- Motor Cognition Group, Max Planck Institute for Neurological Research, Cologne, Germany
The striatum has been established as a carrier of reward-related prediction errors. This prediction error signal concerns the difference between how much reward was predicted and how much reward is gained. However, it remains to be established whether general breaches of expectation, i.e., perceptual prediction errors, are also implemented in the striatum. The current study used functional magnetic resonance imaging (fMRI) to investigate the role of caudate nucleus in breaches of expectation. Importantly, breaches were not related to the occurrence or absence of reward. Preceding the fMRI study, participants were trained to produce a sequence of whole-body movements according to auditory cues. In the fMRI session, they watched movies of a dancer producing the same sequences either according to the cue (88%) or not (12%). Caudate nucleus was activated for the prediction-violating movements. This activation was flanked by activity in posterior superior temporal sulcus, the temporo-parietal junction and adjacent angular gyrus, a network that may convey the deviating movement to caudate nucleus, while frontal areas may reflect adaptive adjustments of the current prediction. Alternative interpretations of caudate activity relating either to the saliency of breaches of expectation or to behavioral adaptation could be excluded by two control contrasts. The results foster the notion that neurons in the caudate nucleus code for a breach in expectation, and point toward a distributed network involved in detecting, signaling and adjusting behavior and expectations toward violated prediction.
Introduction
The striatum was once considered a site of solely motor function, but research over the last three decades has put its cognitive functions more and more into focus (e.g., Alexander et al., 1986; Saint-Cyr, 2003; Grahn et al., 2008). One prominent function of the striatum is the coding of a reward prediction error in learning. These prediction errors are triggered by reinforcement or reward in conditioning paradigms (Schultz et al., 1997, 1998; Schultz, 2000). Reward prediction errors are signified by increases in striatal firing in the presence of unexpected reward or the presence of a reward-predicting cue, or by a decrease of firing when predicted reward is omitted. The underlying notion to a reward prediction error is that the brain is capable of associating the current circumstances with a specific future state (Wolpert and Flanagan, 2001; Friston, 2010). If the future becomes present and the state is different from what was predicted, this violation of predictions causes a prediction error, which in turn incites learning.
That the brain is a “predictive machine” is a feature of many models concerned with learning, action, and perception (Rescorla and Wagner, 1972; Schultz and Dickinson, 2000 Kiebel et al., 2008; Bubic et al., 2009; Friston, 2010). In an extension of the theory of motor control (Wolpert and Flanagan, 2001), the brain’s ability to constantly predict ongoing movement, be it in the motor domain or in perception, has been emphasized (Schutz-Bosbach and Prinz, 2007). Presence of prediction implies the possibility of computing prediction errors, to adjust internal models according to perceptions and thus shape the correct predictions. The exact anatomic implementation of not reward-related prediction error signals, that code for unexpected perceptions has yet to be revealed (Zacks et al., 2007). The proposed involvement of dopamine (Zacks et al., 2007) and the striatum’s extensive connectivity (Alexander et al., 1986; Saint-Cyr, 2003) render it a likely candidate as a site of not only reward-related prediction errors but also more general not reward-related prediction errors.
In fact, there is some evidence that striatal firing-patterns indeed convey prediction errors that are not related to reward. The respective authors likewise used the term prediction error to describe this dorso-striatal activity (Horvitz, 2000; Schultz and Dickinson, 2000; Graybiel, 2005). For the sake of distinction, we will refer to activation that has an amplitude that varies with the amount of (expected) reward as “reward prediction errors.” The prediction errors investigated in the current study, that have a positive amplitude to all violated predictions, will be called “breach of expectation” signals. This breach signal is related to a violated prediction in the simplest sense, i.e., a prediction of any given content is not fulfilled. Accordingly, increased activity toward every unexpected stimulus signifies the breach of expectation signal in dorsal striatum. Indeed, recent imaging studies in humans report caudate nucleus activity for unexpected changes in context, rules, and contingencies (Bunge et al., 2003; Delgado et al., 2005; O’Doherty et al., 2006; Badgaiyan et al., 2007; Koch et al., 2008; den Ouden et al., 2009, 2010). These activations can be broadly interpreted as corresponding to the occurrence of unpredicted stimuli. The study of Davidson et al. (2004), for example, revealed a negative response of the caudate to unexpected target omission, which could be reframed as occurrence of a unpredicted target-free stimulus.
Taken collectively, the data suggest that caudate prediction error signals are not restricted to conditioning protocols and that they do not revolve solely around the availability of reward. The empirical evidence implies the presence of breach of expectation signals in caudate nucleus when an event deviates from predictions, but there is a need to probe the assumption directly.
The lack of studies that target breach of expectation signals is surprising given not only the role they play in current computer models on the matter (Kilner et al., 2007; Kiebel et al., 2008; Friston, 2010) but the enormous relevance of correcting false assumptions to prevent possibly fatal future mistakes. The educative effect of breaches of expectation is so strong that it operates even when observing other peoples behavior. Consider the example of seeing someone being bitten by a bulldog after having tread on its paw. If you used to regard bulldogs as aggressive animals, this would not breach your expectations and not incite changes in your views on bulldogs and your behavior toward them. In other words, you wouldn’t have learned anything. Now consider watching someone being bitten by a poodle after stepping on its paw. This may be a severe breach of your expectations and teach you to regard poodles more suspiciously in future and adapt your behavior toward them accordingly. This is an example of observational learning, which does not imply direct reinforcement – it also embellishes two things. The first is the importance of the severity of a breach of expectation to learning. The second is the bonus derived from valid forward models in guiding behavior.
The current study investigates whether caudate nucleus signals for breaches of expectation in a movement observation paradigm. We hypothesized that watching a dancer make a mistake in a setting of clear-cut cue-movement schedule would yield a caudate response. To keep track of the dancer’s performance, participants had to register auditory cues that determined what movement the dancer was to perform next, and watch the ensuing movements. To ensure that all participants were capable of the required prediction, we subdued them to motor training, where they had to accord to the cue-movement schedule themselves.
Breaches of expectation carry two secondary attributes that could each potentially cause striatal activations. Specifically, these events are of an increased saliency and often prompt to modify ongoing behavior. Saliency can be conceived of as a function of stimulus frequency (Zink et al., 2003) and is an attribute carried of not-habituated stimuli (Redgrave and Gurney, 2006). As violated predictions were rarely encountered in the present paradigm, i.e., infrequent, they might hence elicit striatal activation due to their saliency (Horvitz, 2000; Redgrave and Gurney, 2006). Movement switches as opposed to executing one movement repeatedly have also been associated with striatal activity (Roy et al., 1993; Graybiel, 2005). Encountering violated predictions in the paradigms is related to having to switch to a new internal movement simulation to keep track of the task. Moreover, as the paradigm included extensive training, there may have been an association of movement errors with initiation of a new movement. Hence, saliency and movement switches had to be investigated separately, to ensure that these attributes of violated predictions could not account for potentially recorded striatal activity.
We employed an experimental design that allowed to test whether caudate activity actually reflects breaches of expectation (violation hypothesis – i), or is rather dependent on effects of saliency (saliency hypothesis – ii) or switching to a different behavior (change hypothesis – iii). Breaches of expectation were modeled by contrasting predicted with prediction-violating movements. In accordance with the frequency or habituation approach in the literature, we modeled saliency as a function of stimulus frequency in the immediate trial history. Initiation of a new movement was implemented in the movement observation paradigm by contrasting the cues that indicated a new upcoming movement against cues that indicated a movement repetition.
Although the present study focused on striatal responses, it was to be expected that they come along with cortical activations, as a prominent characteristic of the neostriatum is its pronounced connectivity with a large number of cortical regions and thalamic nuclei (Alexander et al., 1986; Saint-Cyr, 2003). More specifically, activation could be expected in regions related to the processing of biological motion (due to the mismatch between perceived and expected stimulus; Keysers and Perrett, 2004) and those related to attentional modulation more generally (due to the explicit instruction rendering breaches of expectation task-relevant; Corbetta and Shulman, 2002).
Materials and Methods
Participants
Fourteen right-handed, healthy participants (eight women, age 22–29, mean age 24.8) took part in the study. Each participant’s laterality quotient, as assessed with the Edinburgh Handedness Inventory (Oldfield, 1971) was higher than 60. All participants were health screened by a physician and gave informed written consent.
