- Colder Scientific, McLean, VA, USA
Emulations, defined as ongoing internal representations of potential actions and the futures those actions are expected to produce, play a critical role in directing human bodily activities. Studies of gross motor behavior, perception, allocation of attention, response to errors, interoception, and homeostatic activities, and higher cognitive reasoning suggest that the proper execution of all these functions relies on emulations. Further evidence supports the notion that reinforcement learning in humans is aimed at updating emulations, and that action selection occurs via the advancement of preferred emulations toward realization of their action and environmental prediction. Emulations are hypothesized to exist as distributed active networks of neurons in cortical and sub-cortical structures. This manuscript ties together previously unrelated theories of the role of prediction in different aspects of human information processing to create an integrated framework for cognition.
An Integrated Framework
New scientific findings regularly add to our knowledge of how humans and other animals function in their environments. However, it is often difficult to relate new findings obtained from the different sub-fields of cognitive science, neuroscience, and psychology, because no common theoretical framework easily connects all these areas. For example, we know that the frontal lobes are important for planning, and that mental models are important for human reasoning. These facts may lead us to suspect that mental models and the frontal lobes are closely related, but in the absence of a theory relating the two concepts, it is difficult to design experiments that could shed light on the relationship. More specifically, research into the EEG signals that accompany errors in highly trained pianists (Ruiz et al., 2009) does not have an obvious impact on the interpretation of fMRI results from patients led to expect a less aversive taste than they were given (Nitschke et al., 2006), or on the design of experiments aimed at understanding the brain regions involved in reasoning, such as those conducted by Knauff (2009).
My goal is to present a theoretical framework that offers the promise of relating research in many sub-fields of cognitive science, neuroscience, and psychology, including the findings described in the above paragraph. The framework is based on the concept that cognition is organized around optimizing predictions of environmental and organism state. Various forms of this concept have been previously published (see Llinas, 2001; Grush, 2004; Hawkins and Blakeslee, 2004; Friston and Stephan, 2007; Pezzulo et al., 2008; Bar, 2009; Bubic et al., 2010). There have also been many previous descriptions of the role of prediction in individual aspects of cognition, some of which are reviewed below. This manuscript goes farther by proposing a theoretical framework for information processing that explicitly relates many separately studied components of cognition based on how they operate on predictive representations. It is the common use of predictive representations across perception, motor planning, attention, and learning and higher cognitive reasoning that offers the promise of integrating these fields into a single theory of cognition.
Description of Emulation
Humans and other animals actively participate in shaping their environments. We move through the world to find objects or areas that help us fulfill our goals, and we may take actions to change the objects we encounter. The action planning problem faced by human organisms is made more complex by the fact that planned actions take time to execute, while elements in the environment may be in motion – consider the problem of moving one’s hand to catch a thrown ball. Given delays in action execution, and moving objects in the environment, effective action selection requires some ability to predict the effects of actions in the future (if I put my hand there, I’ll catch the ball when it arrives). One way human bodies and brains might solve this problem is to create representations of what the future might be like given potential actions by the organism, evaluate those future possibilities, and select for execution the action associated with the “best” future state. Animals are also faced with the need to process an enormous amount of sensory stimuli at very high speeds. Representations of the expected future sensory environment could also be useful for reducing this problem of interpreting massive sensory stimuli de novo at every moment to the simpler problem of resolving differences between the predicted and sensed environments. Note that the predicted sensory environment includes the kinematic sensations associated with the positions of the organism’s body parts.
Converging lines of neuroscience and cognitive science research suggest that the importance of “emulations” (from Grush, 2004), which are ongoing representations of potential organism actions and the futures those actions are expected to produce, goes well beyond the action planning and sensory decoding described in the example above. The initial sections of this paper describe theories and evidence originating from distinct lines of scientific inquiry that all separately support the importance of emulations for human cognition. A single framework for cognition that combines the separately developed theories is then presented, followed by a discussion of the possible underlying neural implementation. These sections are followed by a “General Discussion.”
Emulations in Motor Control – Forward Models
Grush (2004) has proposed an information processing theory to explain how animals demonstrate the ability to predict future environmental states and monitor ongoing action. His “emulation theory of representation” holds that Kalman-filter-like controllers utilize an ongoing representation of the future environment (a “forward model”) to select actions that optimize the emulated future. This hypothesis is supported by evidence from studies indicating the necessity of forward models in skeletal movements (Mehta and Schaal, 2002; Diedrichsen et al., 2007; Miall et al., 2007) and smooth pursuit eye movements (Deno et al., 1995; Poliakoff et al., 2004). Other theories of motor control also invoke control systems that minimize an error between incoming sensory information and environmental representations maintained by forward models that are informed by knowledge of ongoing actions (Kawato, 1999; Blakemore et al., 2001; Kawato et al., 2003; Davidson and Wolpert, 2005). In particular, multiple theories of cerebellar function postulate that the cerebellum participates in the maintenance of a forward model that produces the current state of the musculo-skeletal system (Wolpert et al., 1998; Nixon, 2003; Miall et al., 2007). The studies cited and many more provide solid evidence that human motor control utilizes predictions of the environment.
Emulations in Perception – Top-Down Influence
Empirical research into the basis of human perception indicates that perception does not result simply from the passive interpretation of incoming sensory “bottom-up” information (Bruner and Postman, 1949). Human perception appears to be a generative process that combines sensory input with potentially multiple sources of “top-down” information already possessed by the human (Teuber, 1966; Kosslyn and Shin, 1994; Bar, 2003). As hypothesized by multiple authors (e.g., Gross et al., 1999; Grush, 2004; Bar, 2007), the top-down information used in the generative process of perception may include a prediction of the future state of the organism’s environment. The theory of “predictive coding” (Rao and Ballard, 1999) holds that the well-known neurophysiological properties of early visual system neurons reflect “difference signals,” which represent just the differences between the predicted state of the environment (projected down to lower-level visual areas) and the actual visual input.
Recent empirical studies of human behavior report compelling evidence for the role of top-down influences in multi-sensory perception. When subjects were led to expect a less aversive taste then they were given, they rated the taste as less averse, and primary taste cortical activation was less intense then when their expectations were not manipulated (Nitschke et al., 2006). fMRI activity prior to stimulus onset in a cued visual attention task was found to significantly correlate with subject’s behavioral responses to stimuli (Slagter et al., 2006). A recent experiment demonstrating fMRI evidence of an internal sensory prediction when subjects were deciding whether ambiguous stimuli were faces (Summerfield et al., 2006) offers more empirical evidence for predictions forming the basis of top-down influence on perception. These empirical examples support the notion that the perceptual process uses the predictions in emulations as a guide to interpreting actual sensory input.
Other theorists proposing that environmental expectations are used to interpret stimuli have also included planned actions in the expectation (e.g., Kosslyn and Sussman, 1994). In a similar vein, Gross et al. (1999) hold that the process of perception uses input from the senses along with learned knowledge of how the organism interacts with objects in the environment to classify visual input in terms of potential interactions. As described by Gross et al. (1999) since the basic understanding of the sensory input is held in the form of potential future interactions, it is the future state of the environment that is considered. These theoretical contributions are all compatible with the hypothesis that perception depends on emulations.
Emulations in Attention – Exogenous and Endogenous Orienting
Research into mechanisms of attention support an important role for emulations in attentional capture. There are two main channels of attentional capture, endogenous, and exogenous orienting (see Ruz and Lupiáñez, 2002 for a review). As described below, current theories for both of these types of attentional capture rely on expectations of future environmental states. Endogenous, or goal-driven orienting is when a person redirects their attention to a location or object as a part of their ongoing task, like checking for traffic before crossing the street. LaBerge (1995) holds that in endogenous attentional capture, the focus of attention is shifted based on expectations of environmental events. According to this theory, humans focus their attention on locations or stimuli that are expected to be meaningful in the current context. Importantly, context is thought to be useful for directing attention because it provides a basis for realistic expectations. A study by Hayhoe et al. (2005) provides more recent support for this notion. These investigators found that when catching and throwing bounced balls, subjects make predictive head, eye, and hand movements to locations consistent with the subjects’ using an internal model of the future environment.
A study of goal-driven orienting found that subjects performing visual search tasks are optimally biased to detect features of the target that are different from known distractors (Navalpakkam and Itti, 2007). Subject performance in this study was consistent with an attention model that boosted the gain on those target features that best separated targets from the distracting stimuli, suggesting that subjects’ performance on this visual search task depends on expectations of the feature distributions of targets and distractors. Similarly, data on where humans fixate during search indicates that we maintain expectations for target location and use eye movements to efficiently update that expectation (Najemnik and Geisler, 2005).
Models of goal-derived, top-down influence in object recognition that rely on a quickly processed “gist” of the visual scene predict eye movements to regions likely to contain objects given the scene context (Oliva et al., 2003; Torralba et al., 2006). These results suggest that expectations for the visual environment derived from initial, fast, incomplete processing of visual information provide top-down modulation for later activity in attention-guided visual tasks.
The literature discussed above indicates that top-down, endogenous effects on attentional capture are tightly tied to predictions of the environment. The same is true of exogenous orienting. Exogenous orienting occurs when attention is involuntarily drawn to stimuli that are not the focus of the current task. This type of bottom-up attentional capture is primarily due to stimulus features, although top-down modulation may occur (Ruz and Lupiáñez, 2002).
