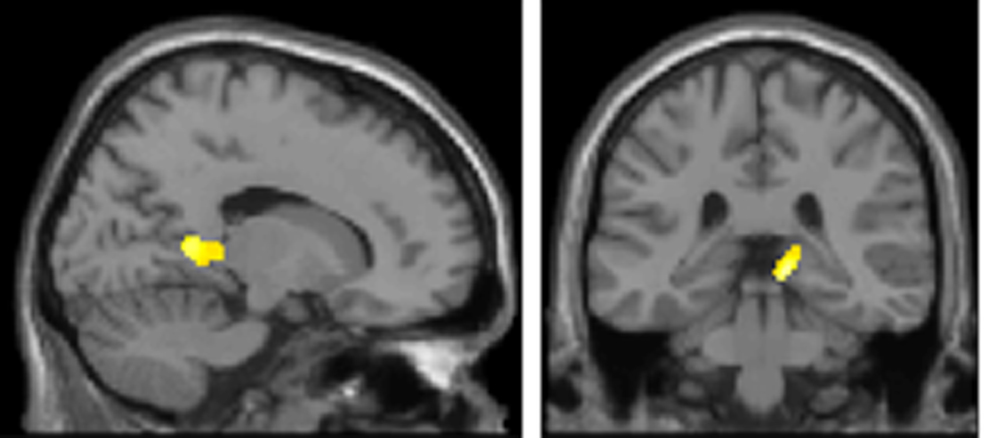
Figure 6. Medial temporal lobe shows predominant low frequency theta for increasing item retention. The SPM (threshold p < 0.005; extent threshold 15 voxels) is overlaid on a sagittal (x = 17) and coronal (y = −34) T1-weighted, canonical section to illustrate hippocampal activation in the opposite interaction to Figure 5A. Occipital and medial temporal regions express low frequency theta frequencies for higher retention requirements, i.e., for the negative K × Band interaction. Peak voxel in left occipital cortex (−34, −86, −12; z = 3.72; p = 0.000) and cluster peak in right medial temporal lobe (12, −32, −4; z = 3.56; p = 0.000).
Our data highlight multiple oscillatory mechanisms operational during VWM. These finding are consistent with new theories of a generalized “global neuronal workspace” whereby effortful cognition is proposed to involve the activity of a distributed network with long-range connectivity that can then in turn mobilize more local, modular cortical processing (Dehaene et al., 1998; Sigman and Dehaene, 2008). Prefrontal cortex is thought to instantiate such a network where it recruits more posterior regions, via long-range axonal projections. It has also been proposed that such a scheme could have oscillatory substrates (Lutz et al., 2002; Palva and Palva, 2007). Indeed several studies of power and coherence at theta and alpha frequencies have reported activity over such networks for different phases of WM processing, including encoding, retention, and retrieval (Bastiaansen et al., 2002; Sauseng et al., 2002, 2004, 2005a,b). In our data we observe a distributed network including dorsolateral prefrontal and parietal regions that adheres to a particular property along the frequency axis co-existing with two localized circuits. The first localized circuit is a posterior occipital network that displays increased theta and alpha power, independent of particular frequency, for increased maintenance (Figure 4). In this analysis we found no evidence for frontal involvement. This modular circuit is consistent with earlier work on oscillatory correlates of WM, which examined the power differences within frequency bands as memory loads are manipulated (Gevins et al., 1997; Jensen and Tesche, 2002). The second localized circuit comprises a hippocampal-centric network, which includes occipital and medial temporal regions, where the rhythms recorded move to lower frequencies to support high WM demands.
In conclusion we show that oscillatory mechanisms of VWM comprise interacting processes across a distributed network that includes prefrontal, occipito-parietal, and medial temporal regions, where capacity limitations are associated with both increased and decreased frequencies in the 4–12 Hz range.
Conflict of Interest Statement
The authors declare that the research was conducted in the absence of any commercial or financial relationships that could be construed as a potential conflict of interest.