Task-Systematic
The movement repertoire consisted of five whole-body movements. Each movement consisted of three sub-movements which engaged a characteristic combination of extremities (Figure 1). Each of these movements was assigned an arbitrary name, comprising three syllables, each associated with one corresponding sub-movement (Ko-re-pa; Fe-so-da; Gu-la-mi; Ba-ki-te; Wa-ne-ro). None of these names is meaningful in German; neither were the combinations of the two first or last syllables of each. Importantly, in the course of the experiment, each movement (e.g., Ko-re-pa) could only be followed by one specific other (e.g., Fe-so-da) or by a repetition of itself (e.g., Ko-re-pa). Two piano chords, easily discernible even in absence of former musical training, were used to cue the transition between two movements. Each cue coincided with the onset of one movement and delivered an instruction on which movement was to follow the respective movement that had began when the cue sounded. The low chord meant that the transition following the current movement had to be a repetition (i.e., the same movement again; if the movement that started when the cue was presented was for example Ko-re-pa, the low chord meant it had to be followed by another Ko-re-pa). The high chord signaled that the transition following the current movement had to be a switch (i.e., the corresponding next movement; if the concurring movement was Ko-re-pa, the high chord meant it had to be followed by Fe-so-da). Switches were always switches to the next movement in a circular order (Figure 1), no movement was ever skipped. Thus, the upcoming movement was fully predictable, even if it differed from the current movement.
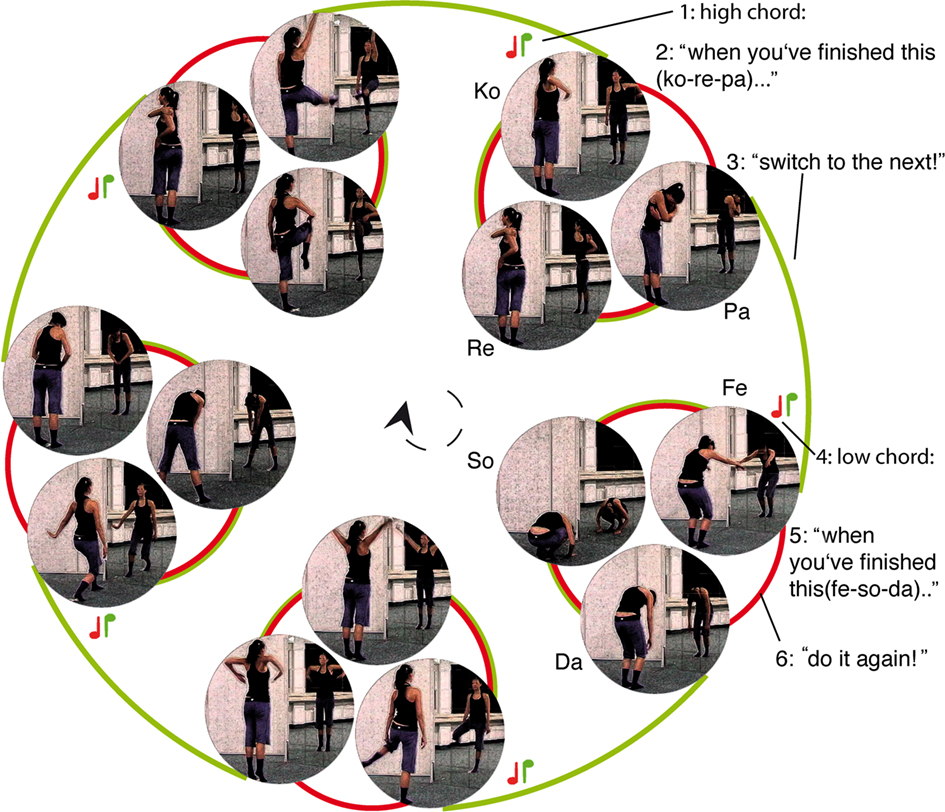
Figure 1. Movement sequence with depiction of respective sub-movements; the cue presented at each movement onset, determines the transition after completion of respective movement; low tune (red note): repetition of the movement (follows red line); high tune (green note): switch to the next movement (follow the green line). Example: (i) a high chord sounds before ko-re-pa (2), indicating that the next movement must be fe-so-da (3 – a switch must take place); before fe-so-da starts, a low chord sounds (4) indicating that fe-so-da (5) must be followed by fe-so-da (6 repetition).
Schedule
The overall experimental schedule compromised three stages. Participants first had to pass a computer based behavioral experiment (stage 1) to be admitted to training (stage 2). If they completed training successfully, they were allowed to participate in the fMRI experiment (stage 3), which was virtually identical to the initial behavioral probe. The two test sessions and the movement training incorporated the same system of dynamically evolving movement sequences.
Stage 1: Behavioral Probe Session
In the computer task, participants watched a dancer performing according to cue, but occasionally making mistakes. Previous to playing the task, the participants were instructed on the cue-movement associations that rule the task (low chord: repetition; high chord: switch). They received a short training where they could choose either four or eight example movies that contained up to 19 cued movements, before they started the task. The movie of the dancer was displayed in the middle of an otherwise gray computer screen, using the Software Presentation 12.0 (Neurobehavioral Systems, San Francisco, CA, USA). Visual input did not extend further than 5° of visual angle. The movies were stopped in irregular intervals and participants had to indicate by button press, whether the dancer had performed correctly immediately before video offset. That is, participants had to indicate whether the very last movement had been correct, irrespective of possible earlier errors. Questions were indicated by a question mark (“?”) displayed in font size 24 for 1500 ms or until the first response. Participants had to press the arrow-to-the-left key (index finger) if they judged the last movement to have been according to cue or press the arrow-to-the-right key (middle finger) if they thought the movement had not been according to cue. Responses had to be given within a timeframe of 1500 ms and were followed by a valid feedback for 400 ms indicating correct, incorrect or delayed responses (“+”/“−”/“0”; Figure 2).
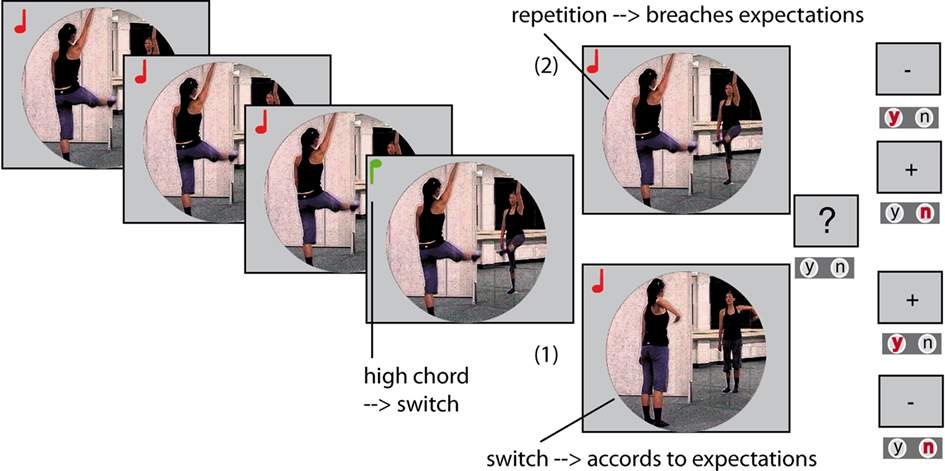
Figure 2. Example for two alternative task-developments: Each picture is one movement within a movie, the notes signify high or low chords. The actress could either perform the last movement before offset correctly (1), meaning the participants would have to judge the performance as correct by index finger button press (“y”). Or the last movement was not according to cue, a breach of expectation (2); the participants would then have to answer by middle-finger button press (“n”) to judge the dancer’s behavior as incorrect. Errors’ during the movie where not to be judged upon. Movie offset and thus relevance of the error to the task was not predictable.
In both the behavioral and the fMRI session, the task encompassed 400 single movements. Thirty-two movements were not according to cue, i.e., the dancer switched to the next movement when a repetition had been cued (16), or a repetition was performed after a switch had been announced (16). Forty breaks disrupted the movie, which was thus divided into 41 videos of varying duration (3–17 movements each). In the behavioral experiment, all 40 breaks were question trials, 20 of them requesting an affirmative answer.
Up to four cues of the same kind, i.e., repetition or switch cues, could appear in a row. At the latest after the fourth identical transition cue (fourth switch or fourth repetition), a dissimilar transition was cued. Across the experiment, four, three, two, and one identical transitions after another appeared equally often. Thus, each cue that was dissimilar from the preceding cues could be differentiated from other first dissimilar cues by the number of preceding transitions that had been identical to each other. For example, a repetition cue could be the first repetition after two switches, or the first repetition after four switches. This randomization was employed to test for the assumptions of the saliency hypothesis (ii). The number of preceding different cues was a measurement of cue saliency against the backdrop of recent cue history.