The “surprise-attention hypothesis” (Meyer et al., 1991, 1997; Horstmann, 2006), states that exogenous orienting is caused by surprising stimuli that capture attention. Surprising stimuli are defined as those that highlight a discrepancy between the actual environment and the current set of expectations. This hypothesis is supported by the findings that attentional capture by an unexpected color singleton was due to the mismatch in expectations for the color of the stimulus (Horstmann, 2005). Further evidence indicating that attention is drawn by unpredicted changes to the environment comes from psychophysical studies validating a computer model of human eye movements (Itti and Baldi, 2006). The model changes its point of focus to “surprising” regions, which are visual regions that contain unexpected information. Predictions made by the model are a good fit for human eye movements over the same visual stimuli.
This brief review of exogenous orienting supports the concept that this type of attentional shift may be caused by violations of the predictions contained within emulations. Thus, theories of both exogenous and endogenous orienting are compatible with the proposal that emulations play an important role in selecting the focus of attention.
The Neurophysiology of Emulation Errors
Thus far we have reviewed evidence suggesting that emulations are involved in directing attention, interpreting incoming stimuli, and planning and executing actions in a dynamic environment. The use of emulations is highly adaptive because it can enable rapid recognition even when given minimal sensory input. Emulations can also allow effective planning of actions that take time to complete. However, a perception–action system that relies on predictions will perform poorly if those predictions are incorrect. Neurophysiological evidence indicates that humans possess one or more specific mechanisms to deal with detections of emulation errors.
Error-Related Negativity
The error-related negativity (ERN) is an EEG evoked potential that is produced when subjects make errors. ERNs are typically seen in reaction time tasks that require a speedy response from the subject. It is a negative potential that begins 50–100 ms after the error is made (Falkenstein et al., 1991; Gehring et al., 1993; see Taylor et al., 2007 for a review), and its origin has been well-localized to the medial frontal cortex around the dorsal anterior cingulate cortex (ACC; Dehaene et al., 1994). Two earlier competing theories put forth to explain the generation of the ERN disagreed over whether it reflected neural process responsible for detecting errors (Holroyd and Coles, 2002) or for detecting conflict (Carter et al., 1998; Botvinick et al., 2001). More recently, multiple authors (Luu and Pederson, 2004; Oliveira et al., 2007) have suggested that both of these viewpoints might be consolidated into the hypothesis that an ERN is produced when a subjects’ internal model of the environment has been proven incorrect.
Luu and Pederson (2004) have proposed that the ERN is produced by the activation of processes that result from violations in the representation of context. Similarly, Botvinick et al. (2004) suggests that the ACC might have a general role in monitoring and evaluating action outcomes. In this schema, ERN generation may occur when action outcomes do not match internal predictions. These theories are entirely compatible with the generation of the ERN by neural processes responsible for detecting errors in emulations.
Recent evidence supporting the role of ACC in generation of neural signals indicating a departure from expectation comes from a study of accomplished pianists (Ruiz et al., 2009). Approximately 50–70 ms before these subjects made an error while playing fast, complex pieces, a negative EEG signal was recorded from mesial frontal electrodes. Since the neurophysiological correlate appears before any auditory feedback of the error exists, Ruiz et al. (2009) conclude that the EEG signal may index the error signal of a forward model guiding movement. Positive feedback can also generate ERN-like neurophysiological signals (Holroyd et al., 2008). Oliveira et al. (2007) specifically tested the hypothesis that the ACC is part of system that detects deviations from expectations, and found that both errors and unexpected positive outcomes produced feedback-related negativities (FRNs) that appear very similar to the ERN.
The evidence presented in this section suggests that the ERN and related neurophysiological signals generated by the ACC are produced by a neural mechanism that detects sensory mismatches from internal predictions.
Reinforcement Learning Corrects Emulations
The neurophysiological responses described above are proposed to result from neural processing that recognizes errors in emulations. Further evidence outlined in Holroyd and Coles (2002) implicates the neural processing underlying the ERN and a similar EEG event, the medial frontal negativity (MFN), in the correction of emulation errors.
Research into learning at the single neuronal level suggests that dopamine-containing neurons in the ventral tegmental area and the substantia nigra pars compacta, send a reinforcement learning signal throughout the cortex (Schultz et al., 1993). These neurons are collectively termed the mesencephalic dopamine system (MDS). Investigation of the time course of excitation in these neurons indicates that their activity is most closely tied to errors in predictions of reward (Schultz et al., 1997; Bayer and Glimcher, 2005). According to Holroyd and Coles (2002), the error-processing function that involves the ERN and the reinforcement learning function attributed to the MDS both come into play when the organism must accommodate information that does not match expectations. They hypothesize that the ERN is generated in the ACC by a reinforcement learning signal from the MDS.
Support for this proposed relationship between the ACC, the ERN, MFN, and FRN and reinforcement learning comes from multiple recent empirical investigations. Monkeys with lesions of the ACC are unable to integrate reward information over time to form optimal long-term behavioral strategies (Kennerley et al., 2006). fMRI activity in the ACC is related to the predictability of the task and reward, and the degree to which ACC activity was related to task predictability was correlated with subject learning rate throughout the task (Behrens et al., 2007). The magnitude of the recorded ERN or FRN can predict later changes in behavior indicating that learning has occurred (Yasuda et al., 2004; Frank et al., 2005; Cohen and Ranganath, 2007). The Cohen and Ranganath (2007) study also found that the magnitude of the FRN was larger over the motor cortical region generating the changed behavior, further advancing the notion that the ERN and FRN are signals arising from a system involved in reinforcement learning, and that the signals may be produced by a neural mechanism acting to change the motor cortex representation of the task movement.
These results suggest that the ERN, FRN, and MFN are produced by a reinforcement learning signal from the MDS (Holroyd and Coles, 2002). If the ERN reflects activity from a system that is designed to correct emulations, as outlined in the preceding section, then this evidence also supports the proposal that reinforcement learning corrects emulations.
Emulation in Homeostasis
As described above, the maintenance of predictions of external environmental state is highly adaptive because it allows the organism to rapidly recognize external objects, and execute action plans for optimal future effect. Humans may similarly use emulations of their internal state to regulate bodily homeostasis. Just as a Kalman-filter-like controller can optimize goal-directed motor activity, the ability to respond to expected disruptions in homeostasis can minimize variations away from an optimal state. This ability would allow the organism to learn how to avoid disruptive states and rapidly respond to unavoidable disruptions.
The role of the insula in signaling impending aversive body states is prominently featured in an integrative theory of the neuroscientific basis of anxiety (Paulus and Stein, 2006). These authors propose that one role of the insula is to integrate information about the salience (appetitive and aversive) of external stimuli with bodily state information to form predictions of future body state, and that the insula produces a neural signal when deviations from an optimal homeostatic state are expected. They go on to suggest that heightened activity in the anterior insula may be associated with an exaggerated expected body state in anxious individuals.
Physiological experiments indicate that the insular cortex maintains an internal representation of the body in humans (Critchley et al., 2004), and activation of the insula is modulated by risk prediction and risk prediction errors (Preuschoff et al., 2008). The anterior insula is activated by anticipation of painful stimuli (Ploghaus et al., 1999) and anticipation of touch, with the magnitude of activation related to the reported subjective intensity of touch (Lovero et al., 2009). Anatomical evidence also strongly supports the idea of a bodily representation in the dominant (right) anterior insular cortex (Craig, 2002).
As reviewed above, ERN amplitudes appear to be related to the degree of learning that occurred, suggesting a role for the ERN in reinforcement learning (Holroyd and Coles, 2002). Paulus and Stein (2006) further propose that in anxious people, pathologically incorrect anticipation of negative outcomes in the insula may generate an (incorrect) error signal in the ACC. These hypotheses may be combined in the idea that negative expectations detected by the anterior insula may generate ERNs in the ACC that result from reinforcement learning.
Recent studies provide evidence upholding different parts of the combined hypothesis. The link between anticipation of aversive body state and the ACC and ERN is brought out in measurements of ERN’s in subjects of varying anxiety (Compton et al., 2007). ERN amplitude was smaller for anxious people viewing aversive pictures (angry faces), and greater for anxious people viewing happy faces. Compton et al. (2007) propose that anxious subjects have different expectations for the effect of stimuli on bodily state, and interpret their results as indicating that ERN amplitude is affected by violations of expectations. The relationship between insula activation and learning is indicated by an fMRI study showing that the magnitude of right anterior insula activation in anticipation of losses was correlated to the degree of learning displayed when subject’s behavior was retested months later (Samanez-Larkin et al., 2008). A review and meta-analysis by Knutson and Greer (2008) similarly found that loss anticipation evoked relatively greater activation in anterior insula, and that anterior insula activation predicted choices made to avoid losses.
Thus it appears as though the right anterior insular cortex may trigger reinforcement learning by signaling the anticipation of disruptions to homeostasis. Since negative expectations for the internal environment seem to produce an error signal similar to that generated when predictions for the external environment do not match sensory stimuli, emulation of the organism’s internal state may default to a prediction of healthy organism function within normal homeostatic limits.