Acknowledgments
This work was funded by a grant from the Ministry of Science, Spain SEJ2006-14571 to Pablo Campo, and partially supported by a Grant from the Comunidad Autónoma de Madrid, Spain (Madr.IB S-SAL-0312-2006). Rosalyn J. Moran was funded by an Award from the Max Planck Society to Raymond J. Dolan; Raymond J. Dolan is supported by the Wellcome Trust.
References
Axmacher, N., Henseler, M. M., Jensen, O., Weinreich, I., Elger, C. E., and Fell, J. (2010). Cross-frequency coupling supports multi-item working memory in the human hippocampus. Proc. Natl. Acad. Sci. U.S.A. 107, 3228–3233.
Barch, D. M., Braver, T. S., Nystrom, L. E., Forman, S. D., Noll, D. C., and Cohen, J. D. (1997). Dissociating working memory from task difficulty in human prefrontal cortex. Neuropsychologia 35, 1373–1380.
Bastiaansen, M. C. M., Posthuma, D., Groot, P. F. C., and de Geus, E. J. C. (2002). Event-related alpha and theta responses in a visuo-spatial working memory task. Clin. Neurophys. 113, 1882–1893.
Callicott, J. H., Mattay, V. S., Bertolino, A., Finn, K., Coppola, R., Frank, J. A., Goldberg, T. E., and Weinberger, D. R. (1999). Physiological characteristics of capacity constraints in working memory as revealed by functional MRI. Cereb. Cortex 9, 20–26.
Cowan, N. (2001). The magical number 4 in short-term memory: a reconsideration of mental storage capacity. Behav. Brain Sci. 24, 87–185.
Dehaene, S., Kerszberg, M., and Changeux, J.-P. (1998). A neuronal model of a global workspace in effortful cognitive tasks. Proc. Natl. Acad. Sci. U.S.A. 95, 14529–14534.
Deiber, M. P., Missonnier, P., Bertrand, O., Gold, G., Fazio-Costa, L., Ibanez, V., and Giannakopoulos, P. (2007). Distinction between perceptual and attentional processing in working memory tasks: a study of phase-locked and induced oscillatory brain dynamics. J. Cogn. Neurosci. 19, 158–172.
Friston, K. J., Harrison, L., Daunizeau, J., Kiebel, S., Phillips, C., Trujillo-Barreto, N., Henson. R., Flandin, G., and Mattout, J. (2008). Multiple sparse priors for the M/EEG inverse problem. Neuroimage 39, 1104–1120.
Fukuda, K., Awh, E., and Vogel, E. K. (2010). Discrete capacity limits in visual working memory. Curr. Opin. Neurobiol. 20, 177–182.
Gevins, A., Smith, M. E., McEvoy, L., and Yu, D. (1997). High-resolution EEG mapping of cortical activation related to working memory: effects of task difficulty, type of processing, and practice. Cereb. Cortex 7, 374–385.
Grimault, S., Robitaille, N., Grova, C., Lina, J. M., Dubarry, A. S., and Jolicoeur, P. (2009). Oscillatory activity in parietal and dorsolateral prefrontal cortex during retention in visual short-term memory: additive effects of spatial attention and memory load. Hum. Brain Mapp. 30, 3378–3392.
Hyman, J. M., Zilli, E. A., Paley, A. M., and Hasselmo, M. E. (2010). Working memory performance correlates with prefrontal-hippocampal theta interactions but not with prefrontal neuron firing rates. Front. Integr. Neurosci. 4: 2. doi: 10.3389/neuro.07.002.2010.
Ikkai, I., McCullough, A. W., and Vogel, E. (2010). Contralateral delay activity provides a neural measure of the number of representations in visual working memory. J. Neurophys. 103, 163–1968.
Jensen, O., and Lisman, J. E. (1996). Theta/gamma networl(s with slow NMDA channels learn sequences and encode episodic memory: role of NMDA channels in recall. Learn. Mem. 3, 264–278.
Jensen, O., and Lisman, J. E. (1998). An oscillatory short-term memory buffer model can account for data on the sternberg task. J. Neurosci. 18, 10688–10699.