Stage 2: Movement Training
The participants that passed the 85% criterion of the behavioral experiment subsequently received six 1-h movement-training sessions within 10 days in order to establish a routine-like training stage for the cued performance of movement sequences. Training sessions were conducted in a small dance hall, one side walled with a mirror. During the first session, participants were taught the strict order of the five encompassed movements and learnt accurate performance of the single movements and the associated movement names. To that end, they were allowed to watch a model performing the respective movement on a laptop screen as often as they liked. Once the trainers were satisfied with accuracy of movement performance, participants were verbally instructed to conduct movement sequences, starting each movement when it was called out to them. In their second training session, participants learnt to move according to the cues. They started with a two-cue sequence. For example: Participants were told to start with the movement Ko-re-pa, as soon as the first cue sounded. If the cuing chord was low, they performed Ko-re-pa twice. Importantly they had to start the second Ko-re-pa after the second cue had rung. They had to withhold the third movement corresponding to the second cue, as they would only have been allowed to start the movement upon presentation of a third cue. Once every participant mastered this first step, the number of successive movements (cues) was constantly increased. If one or more participants made a mistake, the sequence had to be started from the beginning. This procedure was implemented in every training session forthwith, at the end of which participants mastered up to 18 cues in a row. Importantly, during training, more than four identical transitions in a row were possible. At the same time, participants had to keep moving at a high level of accuracy and trainers would correct them verbally, and, if necessary, by showing the model-video, over the entire course of training. At the end of the last training session, participants were filmed while performing three 15-cue sequences without further assistance (motor probe). During recording, they wore uniform clothing to allow for unbiased assessment of their performance in a later video evaluation.
Stage 3: fMRI Session
In the fMRI session that was scheduled for the day after each participant’s respective last training session, they encountered the same task as in the behavioral probe. Participants lay supine on the scanner. Their head and arms were stabilized using form-fitting cushioning and their hands rested on a rubber foam tablet. On the right hand side, a response panel was mounted and fixed on the tablet. With their right hand index and middle finger resting on two response buttons, participants were able to judge on the correctness of the dancers movements within the same response contingencies as in the behavioral test. They wore earplugs to attenuate scanner noise and received auditory input via headphones. Participants received visual input on a mirror that was built into the head-coil and adjusted individually to allow for a comfortable view of the entire screen. All parameters were identical to the behavioral experiment (stage 1) with the exception that 24 breaks were used for question trials and 16 for null events (empty trials).
Data Acquisition
A 3T Siemens Magnetom Trio scanner (Siemens, Erlangen, Germany) was used in the functional imaging session. In a separate session, prior to the functional MRT, high-resolution 3D T1 weighted whole-brain MDEFT sequences were recorded for every participant (128 slices, field of view 256 mm, 256 × 256 pixel matrix, thickness 1 mm, spacing 0.25 mm). The functional session engaged a single-shot gradient echo-planar imaging (EPI) sequence sensitive to blood oxygen level dependent contrast (28 slices, parallel to the bicommisural plane, echo time 30 ms, flip angle 90°; repetition time 2000 ms; interleaved recording). Following the functional session immediately, a set of T1 weighted 2D-FLASH images was acquired for each participant (28 slices, field of view 200 mm, 128 × 128 pixel matrix, thickness 4 mm, spacing 0.6 mm, in-plane resolution 3 × 3 mm).
fMRI Data Analysis
Functional data were offline motion-corrected using the Siemens motion protocol PACE (Siemens, Erlangen, Germany). Further processing was conducted with the LIPSIA (Lohmann et al., 2001) software package. Cubic-spline interpolation was used to correct for the temporal offset between the slices acquired in one scan. To remove low-frequency signal changes and baseline drifts, a 1/80 Hz filter was applied. The matching parameters (6 degrees of freedom, 3 rotational, 3 translational) of the T1 weighted 2-D FLASH data onto the individual 3-D MDEFT reference set were used to calculate the transformation matrice for linear registration. These Matrices were subsequently normalized to a standardized Talairach brain size (x = 135, y = 175, z = 120 mm; Talairach and Tournoux, 1988) by linear scaling. The normalized transformation matrices were then applied to the functional slices, to transform them using trilinear interpolation and align them with the 3-D reference set in the stereotactic coordinate system. The generated output had thus a spatial resolution of 3 mm × 3 mm × 3 mm (27 mm3).
The statistical evaluation was based on a least-square estimation using the general linear model (GLM) for serially auto-correlated observations. Temporal Gaussian smoothing (4 s FWHM) was applied to deal with temporal autocorrelation and determine the degrees of freedom (Worsley and Friston, 1995). All design matrices were generated by hemodynamic modeling using a γ-function. We conducted the analysis once using only one GLM and once more using three GLMs (triple-GLM hereafter), one for each competing contrast. In the single-GLM approach, the three contrasts were set to compete for variance in one GLM to achieve a thorough model comparison. In the triple-GLM approach, the same whole-brain analyses was conducted with three separate GLMs, in order to not underestimate the effects of the competing alternative hypotheses and give them a more liberal chance to yield potential caudate activity. The onset vectors were modeled in a time-locked event-related fashion, i.e., the duration set to one second. The first derivative was taken into the model to improve model fit for latency effects.
Single-GLM Approach
The events to account for the violation hypothesis (i) were the dancer’s incorrect movements, for the other hypotheses (ii and iii) the modeled events were specific cues. Hence, the model encompassed the following event types: correct movements, incorrect movements, first dissimilar transition cues, switch cues, and repetition cues (see below). The model encompassed null events as an additional vector. The violation hypothesis (i) contrasted invalidly cued switches and invalidly cued repetitions vs. validly cued switches and validly cued repetitions. The saliency hypothesis (ii) parametrically modeled the first dissimilar transition cue after 4, 3, 2, or 1 identical transition cue(s), ascribing the highest activation level (vector amplitude) to the dissimilar successor of four cues identical to each other. (The switch cue in Table 2, for example would have been assigned a vector amplitude of three; the immediately following repetition cue would have been assigned a vector amplitude of one). In addition, to discern whether potential effects would rely more on a first switch after a number of repetitions or first repetition after a number of switches, we modeled these contrasts in the same fashion of increasing vector values separately, too. That is, in one parametric contrast we ascribed a vector amplitude to each first switch according to the number of previous repetitions (saliency of switches contrast); in the other parametric contrast, the vector amplitude of each first repetition accorded to previous switches (saliency of repetitions contrast). The change hypothesis (iii) was modeled by comparing switch cues to repetition cues. Contrast images, i.e., differences of beta-value estimates for the specified conditions, were generated for each participant. All contrast images were fed into a second-level random effects analysis. The group analysis consisted of one-sample t-tests across all contrast images to analyze whether the observed differences between conditions were significantly deviant from 0. Acquired t-values were transformed to z-scores. To correct for false-positive results, an initial z-threshold was set to 2.56 (p < 0.05, one-tailed, uncorrected for multiple comparisons). In a second step, the results were corrected for multiple comparisons at the cluster level, using cluster size and cluster value thresholds that were obtained by Monte-Carlo simulations. The employed significance level was p = 0.05. Hence, the reported activations are significantly activated at p ≤ 0.05, corrected for comparison at cluster level.
Triple-GLM Approach
In the triple-GLM approach the contrasts (i–iii) were calculated from the same events as described above. However, we employed a different GLM for each contrast that encompassed only the events necessary for the contrast and null-events. The GLM for the violation contrast (i) encompassed validly cued switches, validly cues repetition, invalidly cued switches and invalidly cues repetitions, and null-events. The GLM for the saliency contrast (i) encompassed all first dissimilar cues with a vector amplitude reflecting the number of previous identical repetitions and null-events. The GLM for the change hypothesis encompassed all repetition cues and all switch cues. Group analysis and corrections were identical to the single-GLM analysis described above.
Results
Behavioral
Fifteen of 19 volunteers passed the initial behavioral probe at the 85% criterion. All participants completed training and responded correctly to cue – sequences of up to 18 cues. In the fMRI session, 14 out of 15 participants performed to criterion, with a mean rate of 91.1% correct responses, standard deviation (SD) at 5.4%. Mean rate of correct rejections was 91.7% (SD = 7.3%) and that of hits equaled 90.5% (SD = 7.2%). A two-tailed t-test revealed no significant difference between the averages of hits and correct rejections. One participant had to be excluded from further analyses due to insufficient performance (below 2 SDs from mean).
fMRI
Single-GLM Approach
The contrast between movements that deviated from cue and movements that accorded to the previous cue (violation hypothesis, i) yielded significant bilateral activations in the basomedial caudate nucleus (Figure 3) and right medial pallidum, in the habenula, the anterior dorsal insula, mesial frontomedian cortex (Brodmann’s area [BA] 8 and 9), lateral BA 10, and intraparietal sulcus (IPS). Significant lateralized activations were found in left angular gyrus (AG), right posterior superior temporal sulcus (pSTS) and right temporo-parietal junction (TPJ; Figure 4; Table 1).