Emulations and Higher Cognitive Function – Mental Models
Humans and animals process information in many ways, and for many purposes. Dual-process theories (Schneider and Shiffrin, 1977; see Evans, 2008 for a recent review) state that most human activities depend on fast, automatic, unconscious information processing. These rapid processes are said to make up System 1 cognition, and they include the cognitive activities hypothesized in the above sections to depend on emulations. A separate process, System 2, is held to be responsible for slower, conscious thought such as explicit reasoning through complex problems. Here I describe evidence suggesting that this second system for conscious deliberation also operates using emulations.
Mental model theory (Johnson-Laird, 1980, 1983; Johnson-Laird and Byrne, 2009) holds that humans reason their way through difficult problems using “mental models.” Mental models are described as “possibilities,” and are derived from Craik’s (1943) concept that the mind predicts events using small-scale models of reality. The concept of cognition as emulation is thus compatible with mental model theory if mental models are multifaceted emulations that are imagined and analyzed during reasoning tasks.
Empirical evidence suggesting that mental models of this type play a role in higher-level cognition comes from experiments in mechanical reasoning. The time it takes for subjects to mentally rotate a three-dimensional objects is proportional to the amount of rotation (Shepard and Metzler, 1971). A similar result was found for subjects guessing the rate of rotation of interlocking gears (Schwartz and Black, 1996). Hegarty (2004) provides a review of the role of simulation in constructing and operating on mental models used for mechanical reasoning.
Barbey et al. (2009) propose that higher-level cognition depends on mental models that take the form of activated associative memories that are used for planning. Similarly Gilbert and Wilson (2007) hold that humans predict their emotional reaction to potential futures using their immediate emotional reaction to simulations, and Moulton and Kosslyn (2009) claim that mental imagery is used to generate predictions based on past experience. These theories are compatible with the notion that human reasoning may utilize explicit representations of the future environment that can be tested “off-line,” i.e., in the imagination. Functional imaging evidence supporting this mechanism in humans has been provided in a recent series of papers (reviewed in Knauff, 2009), that demonstrated increased activity in visual cortex during problem steps involving construction and maintenance of representations, but no activity in those areas during a later “reasoning” stage. Thus, a body of theory and evidence supports an important role for environmental predictions, in the form of mental models, in human higher cognitive reasoning.
Cognition Means Creating, Maintaining, and Selecting Potential Futures
The preceding sections describe evidence suggesting that many aspects of cognition rely upon emulations. This section integrates those observations into a theory of cognition. I suggest that at a high level, cognition is carried out via a number of constantly ongoing, concurrently running control processes, as shown in Figure 1. Put simply, the theory is that all of human information processing is organized around creating and maintaining accurate emulations. The following paragraphs describe the different aspects of the theory in more detail.
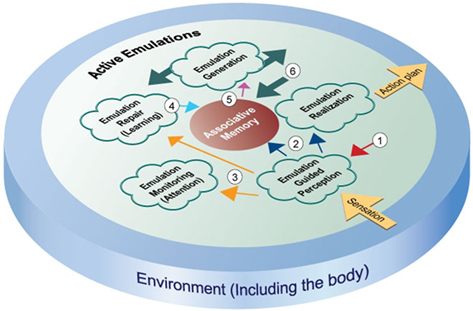
Figure 1. This figure depicts an emulation-based framework for cognition. The constituent control processes that make up the framework create and operate on emulations. The control processes are described in detail in the manuscript text. The figure shows the control processes operating in a pool of active emulations. Thick arrows represent the movement of emulations out from the emulation generation process into the active pool. The meanings of the numbered arrows are given below. (1) Sensory expectations – the red arrow depicts expectations in active emulations that are fed back to lower-level sensory regions, creating a bias in those regions to quickly interpret incoming sensory information in accordance with expectations. (2) Sensory percepts – the purple arrows show how perception affects associative memory and emulation realization. Perceptions of the current environmental state influence the advancement of active emulations, and trigger associations that can generate new emulations. (3) Difference signals – the yellow arrows indicate the use of difference signals in attention and learning. When incoming sensory information differs from the expectations in emulations, difference signals are generated that can trigger increased attention to the unexpected sensations, and reinforcement learning activity to accommodate the new information. (4) Reinforcement learning – the blue arrow depicts the action of learning signals that update associative memory so that future expectations will take current information into account. (5) Actions and associated environmental predictions – the pink arrow indicates the flow of associations used by emulation generation. Action plans and predictions that make up emulations are derived from associations generated by either real or emulated sensory states. (6) Emulation chaining – the thick arrow shows active emulations as input to associative memory. The expected future environment of emulations can trigger associations that generate new emulations, causing chains of emulations to form.
Emulation Generation
Emulations are ongoing representations of actions and predictions of the future environment associated with those actions. Emulations are thus sensorimotor constructs, or put another way, they are representations of actions that include the predicted sensory context. Emulations are generated by incoming sensory stimuli that act as partial cues to trigger the activation of associative memories of similar previous situations, the organism actions associated with those previous situations, and the resulting internal and external environmental states. This explanation for the genesis of emulations generally agrees with multiple hypotheses (Hawkins and Blakeslee, 2004; Schacter and Addis, 2007; Barsalou, 2009; Barbey et al., 2009) that activated long-term memories are used to predict events, and optimal actions are selected using the resulting predictions. Fuster (2006) has also suggested that working memories are long-term memories that have been activated by the current context.
Note that the sensory predictions and actions in emulations need not exactly match the previously occurring actions and environmental states. Since partial cues may retrieve many related memories from neural networks, the predictions and actions in emulations may vary somewhat from actual previous sensorimotor states. The emulations that may be generated by a particular sensory percept are also constrained by the prediction of the internal sensory environment. As described in the Section “Emulation in Homeostasis” above, emulations appear to default to a prediction of healthy organism function.
Emulation Realization – A Theory of Action Selection
Typically, at any given time, many emulations exist within an organism. These simultaneous emulations represent potential futures for the organism. Emulation realization involves evaluation of the emulation’s prediction and preparation for emulation action. This process advances emulations whose environmental predictions are most accurate toward the realization of their potential, including execution of their potential action, and production of their expected future environment. The term “environment” here refers to sensory impressions from both inside (homeostatic information) and outside the body. As described in the Section “Emulation in Homeostasis” above, emulations may default to a prediction of healthy organism function.
This description of emulation realization is similar to the theory of decision-making advanced by Cisek (2006). Cisek (2006) hypothesized that decision-making involves multiple, distributed representations of potential actions that compete for execution. These ideas are supported by neurophysiological evidence indicating that neurons controlling saccadic eye movements initially encode multiple potential actions while the subject is gathering task-relevant information (reviewed in Smith and Ratcliff, 2004). Once enough information is gained to deduce the correct response, these same neurons all represent only the single, correct action. Further evidence consistent with the notion that emulations containing more accurate predictions will advance to execution comes from the finding that cortico-spinal excitability indicating preparation for an action increases when sensory stimuli indicate an increased certainty that the action will be appropriate (Bestmann et al., 2008).
Since emulation realization is an action selection process that advances joint representations of actions and their expected consequences, this proposed process is in agreement with ideo-motor theory (see Stock and Stock, 2004 for a review) and common coding theory (Prinz, 1997), which both emphasize this joint representation. The use of cognitive representations of expected action effects in action planning is separately described as the “action-effect” principle by Prinz (1997).
Emulation-Guided Perception – A Theory of Perception
All of the processes in Figure 1 are proposed to be constantly operating on the information they can access. Specifically, emulation realization operates concurrently with emulation-guided perception. Although there is no temporal order to process operation, the cognitive system does have a directional information flow. An event originating in the (internal or external) environment (e.g., the detection of a predator) enters the system via sensation and first impacts cognition during emulation-guided perception. As described in the Section “Emulations in Perception – Top-Down Influences” above, this process fits raw sensory input to prior expectations of the environment. Along with producing sensory percepts that can generate new emulations and advance matching emulations toward realization, emulation-guided perception also produces “difference signals” (after Rao and Ballard, 1999), describing how sensed reality differs from internally generated predictions.
Emulation Monitoring and Repair – A Theory of Attention and Learning
The emulation monitoring and emulation repair processes are engaged when significantly large difference signals are produced by emulation-guided perception. Emulation monitoring acts to focus attention on the surprising sensory information. Increased attention directs a greater proportion of sensory resources toward those surprising environmental. The resulting increase in information concerning the unexpected environmental event produces new sensory percepts that can adjust the expectations in emulations to reduce future surprise.
Zacks et al. (2007) outlined a theory of human event segmentation (event segmentation theory, or EST) that proposed identical mechanisms (detection of spikes in difference signals leading to increased influence of sensory information) for the detection of event boundaries and the creation of new event context models. Emulations may be a part of the “event models” in EST.
As discussed in the Section “The Neurophysiology of Emulation Errors,” “Reinforcement Learning Corrects Emulations,” and “Emulation in Homeostasis“ ”bove, empirical evidence suggests that specific neural mechanisms are responsible for recognizing errors and engaging reinforcement learning mechanisms. Emulation repair (learning) uses the discrepancy between sensed information and expectations to update associative memory, and reduce the likelihood that errors in emulations are repeated. This process is analogous to updating event schemata in EST.