Jensen, O., and Tesche, C. D. (2002). Frontal theta activity in humans increases with memory load in a working memory task. Eur. J. Neurosci. 15, 1395–1399.
Jensen, O., Jack Gelfand, J., Kounios, J., and Lisman, J. E. (2002). Oscillations in the alpha band (9–12 Hz) increase with memory load during retention in a short-term memory task. Cereb. Cortex 12, 877–882.
Jokisch, D., and Jensen, O. (2007). Modulation of gamma and alpha activity during a working memory task engaging the dorsal or ventral stream. J. Neurosci. 27, 3244–3251.
Kilner, J. M., Kiebel, S. J., and Friston, K. J. (2005). Applications of random field theory to electrophysiology. Neurosci. Lett. 374, 174–178.
Linden, D. E., Bittner, R. A., Muckli, L., Waltz, J. A., Kriegeskorte, N., Goebel, R., Singer, W., and Munk, M. H. (2003). Cortical capacity constraints for visual working memory: dissociation of fMRI load effects in a fronto-parietal network. Neuroimage 20, 1518–1530.
Lisman, J. E. (2005). The theta/gamma discrete phase code occurring during the hippocampal phase precession may be a more general brain coding scheme. Hippocampus 15, 913–922.
Lisman, J. E., and Idiart, M. A. (1995). Storage of 7 ± 2 short-term memories in oscillatory subcycles. Science 267, 1512–1515.
Luck, S. J., and Vogel, E. K. (1997). The capacity of visual working memory for features and conjunctions. Nature 390, 279–281.
Lutz, A., Lachaux, J.-P., Martinerie, J., and Varela, F. J. (2002). Guiding the study of brain dynamics by using first-person data: synchrony patterns correlate with ongoing conscious states during a simple visual task. Proc. Natl. Acad. Sci. U.S.A. 99, 1586–1591.
Mattout, J., Henson, R. N., and Friston, K. J. (2007). Canonical source reconstruction for MEG. Comput. Intell. Neurosci. 67613, 1–10.
McNab, F., and Klingberg, T. (2008). Prefrontal cortex and basal ganglia control access to working memory. Nat. Neurosci. 11, 103–107.
Meltzer, J. A., Zaveri, H. P., Goncharova, I. I., Distasio, M. M., Papademetris, X., Spencer, S. S., Spencer, D. D., and Constable, R. T. (2008). Effects of working memory load on oscillatory power in human intracranial EEG. Cereb. Cortex. 8, 1843–1855.
O’Keefe, J., and Recce, M. L. (1993). Phase relationship between hippocampal place units and the EEG theta rhythm. Hippocampus 3, 317–330.
Onton, J., Delorme, A., and Makeig, S. (2005). Frontal midline EEG dynamics during working memory. Neuroimage 27, 341–356.
Oppenheim, A. V., and Schafer, R. W. (1974). Digital Signal Processing. Englewood Cliffs: Prentice Hall.
Palva, S., and Palva, M. (2007). New vistas for α frequency band oscillations. Trends Neurosci. 30, 150–158.
Raghavachari, S., Lisman, J. E., Tully, M., Madsen, J. R., Bromfield, E. B., and Kahana, M. J. (2006). Theta oscillations in human cortex during a working-memory task: evidence for local generators. J. Neurophysiol. 95, 1630–1638.
Raghavachari, S., Kahana, M. J., Rizzuto, D. S., Caplan, J. B., Kirschen, M. P., Bourgeois, B., Madsen, J. R., and Lisman, J. E. (2001). Gating of human theta oscillations by a working memory task. J. Neurosci. 21, 3175–3183.
Roberts, S. J., and Penny, W. D. (2002). Variational bayes for generalized autoregressive models. IEEE Trans. Signal Process. 50, 2245–2257.
Sarnthein, J., Petsche, H., Rappelsberger, P., Shaw, G. L., and von Stein, A. (1998). Synchronization between prefrontal and posterior association cortex during human working memory. Proc. Natl. Acad. Sci. U.S.A. 95, 7092–7096.