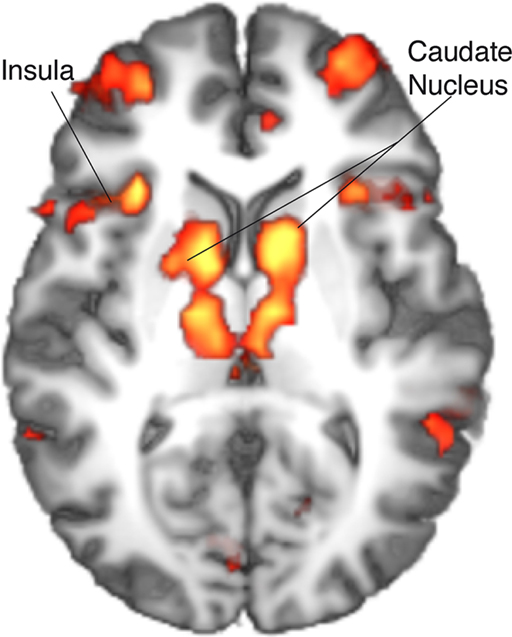
Figure 3. Single-GLM, violation hypothesis (i) contrast: main effect of movements deviating from cue vs. movements accordig to cue; group averaged activations are shown (at z > 3.09) on an axial slice (z = 10) of an individual brain, normalized and aligned to the Talairach stereotactic space. Refer to Table 1 for activation coordinates.
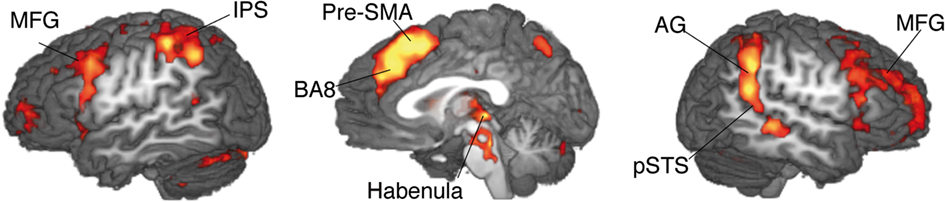
Figure 4. Single-GLM, violation hypothesis (i) contrast: main effect of movements deviating from cue vs. movements according to cue; group averaged activations are shown (at z > 3.09) on sagittal slices (x = −52; 0; 52) of an individual brain, normalized and aligned to the Talairach stereotactic space. Refer to Table 1 for activation coordinates.
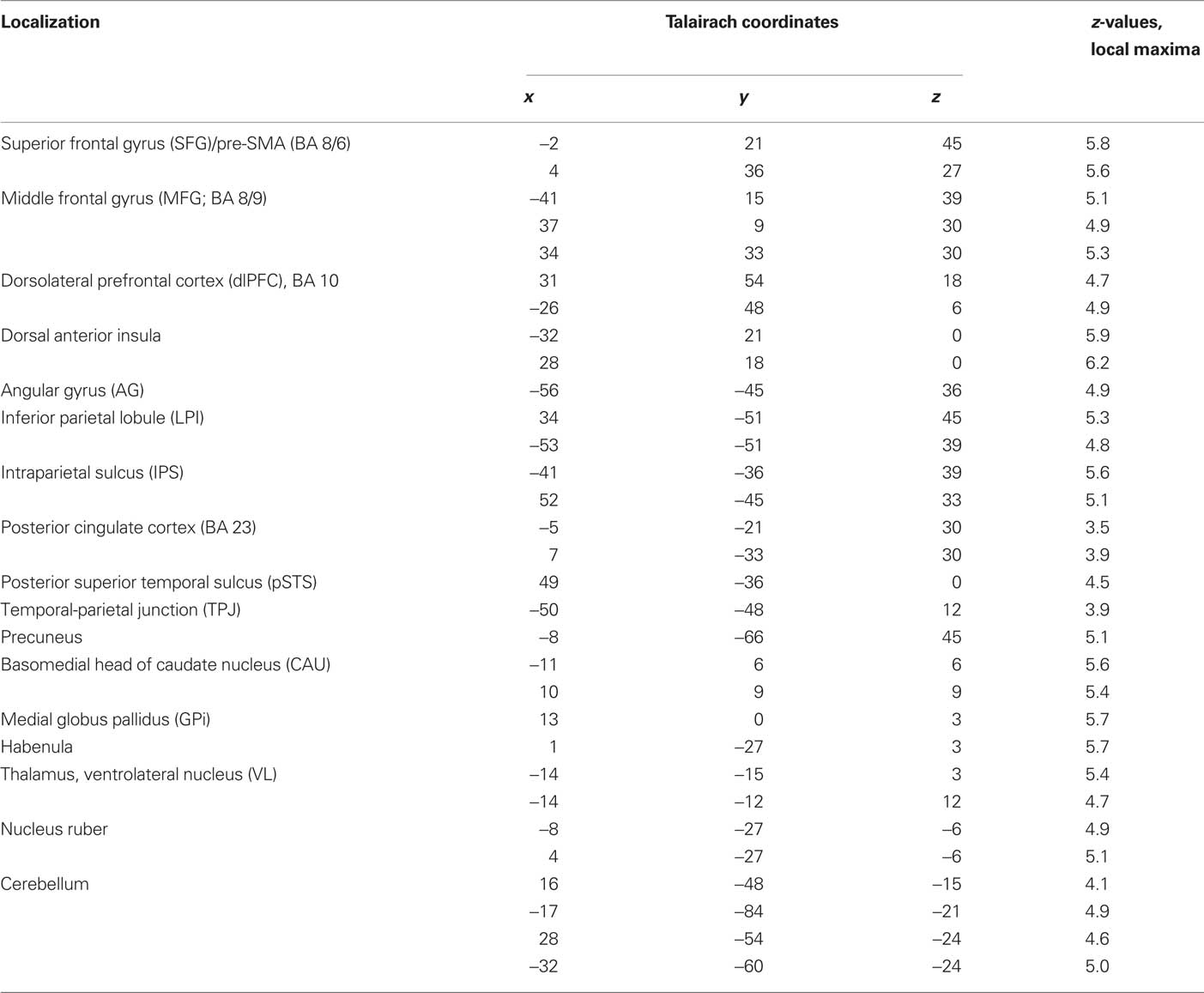
Table 1. Single-GLM, violation hypothesis (i) contrast: Anatomical specification, Talairach coordinates (x,y,z) and maximal z-scores of significantly activated voxels for prediction-violating in contrast to prediction-conform movements.
In the parametric contrasts testing for the saliency hypothesis (ii) only the contrast accounting for activity increase with the number of prior repetitions of identical movements revealed significant activation (saliency of switches contrast). This activity was in supplementary motor area (SMA) and postcentral gyrus. There were no significant correlations with the number of preceding switches. Similarly, there was no significant activation for the general saliency effect, that is number of identical transitions preceding a dissimilar transition, pooled over switches and repetitions. Contrasting switch cues with repetition cues to account for the change hypothesis (iii) yielded the right middle temporal gyrus (MTG) and bilaterally (anterior) IPS. Notably, there was no significant striatal activation, neither in the parametric contrasts relating to the saliency hypothesis (ii), nor in the contrast relating to change hypothesis (iii; Table 2).