Emulation Chaining – A Theory of Higher Cognitive Function
As described in the Section “Emulations and Higher Cognitive Function – Mental Models,” experimental results suggest that human deliberative reasoning makes use of emulations. System 2 cognition including complex reasoning and long-term planning may be made possible by the “chaining” of emulations, such that the predicted future of one emulation may serve as the sensory percept triggering the generation of a new emulation. This method of developing and evaluating sequences of possible actions and predictions of their effects is described in Hesslow (2002). Emulation chaining allows organisms to evaluate the potential course of a series of actions by short-circuiting the actual execution of actions, and examining the resulting sensory predictions “off-line” from the real sensory environment. The ability to create and test action sequences before execution allows humans to develop and execute complex, long-term plans.
A Theory of Cognition
The framework laid out in Figure 1 integrates action selection, perception, attention, and learning, and higher cognitive functions into a single theory of cognition. The common use of, and operation on predictive representations supports the exposition of the relationships between information processing modules. The relationships shown in the Figure can be used to explicitly link findings derived from studying each individual cognitive module.
For example, new experimental results revealing details about how forward models are used to plan actions can now also be used to guide further research into how sensory expectations are used to speed perception, or research into the dependence of attention on expectations for the environment. The theoretical framework supports the application of motor planning results to reinforcement learning and vice versa, it supports the use of knowledge about how perception constrains action to frame studies of higher cognitive function, and so on. The integrated theory of cognition provides a common framework capable of relating these previously disparate fields.
Emulations Exist as Active Distributed Networks (Cognits)
This section outlines the possible neurophysiological and neuroanatomical bases for the hypothesized cognitive processes described above. I propose that emulations are stored in, or maintained by, distributed networks of neurons whose discharge rate has increased above baseline. This description of the physiology of active emulations is inspired by studies of working memory. Classic experiments on the physiology of working memory utilized the delayed match-to-sample paradigm to demonstrate that some frontal cortex neurons increased their firing rates during periods when the animal subject had to retain memories during a relatively brief delay period (Fuster and Alexander, 1971). Later experiments found similar results in the parietal (Gnadt and Andersen, 1988) and temporal (Fuster and Jervey, 1982) cortices. These results and others were later interpreted as indicating that the neurons showing increases in firing rate were participating in a distributed network that represented the stored memory updated for the current context (Fuster, 2006). Like working memories, emulations are maintained by distributed networks of activated neurons (“cognits,” from Fuster, 20061). More work remains to clarify the relationship between emulations and working memories.
Furthermore, all cognitive processes operate on single instantiations of emulations. That is to say, a single activated cognit stores both the motor plan and the sensory prediction of the resulting environment. As that cognit advances to realization and execution of its motor plan, the cognit’s prediction of the external environment will aid in the perception of incoming sensory stimuli, and provide a context to guide attention. The cognit may also be part of a group of cognits that together form a chain of potential actions and predicted effects that are being evaluated as a possible complex action plan.
Importantly, the instantiation of the entire emulation is distributed throughout the active cognit. This means that the motor plan is not just stored in the discharge patterns of motor neurons, it is also maintained by the firing of the cognit’s sensory neurons, and vice versa. Thus, an active cognit closely resembles the neural bases of the simulators proposed by Barsalou (2009) as category representations. Evidence supporting the spread of the entire representation over the entire active cognit comes from research describing brain activity modulation in both motor (Platt and Glimcher, 1999; Tobler et al., 2009) and sensory (Shuler and Bear, 2006; Pleger et al., 2008) cortices by changes in the expected reward. If all neurons participating in an active cognit take part in the maintenance of the entire emulation, then changes to the predicted sensory environment, including the expected reward, may be reflected in the discharge rates of all neurons. Widely distributed emulations are also consistent with Teuber’s (1966) hypothesis that the frontal lobes communicate intended actions to sensory regions to assist in the interpretation of incoming sensory information.
The proposal that potential actions and sensory predictions are represented in widely distributed physical instantiations appears to contradict established findings that align brain regions with specific cognitive functions. If motor plans and environmental predictions are distributed throughout cortex, then how do motor and sensory cortical areas differ? The underlying nature of the representation’s instantiation in a neuronal network may hold the answer. The participation of neurons from a particular brain region may be necessary for an emulation to be useful for that region’s purported function, but neurons from that region are just contributors to the entire emulation. Without the context provided by the activity of participating neurons from other brain regions, no single neuron or group of neurons can instantiate a useful representation.
Downing (2009) describes how multiple brain structures might implement prediction, drawing a distinction between procedural, implicit predictions made by the cerebellum and basal ganglia, and declarative predictions made by the hippocampus, thalamus, and neocortex. By contrast, Coull and Nobre (2008) review evidence implicating the basal ganglia in explicit timing tasks, and multiple cortical areas in implicit timing. The orbitofrontal cortex (OFC) may play an important role in generating affective predictions that aid in interpreting incoming stimuli (Barrett and Bar, 2009) and representing the desirability of expected outcomes (Schoenbaum and Roesch, 2005). Schacter and Addis (2009) review evidence implicating the hippocampus and parahippocampal gyrus in creating and storing predictions. The hippocampus and surrounding cortex are part of the brain’s “default network” of brain regions active when the brain is not engaged in a specific task (Raichle et al., 2001; Buckner et al., 2008). One of the functions of the default network may be to create and store environmental predictions (Raichle, 2006; Buckner and Carroll, 2007).
A specific cortical region that may play a role in sustaining representations of the future is area F5 in the ventral premotor cortex (Gallese, 2000; Garbarini and Adenzato, 2004). “Canonical” neurons in this area increase firing not only during the execution of specific actions (e.g., hammering), but also in response to stimuli that are intimately related to that action (e.g., a hammer; Murata et al., 1997). Similarly, “mirror” neurons from this area increase firing during self-action and also when the subject views the same action performed by others (Gallese et al., 1996; Rizzolatti et al., 1996). We would expect to see these increases in firing rates if visual stimuli related to some action triggered the formation of emulations that included that same actions performed by the organism, and area F5 neurons took part in the instantiation of the emulation.
The information reviewed above suggests that the emulation generation process depicted in Figure 1 is implemented by the brain’s default network by tapping into a general cortical associated memory store. Activated networks are distributed throughout the brain, with participation by the cerebellum, basal ganglia, hippocampus, cortex, and thalamus. Neurons from the insula and the OFC also contribute homeostatic and goal information to emulation instantiation. The pool of existing emulations contributes to emulation-guided perception via cortical feedback into lower-level cortical sensory regions. Cortical sensory regions generate the difference signals between the expected and observed external sensation that produce reinforcement learning activity in the mesencaphalic dopamine system and ACC. Similarly, the right anterior insula signals deviations from homeostasis that can produce reinforcement learning activity through similar pathways. Possible anatomical loci for emulation-handling cognitive processes are summarized in Figure 2.
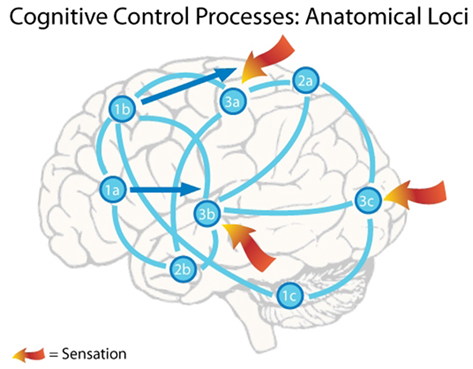
Figure 2. The figure depicts a notional active cognit embodying an emulation. A single emulation is instantiated by active neurons in many locations in the brain. In this case the organism is faced with a complex social situation, trying to break away from a conversation at a party in order to get some food. The emulation stores the possibility of the organism vocalizing his feeling of hunger and moving away from a speaker and toward the area containing food. The following points list the notional active areas and the cognitive control processes their participation embodies. (1) Motor planning – increased discharge rates in these frontal cortical neurons store the emulation’s motor plan. In this case the motor plan consists of both vocalization and movement. Activity in Broca’s area neurons (near 1a) store the vocalization plan, while activity in neurons in the premotor cortex (near 1b) and the cerebellum (near 1c) store the movement plan. If the sensory predictions in this emulation do not deviate too strongly from incoming sensation, the emulation emulation realization will occur as this emulation is advanced toward realization. Advancement of the emulation is enacted by a shift in frontal cortex activity from these association areas along the dark arrows toward the primary motor cortex, eventually resulting in the performance of the stored action. Note that activity from area 1b stores a representation of bodily movement, so that activity shifts to the region of the motor strip that controls the trunk and legs. Similarly, activity from area 1a stores a representation of speech, so that activity moves to the area of the motor strip that controls the mouth. (2) Sensory association – activity patterns in parietal (near 2a) and temporal (near 2b) association areas store higher-level overviews of the expected sensory environment, which is the cessation of speech directed at the organism and the view of movement toward the food. These sensory expectations are communicated to the primary sensory areas (3a,b,c), creating lower-level sensory representations of the expected environment. (3) Primary sensory – activity in primary sensory areas is influenced by both the incoming sensory information and top-down feedback from sensory association areas storing the sensory expectations. The interplay between these two influences produces Emulation-Guided Perception. If incoming information deviates significantly from the top-down expectations, then difference signals (not shown) are sent to the anterior cingulate cortex, triggering an error-related negativity, and emulation repair. The comparison between incoming sensory information and top-down feedback containing sensory expectations is the emulation monitoring process.