Sauseng, P., Klimesch, W., Heise, K. F., Gruber, W. R., Holz, E., Karim, A. A., Glennon, M., Gerloff, C., Birbaumer, N., and Hummel, F. (2009). Brain oscillatory substrates of visual short-term memory capacity. Curr. Biol. 19, 1846–1852.
Sauseng, P., Klimesch, W., Doppelmayr, M., Pecherstorfer, T., Freunberger, R., and Hanslmayr, S. (2005a). EEG alpha synchronization and functional coupling during top-down processing in a working memory task. Hum. Brain Mapp. 26, 148–155.
Sauseng, P., Klimesch, W., Schabus, M., and Doppelmayr, M. (2005b). Fronto-parietal EEG coherence in theta and upper alpha reflect central executive functions of working memory. Int. J. Psychophys. 57, 97–103.
Sauseng, P., Klimesch, W., Doppelmayr, M., Hanslmayr, S., Schabus, M., and Gruber, W. R. (2004). Theta coupling in the human electroencephalogram during a working memory task. Neurosci. Lett. 354, 123–126.
Sauseng, P., Klimesch, W., Gruber, W. R., Doppelmayr, M., Stadler, W., and Schabus, M. (2002). The interplay between theta and alpha oscillations in the human electroencephalogram reflects the transfer of information between memory systems. Neurosci. Lett. 324, 121–124.
Shirvalkara, P. R., Rappb, P. R., and Shapiroa, M. L. (2010). Bidirectional changes to hippocampal theta–gamma comodulation predict memory for recent spatial episodes. Proc. Natl. Acad. Sci. U.S.A. 107, 7054–7059.
Sigman, M., and Dehaene, S. (2008). Brain mechanisms of serial and parallel processing during dual-task performance. J. Neurosci. 28, 7585–7598.
Tallon-Baudry, C., Bertrand, O., Peronnet, F., and Pernier, J. (1998). Induced γ-band activity during the delay of a visual short-term memory task in humans. J. Neurosci. 18, 4244–4254.
Tesche, C. D., and Karhu, J. (2000). Theta oscillations index human hippocampal activation during a working memory task. Proc. Natl. Acad. Sci. U.S.A. 97, 919–924.
Todd, J. J., and Marois, R. (2004). Capacity limit of visual short-term memory in human posterior parietal cortex. Nature 428, 751–754.
Vertes, R. P. (2005). Hippocampal theta rhythm: a tag for short-term memory. Hippocampus 15, 923–935.
Vogel, E. K., and Machizawa, M. G. (2004). Neural activity predicts individual difference in visual working memory capacity. Nature 428, 748–751.
Vogel, E. K., McCollough, A. W., and Machizawa, M. G. (2005). Neural measures reveal individual differences in controlling access to working memory. Nature 438, 500–503.
Keywords: visual working memory, capacity, theta, alpha, oscillations, MEG
Citation: Moran RJ, Campo P, Maestu F, Reilly RB, Dolan RJ and Strange BA (2010) Peak frequency in the theta and alpha bands correlates with human working memory capacity. Front. Hum. Neurosci. 4:200. doi: 10.3389/fnhum.2010.00200
Received: 24 March 2010;
Accepted: 01 October 2010;
Published online: 11 November 2010.
Edited by:
Russell A. Poldrack, University of California, USAReviewed by:
Wolfgang Klimesch, University of Salzburg, Austria;Geoff Woodman, Vanderbilt University, USA
Copyright: © 2010 Moran, Campo, Maestu, Reilly, Dolan and Strange. This is an open-access article subject to an exclusive license agreement between the authors and the Frontiers Research Foundation, which permits unrestricted use, distribution, and reproduction in any medium, provided the original authors and source are credited.
*Correspondence: Rosalyn J. Moran, Wellcome Trust Centre for Neuroimaging, Institute of Neurology, UCL, 12 Queen Square, London WC1N 3BG, UK. e-mail: r.moran@fil.ion.ucl.ac.uk