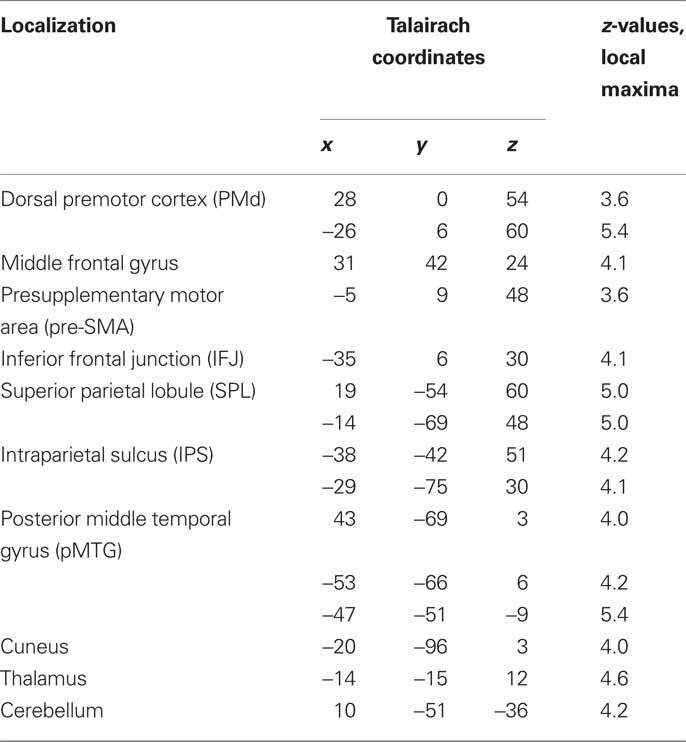
Table 2. Single-GLM, change hypothesis (iii) contrast: Anatomical specification, Talairach coordinates (x,y,z) and maximal z-scores of significantly activated voxels for prediction-violating in contrast to prediction-conform movements.
Triple-GLM Approach
The triple-GLM analysis was employed to calculate the same contrasts as the single-GLM analysis but from three GLMs, optimized for differential effects. This approach yielded caudate activity also only in the violation contrast (i; Table 3; Figure 5). There was no striatal activity either in the saliency (ii) or change contrast (iii). Likewise, cortical activations identified by the violation (i; Table 3, Figures 5 and 6) contrast did not differ largely between the analogous contrasts from the single GLM vs. triple-GLM analyses. The triple-GLM analysis also revealed no significant activity for the saliency (ii) contrast. The parallel change (iii) contrasts from the two analysis approaches revealed quite similar patterns (Tables 2 and 4 for comparison).
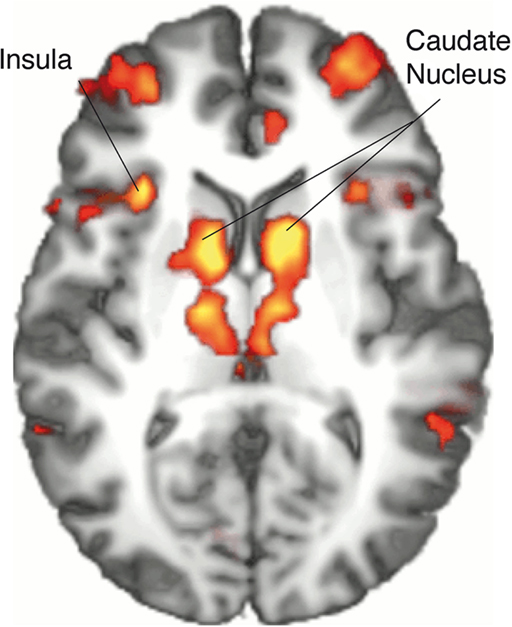
Figure 5. Triple-GLM, violation hypothesis (i) contrast: main effect of movements deviating from cue vs. movements according to cue; group averaged activations are shown (at z > 3.09) on an axial slice (z = 10) of an individual brain, normalized and aligned to the Talairach stereotactic space. Refer to Table 3 for activation coordinates.
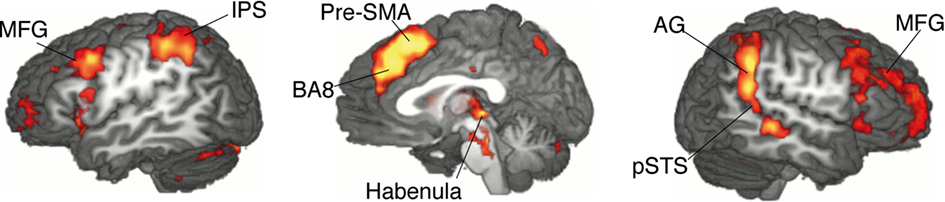
Figure 6. Triple-GLM, violation hypothesis (i) contrast: main effect of movements deviating from cue vs. movements according to cue; group averaged activations are shown (at z > 3.09) on sagittal slices (z = −52; 0; 52) of an individual brain, normalized and aligned to the Talairach stereotactic space. Refer to Table 3 for activation coordinates.
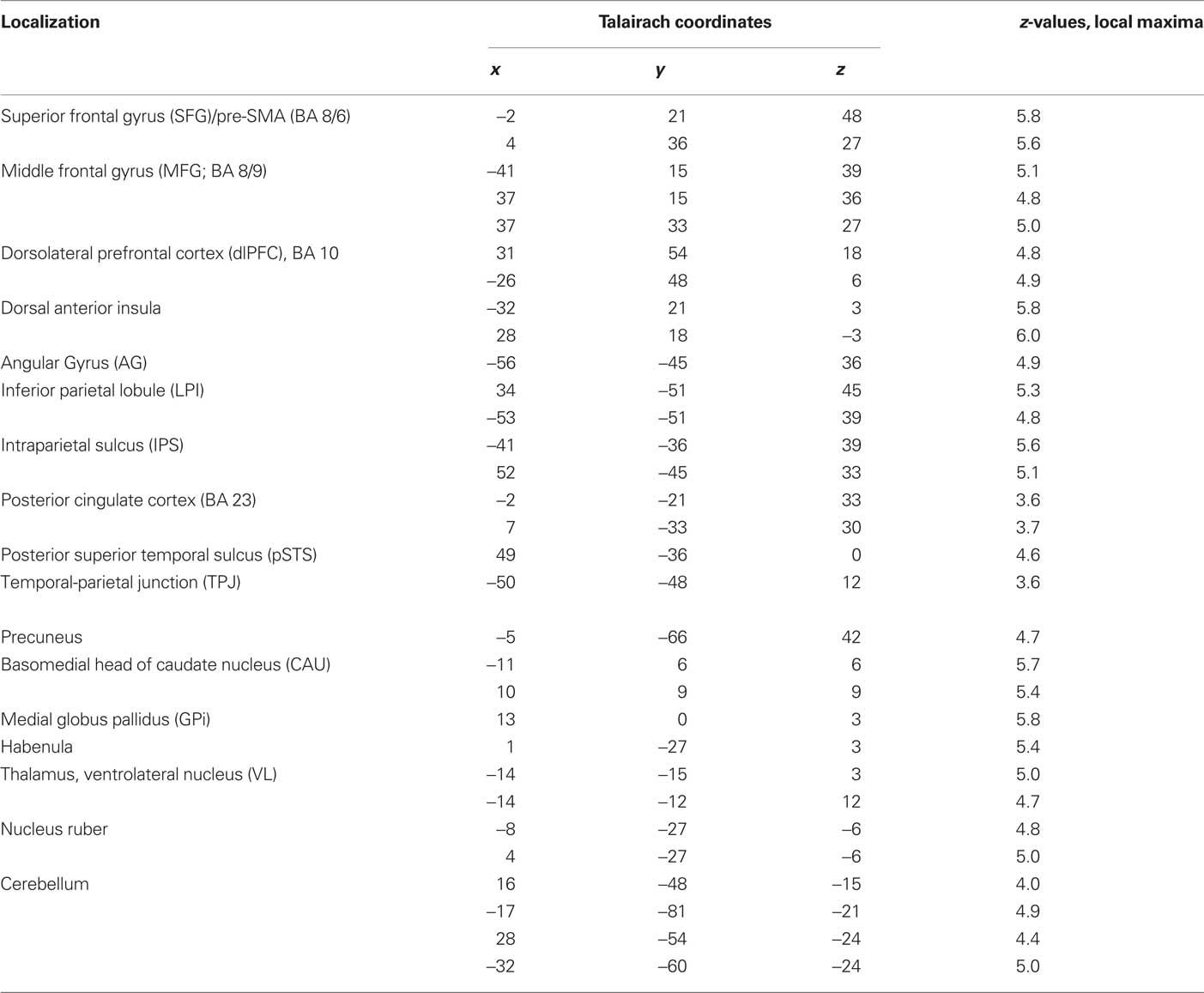
Table 3. Triple-GLM, violation hypothesis (i) contrast: Anatomical specification, Talairach coordinates (x,y,z) and maximal z-scores of significantly activated voxels for prediction-violating in contrast to prediction-conform movements.