Prefrontal Cortex Represents Alternate Futures with Actions
The frontal cortex has long been viewed as playing an important role in planning organism actions. The current proposal indicates that a more precise description of the information processing in frontal cortex is that active neurons in these areas maintain representations of alternate futures for the organism that include upcoming organism actions. This re-description of frontal cortex function was previously suggested by Schubotz (2007). Emulations may often begin with general action plans that can satisfy goals and produce the desired environmental effects. These initial emulations could be contained within networks that include neurons spread throughout frontal cortex. Emulation realization requires more detailed movement planning, and the progressive recruitment of neurons in more caudal regions of frontal cortex into the active distributed network instantiating the emulation, as demonstrated in Figure 2. The movement of active networks toward the primary motor strip occurs while their emulations continue to match incoming sensory data and contain favorable homeostatic information. Chakravarthy et al. (2010) suggest that the basal ganglia are involved in selecting potential actions according to their potential reward. Thus, the basal ganglia may play a key role in gating the caudal movement of active networks toward realization of their emulations.
There is a fine distinction between describing neural activity as taking part in “organism action planning” compared to the current proposal that the frontal cortex “represents alternate futures that include action.” An important difference is that the current proposal implies that frontal cortex activity has a relationship to the future that extends beyond action to include purely sensory aspects of the future environment. Thus, the current proposal accounts for changes in the activity of neurons in frontal regions resulting from incoming environmental stimuli that indicate a different expectation for the future (i.e., “reward cells” in frontal cortex), even in the absence of any change in the motor plan.
Differences in Cognits between System 1 and System 2 Cognition
System 1 cognition operates on a short timescale to aid our navigation through the environment on a moment-by-moment basis. System 2 cognition works in parallel to allows us to formulate and carry out complex plans over a longer timescale. Active networks instantiating both short and longer-term, possibly chained emulations may exist simultaneously, as humans navigate life’s immediate concerns while continuing to work toward long-term goals. Since the final predicted state is generally farther away for System 2 emulations, the details of the final state may be less determined than for System 1 emulations. As a result, active cognits instantiating System 2 emulations may not receive as much participation from lower-level sensory regions compared to cognits for more immediate, System 1 emulations. This testable hypothesis leads to the prediction that greater activation would be seen in primary sensory and motor regions when subjects made plans to execute concrete, immediate tasks (i.e., tell us how you would paint the waiting room), then when they were asked to make high-level plans to accomplish tasks over a longer time period (i.e., tell us how you will prepare for retirement).
Testable Hypotheses
The individual components of the framework outlined in Figure 1 suggest multiple testable hypotheses such as that outlined just above concerning differences in System 1 and System 2 emulations. For instance, the proposed reliance of perception on top-down expectations that are related to specific organism actions suggests that perceptive ability can be modulated by constraining action effects on the environment. If this hypothesis is true, experimental manipulations that prohibited specific classes of organism actions should increase the time to respond to stimuli that would have been produced had the actions been performed. An example paradigm might involve measuring responses to stimuli before and after the elimination of user interface functionality that a subject has learned to associate with those stimuli. Neurophysiological recordings should also reflect changes in brain activity corresponding to the hypothesized reduction in top-down influence.
Another individually testable portion of the proposed framework is the reliance of action planning on the continued correspondence between sensory expectation and ongoing perception. Sufficiently large sensed differences from expectations should cause us to pause our actions, because if current emulations do not contain sensory predictions that are still viable, then no emulations can advance to realization. Accordingly, changing the expected stimuli for subjects that have learned a particular action sequence should introduce delays in the subject’s actions.
The experiments suggested above would serve to test hypotheses about the proposed relation between possible actions and perception. Although the experiments have not been performed, previous authors have advanced the underlying theories (e.g., Prinz, 1997 for the relation of action and perception). This manuscript re-phrases those theories in terms of emulations and places them in a unified framework for cognition.
It is more difficult to design experiments that will produce evidence supporting or refuting the integration of multiple theories of prediction in cognition into a single framework. Obtaining such evidence may require building models based on the framework and comparing model activities to human behavior. Models constructed using the blueprint provided in Figure 1 should not only demonstrate the behavior predicted by the individual theories (e.g., perception and attention are related to potential actions), the models should also process information in a more human-like fashion than other automated agents. For instance, the dependence of perception on expectations will both speed perception and introduce a bias in what is perceived, both of which are human-like traits. Similarly, since differences from expectations produce both a learning signal and the ability to focus attention and sensory resources, instantiations of the framework should be better able to adapt to novel input data than typical machine algorithms. A significant amount of software development must be performed before models based on Figure 1 can be constructed to test these hypotheses.
General Discussion
The role of prediction in cognition has been widely discussed (see Bubic et al., 2010 for a recent review, and Nobre et al., 2007, for a review of temporal prediction). Many authors have focused on how predictions are used in specific cognitive processes, such as perception, action, or attention. A smaller number have emphasized the importance of predictions throughout multiple cognitive processes. Figure 1 of this manuscript presents a theory of how shared operations on predictive representations can be used to integrate the information processing underlying motor planning, perception, attention, and learning, and higher cognitive reasoning into a single framework that is consistent with many previous accounts of the role of prediction in cognition.
Grush (2004) proposed that a Kalman-filter-like mechanism might maintain emulations that were central to motor planning and sensory perception. Grush went on to suggest that these emulations could be a part of mental models that were central to higher cognitive reasoning, and that “visceral emulations” were used to interpret the emotional significance of incoming stimuli. Multiple authors have focused specifically on the role of internal simulations in cognition (Jeannerod, 2001; Hesslow, 2002). Barsalou et al. (2007) consider cognition as a continuous flow of multi-modal predictions, including simulations of actions and their expected results. Similarly Svensson et al. (2009a) have argued that cognition is enacted by internal simulations occurring at multiple sensorimotor levels.
Pezzulo et al. (2008) state that anticipation is an “ordering principle” of cognition. Pezzulo and Castelfranchi (2007) describe a theory of how anticipatory representations evolved from use in error monitoring and sensory interpretation to be utilized as complex goals and expectations that are not anchored in space and time. Multiple aspects of the framework presented in Figure 1 are present in a model of surprise-related processes developed by Meyer et al. (1997). Much like the emulation monitoring and repair processes proposed herein, these authors hypothesized that unexpected events attracted attention and caused updating of the internal schema that drove the violated expectations. Meyer et al. (1997) also stated that surprising events caused a re-appraisal of planned actions, which is consistent with emulation realization.
Another line of theory is centered on the use of sensory predictions for interpreting incoming sensory input (Bar, 2007), and generating signals when actual sensory information does not match expectations (Rao and Ballard, 1999; Friston and Kiebel, 2009). Llinas points out the importance of prediction, especially for directing movement (Llinas, 2001; Llinas and Roy, 2009). Kunde et al. (2007) separated two distinct functions for anticipation in planning and executing actions. Firstly, he stressed the role of anticipating the effects of action in order to select an action plan that satisfied organism goals. His second role for anticipation was in the description of a predicted set of sensory events that would serve as a trigger for action execution. This manuscript builds on the work of these many authors by explicitly integrating multiple cognitive neuroscience hypotheses in a single theoretical framework based on emulations.
Notional Cognitive Architecture
The functional overview of cognitive control processes outlined in Figure 1 suggests a computational architecture that could serve as a blueprint for building machines that process information like humans. Ziemke and colleagues have investigated robot architectures that are based on simulation of actions and the anticipation of environmental consequences (Ziemke et al., 2005; Svensson et al., 2009b), as have Pezzulo and colleagues (Pezzulo et al., 2008; Pezzulo, 2009). Many elements of the notional cognitive control processes depicted in Figure 1 are also implemented in the DiPRA layered agent architecture (Pezzulo, 2009). Actions in DiPRA are a part of sensorimotor schemas that also contain environmental predictions (analogous to emulations). Similar to the proposed process of emulation realization, actions in DiPRA advance toward realization as evidence accumulates that the predictions in their associated sensorimotor schemas are more accurate.
Perception–Action Cycle
Many previous authors describe a “perception–action cycle” (e.g., Arbib, 1981; Fuster, 1985). In contrast, I suggest that cognition is not best understood as a cycle in which cognitive processes occur in a sequence. Specifically there is no time order to the cognitive control processes in Figure 1, they are all occurring simultaneously. Evidence that sensory information is brought to bear on actions in many stages of planning (e.g., Bestmann et al., 2008) supports this view. There is, however, a cyclical flow of information that influences cognitive activities related to specific environmental events, such as when a response is required to receive a reward in a laboratory setting. In these and similar circumstances, when the only cognition that is being measured relates to the environmental cues of the experiment and the actions required in the experimental paradigm, the multiple, concurrently operating control processes outlined in Figure 1 may well have their effects in a fixed sequence, or cycle.