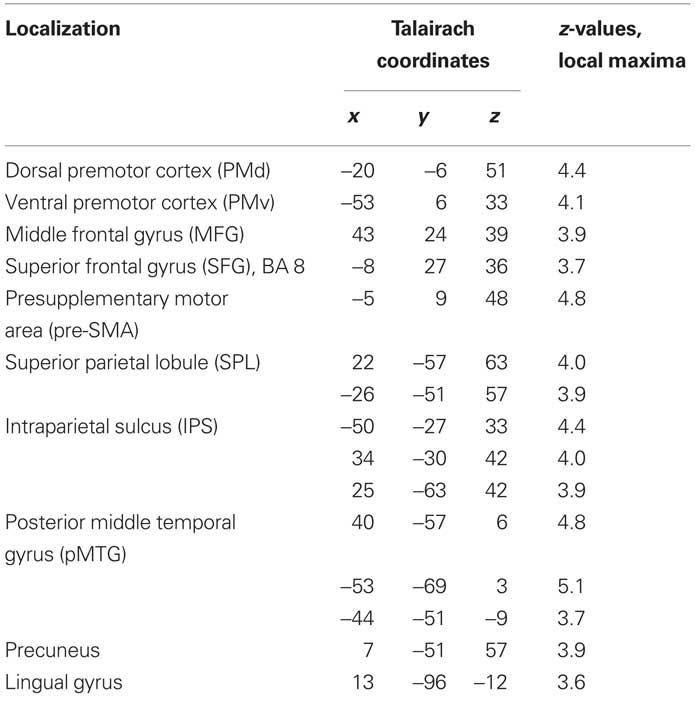
Table 4. Triple-GLM, change hypothesis (iii) contrast: Anatomical specification, Talairach coordinates (x,y,z) and maximal z-scores of significantly activated voxels for prediction-violating in contrast to prediction-conform movements.
Discussion
The present study set out to investigate the role of the caudate nucleus in events that violate predictions (i). In contrast to previous studies, these events were not feedback in an operant conditioning task and involved neither reinforcement nor punishment. Hence, we termed these prediction-violating events “breaches of expectation” to distinguish them from prediction errors conceived as activity dependent on (future) reception of reward. Moreover, we extended the study-design to exclude the possibility that the striatal activity could be a consequence of potential secondary characteristics of violated predictions, that is responses to salient events (ii) and events that provoke a change in behavior (iii).
The contrast accounting for the violation hypothesis (i) yielded activation in the basomedial caudate nucleus. On the contrary, striatal activation was absent in the contrasts that accounted for the saliency (ii) or change (iii) hypotheses, even when we calculated these contrasts from separate optimized GLMs in the triple-GLM approach. This pattern of results suggests the dorsal striatum to be tuned to violations of current predictions rather than to these events’ saliency or implied incite to switch behavior. Moreover, the results show that dorso-striatal responses to violated predictions are not restricted to reinforcement or punishment protocols.
Caudate Nucleus Signals for Breaches of Expectation
The results of the current study suggest that activity in the head of caudate nucleus signals breaches of expectation, i.e., violated predictions, more generally than previously assumed. This finding may explain why this area is often found in trial and error learning, where its activity diminishes once learning has occurred (Jueptner and Weiller, 1998; Delgado et al., 2005; Shohamy et al., 2008; Ruge and Wolfensteller, 2009). Trial and error learning means building up predictions what cues demand which action to gain reward. If the predictions fail, actions have to be altered. If the predictions are fulfilled, the deterministics of the task are apparently understood. Accordingly, the diminution of caudate activity over the course of learning is rooted in the fact that only as long as the rules of the task are unknown (at the beginning of learning), predictions are constantly violated, driving caudate activity. Once the rules have been established, breaches of expectation wane and so does caudate activity. The notion that a breach of expectation signal is generated in caudate nucleus could also account for impairments of Parkinson’s disease patients in trial and error learning (Shohamy et al., 2008). Their compromised breach of expectation signal, due to neostriatal dysfunction, hinders updating wrong beliefs and accordingly adapting behavior. More evidence for a caudate signal for breaches of expectation comes from studies showing that caudate activity ceases the earlier, the easier it is to learn the association between cues and correct actions, i.e., the easier it is to build up operative predictions (Delgado et al., 2005; Koch et al., 2008). The same activity is persistent for cues that are non-informative, and this is for the same reasons, i.e., that they predict that either of two actions could be correct with the same probability. These cues make it impossible to establish reliable predictions (Delgado et al., 2005).
The present findings add to these results in an important fashion, showing caudate nucleus involvement for general breaches of expectation, independent of ensuing feedback, in a movement observation paradigm. Breaches of expectation yielded caudate activity, even if the violated prediction was not a prediction on the availability of reinforcement, but only on the next movement that was to be observed. This finding of a “perceptual prediction error” (Zacks et al., 2007) stands in stark contrast to the aforementioned studies that investigated the caudate prediction error signal in relation to feedback on whether the participants had gained or lost money by their last action (Delgado et al., 2005; Koch et al., 2008; Tricomi and Fiez, 2008) Moreover, as the breaches of expectation in this study reflect perceptual prediction error, it establishes that this perceptual prediction error has a neural correlate in caudate nucleus.
Cortical Areas Co-Active with Caudate Nucleus
We found a number of cortical areas co-activated for the violation contrast, including the right posterior superior temporal sulcus (pSTS) and the adjacent tempo-parietal junction (TPJ) extending into AG. All three cortical regions are connected to the neostriatum by the fronto-occipital fasciculus as well as by the joint fasciculus subcallosal of Muratoff (Schmahmann and Pandya, 2006). This white-matter connectivity points to functionally closely interrelated areas. As the Muratoff bundle directly projects into the dorsal striatum, a fast transmission of perceived deviations to neostriatum is accounted for. Moreover, projections from the AG to caudate nucleus have recently been confirmed by diffusion-tensor imaging in humans (Uddin et al., 2010).
With regard to this connectivity and these regions’ functions as described in the literature, the concurrent activation for the violation contrast is quite plausible. The pSTS is activated when perceiving biological motion and shows enhanced activation for movements that deviate from expectations (Keysers and Perrett, 2004). Adjacent TPJ is involved in predicting the end-state of movements (Arzy et al., 2006) and also in reorienting in space (Blanke et al., 2004; Van Overwalle and Baetens, 2009). Accordingly, pSTS enhancement may indicate the dissimilarity between a covert motor plan in our highly trained subjects and the actually perceived (false) movement. TPJ activation, moreover, may result from perceiving limb trajectories toward end-states that differed from the expected (or even covertly prepared) ones. The spreading of activation into AG fosters the idea put forward by other authors that TPJ, extending into AG, actually responds to breaches of expectation (Vossel et al., 2006; Shulman et al., 2009). These authors employed paradigms where a number of cues signaled where a target would appear with different probabilities. Interestingly, when a cue that indicated a high probability of a certain target position was violated, the resulting activation was higher than for the violation of less predictive cues. It therefore seems as if the more surprising an outcome is, that is, the more it violates a current prediction, the higher is the resultant AG activation (Vossel et al., 2006; Shulman et al., 2009). Besides, the aforementioned studies used abstract stimuli, but employed paradigms demanding reorienting in space (Blanke et al., 2004; Vossel et al., 2006; Shulman et al., 2009; Van Overwalle and Baetens, 2009). The current study employed solely stimuli that represented human motion, but results agree with the literature that the function of reorienting of attention is related to activity in the temporo-parietal junction and posterior parietal cortex (Corbetta and Shulman, 2002). Within this framework, TPJ would be reframed as “circuit breaker,” which still implies the same function of detecting a salient stimulus that deviates from expectations (Corbetta and Shulman, 2002).
It can be suggested that after transmission of the perceived violation of prediction from the temporo-parietal network to the dorsal striatum, a breach of expectation signal is provided by the dorsal striatum that incites the mediation of frontal responses (Ridderinkhof et al., 2004). The consequential frontal network comprised the mesial frontal cortex bilaterally, specifically Brodmann area 8 (BA), the anterior cingulate cortex (ACC) and the presupplementary motor area (pre-SMA), anterior dorsal insula and middle frontal gyrus (MFG). Of those, the anterior dorsal insula, ACC and mesial BA 8 have been implicated in situations characterized by uncertainty (Volz et al., 2003; Wager and Feldman, 2004; Volz, 2005), signifying the likelihood of errors and a need to adapt one’s expectations. In the present study, participants expected to encounter events that would deviate from current predictions, but they lacked information regarding the time-point and frequency of such deviating events. Consequently, there was uncertainty toward the ruling probabilistic of the task.
The involvement of MFG signifies the high impact the task had on working memory (Goldman-Rakic, 1987; Braver et al., 1997). The participants had to judge a movement according to an auditory cue that had preceded the currently presented cue. At the same time they had to register the current cue to predict the next movement. This protocol amounts to a one-back-task. Thus, MFG may reflect active retrieval of the last cue in order to judge whether the caudate-conveyed deviation signal was meaningful or not. Engaging working memory in response to a signaled deviation accords to the assumption that in uncertain situations, PFC explores alternatively operating models (Daw et al., 2005).
Subcortical Breach of Expectation Coding
Apart from caudate nucleus, an important subcortical component of the activity in the violation contrast was the habenula. The habenula codes exclusively for negative prediction errors, and thus corresponds in an antagonistic fashion to the classic reward prediction error (Hikosaka et al., 2008). Unexpected positive reinforcement causes a decrease in habenula activity. Meanwhile, during punishment or reward omission the habenula shows an activity increase (Matsumoto and Hikosaka, 2009). In contrast, caudate activity has been suggested to be different from both opponents in that it is increased in activity for every breach of expectation, regardless its valence (Horvitz, 2000). In the present study, the violations of current predictions did not entail negative consequences. However, making a mistake during behavioral training could well have been ascribed a negative valence. We suggest that as the participants probably engaged in motor imagery to solve the task, exploiting their own memorized experiences during training, seeing the dancer deviating from the protocol was regarded an error with all negative implications (Preston and de Waal, 2001; Decety and Jackson, 2004; Singer et al., 2004). However, the fact that in this study the signal to violated predictions in caudate nucleus is enhanced at the same time that the habenula codes for a negative prediction error, underpins the breach of expectation nature of caudate activity. We find an activity increase for events that are not predicted in the current forward model, not a typical negative prediction error, as the corresponding habenula activity may suggest (Jocham and Ullsperger, 2009).
Predictions, Deviations, and Learning
In the animal literature, prediction errors are mostly described as resultant from the occurrence or omission of reward (Schultz et al., 1997), thereby related to satisfying or averse (external) stimuli. Prediction errors are defined as decreased activity in the face of omitted reward or punishment. However, the current study revealed evidence for heightened caudate activity toward violated predictions in insufficient or failing forward models, and hence breaches of expectation. Establishing predictions and especially signaling for a breach of expectation may be as important as coding how much reward (e.g., food or money) is available, or how unpredicted primary reward was (Spicer et al., 2007). The limitations of fMRI in proving neurotransmitter involvement do not allow drawing the inference that this caudate activity was based in a dopaminergic response (Düzel et al., 2009). The dopaminergic innervation of the dorsal striatum (see Joel and Weiner, 2000 #197 for a review) and the response of the habenula (Jocham and Ullsperger, 2009) implicate the dopaminergic system, but further studies are needed to decide whether dopamine is involved in not reward related breaches of expectation. New approaches, especially the free-energy principle (Friston, 2010) stress the value of predictive capability per se, i.e., the ability to detect breaches of expectation (Kiebel et al., 2008; Suddendorf et al., 2009; Friston, 2010). This model regards correct predictions as prerequisite for successful interaction with the environment, because recognizing a situation and acting accordingly is a capability owed to the disposal of valid forward models (Kiebel et al., 2008). The according actions may be to the end of satisfying primary needs or an evolved want. A typical aim in a social interaction would be, e.g., to adhere to the arrangement and to the task instruction in an fMRI study that were formerly mutually agreed upon. Operative forward models themselves can be valuable enough to be perceived as rewarding, even though they do not yield primary reward or reinforcement. Consider, for example, the psychological importance of a sense of control. In learned-helplessness paradigms, where animals are not able to predict and avoid punishment, pseudo-depression is a consequence (Seligman and Maier, 1967). Unpredictability is just another facet of non-operative forward-models. To establish operative forward models, breaches of expectation must be registered. If they cease to occur, this can be regarded as evidence that learning, i.e., model adaptation, was sufficient. The sense of accomplishment that goes with feeling in control of a situation provides indeed a powerful motivation to learn. Taken together, breaches of expectation that allow for the generation and improvement of an internal model are of utmost importance to survival, but also psychological wellbeing.
Conclusion
The results of the current study foster the idea that the caudate nucleus signals for occurrence of events that violate the predictions of the operative forward model. This signal is not due to the perception of salient events or the need to change one’s behavior, and it is not based on direct reinforcement or punishment. Frontal activation that we observed may be triggered by this signal from the caudate nucleus and operate to deal with present altered environmental demands; either via update of the current forward model or via assessment of the probability of certain event alternatives.
Conflict of Interest Statement
The authors declare that the research was conducted in the absence of any commercial or financial relationships that could be construed as a potential conflict of interest.
Supplementary Material
The Movies S1 and S2 for this article can be found online at http://www.frontiersin.org/human_neuroscience/10.3389/fnhum.2011.00038/abstract/
References
Alexander, G. E., DeLong, M. R., and Strick, P. L. (1986). Parallel organization of functionally segregated circuits linking basal ganglia and cortex. Annu. Rev. Neurosci. 9, 357–381.
Arzy, S., Thut, G., Mohr, C., Michel, C. M., and Blanke, O. (2006). Neural basis of embodiment: distinct contributions of temporoparietal junction and extrastriate body area. J. Neurosci. 26, 8074–8081.
Badgaiyan, R. D., Fischman, A. J., and Alpert, N. M. (2007). Striatal dopamine release in sequential learning. Neuroimage 38, 549–556.
Blanke, O., Theodor, L., Laurent, S., and Margitta, S. (2004). Out-of-body experience and autoscopy of neurological origin. Brain 127, 243–258.
Braver, T. S., Cohen, J. D., Nystrom, L. E., Jonides, J., Smith, E. E., and Noll, D. C. (1997). A parametric study of prefrontal cortex involvement in human working memory. Neuroimage 5, 49–62.
Bubic, A., Yves Von Cramon, D., and Schubotz, R. I. (2009). Prediction, cognition and the brain. Front. Hum. Neurosci. 5:12. doi: 10.3389/fnhum.2010.00025
Bunge, S. A., Kahn, I., Wallis, J. D., Miller, E. K., and Wagner, A. D. (2003). Neural circuits subserving the retrieval and maintenance of abstract rules. J. Neurophysiol. 90, 3419–3428.
Corbetta, M., and Shulman, G. L. (2002). Control of goal-directed and stimulus-driven attention in the brain. Nat. Rev. Neurosci. 3, 201–215.
Davidson, M. C., Horvitz, J. C., Tottenham, N., Fossella, J. A., Watts, R., Ulug, A. M., and Casey, B. J. (2004). Differential cingulate and caudate activation following unexpected nonrewarding stimuli. Neuroimage 23, 1039–1045.
Daw, N. D., Niv, Y., and Dayan, P. (2005). Uncertainty-based competition between prefrontal and dorsolateral striatal systems for behavioral control. Nat. Neurosci. 8, 1704–1711.
Decety, J., and Jackson, P. L. (2004). The functional architecture of human empathy. Behav. Cogn. Neurosci. Rev. 3, 71–100.
Delgado, M. R., Miller, M. M., Inati, S., and Phelps, E. A. (2005). An fMRI study of reward-related probability learning. Neuroimage 24, 862–873.
den Ouden, H. E., Danizeau, J., Roiser, J., Friston, K. J., and Stephan, K. E. (2010). Striatal prediction error modulates cortical coupling. J. Neurosci. 30, 3210–3219.
den Ouden, H. E., Friston, K. J., Daw, N. D., McIntosh, A. R., and Stephan, K. E. (2009). A dual role for prediction error in associative learning. Cerebr. Cortex 19, 1175.
Düzel, E., Bunzeck, N., Guitart-Masip, M., Wittmann, B., Schott, B. H., and Tobler, P. N. (2009). Functional imaging of the human dopaminergic midbrain. Trends Neurosci. 32, 321–328.
Friston, K. (2010). The free-energy principle: a unified brain theory? Nat. Rev. Neurosci. 11, 127–138.
Goldman-Rakic, P. S. (1987). “Circuitry of primate prefrontal cortex and regulation of behavior by representational memory,” in Handbook of Physiology – The Nervous System, Vol. 5. eds F. Plum and V. Mountcastle (Bethesda), 373–417.
Grahn, J. A., Parkinson, J. A., and Owen, A. M. (2008). The cognitive functions of the caudate nucleus. Prog. Neurobiol. 86, 141–155.
Graybiel, A. M. (2005). The basal ganglia: learning new tricks and loving it. Curr. Opin. Neurobiol. 15, 638–644.
Hikosaka, O., Sesack, S. R., Lecourtier, L., and Shepard, P. D. (2008). Habenula: crossroad between the basal ganglia and the limbic system. J. Neurosci. 28, 11825–11829.
Horvitz, J. C. (2000). Mesolimbocortical and nigrostriatal dopamine responses to salient non-reward events. Neuroscience 96, 651–656.