Gathering Information for Action
The “free-energy principle” developed by Friston and colleagues (Friston et al., 2006; Friston, 2009) holds that a goal of human action is to reduce uncertainty in environmental predictions. In a similar vein, multiple theories of gaze allocation suppose that eye movements are directed to locations in the environment where uncertainty is either high (Itti and Baldi, 2006) or especially costly (Sprague et al., 2007; Jovancevid-Misic and Hayhoe, 2009). A recent model of action allows decisions to be made using knowledge of uncertainty in sensory estimation, which supports the role of information-gathering actions (Rao, 2010). The use of actions to increase prediction accuracy fits well with the hypothesized process of emulation realization that advances emulations whose environmental predictions are most consistent with sensed reality.
Memories of the Future
Some current theories of memory propose that memory works both backward in time, enabling recall of past events, and forward in time, enabling prediction of future events (Ingvar, 1985; Schacter and Addis, 2007; Suddendorf et al., 2009). Many authors have also suggested that an important function of memory is to form the basis for future predictions (Hawkins and Blakeslee, 2004; Fuster, 2006; Schacter and Addis, 2007; Barsalou, 2009; Barbey et al., 2009; Hassabis and Maguire, 2009; Suddendorf et al., 2009). By making the long-term associative memory store the source of emulations in Figure 1, the current framework integrates these proposals into a larger theory of human cognition.
Summary
This manuscript reviews evidence suggesting that human bodies work by continuously considering the future. A theoretical framework is laid out in Figure 1 describing how emulations, which are representations of potential actions and the futures they will generate, are used to interpret incoming sensory stimuli, focus attention, learn, and guide action selection. The framework is derived from existing theories in multiple sub-fields of cognitive neuroscience, and ultimately from data collected using a wide variety of experimental paradigms. Evidence bearing on the accuracy of this theory of human information processing may be provided by future efforts to build models derived from the blueprint in Figure 1.
As described earlier, Paulus and Stein (2006) have combined neurophysiological information about the responsiveness of neurons in the anterior insula with psychological findings from studies of anxious individuals, and information on the anatomical connections between the insula and anterior cingulate. These varied scientific results have been bound into a coherent, insightful theory of human anxiety through the use of an assumption that human cognition uses predictions of internal body state. Without the framework provided by the notion that cognition makes use of predictions, the factual underpinnings of the anxiety theory would be difficult to integrate. The emulation-based framework for cognition described in Figure 1 has the potential to similarly enable the integration of theoretical and empirical results from across the cognitive sciences.
Conflict of Interest Statement
The author declares that the research was conducted in the absence of any commercial or financial relationships that could be construed as a potential conflict of interest.
Acknowledgments
I would like to thank Kevin Knowles for providing the artwork for the figures. Julia High, Hal Greenwald, Jeff Colombe, Michelle Carras, Arnold Scheibel, Joaquin Fuster, Cartsen Oertel, and Joeanna Arthur provided helpful comments on earlier versions of this manuscript. Partial funding for this effort was provided by Booz Allen Hamilton and the National Geospatial-Intelligence Agency.
Footnote
- ^Fuster (2006) uses cognits to describe distributed networks of strictly cortical neurons, but I do not restrict the locations of active neurons in cognits to the neocortex.
References
Arbib, M. A. (1981). “Perceptual structures and distributed motor control,” in Handbook of Physiology, Section 2: The Nervous System, Vol. II, Motor Control, Part 1, ed. V. B. Brooks (Bethesda, MD: American Physiological Society), 1449–1480.
Bar, M. (2003). A cortical mechanism for triggering top-down facilitation in visual object recognition. J. Cogn. Neurosci. 15, 600–609.
Bar, M. (2007). The proactive brain: using analogies and associations to generate predictions. Trends Cogn. Sci. (Regul. Ed.) 11, 280–289.
Bar, M. (2009). Predictions: a universal principle in the operation of the human brain. Philos. Trans. R. Soc. Lond. B Biol. Sci. 364, 1181–1182.
Barbey, A. K., Krueger, F., and Grafman, J. (2009). Structured event complexes in the medial prefrontal cortex support counterfactual representations for future planning. Philos. Trans. R. Soc. Lond. B Biol. Sci. 364, 1291–1300.
Barrett, L. F., and Bar, M. (2009). See it with feeling: affective predictions during object perception. Philos. Trans. R. Soc. Lond. B Biol. Sci. 364, 1325–1334.
Barsalou, L. W. (2009). Simulation, situated conceptualization, and prediction. Philos. Trans. R. Soc. Lond. B Biol. Sci. 364, 1281–1289.
Barsalou, L. W., Breazeal, C., and Smith, L. B. (2007). Cognition as coordinated non-cognition. Cogn. Process. 8, 79–91.
Bayer, H. M., and Glimcher, P. W. (2005). Midbrain dopamine neurons encode a quantitative reward prediction error signal. Neuron 47, 129–141.
Behrens, T., Woolrich, M., Walton, M., and Rushworth, M. (2007). Learning the value of information in an uncertain world. Nat. Neurosci. 10, 1214–1221.
Bestmann, S., Harrison, L., Blankenburg, F., Mars, R., Haggard, P., Friston, K., and Rothwell, J. (2008). Influence of uncertainty and surprise on human corticospinal excitability during preparation for action. Curr. Biol. 18, 775–780.
Blakemore, S., Frith, C., and Wolpert, D. (2001). The cerebellum is involved in predicting the sensory consequences of action. Neuroreport 12, 1879–1885.
Botvinick, M., Braver, T., Barch, D., Carter, C., and Cohen, J. (2001). Conflict monitoring and cognitive control. Psychol. Rev. 108, 624–652.
Botvinick, M., Cohen, J. D., and Carter, C. S. (2004). Conflict monitoring and anterior cingulate cortex: an update. Trends Cogn. Sci. (Regul. Ed.) 8, 539–546.
Bruner, J. S., and Postman, L. (1949). On the perception of incongruity: a paradigm. J. Pers. 18, 206–223.
Bubic, A., von Cramon, D. Y., and Schubotz, R. I. (2010). Prediction, cognition and the brain. Front. Hum. Neurosci. 4:25. doi: 10.3389/fnhum.2010.00025
Buckner, R., Andrews-Hanna, J., and Schacter, D. (2008). The brain’s default network: anatomy, function, and relevance to disease. Ann. N. Y. Acad. Sci. 1124, 1–38.
Buckner, R. L., and Carroll, D. C. (2007). Self-projection and the brain. Trends Cogn. Sci. (Regul. Ed.) 11, 49–57.
Carter, C., Braver, T., Barch, D., Botvinick, M., Noll, D., and Cohen, J. (1998). Anterior cingulate cortex, error detection, and the online monitoring of performance. Science 280, 747.
Chakravarthy, V. S., Joseph, D., and Bapi, R. S. (2010). What do the basal ganglia do? A modeling perspective. Biol. Cybern. 103, 237–253.
Cisek, P. (2006). Integrated neural processes for defining potential actions and deciding between them: a computational model. J. Neurosci. 26, 9761–9770.
Cohen, M. X., and Ranganath, C. (2007). Reinforcement learning signals predict future decisions. J. Neurosci. 27, 371–378.
Compton, R. J., Carp, J., Chaddock, L., Fineman, S. L., Quandt, L. C., and Ratliff, J. B. (2007). Anxiety and error monitoring: increased error sensitivity or altered expectations? Brain Cogn. 64, 247–256.
Coull, J. T., and Nobre, A. C. (2008). Dissociating explicit timing from temporal expectation with fMRI. Curr. Opin. Neurobiol. 18, 137–144.
Craig, A. D. (2002). How do you feel? Interoception: the sense of the physiological condition of the body. Nat. Rev. Neurosci. 3, 655–666.
Critchley, H. D., Wiens, S., Rotshtein, P., Ohman, A., and Dolan, R. J. (2004). Neural systems supporting interoceptive awareness. Nat. Neurosci. 7, 189–195.
Davidson, P. R., and Wolpert, D. M. (2005). Widespread access to predictive models in the motor system: a short review. J. Neural Eng. 2, 8313–8319.
Dehaene, S., Posner, M. I., and Tucker, D. M. (1994). Localization of a neural system for error detection and compensation. Psychol. Sci. 5, 303–305.
Deno, D. C., Crandall, W. F., Sherman, K., and Keller, E. L. (1995). Characterization of prediction in the primate visual smooth pursuit system. Biosystems 34, 107–128.
Diedrichsen, J., Verstynen, T. D., Hon, A., Zhang, Y., and Ivry, R. B. (2007). Illusions of force perception: the role of sensori-motor predictions, visual information, and motor errors. J. Neurophysiol. 97, 3305–3313.
Evans, J. S. B. T. (2008). Dual-processing accounts of reasoning, judgment, and social cognition. Annu. Rev. Psychol. 59, 255–278.
Falkenstein, M., Hohnsbein, J., Hoormann, J., and Blanke, L. (1991). Effects of crossmodal divided attention on late ERP components: II. Error processing in choice reaction tasks. Electroencephalogr. Clin. Neurophysiol. 78, 447–455.
Frank, M. J., Woroch, B., and Curran, T. (2005). Error-related negativity predicts reinforcement learning and conflict biases. Neuron 47, 495–501.