Jocham, G., and Ullsperger, M. (2009). Neuropharmacology of performance monitoring. Neurosci. Biobehav. Rev. 33, 48–60.
Joel, D., and Weiner, I. (2000). The connections of the dopaminergic system with the striatum in rats and primates: an analysis with respect to the functional and compartmental organization of the striatum. Neuroscience, 96, 451–474.
Jueptner, M., and Weiller, C. (1998). A review of differences between basal ganglia and cerebellar control of movements as revealed by functional imaging studies. Brain 121, 1437–1449.
Keysers, C., and Perrett, D. I. (2004). Demystifying social cognition: a Hebbian perspective. Trends Cogn. Sci. 8, 501–507.
Kiebel, S. J., Daunizeau, J., and Friston, K. J. (2008). A hierarchy of time-scales and the brain. PLoS Comput. Biol. 4:e1000209. doi: 10.1371/journal.pcbi.1000209
Kilner, J. M., Friston, K. J., and Frith, C. D. (2007). Predictive coding: an account of the mirror neuron system. Cogn. Process 8, 159–166.
Koch, K., Claudia, S., Gerd, W., Reichenbach, J. R., Sauer, H., and Schlösser, R. (2008). The neural correlates of reward-related trial-and-error learning: an fMRI study with a probabilistic learning task. Learn. Mem. 15, 728–732.
Lohmann, G., Karsten, M., Volker, B., Heiko, M., Sven, H., Lin, C., Zysset, S., and Yves von Cramon, D. (2001). Lipsia – a new software system for the evaluation of functional magnetic resonance images of the human brain. Comput. Med. Imaging Graph 25, 449–457.
Matsumoto, M., and Hikosaka, O. (2009). Representation of negative motivational value in the primate lateral habenula. Nat. Neurosci. 12, 77–84.
O’Doherty, J. P., Buchanan, T. W., Seymour, B., and Dolan, R. J. (2006). Predictive neural coding of reward preference involves dissociable responses in human ventral midbrain and ventral striatum. Neuron 49, 157–166.
Oldfield, R.C. (1971). The assessment and analysis of handedness: the Edinburgh inventory. Neuropsychologia, 9, 97–113.
Preston, S. D., and de Waal, F. B. (2001). Empathy: its ultimate and proximate bases. Behav. Brain Sci. 25, 1–71.
Redgrave, P., and Gurney, K. (2006). The short-latency dopamine signal: a role in discovering novel actions? Nat. Rev. Neurosci. 7, 967–975.
Rescorla, R. A., and Wagner, A. W. (1972). “A theory of Pavlovian conditioning: variations in the effectiveness of reinforcement and nonreinforcement,” in Classical Conditioning II: Current Research and Theory, eds A. H. Black and W. F. Prokasy (New York: Appleton-Century-Crofts), 64–99.
Ridderinkhof, K. R., Ullsperger, M., Crone, E. A., and Nieuwenhuis, S. (2004). The role of the medial frontal cortex in cognitive control. Science 306, 443–447.
Roy, E. A., Saint-Cyr, J., Taylor, A., and Lang, A. (1993). Movement sequencing disorders in Parkinson’s disease. Int. J. Neurosci. 73, 183–194.
Ruge, H., and Wolfensteller, U. (2009). Rapid formation of pragmatic rule representations in the human brain during instruction-based learning. Cereb. Cortex 20, 1656–1667.
Saint-Cyr, J. A. (2003). Frontal-striatal circuit functions: context, sequence, and consequence. J. Int. Neuropsychol. Soc. 9, 103–127.
Schmahmann, J. D., and Pandya, D. N. (2006). Fiber Pathways of The Brain, 1 Edn. New York: Oxford University Press.
Schultz, W., Dayan, P., and Montague, P. R. (1997). A neural substrate of prediction and reward. Science 275, 1593–1599.
Schultz, W., and Dickinson, A. (2000). Neuronal coding of prediction errors. Ann. Rev. Neurosci. 23, 473–500.
Schultz, W., Tremblay, L., and Hollerman, J. R. (1998). Reward prediction in primate basal ganglia and frontal cortex. Neuropharmacology 37, 421–429.
Schutz-Bosbach, S., and Prinz, W. (2007). Prospective coding in event representation. Cogn. Process 8, 93–102.
Seligman, M. E., and Maier, S. F. (1967). Failure to escape traumatic shock. J. Exp. Psychol. 74, 1–9.
Shohamy, D., Myers, C. E., Kalanithi, J., and Gluck, M. A. (2008). Basal ganglia and dopamine contributions to probabilistic category learning. Neurosci. Biobehav. Rev. 32, 219–236.
Shulman, G. L., Astafiev, S. V., Franke, D., Pope, D. L., Snyder, A. Z., McAvoy, M. P., and Corbetta, M. (2009). Interaction of stimulus-driven reorienting and expectation in ventral and dorsal frontoparietal and basal ganglia-cortical networks. J. Neurosci. 29, 4392–4407.
Singer, T., Seymour, B., O’Doherty, J. P., Kaube, H., Dolan, R. J., and Frith, C. D. (2004). Empathy for pain involves the affective but not sensory components of pain Science 303, 1157–1162.
Spicer, J., Galvan, A. G., Hare, T. A., Voss, H., Glover, G., and Casey, B. J. (2007). Sensitivity of the nucleus accumbens to violations in expectation of reward. Neuroimage 34, 455–461.
Suddendorf, T., Donna, R. A., and Corballis, M. C. (2009). Mental time travel and the shaping of the human mind. Philos. Trans. R. Soc. B. Biol. Sci. 364, 1317–1324.
Talairach, J., and Tournoux, P. (1988). Co-planar stereotaxic atlas of the human brain. New York: Thieme.
Tricomi, E., and Fiez, J. A. (2008). Feedback signals in the caudate reflect goal achievement on a declarative memory task. Neuroimage 41, 1154–1167.
Uddin, L. Q., Supekar, K., Amin, H., Rykhlevskaia, E., Nguyen, D. A., Greicius, M. D., and Menon, V. (2010). Dissociable connectivity within human angular gyrus and intraparietal sulcus: evidence from functional and structural connectivity. Cereb. Cortex 20, 2636–2646.
Van Overwalle, F., and Baetens, K. (2009). Understanding others’ actions and goals by mirror and mentalizing systems: a meta-analysis. Neuroimage 48, 564–584.
Volz, K. G. (2005). Variants of uncertainty in decision-making and their neural correlates. Brain Res. Bull. 67, 403–412.
Volz, K. G., Schubotz, R. I., and von Cramon, D. Y. (2003). Predicting events of varying probability: uncertainty investigated by fMRI. Neuroimage 19(2 Pt 1), 271–280.
Vossel, S., Thiel, C. M., and Fink, G. R. (2006). Cue validity modulates the neural correlates of covert endogenous orienting of attention in parietal and frontal cortex. Neuroimage 32, 1257–1264.
Wager, T. D., and Feldman, B. L. (2004). From affect to control: functional specialization of the insula in motivation and regulation. Retrieved from www.apa.org/psycextra/ on 29 March 2011.
Worsley, K. J., and Friston, K. J. (1995). Analysis of FMRI time series revisited – again. Neuroimage 2, 173–181.
Zacks, J. M., Speer, N. K., Swallow, K. M., Braver, T. S., and Reynolds, J. R. (2007). Event perception: a mind-brain perspective. Psychol. Bull. 133, 273–293.
Keywords: caudate nucleus, movement observation, prediction, fMRI, internal model, biological motion, expectation, frontal lobe
Citation: Schiffer A-M and Schubotz RI (2011) Caudate nucleus signals for breaches of expectation in a movement observation paradigm. Front. Hum. Neurosci. 5:38. doi: 10.3389/fnhum.2011.00038
Received: 14 January 2011; Accepted: 27 March 2011;
Published online: 08 April 2011.
Edited by:
Hauke R. Heekeren, Max Planck Institute for Human Development, GermanyReviewed by:
Jeffrey M. Zacks, Washington University, USASimone Schütz-Bosbach, Max Planck Institute, Germany
Copyright: © 2011 Schiffer and Schubotz. This is an open-access article subject to a non-exclusive license between the authors and Frontiers Media SA, which permits use, distribution and reproduction in other forums, provided the original authors and source are credited and other Frontiers conditions are complied with.
*Correspondence: Anne-Marike Schiffer, Motor Cognition Group, Max Planck Institute for Neurological Research, Gleueler Straße 50, 50931 Cologne, Germany. e-mail:c2NoaWZmZXJAbmYubXBnLmRl