Friston, K. (2009). The free-energy principle: a rough guide to the brain? Trends Cogn.Sci. (Regul. Ed.) 13, 293–301.
Friston, K., and Kiebel, S. (2009). Predictive coding under the free-energy principle. Philos. Trans. R. Soc. Lond. B Biol. Sci. 364, 1211–1221.
Friston, K., Kilner, J., and Harrison, L. (2006). A free energy principle for the brain. J. Physiol. Paris 100, 70–87.
Fuster, J. M. (1985). The prefrontal cortex, mediator of cross-temporal contingencies. Hum. Neurobiol. 4, 169–179.
Fuster, J. M. (2006). The cognit: a network model of cortical representation. Int. J. Psychophysiol. 60, 125–132.
Fuster, J. M., and Alexander, G. E. (1971). Neuron activity related to short-term memory. Science 173, 652–654.
Fuster, J. M., and Jervey, J. P. (1982). Neuronal firing in the inferotemporal cortex of the monkey in a visual memory task. J. Neurosci. 2, 361–375.
Gallese, V. (2000). The inner sense of action: agency and motor representations. J. Conscious. Stud. 7, 23–40.
Gallese, V., Fadiga, L., Fogassi, L., and Rizzolatti, G. (1996). Action recognition in the premotor cortex. Brain 119, 593–609.
Garbarini, F., and Adenzato, M. (2004). At the root of embodied cognition: cognitive science meets neurophysiology. Brain Cogn. 56, 100–106.
Gehring, W. J., Goss, B., Coles, M. G. H., Meyer, D. E., and Donchin, E. (1993). A neural system for error detection and compensation. Psychol. Sci. 4, 385–390.
Gilbert, D. T., and Wilson, T. D. (2007). Prospection: experiencing the future. Science 317, 1351–1354.
Gnadt, J., and Andersen, R. (1988). Memory related motor planning activity in parietal cortex of Macaque. Exp. Brain Res. 70, 216–220.
Gross, H., Heinze, A., Seiler, T., and Stephan, V. (1999). Generative character of perception: a neural architecture for sensorimotor anticipation. Neural Netw. 12, 1101–1129.
Grush, R. (2004). The emulation theory of representation: motor control, imagery, and perception. Behav. Brain Sci. 27,377–442.
Hassabis, D., and Maguire, E. A. (2009). The construction system of the brain. Philos. Trans. R. Soc. Lond. B Biol. Sci. 364, 1263–1271.
Hayhoe, M., Mennie, N., Sullivan, B., and Gorgos, K. (2005). “The role of internal models and prediction in catching balls,” in From Reactive to Anticipatory Cognitive Embodied Systems, ed. C. Castelfranchi (Menlo Park, CA: American Association for Artificial Intelligence), 78–82.
Hegarty, M. (2004). Mechanical reasoning by mental simulation. Trends Cogn. Sci. (Regul. Ed.) 8, 280–285.
Hesslow, G. (2002). Conscious thought as simulation of behaviour and perception. Trends Cogn. Sci. (Regul. Ed.) 6, 242–247.
Holroyd, C. B., and Coles, M. G. H. (2002). The neural basis of human error processing: reinforcement learning, dopamine, and the error-related negativity. Psychol. Rev. 109, 679–709.
Holroyd, C. B., Pakzad-Vaezi, K. L., and Krigolson, O. E. (2008). The feedback correct-related positivity: sensitivity of the event-related brain potential to unexpected positive feedback. Psychophysiology 45, 688–697.
Horstmann, G. (2005). Attentional capture by an unannounced color singleton depends on expectation discrepancy. J. Exp. Psychol. Hum. Percept. Perform. 31, 1039–1060.
Horstmann, G. (2006). The time course of intended and unintended allocation of attention, Psychol. Res. 70, 13–25.
Ingvar, D. H. (1985). Memory of the future: an essay on the temporal organization of conscious awareness. Hum. Neurobiol. 4, 127–136.
Itti, L., and Baldi, P. (2006). “Bayesian surprise attracts human attention,” in Advances in Neural Information Processing Systems, Vol. 19 (NIPS*2005), eds Y. Weiss, B. Schölkopf, and J. Platt (Cambridge, MA: MIT Press), 547–554.
Jeannerod, M. (2001). Neural simulation of action: a unifying mechanism for motor cognition. NeuroImage 14, S103–S109.
Johnson-Laird, P. N. (1983). Mental Models: Towards a Cognitive Science of Language, Inference, and Consciousness. Cambridge: Cambridge University Press.
Johnson-Laird, P., and Byrne, R. (2009). Mental Models. http://www.tcd.ie/Psychology/other/Ruth_Byrne/mental_models/ [accessed August 7, 2009].
Jovancevid-Misic, J., and Hayhoe, M. (2009). Adaptive gaze control in natural environments. J. Neurosci. 29, 6234–6238.
Kawato, M. (1999). Internal models for motor control and trajectory planning. Curr. Opin. Neurobiol. 9, 718–727.
Kawato, M., Kuroda, T., Imamizu, H., Nakano, E., Miyauchi, S., and Yoshioka, T. (2003). Internal forward models in the cerebellum: fMRI study on grip force and load force coupling. Prog. Brain Res. 142, 171–188.
Kennerley, S., Walton, M., Behrens, T., Buckley, M., and Rushworth, M. (2006). Optimal decision making and the anterior cingulate cortex. Nat. Neurosci. 9, 940–947.
Knauff, M. (2009). A neuro-cognitive theory of deductive relational reasoning with mental models and visual images. Spat. Cogn. Comput. 9, 109–137.
Knutson, B., and Greer, S. M. (2008). Anticipatory affect: neural correlates and consequences for choice. Philos. Trans. R. Soc. Lond. B Biol. Sci. 363, 3771–3786.
Kosslyn, S. M., and Shin, L. M. (1994). “Visual mental images in the brain: current issues,” in The Neuropsychology of High-Level Vision: Collected Tutorial Essays, eds M. J. Farah, and G. Ratcliff (Hillsdale, NJ: Lawrence Erlbaum), 269–296.
Kosslyn, S. M., and Sussman, A. L. (1994). “Roles of imagery in perception: or, there is no such thing as immaculate perception,” in The Cognitive Neurosciences ed. M. S. Gazzaniga (Cambridge, MA: MIT Press), 1035–1042.
Kunde, W., Elsner, K., and Kiesel, A. (2007). No anticipation- no action. The role of anticipation in action and perception. Cogn. Process. 8, 71–78.
LaBerge, D. (1995). Attentional Processing: The Brains Art of Mindfulness. Cambridge, MA: Harvard University Press.
Llinas, R. R., and Roy, S. (2009). The prediction imperative as the basis for self-awareness. Philos. Trans. R. Soc. Lond. B Biol. Sci. 364, 1301–1307.
Lovero, K., Simmons, A., Aron, J., and Paulus, M. (2009). Anterior insular cortex anticipates impending stimulus significance. Neuroimage 45, 976–983.
Luu, P., and Pederson, S. M. (2004). “The anterior cingulate cortex: regulating actions in context,” in Cognitive Neuroscience of Attention ed. M. I. Posner (New York: Guilford Publication Inc.,), 232–242.
Mehta, B., and Schaal, S. (2002). Forward models in visuomotor control. J. Neurophysiol. 88, 942–953.
Meyer, W., Niepel, M., Rudolph, U., and Schützwohl, A. (1991). An experimental analysis of surprise. Cogn. Emot. 5, 295–311.
Meyer, W.-U., Reisenzein, R., and Schützwohl, A. (1997). Toward a process analysis of emotions: the case of surprise. Motiv. Emot. 21, 251–274.
Miall, R., Christensen, L., Cain, O., and Stanley, J. (2007). Disruption of state estimation in the human lateral cerebellum. PLoS Biol. 5, 2733–2744. doi: 10.1371/journal.pbio.0050316
Moulton, S., and Kosslyn, S. (2009). Imagining predictions: mental imagery as mental emulation. Philos. Trans. R. Soc. Lond. B Biol. Sci. 364, 1273–1280.
Murata, A., Fadiga, L., Fogassi, L., Gallese, V., Raos, V., and Rizzolatti, G. (1997). Object representation in the ventral premotor cortex (area F5) of the monkey. J. Neurophysiol. 78, 2226–2230.
Najemnik, J., and Geisler, W. S. (2005). Optimal eye movement strategies in visual search. Nature 434, 387–391.
Navalpakkam, V., and Itti, L. (2005). Modeling the influence of task on attention. Vision Res. 45, 205–231.
Navalpakkam, V., and Itti, L. (2007). Search goal tunes visual features optimally. Neuron 53, 605–617.
Nitschke, J., Sarinopoulos, I., Mackiewicz, K., Schaefer, H., and Davidson, R. (2006). Functional neuroanatomy of aversion and its anticipation. Neuroimage 29, 106–116.
Nixon, P. (2003). The role of the cerebellum in preparing responses to predictable sensory events. Cerebellum 2, 114–122.
Nobre, A. C., Correa, A., and Coull, J. T. (2007). The hazards of time. Curr. Opin. Neurobiol. 17, 465–470.
Oliva, A., Torralba, A., Castelhano, M., and Henderson, J. (2003). Top-down control of visual attention in object detection. Int. Conf. Image Proc. 1, 253–256.
Oliveira, F., McDonald, J., and Goodman, D. (2007). Performance monitoring in the anterior cingulate is not all error related: expectancy deviation and the representation of action-outcome associations. J. Cogn. Neurosci. 19, 1994–2004.
Pezzulo, G. (2009). DiPRA: a layered agent architecture which integrates practical reasoning and sensorimotor schemas. Conn. Sci. 21, 297–326.
Pezzulo, G., Butz, M., Castelfranchi, C., and Falcone, R. (2008). “Anticipation in natural and artificial cognition,” in The Challenge of Anticipation: A Unifying Framework for the Analysis and Design of Artificial Cognitive Systems, eds G. Pezzulo, M. Butz, C. Castelfranchi, and R. Falcone (New York: Springer), 3–22.
Pezzulo, G., and Castelfranchi, C. (2007). The symbol detachment problem. Cogn. Process. 8, 115–131.
Platt, M., and Glimcher, P. W. (1999). Decision variables represented by parietal neurons during movement choice. Soc. Neurosci. Abstr.
Pleger, B., Blankenburg, F., Ruff, C., Driver, J., and Dolan, R. (2008). Reward facilitates tactile judgments and modulates hemodynamic responses in human primary somatosensory cortex. J. Neurosci. 28, 8161–8168.
Ploghaus, A., Tracey, I., Gati, J., Clare, S., Menon, R., Matthews, P., and Rawlins, J. (1999). Dissociating pain from its anticipation in the human brain. Science 284, 1979–1981.
Poliakoff, E., Barnes, G., and Collins, C. (2004). Target selection for predictive smooth pursuit eye movements. Exp. Brain Res. 155, 129–133.
Posner, M., Snyder, C., and Davidson, B. (1980). Attention and the detection of signals. J. Exp. Psychol. 109, 160–174.
Preuschoff, K., Quartz, S., and Bossaerts, P. (2008). Human insula activation reflects risk prediction errors as well as risk. J. Neurosci. 28, 2745–2752.
Raichle, M., MacLeod, A., Snyder, A., Powers, W., Gusnard, D., and Shulman, G. (2001). A default mode of brain function. Proc. Natl. Acad. Sci. U.S.A. 98, 676–682.
Rao, R. (2010). Decision-making under uncertainty: a neural model based on partially observable Markov decision processes. Front Comput Neurosci 4:146. doi: 10.3389/fncom.2010.00146
Rao, R., and Ballard, D. (1999). Predictive coding in the visual cortex: a functional interpretation of some extra-classical receptive-field effects. Nat. Neurosci. 2, 79–87.
Rizzolatti, G., Fadiga, L., Gallese, V., and Fogassi, L. (1996). Premotor cortex and the recognition of motor actions cognit. Brain Res. 3, 131–141.
Ruiz, M., Jabusch, H., and Altenmuller, E. (2009). Detecting wrong notes in advance: neuronal correlates of error monitoring in pianists. Cereb. Cortex 19, 2625–2639.
Ruz, M., and Lupiáñez, J. (2002). A review of attentional capture: on its automaticity and sensitivity to endogenous control. Psicológica 23, 283–309.
Samanez-Larkin, G., Hollon, N., Carstensen, L., and Knutson, B. (2008). Individual differences in insular sensitivity during loss anticipation predict avoidance learning. Psychol. Sci. 19, 320–323.
Schacter, D., and Addis, D. (2007). The cognitive neuroscience of constructive memory: remembering the past and imagining the future. Philos. Trans. R. Soc. Lond. B Biol. Sci. 362, 773–786.
Schacter, D., and Addis, D. (2009). On the nature of medial temporal lobe contributions to the constructive simulation of future events. Philos. Trans. R. Soc. Lond. B Biol. Sci. 364, 1245–1253.
Schneider, W., and Shiffrin, R. (1977). Controlled and automatic human information processing: I. Detection, search, and attention. Psychol. Rev. 84, 1–66.
Schoenbaum, G., and Roesch, M. (2005). Orbitofrontal cortex, associative learning, and expectancies. Neuron 47, 633–636.
Schubotz, R. (2007). Prediction of external events with our motor system: towards a new framework. Trends Cogn. Sci. (Regul. Ed.) 11, 211–218.
Schultz, W., Apicella, P., and Ljungberg, T. (1993). Responses of monkey dopamine neurons to reward and conditioned stimuli during successive steps of learning a delayed response task. J. Neurosci. 13, 900–991.
Schultz, W., Dayan, P., and Montague, P. (1997). A neural substrate of prediction and reward. Science 275, 1593–1599.
Schwartz, D., and Black, J. (1996). Analog imagery in mental model reasoning: depictive models. Cogn. Psychol. 30, 154–219.
Shepard, R., and Metzler, J. (1971). Mental rotation of three-dimensional objects. Science 171, 701–703.
Shuler, M., and Bear, M. (2006). Reward timing in the primary visual cortex. Science 311, 1606–1609.
Slagter, H., Weissman, D., Giesbrecht, B., Kenemans, J., Mangun, G., Kok, A., and Woldorff, M. (2006). Brain regions activated by endogenous preparatory set shifting as revealed by fMRI. Cogn. Affect. Behav. Neurosci. 6, 175–189.
Smith, P., and Ratcliff, R. (2004). Psychology and neurobiology of simple decisions. Trends Neurosci. 27, 161–168.
Sprague, N., Ballard, D., and Robinson, A. (2007). Modeling embodied visual behaviors. ACM Trans. Appl. Percept. 4, 11.
Suddendorf, T., Addis, D., and Corballis, M. (2009). Mental time travel and the shaping of the human mind. Philos. Trans. R. Soc. Lond. B Biol. Sci. 364, 1317–1324.
Summerfield, C., Egner, T., Greene, M., Koechlin, E., Mangels, J., and Hirsch, J. (2006). Predictive codes for forthcoming perception in the frontal cortex. Science 314, 1311–1314.
Svensson, H., Morse, A. F., and Ziemke, T. (2009a). “Representation as internal simulation: a minimalistic robotic model,” in Proceedings of the Thirty-First Annual Conference of the Cognitive Science Society, eds N. Taatgen, and H. van Rijn (Austin, TX: Cognitive Science Society), 2890–2895.
Svensson, H., Morse, A. F., and Ziemke, T. (2009b). ”Neural pathways of embodied simulation,” in Anticipatory Behavior in Adaptive Learning Systems, ed. G. Pezzulo, M. V. Butz, O. Sigaud, and G. Baldassarre (Berlin: Springer-Verlag), 95–114.
Taylor, S., Stern, E., and Gehring, W. (2007). Neural systems for error monitoring: recent findings and theoretical perspectives. Neuroscientist 13, 162–172.
Teuber, H. (1966). The frontal lobes and their functions: further observations on rodents, carnivores, subhuman primates, and man. Int. J. Neurol. 5, 282–300.
Tobler, P., Christopoulos, G., ODoherty, J., Dolan, R., and Schultz, W. (2009). Risk-dependent reward value signal in human prefrontal cortex. Proc. Natl. Acad. Sci. U.S.A. 106, 7185–7190.
Torralba, A., Oliva, A., Castelhano, M., and Henderson, J. (2006). Contextual guidance of eye movements and attention in real-world scenes: the role of global features in object search. Psychol. Rev. 113, 766–786.
Wolfe, J. (2007). “Guided search 4.0: current progress with a model of visual search,” in Integrated Models of Cognitive Systems, ed. W. Gray (New York: Oxford), 99–119.
Wolpert, D., Miall, R., and Kawato, M. (1998). Internal models in the cerebellum. Trends Cogn. Sci. (Regul. Ed.) 2, 338–347.
Yasuda, A., Sato, A., Miyawaki, K., Kumano, H., and Kuboki, T. (2004). Error-related negativity reflects detection of negative reward prediction error. Neuroreport 15, 2561–2565.
Zacks, J. M., Speer, N. K., Swallow, K. M., Braver, T. S., and Reynolds, J. R. (2007). Event perception: a mind/brain perspective. Psychol. Bull. 133, 273–293.
Keywords: simulation, cognitive architecture, cognitive neuroscience, theory, prediction, context
Citation: Colder B (2011) Emulation as an integrating principle for cognition. Front. Hum. Neurosci. 5:54. doi: 10.3389/fnhum.2011.00054
Received: 19 January 2011;
Accepted: 18 May 2011;
Published online: 27 May 2011.
Edited by:
Leon Y. Deouell, The Hebrew University of Jerusalem, IsraelReviewed by:
Erich Schröger, University of Leipzig, GermanySara L. Gonzalez Andino, HUG, Switzerland
Copyright: © 2011 Colder. This is an open-access article subject to a non-exclusive license between the authors and Frontiers Media SA, which permits use, distribution and reproduction in other forums, provided the original authors and source are credited and other Frontiers conditions are complied with.
*Correspondence: Brian Colder, Colder Scientific, 1553 Mary Ellen Ct, McLean, VA 22101, USA. e-mail:YnJpYW5AY29sZGVyc2NpZW50aWZpYy5jb20=